Basic Business Statistics Concepts and Applications 14th Edition - PDF
Document Details
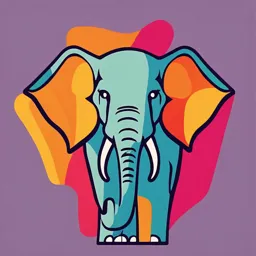
Uploaded by ImpeccableCitrine6447
Montclair State University
2020
Mark L. Berenson, David M. Levine, Kathryn A. Szabat, David F. Stephan
Tags
Summary
This book is a textbook on basic business statistics, providing concepts and applications. The 14th edition, global edition, covers a range of statistical methods for analyzing data. The book focuses on using the concepts in real-world business settings.
Full Transcript
Untitled-1 1 27/02/2019 21:19 Career Readiness Preparing students for careers is a priority. Now with the Open in Excel functionality in homework problems, students can open data sets directly in Excel and gain experience with the tools they will use in their careers. Bring Statistics to Life Us...
Untitled-1 1 27/02/2019 21:19 Career Readiness Preparing students for careers is a priority. Now with the Open in Excel functionality in homework problems, students can open data sets directly in Excel and gain experience with the tools they will use in their careers. Bring Statistics to Life Using the StatCrunch Twitter app you can see what people are tweeting about in real time. You can easily load tweets into StatCrunch and construct an interactive word wall showing the most commonly used words. Visit pearson.com/myLab/statistics and click Get Trained to make sure you’re getting the most out of MyLab Statistics. A Roadmap for Selecting a Statistical Method Data Analysis Task For Numerical Variables For Categorical Variables Describing a group or Ordered array, stem-and-leaf display, frequency Summary table, bar chart, pie chart, several groups distribution, relative frequency distribution, doughnut chart, Pareto chart percentage distribution, cumulative percentage (Sections 2.1 and 2.3) distribution, histogram, polygon, cumulative percentage polygon (Sections 2.2, 2.4) Mean, median, mode, geometric mean, quartiles, range, interquartile range, standard deviation, variance, coefficient of variation, skewness, kurtosis, boxplot, normal probability plot (Sections 3.1, 3.2, 3.3, 6.3) Index numbers (online Section 16.8) Dashboards (Section 17.2) Inference about one Confidence interval estimate of the mean Confidence interval estimate of the group (Sections 8.1 and 8.2) proportion (Section 8.3) t test for the mean (Section 9.2) Z test for the proportion Chi-square test for a variance or standard deviation (Section 9.4) (online Section 12.7) Comparing two groups Tests for the difference in the means of two Z test for the difference between two independent populations (Section 10.1) proportions (Section 10.3) Wilcoxon rank sum test (Section 12.4) Chi-square test for the difference between Paired t test (Section 10.2) two proportions (Section 12.1) F test for the difference between two variances McNemar test for two related (Section 10.4) samples (online Section 12.6) Wilcoxon signed ranks test (online Section 12.8) Comparing more than One-way analysis of variance for comparing Chi-square test for differences two groups several means (Section 11.1) among more than two proportions Kruskal-Wallis test (Section 12.5) (Section 12.2) Randomized block design (online Section 11.3) Two-way analysis of variance (Section 11.2) Friedman rank test (online Section 12.9) Analyzing the Scatter plot, time series plot (Section 2.5) Contingency table, side-by-side bar relationship between Covariance, coefficient of correlation (Section 3.5) chart, PivotTables two variables (Sections 2.1, 2.3, 2.6) Simple linear regression (Chapter 13) Chi-square test of independence t test of correlation (Section 13.7) (Section 12.3) Time-series forecasting (Chapter 16) Sparklines (Section 2.7) Analyzing the Colored scatter plots, bubble chart, treemap Multidimensional contingency tables relationship between (Section 2.7) (Section 2.6) two or more variables Multiple regression (Chapters 14 and 15) Drilldown and slicers (Section 2.7) Dynamic bubble charts (Section 17.2) Logistic regression (Section 14.7) Regression trees (Section 17.3) Classification trees (Section 17.4) Cluster analysis (Section 17.5) Multiple correspondence analysis Multidimensional scaling (Section 17.6) (Section 17.6) Basic Business Statistics Concepts and Applications This page intentionally left blank A01_THOM6233_05_SE_WALK.indd 9 1/13/17 6:50 PM Basic Business Statistics Concepts and Applications F O URT E E NT H E D IT IO N Glo b a l E d itio n Mark L. Berenson Department of Information Management and Business Analytics School of Business, Montclair State University David M. Levine Department of Information Systems and Statistics Zicklin School of Business, Baruch College, City University of New York Kathryn A. Szabat Department of Business Systems and Analytics School of Business, La Salle University David F. Stephan Two Bridges Instructional Technology Harlow, England London New York Boston San Francisco Toronto Sydney Dubai Singapore Hong Kong Tokyo Seoul Taipei New Delhi Cape Town Sao Paulo Mexico City Madrid Amsterdam Munich Paris Milan Director, Portfolio Management: Marcia Horton Product Marketing Manager: Kaylee Carlson Editor in Chief: Deirdre Lynch Product Marketing Assistant: Shannon McCormack Courseware Portfolio Manager: Suzanna Bainbridge Field Marketing Manager: Thomas Hayward Courseware Portfolio Management Assistant: Morgan Danna Field Marketing Assistant: Derrica Moser Associate Editor, Global Edition: Ananya Srivastava Media Manager, Global Edition: Vikram Kumar Assistant Editor, Global Edition: Prerna Grewal Senior Author Support/Technology Specialist: Joe Vetere Managing Producer: Karen Wernholm Manager, Rights and Permissions: Gina Cheselka Content Producer: Sherry Berg and Dana Bettez Manufacturing Buyer: Carol Melville, LSC Communications Senior Producer: Stephanie Green Manufacturing Controller, Global Edition: Kay Holman Associate Content Producer: Sneh Singh Composition and Production Coordination: Pearson CSC Manager, Courseware QA: Mary Durnwald Text Design: Cenveo Publisher Services Manager, Content Development: Robert Carroll Senior Designer and Cover Design: Barbara T. Atkinson Content Producer, Global Edition: Nitin Shankar Cover Image: J.D.S./Shutterstock Microsoft® Windows®, and Microsoft office® are registered trademarks of the Microsoft Corporation in the U.S.A. and other countries. This book is not sponsored or endorsed by or affiliated with the Microsoft Corporation. Microsoft and/or its respective suppliers make no representations about the suitability of the information contained in the documents and related graphics published as part of the services for any purpose. All such documents and related graphics are provided “as is” without warranty of any kind. Microsoft and/or its respective suppliers hereby disclaim all warranties and conditions with regard to this information, including all warranties and conditions of merchantability, whether express, implied or statutory, fitness for a particular purpose, title and non-infringement. In no event shall microsoft and/or its respective suppliers be liable for any special, indirect or consequential damages or any damages whatsoever resulting from loss of use, data or profits, whether in an action of contract, negligence or other tortious action, arising out of or in connection with the use or performance of information available from the services. The documents and related graphics contained herein could include technical inaccuracies or typographical errors. Changes are periodically added to the information herein. Microsoftand/or its respective suppliers may make improvements and/or changes in the product(s) and/or the program(s) described herein at any time. Partial screen shots may be viewed in full within the software version specified. Pearson Education Limited