Introduction to T Tests PDF
Document Details
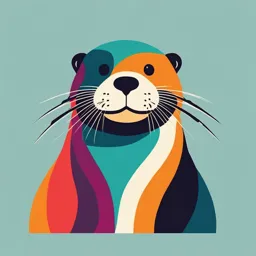
Uploaded by PrizeRetinalite5305
National Teachers College
Tags
Summary
This document introduces t-tests, a family of statistical tests used in behavioral science research. It explains the concept of t-tests, its applications, and the assumptions behind this test, while also highlighting the difference between T and Z scores and the influence of sample size and variance. It explains why a large variance is bad for inferential statistics and how a large sample size tends to produce bigger t-statistics, leading to more significant results. The document concludes by discussing how to report the results of a t-test and the importance of including effect size in the report.
Full Transcript
Introduction to t Statistics Psychological Statistics Lesson Objectives Discuss how t statistics is conducted Compute for t statistics using softwares Interpret statistical data...
Introduction to t Statistics Psychological Statistics Lesson Objectives Discuss how t statistics is conducted Compute for t statistics using softwares Interpret statistical data analyzed using the three different t statistics. What are the t statistics? In this part, we begin to introduce some of the inferential procedures that are actually used in behavioral science research. Specifically, we look at a family of t statistics that use sample means and mean differences to draw inferences about the corresponding population means and mean differences. ○ However, the t statistics do not require any prior knowledge about the population being evaluated. What are the t statistics? The three t statistics introduced apply to three distinct research situations: ○ Using a single sample to draw an inference about the unknown mean for a single population. (t test for single samples) ○ Using two separate samples to draw an inference about the mean difference between two unknown populations. (t test for independent samples) ○ Using one sample, with each individual tested in two different treatment conditions, to draw an inference about the population mean difference between the two conditions. (t test for dependent/paired samples) THE PROBLEM WITH z-SCORES Specifically, a z-score requires that we know the value of the population standard deviation (or variance), which is needed to compute the standard error. ○ In most situations, however, the standard deviation for the population is not known. You want to use a z-score to find out about an unknown population, but you must know about the population before you can compute a z-score. T statistic - an alternative to z scores INTRODUCING THE t STATISTIC INTRODUCING THE t STATISTIC DEGREES OF FREEDOM AND THE t STATISTIC Reviewing briefly, you must know the sample mean before you can compute sample variance. ○ This places a restriction on sample variability such that only n – 1 scores in a sample are independent and free to vary. ○ The value n – 1 is called the degrees of freedom (or df) for the sample variance. The greater the value of df for a sample, the better the sample variance, represents the population variance, and the better the t statistic approximates the z-score. This should make sense because the larger the sample (n) is, the better the sample represents its population. HYPOTHESIS TESTS WITH THE t STATISTIC HYPOTHESIS TESTS WITH THE t STATISTIC In the hypothesis-testing situation, we begin with a population with an unknown mean and an unknown variance, often a population that has received some treatment. The goal is to use a sample from the treated population (a treated sample) as the basis for determining whether the treatment has any effect. HYPOTHESIS TESTS WITH THE t STATISTIC As always, the null hypothesis states that the treatment has no effect; specifically, H0 states that the population mean is unchanged. ○ Thus, the null hypothesis provides a specific value for the unknown population mean. HYPOTHESIS TESTS WITH THE t STATISTIC it also permits hypothesis testing in situations for which you do not have a known population mean to serve as a standard. ○ Specifically, the t test does not require any prior knowledge about the population mean or the population variance. All you need to compute a t statistic is a null hypothesis and a sample from the unknown population. ○ Thus, a t test can be used in situations for which the null hypothesis is obtained from a theory, a logical prediction, or just wishful thinking. ASSUMPTIONS OF THE t TEST ASSUMPTIONS OF THE t TEST The values in the sample must consist of independent observations. The population that is sampled must be normal. ○ However, violating this assumption has little practical effect on the results obtained for a t statistic, especially when the sample size is relatively large. With very small samples, a normal population distribution is important. THE INFLUENCE OF SAMPLE SIZE AND SAMPLE VARIANCE THE INFLUENCE OF SAMPLE SIZE AND SAMPLE VARIANCE The estimated standard error is directly related to the sample variance so that the larger the variance, the larger the error. ○ Thus, large variance means that you are less likely to obtain a significant treatment effect. In general, large variance is bad for inferential statistics. ○ Large variance means that the scores are widely scattered, which makes it difficult to see any consistent patterns or trends in the data. In general, high variance reduces the likelihood of rejecting the null hypothesis. THE INFLUENCE OF SAMPLE SIZE AND SAMPLE VARIANCE If all other factors are held constant, large samples tend to produce bigger t statistics and therefore are more likely to produce significant results. ○ For example, a 2-point mean difference with a sample of n = 4 may not be convincing evidence of a treatment effect. ○ However, the same 2-point difference with a sample of n = 100 is much more compelling. MEASURING EFFECT SIZE FOR THE t STATISTIC A hypothesis test simply determines whether the treatment effect is greater than chance, where “chance” is measured by the standard error. ○ In particular, it is possible for a very small treatment effect to be “statistically significant,” especially when the sample size is very large. ○ To correct for this problem, it is recommended that the results from a hypothesis test be accompanied by a report of effect size, such as Cohen’s d. MEASURING EFFECT SIZE FOR THE t STATISTIC REPORTING THE RESULTS OF A t TEST REPORTING THE RESULTS OF A t TEST. First, recall that a scientific report typically uses the term significant to indicate that the null hypothesis has been rejected and the term not significant to indicate failure to reject H0. Additionally, there is a prescribed format for reporting the calculated value of the test statistic, degrees of freedom, and alpha level for a t test. REPORTING THE RESULTS OF A t TEST The first statement reports the descriptive statistics, the mean (M = 13) and the standard deviation (SD = 3), The next statement provides the results of the inferential statistical analysis. Note that the degrees of freedom are reported in parentheses immediately after the symbol t. The value for the obtained t statistic follows (3.00), and next is the probability of committing a Type I error (less than 5%). Finally, the effect size is reported, Lesson Objectives Discuss how t statistics is conducted Compute for t statistics using softwares Interpret statistical data analyzed using the three different t statistics.