KAO Two KAO Park Harlow CM17 9NA United Kingdom and Associated Companies throughout the world Visit us on the World Wide Web at: www.pearsonglobaleditions.com © Pearson Education Limited 2020 The rights of Mark L. Berenson, David M. Levine, Kathryn A. Szabat, and David F. Stephan to be identified as the authors of this work have been asserted by them in accordance with the Copyright, Designs and Patents Act 1988. Authorized adaptation from the United States edition, entitled Basic Business Statistics: Concepts and Applications, 14th Edition, ISBN 978-0-13-468484-0 by Mark L. Berenson, David M. Levine, Kathryn A. Szabat, and David F. Stephan, published by Pearson Education © 2019. All rights reserved. No part of this publication may be reproduced, stored in a retrieval system, or transmitted in any form or by any means, electronic, mechanical, photocopying, recording or otherwise, without either the prior written permission of the publisher or a license permitting restricted copying in the United Kingdom issued by the Copyright Licensing Agency Ltd, Saffron House, 6–10 Kirby Street, London EC1N 8TS. All trademarks used herein are the property of their respective owners. The use of any trademark in this text does not vest in the author or publisher any trademark ownership rights in such trademarks, nor does the use of such trademarks imply any affiliation with or endorsement of this book by such owners. ISBN 10: 1-292-26503-5 ISBN 13: 978-1-292-26503-2 eBook ISBN 13: 978-1-292-26513-1 British Library Cataloguing-in-Publication Data A catalogue record for this book is available from the British Library 10 9 8 7 6 5 4 3 2 1 Typeset in Times LT Pro by Pearson CSC Printed and bound by Vivar in Malaysia A01_BERE5032_14_GE_FM.indd 6 04/03/2019 14:43 To our spouses and children, Rhoda, Marilyn, Mary, Kathy, Lori, Sharyn, and Mark and to our parents, in loving memory, Nat, Ethel, Lee, Reuben, Mary, William, Ruth and Francis J. About the Authors Mark L. Berenson, David M. Levine, Kathryn A. Szabat, and David F. Stephan are all experienced business school educators committed to innovation and improving instruction in business sta- tistics and related subjects. Mark L. Berenson is Professor of Information Management and Business Analytics at Montclair State University and also Professor Emeritus of Information Systems and Statistics at Baruch College. He currently teaches graduate and undergraduate courses in statis- tics and in operations management in the School of Business and an undergraduate course in international justice and human rights that he co-developed in the College of Humanities and Social Sciences. Kathryn Szabat, David Levine, Mark Berenson, and David Stephan Berenson received a B.A. in economic statistics and an M.B.A. in business statistics from City College of New York and a Ph.D. in business from the City University of New York. Berenson’s research has been published in Decision Sciences Journal of Innovative Education, Review of Business Research, The American Statistician, Communications in Statistics, Psychometrika, Educational and Psychological Measurement, Journal of Management Sciences and Applied Cybernetics, Research Quarterly, Stats Magazine, The New York Statistician, Journal of Health Administration Education, Journal of Behavioral Medicine, and Journal of Surgical Oncology. His invited articles have appeared in The Encyclopedia of Measurement & Statistics and Encyclopedia of Statistical Sciences. He has coauthored numerous statistics texts published by Pearson. Over the years, Berenson has received several awards for teaching and for innovative contributions to statistics education. In 2005, he was the first recipient of the Catherine A. Becker Service for Educational Excellence Award at Montclair State University and, in 2012, he was the recipient of the Khubani/Telebrands Faculty Research Fellowship in the School of Business. David Levine, Professor Emeritus of Statistics and CIS at Baruch College, CUNY, is a nationally recognized innovator in statistics education for more than three decades. Levine has coauthored 14 books, including several business statistics textbooks; textbooks and professional titles that explain and explore quality management and the Six Sigma approach; and, with David Stephan, a trade paperback that explains statistical concepts to a general audience. Levine has presented or chaired numerous sessions about business education at leading conferences conducted by the Decision Sciences Institute (DSI) and the American Statistical Association, and he and his coauthors have been active participants in the annual DSI Data, Analytics, and Statistics Instruction (DASI) mini-conference. During his many years teaching at Baruch College, Levine was recognized for his contributions to teaching and curriculum development with the College’s highest distinguished teaching honor. He earned B.B.A. and M.B.A. degrees from CCNY. and a Ph.D. in industrial engi- neering and operations research from New York University. As Associate Professor of Business Systems and Analytics at La Salle University, Kathryn Szabat has transformed several business school majors into one interdisciplinary major that better sup- ports careers in new and emerging disciplines of data analysis including analytics. Szabat strives to inspire, stimulate, challenge, and motivate students through innovation and curricular enhance- ments, and shares her coauthors’ commitment to teaching excellence and the continual improvement of statistics presentations. Beyond the classroom she has provided statistical advice to numerous business, nonbusiness, and academic communities, with particular interest in the areas of education, medicine, and nonprofit capacity building. Her research activities have led to journal publications, chapters in scholarly books, and conference presentations. Szabat is a member of the American Statistical Association (ASA), DSI, Institute for Operation Research and Management Sciences (INFORMS), and DSI DASI. She received a B.S. from SUNY-Albany, an M.S. in statistics from the Wharton School of the University of Pennsylvania, and a Ph.D. degree in statistics, with a cognate in operations research, from the Wharton School of the University of Pennsylvania. 8 ABOUT THE AUTHORS 9 Advances in computing have always shaped David Stephan’s professional life. As an under- graduate, he helped professors use statistics software that was considered advanced even though it could compute only several things discussed in Chapter 3, thereby gaining an early appreciation for the benefits of using software to solve problems (and perhaps positively influencing his grades). An early advocate of using computers to support instruction, he developed a prototype of a main- frame-based system that anticipated features found today in Pearson’s MathXL and served as spe- cial assistant for computing to the Dean and Provost at Baruch College. In his many years teaching at Baruch, Stephan implemented the first computer-based classroom, helped redevelop the CIS curriculum, and, as part of a FIPSE project team, designed and implemented a multimedia learning environment. He was also nominated for teaching honors. Stephan has presented at SEDSI and DSI DASI (formerly MSMESB) mini-conferences, sometimes with his coauthors. Stephan earned a B.A. from Franklin & Marshall College and an M.S. from Baruch College, CUNY, and completed the instructional technology graduate program at Teachers College, Columbia University. For all four coauthors, continuous improvement is a natural outcome of their curiosity about the world. Their varied backgrounds and many years of teaching experience have come together to shape this book in ways discussed in the Preface. This page intentionally left blank A01_THOM6233_05_SE_WALK.indd 9 1/13/17 6:50 PM Brief Contents Preface 24 First Things First 33 1 Defining and Collecting Data 48 2 Organizing and Visualizing Variables 73 3 Numerical Descriptive Measures 152 4 Basic Probability 200 5 Discrete Probability Distributions 231 6 The Normal Distribution and Other Continuous Distributions 255 7 Sampling Distributions 284 8 Confidence Interval Estimation 307 9 Fundamentals of Hypothesis Testing: One-Sample Tests 343 10 Two-Sample Tests 383 11 Analysis of Variance 430 12 Chi-Square and Nonparametric Tests 472 13 Simple Linear Regression 516 14 Introduction to Multiple Regression 568 15 Multiple Regression Model Building 624 16 Time-Series Forecasting 661 17 Business Analytics 710 18 Getting Ready to Analyze Data in the Future 736 19 Statistical Applications in Quality Management (online) 19-1 20 Decision Making (online) 20-1 Appendices A-H 743 Self-Test Solutions and Answers to Selected Even-Numbered Problems 793 Index 825 Credits 837 11 This page intentionally left blank A01_THOM6233_05_SE_WALK.indd 9 1/13/17 6:50 PM Contents Preface 24 1.3 Types of Sampling Methods 53 Simple Random Sample 54 Systematic Sample 54 First Things First 33 Stratified Sample 55 Cluster Sample 55 USING STATISTICS: “The Price of Admission” 33 1.4 Data Cleaning 56 Invalid Variable Values 57 FTF.1 Think Differently About Statistics 34 Coding Errors 57 Statistics: A Way of Thinking 34 Data Integration Errors 57 Statistics: An Important Part of Your Business Education 35 Missing Values 58 FTF.2 Business Analytics: The Changing Face of Statistics 36 Algorithmic Cleaning of Extreme Numerical Values 58 “Big Data” 36 1.5 Other Data Preprocessing Tasks 58 FTF.3 Starting Point for Learning Statistics 37 Data Formatting 58 Statistic 37 Stacking and Unstacking Data 59 Can Statistics (pl., statistic) Lie? 38 Recoding Variables 59 FTF.4 Starting Point for Using Software 38 Using Software Properly 40 1.6 Types of Survey Errors 60 REFERENCES 41 Coverage Error 60 KEY TERMS 41 Nonresponse Error 60 EXCEL GUIDE 42 Sampling Error 60 EG.1 Getting Started with Excel 42 Measurement Error 61 EG.2 Entering Data 42 Ethical Issues About Surveys 61 EG.3 Open or Save a Workbook 42 CONSIDER THIS: New Media Surveys/Old Survey EG.4 Working with a Workbook 43 Errors 61 EG.5 Print a Worksheet 43 EG.6 Reviewing Worksheets 43 USING STATISTICS: Defining Moments, Revisited 63 EG.7 If You Use the Workbook Instructions 43 SUMMARY 63 JMP GUIDE 44 REFERENCES 63 JG.1 Getting Started with JMP 44 KEY TERMS 63 JG.2 Entering Data 45 CHECKING YOUR UNDERSTANDING 64 JG.3 Create New Project or Data Table 45 JG.4 Open or Save Files 45 CHAPTER REVIEW PROBLEMS 64 JG.5 Print Data Tables or Report Windows 45 CASES FOR CHAPTER 1 65 JG.6 JMP Script Files 45 Managing Ashland MultiComm Services 65 MINITAB GUIDE 46 MG.1 Getting Started with Minitab 46 CardioGood Fitness 65 MG.2 Entering Data 46 Clear Mountain State Student Survey 66 MG.3 Open or Save Files 46 Learning with the Digital Cases 66 MG.4 Insert or Copy Worksheets 47 MG.5 Print Worksheets 47 CHAPTER 1 EXCEL GUIDE 67 EG1.1 Defining Variables 67 EG1.2 Collecting Data 67 1 Defining and Collecting Data 48 EG1.3 Types of Sampling Methods 67 EG1.4 Data Cleaning 68 EG1.5 Other Data Preprocessing 68 USING STATISTICS: Defining Moments 48 1.1 Defining Variables 49 CHAPTER 1 JMP GUIDE 69 Classifying Variables by Type 49 JG1.1 Defining Variables 69 Measurement Scales 50 JG1.2 Collecting Data 69 1.2 Collecting Data 51 JG1.3 Types of Sampling Methods 69 Populations and Samples 52 JG1.4 Data Cleaning 70 Data Sources 52 JG1.5 Other Preprocessing Tasks 71 13 14 CONTENTS CHAPTER 1 MINITAB GUIDE 71 CASES FOR CHAPTER 2 120 MG1.1 Defining Variables 71 Managing Ashland MultiComm Services 120 MG1.2 Collecting Data 71 Digital Case 120 MG1.3 Types of Sampling Methods 71 CardioGood Fitness 121 MG1.4 Data Cleaning 72 The Choice Is Yours Follow-Up 121 MG1.5 Other Preprocessing Tasks 72 Clear Mountain State Student Survey 121 CHAPTER 2 EXCEL GUIDE 122 2 Organizing and Visualizing EG2.1 Organizing Categorical Variables 122 EG2.2 Organizing Numerical Variables 124 Variables 73 EG2 Charts Group Reference 126 EG2.3 Visualizing Categorical Variables 126 USING STATISTICS: “The Choice Is Yours” 73 EG2.4 Visualizing Numerical Variables 128 2.1 Organizing Categorical Variables 74 EG2.5 Visualizing Two Numerical Variables 131 The Summary Table 74 EG2.6 Organizing a Mix of Variables 132 The Contingency Table 75 EG2.7 Visualizing a Mix of Variables 133 EG2.8 Filtering and Querying Data 134 2.2 Organizing Numerical Variables 78 The Frequency Distribution 79 CHAPTER 2 JMP GUIDE 134 Classes and Excel Bins 81 JG2 JMP Choices for Creating Summaries 134 The Relative Frequency Distribution and the JG2.1 Organizing Categorical Variables 135 Percentage Distribution 81 JG2.2 Organizing Numerical Variables 136 The Cumulative Distribution 83 JG2.3 Visualizing Categorical Variables 138 2.3 Visualizing Categorical Variables 86 JG2.4 Visualizing Numerical Variables 139 The Bar Chart 86 JG2.5 Visualizing Two Numerical Variables 141 The Pie Chart and the Doughnut Chart 87 JG2.6 Organizing a Mix of Variables 142 The Pareto Chart 88 JG2.7 Visualizing a Mix of Variables 142 Visualizing Two Categorical Variables 90 JG2.8 Filtering and Querying Data 143 JMP Guide Gallery 144 2.4 Visualizing Numerical Variables 93 The Stem-and-Leaf Display 93 CHAPTER 2 MINITAB GUIDE 145 The Histogram 93 MG2.1 Organizing Categorical Variables 145 The Percentage Polygon 95 MG2.2 Organizing Numerical Variables 145 The Cumulative Percentage Polygon (Ogive) 96 MG2.3 Visualizing Categorical Variables 145 MG2.4 Visualizing Numerical Variables 147 2.5 Visualizing Two Numerical Variables 99 MG2.5 Visualizing Two Numerical Variables 149 The Scatter Plot 99 MG2.6 Organizing a Mix of Variables 150 The Time-Series Plot 100 MG2.7 Visualizing a Mix of Variables 150 2.6 Organizing a Mix of Variables 102 MG2.8 Filtering and Querying Data 151 Drill-down 103 2.7 Visualizing a Mix of Variables 104 Colored Scatter Plot 104 3 Numerical Descriptive Bubble Charts 105 Measures 152 PivotChart (Excel) 105 Treemap (Excel, JMP) 105 USING STATISTICS: More Descriptive Choices 152 Sparklines (Excel) 106 3.1 Measures of Central Tendency 153 2.8 Filtering and Querying Data 107 The Mean 153 Excel Slicers 107 The Median 155 2.9 Pitfalls in Organizing and Visualizing Variables 109 The Mode 156 Obscuring Data 109 The Geometric Mean 157 Creating False Impressions 110 3.2 Measures of Variation and Shape 158 Chartjunk 111 The Range 158 EXHIBIT: Best Practices for Creating Visual Summaries 112 The Variance and the Standard Deviation 159 USING STATISTICS: “The Choice Is Yours,” Revisited 113 The Coefficient of Variation 162 SUMMARY 113 Z Scores 162 REFERENCES 114 Shape: Skewness 164 Shape: Kurtosis 164 KEY EQUATIONS 114 3.3 Exploring Numerical Variables 169 KEY TERMS 115 Quartiles 169 CHECKING YOUR UNDERSTANDING 115 EXHIBIT: Rules for Calculating the Quartiles from a Set CHAPTER REVIEW PROBLEMS 115 of Ranked Values 169 CONTENTS 15 The Interquartile Range 171 4.2 Conditional Probability 210 The Five-Number Summary 171 Computing Conditional Probabilities 210 The Boxplot 173 Decision Trees 211 3.4 Numerical Descriptive Measures for a Population 175 Independence 213 The Population Mean 176 Multiplication Rules 214 The Population Variance and Standard Deviation 176 Marginal Probability Using the General Multiplication Rule 215 The Empirical Rule 177 4.3 Ethical Issues and Probability 217 Chebyshev’s Theorem 178 4.4 Bayes’ Theorem 218 3.5 The Covariance and the Coefficient of Correlation 180 CONSIDER THIS: Divine Providence and Spam 220 The Covariance 180 The Coefficient of Correlation 180 4.5 Counting Rules 221 3.6 Descriptive Statistics: Pitfalls and Ethical Issues 184 USING STATISTICS: Possibilities at M&R Electronics World, Revisited 224 USING STATISTICS: More Descriptive Choices, Revisited 185 SUMMARY 225 SUMMARY 185 REFERENCES 225 REFERENCES 186 KEY EQUATIONS 225 KEY EQUATIONS 186 KEY TERMS 226 KEY TERMS 186 CHECKING YOUR UNDERSTANDING 226 CHECKING YOUR UNDERSTANDING 187 CHAPTER REVIEW PROBLEMS 226 CHAPTER REVIEW PROBLEMS 187 CASES FOR CHAPTER 4 228 CASES FOR CHAPTER 3 190 Digital Case 228 Managing Ashland MultiComm Services 190 CardioGood Fitness 228 Digital Case 190 The Choice Is Yours Follow-Up 228 CardioGood Fitness 190 Clear Mountain State Student Survey 228 More Descriptive Choices Follow-up 191 CHAPTER 4 EXCEL GUIDE 229 Clear Mountain State Student Survey 191 EG4.1 Basic Probability Concepts 229 CHAPTER 3 EXCEL GUIDE 192 EG4.4 Bayes’ Theorem 229 EG3.1 Measures of Central Tendency 192 EG4.5 Counting Rules 229 EG3.2 Measures of Variation and Shape 193 EG3.3 Exploring Numerical Variables 193 CHAPTER 4 JMP EG3.4 Numerical Descriptive Measures for a Population 194 JG4.4 Bayes’ Theorem 230 EG3.5 The Covariance and the Coefficient of Correlation 194 CHAPTER 4 MINITAB GUIDE 230 CHAPTER 3 JMP GUIDE 195 MG4.5 Counting Rules 230 JG3.1 Measures of Central Tendency 195 JG3.2 Measures of Variation and Shape 195 JG3.3 Exploring Numerical Variables 196 5 Discrete Probability JG3.4 Numerical Descriptive Measures for a Population 196 Distributions 231 JG3.5 The Covariance and the Coefficient of Correlation 196 CHAPTER 3 MINITAB GUIDE 197 USING STATISTICS: Events of Interest at Ricknel MG3.1 Measures of Central Tendency 197 Home Centers 231 MG3.2 Measures of Variation and Shape 198 5.1 The Probability Distribution for a Discrete Variable 232 MG3.3 Exploring Numerical Variables 198 Expected Value of a Discrete Variable 232 MG3.4 Numerical Descriptive Measures for a Population 199 MG3.5 The Covariance and the Coefficient of Correlation 199 Variance and Standard Deviation of a Discrete Variable 233 5.2 Binomial Distribution 236 EXHIBIT: Properties of the Binomial Distribution 236 4 Basic Probability 200 Histograms for Discrete Variables 239 Summary Measures for the Binomial Distribution 240 USING STATISTICS: Possibilities at M&R Electronics 5.3 Poisson Distribution 243 World 200 5.4 Covariance of a Probability Distribution and Its 4.1 Basic Probability Concepts 201 Application in Finance 246 Events and Sample Spaces 201 5.5 Hypergeometric Distribution (online) 246 Types of Probability 202 5.6 Using the Poisson Distribution to Approximate the Summarizing Sample Spaces 203 Binomial Distribution (online) 246 Simple Probability 204 Joint Probability 205 USING STATISTICS: Events of Interest … , Revisited 247 Marginal Probability 206 SUMMARY 247 General Addition Rule 206 REFERENCES 247 16 CONTENTS KEY EQUATIONS 247 CASES FOR CHAPTER 6 278 KEY TERMS 248 Managing Ashland MultiComm Services 278 CHECKING YOUR UNDERSTANDING 248 CardioGood Fitness 279 CHAPTER REVIEW PROBLEMS 248 More Descriptive Choices Follow-up 279 Clear Mountain State Student Survey 279 CASES FOR CHAPTER 5 250 Digital Case 279 Managing Ashland MultiComm Services 250 Digital Case 250 CHAPTER 6 EXCEL GUIDE 280 EG6.2 The Normal Distribution 280 CHAPTER 5 EXCEL GUIDE 251 EG6.3 Evaluating Normality 280 EG5.1 The Probability Distribution for a Discrete Variable 251 EG5.2 Binomial Distribution 251 CHAPTER 6 JMP GUIDE 281 EG5.3 Poisson Distribution 251 JG6.2 The Normal Distribution 281 JG6.3 Evaluating Normality 281 CHAPTER 5 JMP GUIDE 252 JG5.1 The Probability Distribution for a Discrete Variable 252 CHAPTER 6 MINITAB GUIDE 282 JG5.2 Binomial Distribution 252 MG6.2 The Normal Distribution 282 JG5.3 Poisson Distribution 253 MG6.3 Evaluating Normality 282 CHAPTER 5 MINITAB GUIDE 253 MG5.1 The Probability Distribution for a Discrete Variable 253 MG5.2 Binomial Distribution 254 MG5.3 Poisson Distribution 254 7 Sampling Distributions 284 USING STATISTICS: Sampling Oxford Cereals 284 6 The Normal Distribution 7.1 Sampling Distributions 285 and Other Continuous 7.2 Sampling Distribution of the Mean 285 Distributions 255 The Unbiased Property of the Sample Mean 285 Standard Error of the Mean 287 USING STATISTICS: Normal Load Times at MyTVLab 255 Sampling from Normally Distributed Populations 288 Sampling from Non-normally Distributed Populations— 6.1 Continuous Probability Distributions 256 The Central Limit Theorem 291 6.2 The Normal Distribution 256 EXHIBIT: Normality and the Sampling Distribution of the EXHIBIT: Normal Distribution Important Theoretical Mean 292 Properties 257 VISUAL EXPLORATIONS: Exploring Sampling Role of the Mean and the Standard Deviation 258 Distributions 295 Calculating Normal Probabilities 259 7.3 Sampling Distribution of the Proportion 296 VISUAL EXPLORATIONS: Exploring the Normal Distribution 263 7.4 Sampling from Finite Populations (online) 299 Finding X Values 264 USING STATISTICS: Sampling Oxford Cereals, Revisited 299 CONSIDER THIS: What Is Normal? 267 SUMMARY 300 6.3 Evaluating Normality 269 REFERENCES 300 Comparing Data Characteristics to Theoretical Properties 269 KEY EQUATIONS 300 Constructing the Normal Probability Plot 270 KEY TERMS 300 6.4 The Uniform Distribution 273 CHECKING YOUR UNDERSTANDING 301 6.5 The Exponential Distribution (online) 275 CHAPTER REVIEW PROBLEMS 301 6.6 The Normal Approximation to the Binomial CASES FOR CHAPTER 7 302 Distribution (online) 275 Managing Ashland MultiComm Services 302 USING STATISTICS: Normal Load Times … , Revisited 275 Digital Case 303 SUMMARY 275 CHAPTER 7 EXCEL GUIDE 304 REFERENCES 276 EG7.2 Sampling Distribution of the Mean 304 KEY EQUATIONS 276 CHAPTER 7 JMP GUIDE 305 KEY TERMS 276 JG7.2 Sampling Distribution of the Mean 305 CHECKING YOUR UNDERSTANDING 277 CHAPTER 7 MINITAB GUIDE 306 CHAPTER REVIEW PROBLEMS 277 MG7.2 Sampling Distribution of the Mean 306 CONTENTS 17 MG8.3 Confidence Interval Estimate for the Proportion 342 8 Confidence Interval MG8.4 Determining Sample Size 342 Estimation 307 USING STATISTICS: Getting Estimates at Ricknel 9 Fundamentals of Hypothesis Home Centers 307 Testing: One-Sample Tests 343 8.1 Confidence Interval Estimate for the Mean (s Known) 308 Sampling Error 309 USING STATISTICS: Significant Testing at Oxford Can You Ever Know the Population Standard Deviation? 312 Cereals 343 8.2 onfidence Interval Estimate for the Mean C 9.1 Fundamentals of Hypothesis Testing 344 (s Unknown) 313 EXHIBIT: Fundamental Hypothesis Testing Concepts 345 Student’s t Distribution 314 The Critical Value of the Test Statistic 345 The Concept of Degrees of Freedom 314 Regions of Rejection and Nonrejection 346 Properties of the t Distribution 314 Risks in Decision Making Using Hypothesis Testing 346 The Confidence Interval Statement 316 Z Test for the Mean (s Known) 348 8.3 Confidence Interval Estimate for the Proportion 321 Hypothesis Testing Using the Critical Value Approach 349 EXHIBIT: The Critical Value Approach to Hypothesis 8.4 Determining Sample Size 324 Testing 350 Sample Size Determination for the Mean 324 Hypothesis Testing Using the p-Value Approach 352 Sample Size Determination for the Proportion 326 EXHIBIT: The p-Value Approach to Hypothesis Testing 353 8.5 Confidence Interval Estimation and Ethical Issues 329 A Connection Between Confidence Interval Estimation 8.6 Application of Confidence Interval Estimation in and Hypothesis Testing 354 Auditing (online) 329 Can You Ever Know the Population Standard Deviation? 355 8.7 Estimation and Sample Size Estimation for Finite 9.2 t Test of Hypothesis for the Mean (s Unknown) 356 Populations (online) 330 The Critical Value Approach 357 8.8 Bootstrapping (online) 330 p-Value Approach 358 Checking the Normality Assumption 359 USING STATISTICS: Getting Estimates … , Revisited 330 9.3 One-Tail Tests 362 SUMMARY 330 The Critical Value Approach 362 REFERENCES 331 The p-Value Approach 363 KEY EQUATIONS 331 EXHIBIT: The Null and Alternative Hypotheses in One-Tail KEY TERMS 331 Tests 365 CHECKING YOUR UNDERSTANDING 331 9.4 Z Test of Hypothesis for the Proportion 366 CHAPTER REVIEW PROBLEMS 332 The Critical Value Approach 367 CASES FOR CHAPTER 8 334 The p-Value Approach 368 Managing Ashland MultiComm Services 334 9.5 Potential Hypothesis-Testing Pitfalls and Ethical Issues 370 Digital Case 335 EXHIBIT: Questions for the Planning Stage of Hypothesis Testing 370 Sure Value Convenience Stores 336 Statistical Significance Versus Practical Significance 370 CardioGood Fitness 336 Statistical Insignificance Versus Importance 371 More Descriptive Choices Follow-Up 336 Reporting of Findings 371 Clear Mountain State Student Survey 336 Ethical Issues 371 CHAPTER 8 EXCEL GUIDE 337 9.6 Power of the Test (online) 371 EG8.1 Confidence Interval Estimate for the Mean (s Known) 337 USING STATISTICS: Significant Testing … Revisited 372 EG8.2 Confidence Interval Estimate for the Mean (s Unknown) 337 SUMMARY 372 EG8.3 Confidence Interval Estimate for the Proportion 338 REFERENCES 372 EG8.4 Determining Sample Size 338 KEY EQUATIONS 373 CHAPTER 8 JMP GUIDE 339 KEY TERMS 373 JG8.1 Confidence Interval Estimate for the Mean (s Known) 339 JG8.2 Confidence Interval Estimate for the Mean (s Unknown) 339 CHECKING YOUR UNDERSTANDING 373 JG8.3 Confidence Interval Estimate for the Proportion 340 CHAPTER REVIEW PROBLEMS 373 JG8.4 Determining Sample Size 340 CASES FOR CHAPTER 9 375 CHAPTER 8 MINITAB GUIDE 341 Managing Ashland MultiComm Services 375 MG8.1 Confidence Interval Estimate for the Mean (s Known) 341 Digital Case 375 MG8.2 Confidence Interval Estimate for the Mean (s Unknown) 341 Sure Value Convenience Stores 376 18 CONTENTS CHPATER 9 EXCEL GUIDE 377 CHAPTER 10 EXCEL GUIDE 420 EG9.1 Fundamentals of Hypothesis Testing 377 EG10.1 Comparing the Means of Two Independent EG9.2 t Test of Hypothesis for the Mean (s Unknown) 377 Populations 420 EG9.3 One-Tail Tests 378 EG10.2 Comparing the Means of Two Related Populations 422 EG9.4 Z Test of Hypothesis for the Proportion 378 EG10.3 Comparing the Proportions of Two Independent Populations 423 CHAPTER 9 JMP GUIDE 379 EG10.4 F Test for the Ratio of Two Variances 424 JG9.1 Fundamentals of Hypothesis Testing 379 JG9.2 t Test of Hypothesis for the Mean (s Unknown) 379 CHAPTER 10 JMP GUIDE 425 JG9.3 One-Tail Tests 380 JG10.1 Comparing the Means of Two Independent Populations 425 JG9.4 Z Test of Hypothesis for the Proportion 380 JG10.2 Comparing the Means of Two Related Populations 426 JG10.3 Comparing the Proportions of Two Independent CHAPTER 9 MINITAB GUIDE 380 Populations 426 MG9.1 Fundamentals of Hypothesis Testing 380 JG10.4 F Test for the Ratio of Two Variances 426 MG9.2 t Test of Hypothesis for the Mean (s Unknown) 381 MG9.3 One-Tail Tests 381 CHAPTER 10 MINITAB GUIDE 427 MG10.1 Comparing the Means of Two Independent Populations 427 MG9.4 Z Test of Hypothesis for the Proportion 381 MG10.2 Comparing the Means of Two Related Populations 428 MG10.3 Comparing the Proportions of Two Independent Populations 428 10 Two-Sample Tests 383 MG10.4 F Test for the Ratio of Two Variances 429 USING STATISTICS: Differing Means for Selling Streaming Media Players at Arlingtons? 383 11 Analysis of Variance 430 10.1 Comparing the Means of Two Independent Populations 384 USING STATISTICS: The Means to Find Differences Pooled-Variance t Test for the Difference Between at Arlingtons 430 Two Means Assuming Equal Variances 384 11.1 The Completely Randomized Design: One-Way Evaluating the Normality Assumption 387 ANOVA 431 Confidence Interval Estimate for the Difference Between Analyzing Variation in One-Way ANOVA 432 Two Means 389 F Test for Differences Among More Than Two Means 434 Separate-Variance t Test for the Difference Between One-Way ANOVA F Test Assumptions 439 Two Means, Assuming Unequal Variances 390 Levene Test for Homogeneity of Variance 439 CONSIDER THIS: Do People Really Do This? 391 Multiple Comparisons: The Tukey-Kramer Procedure 441 10.2 Comparing the Means of Two Related Populations 393 The Analysis of Means (ANOM) 443 Paired t Test 394 11.2 The Factorial Design: Two-Way ANOVA 446 Confidence Interval Estimate for the Mean Difference 399 Factor and Interaction Effects 447 10.3 Comparing the Proportions of Two Independent Testing for Factor and Interaction Effects 448 Populations 401 Multiple Comparisons: The Tukey Procedure 452 Z Test for the Difference Between Two Proportions 401 Visualizing Interaction Effects: The Cell Means Plot 453 Confidence Interval Estimate for the Difference Between Interpreting Interaction Effects 454 Two Proportions 406 11.3 The Randomized Block Design (online) 458 10.4 F Test for the Ratio of Two Variances 408 11.4 Fixed Effects, Random Effects, and Mixed Effects 10.5 Effect Size (online) 412 Models (online) 458 USING STATISTICS: Differing Means for Selling … , USING STATISTICS: The Means to Find Differences Revisited 413 at Arlingtons Revisited 458 SUMMARY 413 SUMMARY 458 REFERENCES 414 REFERENCES 459 KEY EQUATIONS 414 KEY EQUATIONS 459 KEY TERMS 415 KEY TERMS 460 CHECKING YOUR UNDERSTANDING 415 CHECKING YOUR UNDERSTANDING 460 CHAPTER REVIEW PROBLEMS 415 CHAPTER REVIEW PROBLEMS 460 CASES FOR CHAPTER 10 417 CASES FOR CHAPTER 11 462 Managing Ashland MultiComm Services 417 Managing Ashland MultiComm Services 462 Digital Case 418 Digital Case 463 Sure Value Convenience Stores 418 Sure Value Convenience Stores 463 CardioGood Fitness 418 CardioGood Fitness 463 More Descriptive Choices Follow-Up 418 More Descriptive Choices Follow-Up 463 Clear Mountain State Student Survey 419 Clear Mountain State Student Survey 463 CONTENTS 19 CHAPTER 11 EXCEL GUIDE 464 EG12.3 Chi-Square Test of Independence 511 EG11.1 The Completely Randomized Design: One-Way ANOVA 464 EG12.4 Wilcoxon Rank Sum Test: A Nonparametric Method for EG11.2 The Factorial Design: Two-Way ANOVA 466 Two Independent Populations 511 EG12.5 Kruskal-Wallis Rank Test: A Nonparametric Method for CHAPTER 11 JMP GUIDE 467 the One-Way ANOVA 512 JG11.1 The Completely Randomized Design: One-Way ANOVA 467 JG11.2 The Factorial Design: Two-Way ANOVA 468 CHAPTER 12 JMP GUIDE 513 CHAPTER 11 MINITAB GUIDE 469 JG12.1 Chi-Square Test for the Difference Between Two Proportions 513 MG11.1 The Completely Randomized Design: One-Way ANOVA 469 JG12.2 Chi-Square Test tor Difference Among More Than MG11.2 The Factorial Design: Two-Way ANOVA 470 Two Proportions 513 JG12.3 Chi-Square Test Of Independence 513 JG12.4 Wilcoxon Rank Sum Test for Two Independent 12 Chi-Square and Populations 513 Nonparametric Tests 472 JG12.5 Kruskal-Wallis Rank Test for the One-Way ANOVA 514 CHAPTER 12 MINITAB GUIDE 514 USING STATISTICS: Avoiding Guesswork About MG12.1 Chi-Square Test for the Difference Between Resort Guests 472 Two Proportions 514 12.1 Chi-Square Test for the Difference Between Two MG12.2 Chi-Square Test for Differences Among More Than Two Proportions 515 Proportions 473 MG12.3 Chi-Square Test of Independence 515 12.2 Chi-Square Test for Differences Among More Than MG12.4 Wilcoxon Rank Sum Test: A Nonparametric Method Two Proportions 480 for Two Independent Populations 515 The Marascuilo Procedure 483 MG12.5 Kruskal-Wallis Rank Test: A Nonparametric Method The Analysis of Proportions (ANOP) 485 for the One-Way ANOVA 515 12.3 Chi-Square Test of Independence 486 12.4 Wilcoxon Rank Sum Test for Two Independent Populations 492 13 Simple Linear Regression 516 12.5 Kruskal-Wallis Rank Test for the One-Way ANOVA 498 Assumptions of the Kruskal-Wallis Rank Test 501 USING STATISTICS: Knowing Customers at Sunflowers 12.6 McNemar Test for the Difference Between Two Apparel 516 Proportions (Related Samples) (online) 502 Preliminary Analysis 517 12.7 Chi-Square Test for the Variance or Standard 13.1 Simple Linear Regression Models 518 Deviation (online) 502 13.2 Determining the Simple Linear Regression 12.8 Wilcoxon Signed Ranks Test for Two Related Equation 519 Populations (online) 503 The Least-Squares Method 519 12.9 Friedman Rank Test for the Randomized Block Predictions in Regression Analysis: Interpolation Versus Design (online) 503 Extrapolation 522 USING STATISTICS: Avoiding Guesswork … , Computing the Y Intercept, b0 and the Slope, b1 523 Revisited 503 VISUAL EXPLORATIONS: Exploring Simple Linear SUMMARY 503 Regression Coefficients 525 REFERENCES 504 13.3 Measures of Variation 527 KEY EQUATIONS 504 Computing the Sum of Squares 527 KEY TERMS 505 The Coefficient of Determination 528 CHECKING YOUR UNDERSTANDING 505 Standard Error of the Estimate 530 CHAPTER REVIEW PROBLEMS 505 13.4 Assumptions of Regression 532 CASES FOR CHAPTER 12 507 13.5 Residual Analysis 532 Managing Ashland MultiComm Services 507 Evaluating the Assumptions 532 Digital Case 508 13.6 Measuring Autocorrelation: The Durbin-Watson Sure Value Convenience Stores 508 Statistic 536 CardioGood Fitness 508 Residual Plots to Detect Autocorrelation 536 More Descriptive Choices Follow-Up 509 The Durbin-Watson Statistic 537 Clear Mountain State Student Survey 509 13.7 Inferences About the Slope and Correlation CHAPTER 12 EXCEL GUIDE 510 Coefficient 540 EG12.1 Chi-Square Test for the Difference Between t Test for the Slope 540 Two Proportions 510 F Test for the Slope 541 EG12.2 Chi-Square Test for Differences Among More Than Confidence Interval Estimate for the Slope 543 Two Proportions 510 t Test for the Correlation Coefficient 543 20 CONTENTS 13.8 Estimation of Mean Values and Prediction of 14.3 Multiple Regression Residual Analysis 578 Individual Values 546 14.4 Inferences About the Population Regression The Confidence Interval Estimate for the Mean Response 547 Coefficients 579 The Prediction Interval for an Individual Response 548 Tests of Hypothesis 580 13.9 Potential Pitfalls in Regression 550 Confidence Interval Estimation 581 EXHIBIT: Seven Steps for Avoiding the Potential Pitfalls 550 14.5 Testing Portions of the Multiple Regression Model 583 USING STATISTICS: Knowing Customers … , Revisited 552 Coefficients of Partial Determination 587 SUMMARY 553 14.6 Using Dummy Variables and Interaction Terms 589 REFERENCES 554 Interactions 592 KEY EQUATIONS 554 14.7 Logistic Regression 601 KEY TERMS 555 14.8 Influence Analysis (online) 607 CHECKING YOUR UNDERSTANDING 555 USING STATISTICS: The Multiple Effects … , Revisited 607 CHAPTER REVIEW PROBLEMS 556 SUMMARY 607 CASES FOR CHAPTER 13 559 REFERENCES 609 Managing Ashland MultiComm Services 559 KEY EQUATIONS 609 Digital Case 559 KEY TERMS 610 Rye Packaging 560 CHECKING YOUR UNDERSTANDING 610 CHAPTER 13 EXCEL GUIDE 561 CHAPTER REVIEW PROBLEMS 610 EG13.2 Determining the Simple Linear Regression Equation 561 CASES FOR CHAPTER 14 613 EG13.3 Measures of Variation 562 Managing Ashland MultiComm Services 613 EG13.4 Assumptions of Regression 562 EG13.5 Residual Analysis 562 Digital Case 613 EG13.6 Measuring Autocorrelation: the Durbin-Watson Statistic 563 CHAPTER 14 EXCEL GUIDE 614 EG13.7 Inferences about the Slope and Correlation Coefficient 563 EG14.1 Developing a Multiple Regression Model 614 EG13.8 Estimation of Mean Values and Prediction of Individual EG14.2 r 2, Adjusted r 2, and the Overall F Test 615 Values 563 EG14.3 Multiple Regression Residual Analysis 615 CHAPTER 13 JMP GUIDE 564 EG14.4 Inferences about the Population Regression Coefficients 616 JG13.2 Determining the Simple Linear Regression Equation 564 EG14.5 Testing Portions of the Multiple Regression Model 616 JG13.3 Measures of Variation 564 EG14.6 Using Dummy Variables and Interaction Terms 616 JG13.4 Assumptions of Regression 564 EG14.7 Logistic Regression 617 JG13.5 Residual Analysis 564 CHAPTER 14 JMP GUIDE 617 JG13.6 Measuring Autocorrelation: the Durbin-Watson Statistic 564 JG14.1 Developing a Multiple Regression Model 617 JG13.7 Inferences about the Slope and Correlation Coefficient 564 JG14.2 r 2, Adjusted r 2, and the Overall F Test Measures JG13.8 Estimation of Mean Values and Prediction of Individual of Variation 618 Values 565 JG14.3 Multiple Regression Residual Analysis 618 CHAPTER 13 MINITAB GUIDE 566 JG14.4 Inferences about the Population 618 MG13.2 Determining the Simple Linear Regression Equation 566 JG14.5 Testing Portions of the Multiple Regression Model 619 MG13.3 Measures of Variation 567 JG14.6 Using Dummy Variables and Interaction Terms 619 MG13.4 Assumptions of Regression 567 JG14.7 Logistic Regression 619 MG13.5 Residual Analysis 567 CHAPTER 14 MINITAB GUIDE 620 MG13.6 Measuring Autocorrelation: the Durbin-Watson Statistic 567 MG14.1 Developing a Multiple Regression Model 620 MG13.7 Inferences about the Slope and Correlation Coefficient 567 MG14.2 r 2, Adjusted r 2, and the Overall F Test 621 MG13.8 Estimation of Mean Values and Prediction of Individual MG14.3 Multiple Regression Residual Analysis 621 Values 567 MG14.4 Inferences about the Population Regression Coefficients 621 MG14.5 Testing Portions of the Multiple Regression Model 621 14 Introduction to Multiple MG14.6 Using Dummy Variables and Interaction Terms in Regression Models 621 Regression 568 MG14.7 Logistic Regression 622 MG14.8 Influence Analysis 623 USING STATISTICS: The Multiple Effects of OmniPower Bars 568 14.1 Developing a Multiple Regression Model 569 15 Multiple Regression Model Interpreting the Regression Coefficients 570 Predicting the Dependent Variable Y 572 Building 624 14.2 r 2, Adjusted r 2, and the Overall F Test 574 Coefficient of Multiple Determination 574 USING STATISTICS: Valuing Parsimony at WSTA-TV 624 Adjusted r 2 575 15.1 Quadratic Regression Model 625 Test for the Significance of the Overall Multiple Regression Finding the Regression Coefficients and Predicting Y 626 Model 575 Testing for the Significance of the Quadratic Model 628 CONTENTS 21 Testing the Quadratic Effect 629 EXHIBIT: Model Selection Using First, Second, and Percentage The Coefficient of Multiple Determination 631 Differences 674 15.2 Using Transformations in Regression Models 633 16.4 Autoregressive Modeling for Trend Fitting and The Square-Root Transformation 633 Forecasting 679 The Log Transformation 635 Selecting an Appropriate Autoregressive Model 680 15.3 Collinearity 637 Determining the Appropriateness of a Selected Model 681 15.4 Model Building 639 EXHIBIT: Autoregressive Modeling Steps 683 EXHIBIT: Sucessful Model Building 639 16.5 Choosing an Appropriate Forecasting Model 687 The Stepwise Regression Approach to Model Building 641 Residual Analysis 687 The Best Subsets Approach to Model Building 642 The Magnitude of the Residuals Through Squared Model Validation 645 or Absolute Differences 688 15.5 Pitfalls in Multiple Regression and Ethical Issues 647 The Principle of Parsimony 688 Pitfalls in Multiple Regression 647 A Comparison of Four Forecasting Methods 689 Ethical Issues 648 16.6 Time-Series Forecasting of Seasonal Data 691 USING STATISTICS: Valuing Parsimony … , Revisited 648 Least-Squares Forecasting with Monthly or Quarterly Data 691 SUMMARY 649 16.7 Index Numbers (online) 697 REFERENCES 650 KEY EQUATIONS 650 CONSIDER THIS: Let the Model User Beware 697 KEY TERMS 650 USING STATISTICS: Is the ByYourDoor … , Revisited 697 CHECKING YOUR UNDERSTANDING 650 SUMMARY 697 CHAPTER REVIEW PROBLEMS 650 REFERENCES 698 CASES FOR CHAPTER 15 652 KEY EQUATIONS 698 The Mountain States Potato Company 652 KEY TERMS 699 Sure Value Convenience Stores 653 CHECKING YOUR UNDERSTANDING 700 Digital Case 653 CHAPTER REVIEW PROBLEMS 700 The Craybill Instrumentation Company Case 653 CASES FOR CHAPTER 16 701 More Descriptive Choices Follow-Up 654 Managing Ashland MultiComm Services 701 CHAPTER 15 EXCEL GUIDE 655 Digital Case 701 EG15.1 The Quadratic Regression Model 655 CHAPTER 16 EXCEL GUIDE 702 EG15.2 Using Transformations in Regression Models 655 EG16.2 Smoothing an Annual Time Series 702 EG15.3 Collinearity 656 EG16.3 Least-Squares Trend Fitting and Forecasting 703 EG15.4 Model Building 656 EG16.4 Autoregressive Modeling for Trend Fitting and CHAPTER 15 JMP GUIDE 657 Forecasting 703 JG15.1 The Quadratic Regression Model 657 EG16.5 Choosing an Appropriate Forecasting Model 704 JG15.2 Using Transformations in Regression Models 657 EG16.6 Time-Series Forecasting of Seasonal Data 704 JG15.3 Collinearity 657 CHAPTER 16 JMP GUIDE 705 JG15.4 Model Building 657 JG16.2 Smoothing an Annual Time Series 705 CHAPTER 15 MINITAB GUIDE 658 JG16.3 Least-Squares Trend Fitting and Forecasting 706 MG15.1 The Quadratic Regression Model 658 JG16.4 Autoregressive Modeling for Trend Fitting and Forecasting 706 JG16.5 Choosing an Appropriate Forecasting Model 707 MG15.2 Using Transformations in Regression Models 659 JG16.6 Time-Series Forecasting of Seasonal Data 707 MG15.3 Collinearity 659 MG15.4 Model Building 659 CHAPTER 16 MINITAB GUIDE 707 MG16.2 Smoothing an Annual Time Series 707 MG16.3 Least-Squares Trend Fitting and Forecasting 708 16 Time-Series Forecasting 661 MG16.4 Autoregressive Modeling for Trend Fitting and Forecasting 709 MG16.5 Choosing an Appropriate Forecasting Model 709 USING STATISTICS: Is the ByYourDoor Service MG16.6 Time-Series Forecasting of Seasonal Data 709 Trending? 661 16.1 Time Series Component Factors 662 16.2 Smoothing an Annual Time Series 664 17 Business Analytics 710 Moving Averages 665 Exponential Smoothing 667 USING STATISTICS: Back to Arlingtons for the Future 710 16.3 Least-Squares Trend Fitting and Forecasting 669 17.1 Business Analytics Categories 711 The Linear Trend Model 669 Inferential Statistics and Predictive Analytics 712 The Quadratic Trend Model 671 Supervised and Unsupervised Methods 712 The Exponential Trend Model 672 Model Selection Using First, Second, and Percentage CONSIDER THIS: What’s My Major if I Want to be a Differences 674 Data Miner? 713 22 CONTENTS 17.2 Descriptive Analytics 714 Predict the Proportion of Items of Interest Based on the Dashboards 714 Values of Other Variables? 740 Data Dimensionality and Descriptive Analytics 715 Classify or Associate Items 740 Determine Whether the Proportion of Items of Interest Is 17.3 Predictive Analytics for Prediction 716 Stable Over Time? 740 17.4 Predictive Analytics for Classification 719 USING STATISTICS: The Future to Be Visited 741 17.5 Predictive Analytics for Clustering 720 CHAPTER REVIEW PROBLEMS 741 17.6 Predictive Analytics for Association 723 Multidimensional scaling (MDS) 724 17.7 Text Analytics 725 19 Statistical Applications 17.8 Prescriptive Analytics 726 in Quality Management USING STATISTICS: Back to Arlingtons … , Revisited 727 (online) 19-1 REFERENCES 727 KEY EQUATIONS 728 USING STATISTICS: Finding Quality at the KEY TERMS 728 Beachcomber 19-1 CHECKING YOUR UNDERSTANDING 728 19.1 The Theory of Control Charts 19-2 CHAPTER REVIEW PROBLEMS 728 19.2 Control Chart for the Proportion: The p Chart 19-4 CASES FOR CHAPTER 17 730 19.3 The Red Bead Experiment: Understanding Process The Mountain States Potato Company 730 Variability 19-10 The Craybill Instrumentation Company 730 19.4 Control Chart for an Area of Opportunity: CHAPTER 17 SOFTWARE GUIDE 731 The c Chart 19-11 Introduction 731 19.5 Control Charts for the Range and the Mean 19-15 SG17.2 Descriptive Analytics 731 The R Chart 19-15 SG17.3 Predictive Analytics for Prediction 733 The X Chart 19-18 SG17.4 Predictive Analytics for Classification 733 19.6 Process Capability 19-21 SG17.5 Predictive Analytics for Clustering 734 Customer Satisfaction and Specification Limits 19-21 SG17.6 Predictive Analytics for Association 734 Capability Indices 19-23 CPL, CPU, and Cpk 19-24 18 Getting Ready to Analyze 19.7 Total Quality Management 19-26 Data in the Future 736 19.8 Six Sigma 19-27 The DMAIC Model 19-28 Roles in a Six Sigma Organization 19-29 USING STATISTICS: Mounting Future Analyses 736 Lean Six Sigma 19-29 18.1 Analyzing Numerical Variables 737 USING STATISTICS: Finding Quality at the Beachcomber, EXHIBIT: Questions to Ask When Analyzing Numerical Revisited 19-30 Variables 737 SUMMARY 19-30 Describe the Characteristics of a Numerical Variable? 737 REFERENCES 19-31 Reach Conclusions About the Population Mean or the Standard Deviation? 737 KEY EQUATIONS 19-31 Determine Whether the Mean and/or Standard Deviation KEY TERMS 19-32 Differs Depending on the Group? 738 CHAPTER REVIEW PROBLEMS 19-32 Determine Which Factors Affect the Value of a Variable? 738 CASES FOR CHAPTER 19 19-34 Predict the Value of a Variable Based on the Values of Other Variables? 739 The Harnswell Sewing Machine Company Case 19-34 Classify or Associate Items 739 Managing Ashland Multicomm Services 19-37 Determine Whether the Values of a Variable Are Stable CHAPTER 19 EXCEL GUIDE 19-38 Over Time? 739 EG19.2 Control Chart for the Proportion: The p Chart 19-38 18.2 Analyzing Categorical Variables 739 EG19.4 Control Chart for an Area of Opportunity: The c Chart 19-39 EXHIBIT: Questions to Ask When Analyzing Categorical EG19.5 Control Charts for the Range and the Mean 19-40 Variables 739 EG19.6 Process Capability 19-41 Describe the Proportion of Items of Interest in Each Category? 739 CHAPTER 19 JMP GUIDE 19-41 Reach Conclusions About the Proportion of Items of JG19.2 Control Chart for the Proportion: The p Chart 19-41 Interest? 740 JG19.4 Control Chart for an Area of Opportunity: The c Chart 19-41 Determine Whether the Proportion of Items of Interest Differs JG19.5 Control Charts for the Range and the Mean 19-42 Depending on the Group? 740 JG19.6 Process Capability 19-42 A01_BERE5032_14_GE_FM.indd 22 27/02/19 2:55 PM CONTENTS 23 CHAPTER 19 MINITAB GUIDE 19-42 B.6 Creating Histograms for Discrete Probability MG19.2 Control Chart for the Proportion: The p Chart 19-42 Distributions (Excel) 756 MG19.4 Control Chart for an Area of Opportunity: B.7 Deleting the “Extra” Histogram Bar (Excel) 757 The c Chart 19-43 C. Online Resources 758 MG19.5 Control Charts for the Range and the Mean 19-43 MG19.6 Process Capability 19-43 C.1 About the Online Resources for This Book 758 C.2 Data Files 758 C.3 Files Integrated With Microsoft Excel 765 20 Decision Making (online) 20-1 C.4 Supplemental Files 765 D. Configuring Software 766 USING STATISTICS: Reliable Decision Making 20-1 D.1 Microsoft Excel Configuration 766 20.1 Payoff Tables and Decision Trees 20-2 D.2 JMP Configuration 768 20.2 Criteria for Decision Making 20-2 D.3 Minitab Configuration 768 Maximax Payoff 20-6 Maximin Payoff 20-7 E. Table 769 Expected Monetary Value 20-7 E.1 Table of Random Numbers 769 Expected Opportunity Loss 20-9 E.2 The Cumulative Standardized Normal Return-to-Risk Ratio 20-11 Distribution 771 20.3 Decision Making with Sample Information 20-16 E.3 Critical Values of t 773 20.4 Utility 20-21 E.4 Critical Values of x2 775 CONSIDER THIS: Risky Business 20-22 E.5 Critical Values of F 776 USING STATISTICS: Reliable Decision-Making, E.6 Lower and Upper Critical Values, T1, of the Wilcoxon Revisited 20-22 Rank Sum Test 780 SUMMARY 20-23 E.7 Critical Values of the Studentized Range, Q 781 REFERENCES 20-23 E.8 Critical Values, dL and dU, of the Durbin–Watson KEY EQUATIONS 20-23 Statistic, D (Critical Values Are One–Sided) 783 KEY TERMS 20-23 E.9 Control Chart Factors 784 CHAPTER REVIEW PROBLEMS 20-23 E.10 The Standardized Normal Distribution 785 CASES FOR CHAPTER 20 20-26 F. Useful Knowledge 786 Digital Case 20-26 F.1 Keyboard Shortcuts 786 CHAPTER 20 EXCEL GUIDE 20-27 F.2 Understanding the Nonstatistical Functions 786 EG20.1 Payoff Tables and Decision Trees 20-27 G. Software FAQs 788 EG20.2 Criteria for Decision Making 20-27 G.1 Microsoft Excel FAQs 788 G.2 PHStat FAQs 788 Appendices 743 G.3 JMP FAQs 789 A. Basic Math Concepts and Symbols 744 G.4 Minitab FAQs 789 A.1 Operators 744 H. All About PHStat 790 A.2 Rules for Arithmetic Operations 744 H.1 What is PHStat? 790 A.3 Rules for Algebra: Exponents and Square Roots 744 H.2 Obtaining and Setting Up PHStat 791 A.4 Rules for Logarithms 745 H.3 Using PHStat 791 A.5 Summation Notation 746 H.4 PHStat Procedures, by Category 792 A.6 Greek Alphabet 749 B. Important Software Skills and Concepts 750 Self-Test Solutions and Answers to B.1 Identifying the Software Version 750 Selected Even-Numbered Problems 793 B.2 Formulas 750 B.3 Excel Cell References 752 Index 825 B.4 Excel Worksheet Formatting 753 Credits 837 B.5E Excel Chart Formatting 754 B.5J JMP Chart Formatting 755 B.5M Minitab Chart Formatting 756 A01_BERE5032_14_GE_FM.indd 23 27/02/19 2:55 PM Preface A s business statistics evolves and becomes an increasingly important part of one’s business education, how business statistics gets taught and what gets taught becomes all the more important. We, the authors, think about these issues as we seek ways to continuously improve the teaching of business statistics. We actively participate in Decision Sciences Institute (DSI), American Statistical Association (ASA), and Data, Analytics, and Statistics Instruction and Business (DASI) conferences. We use the ASA’s Guidelines for Assessment and Instruction (GAISE) reports and combine them with our experiences teaching business statistics to a diverse student body at several universities. When writing for introductory business statistics students, five principles guide us. Help students see the relevance of statistics to their own careers by using examples from the functional areas that may become their areas of specialization. Students need to learn statistics in the context of the functional areas of business. We present each statistics topic in the context of areas such as accounting, finance, management, and marketing and explain the application of specific methods to business activities. Emphasize interpretation and analysis of statistical results over calculation. We emphasize the interpretation of results, the evaluation of the assumptions, and the discussion of what should be done if the assumptions are violated. We believe that these activities are more important to students’ futures and will serve them better than focusing on tedious manual calculations. Give students ample practice in understanding how to apply statistics to business. We believe that both classroom examples and homework exercises should involve actual or r ealistic data, using small and large sets of data, to the extent possible. Familiarize students with the use of data analysis software. We integrate using Microsoft Excel, JMP, and Minitab into all statistics topics to illustrate how software can assist the busi- ness decision making process. (Using software in this way also supports our second point about emphasizing interpretation over calculation). Provide clear instructions to students that facilitate their use of data analysis s oftware. We believe that providing such instructions assists learning and minimizes the chance that the software will distract from the learning of statistical concepts. What’s New in This Edition? This fourteenth edition of Basic Business Statistics features many passages rewritten in a more concise style that emphasize definitions as the foundation for understanding statistical con- cepts. In addition to changes that readers of past editions have come to expect, such as new examples and Using Statistics case scenarios and an extensive number of new end-of-section or end-of-chapter problems, the edition debuts: A First Things First Chapter that builds on the previous edition’s novel Important Things to Learn First Chapter by using real-world examples to illustrate how developments such as the increasing use of business analytics and “big data” have made knowing and understanding statistics that much more critical. This chapter is available as c omplimentary online download, allowing students to get a head start on learning. 24 PREFACE 25 JMP Guides that provide detailed, hands-on instructions for using JMP to illustrate the concepts that this book teaches. JMP provides a starting point for continuing studies in business statistics and business analytics and features visualizations that are easy to con- struct and that summarize data in innovative ways. The JMP Guides join the Excel and Minitab Guides, themselves updated to reflect the most recent editions of those programs. Tabular Summaries that state hypothesis test and regression example results along with the conclusions that those results support now appear in Chapters 9 through 15. An All-New Business Analytics Chapter (Chapter 17) that makes extensive use of JMP and Minitab to illustrate predictive analytics for prediction, classification, clustering, and association as well as explaining what text analytics does and how descriptive and prescrip- tive analytics relate to predictive analytics. This chapter benefits from the insights the coau- thors have gained from teaching and lecturing on business analytics as well as research the coauthors have done for a companion title on business analytics for Fall 2018. Continuing Features that Readers Have Come to Expect This edition of Basic Business Statistics continues to incorporate a number of distinctive fea- tures that has led to its wide adoption over the previous editions. Table 1 summaries these carry-over features: TABLE 1 Distinctive Features Continued in the Fourteenth Edition Feature Details Using Statistics Business Scenarios A Using Statistics scenario that highlights how statistics is used in a business functional area begins each chapter. Each scenario provides an applied con- text for learning in its chapter. End-of-chapter “Revisited” sections reinforces the statistical methods that a chapter discusses and apply those methods to the questions raised in the scenario. In this edition, seven chapters have new or revised Using Statistics scenarios. Emphasis on Data Analysis Basic Business Statistics was among the first business statistics textbooks and Interpretation of Results to focus on interpretation of the results of a statistical method and not on the mathematics of a method. This tradition continues, now supplemented by JMP results complimenting the Excel and Minitab results of recent prior editions. Software Integration Software instructions in this book feature chapter examples and were per- sonally written by the authors, who collectively have over one hundred years experience teaching the application of software to business. Software usage also features templates and applications developed by the authors that mini- mize the frustration of using software while maximizing statistical learning Opportunities for Additional Student Tips, LearnMore bubbles, and Consider This features extend Learning student-paced learning by reinforcing important points or examining side issues or answering questions that arise while studying business statistics such as “What is so ‘normal’ about the normal distribution?” Highly Tailorable Context With an extensive library of separate online topics, sections, and even two full chapters, instructors can combine these materials and the opportunities for additional learning to meet their curricular needs. Software Flexibility With modularized software instructions, instructors and students can switch among Excel, Excel with PHStat, JMP, and Minitab as they use this book, taking advantage of the strengths of each program to enhance learning. 26 PREFACE TABLE 1 Distinctive Features Continued in the Fourteenth Edition (continued) Feature Details End-of-Section and End-of-Chapter “Exhibits” summarize key processes throughout the book. “Key Terms” Reinforcements provides an index to the definitions of the important vocabulary of a chapter. “Learning the Basics” questions test the basic concepts of a chapter. “Apply- ing the Concepts” problems test the learner’s ability to apply those problems to