Advanced Statistical Analysis Lecture Notes (Week 4)
Document Details
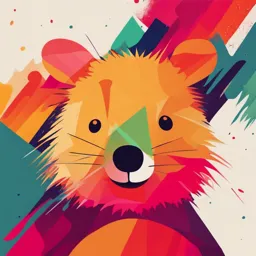
Uploaded by ClearerKoala
University of Groningen
2023
Mark van Duijn
Tags
Related
- Advanced Statistical Analysis - Week 1 Lecture 2
- Advanced Statistical Analysis Lecture Notes
- Advanced Statistical Analysis Lecture Notes - University of Groningen
- Advanced Statistical Analysis Lecture Notes (University of Groningen)
- Advanced Statistical Analysis Lecture Notes - 27 Feb 2023
- Financial Econometrics Exercise Sheet 4 Solutions PDF
Summary
This document provides lecture notes on advanced statistical analysis, focusing on endogeneity and instrumental variables. The lecture notes cover topics such as the causes of endogeneity, correcting for endogeneity, testing for endogeneity, instrumental variables, and two-stage least squares (2SLS).
Full Transcript
Advanced Statistical Analysis Week 4 - Lecture 8 Dr. Mark van Duijn Department of Economic Geography Department of DemographyUniversity of Groningen [email protected] 2 Mar, 2023 Introduction Endogeneity Recap Conclusions Agenda Part I: Endogeneity and 2SLS / Instrumental variable approach Part...
Advanced Statistical Analysis Week 4 - Lecture 8 Dr. Mark van Duijn Department of Economic Geography Department of DemographyUniversity of Groningen [email protected] 2 Mar, 2023 Introduction Endogeneity Recap Conclusions Agenda Part I: Endogeneity and 2SLS / Instrumental variable approach Part II: Recap first four weeks Dr. Mark van Duijn (RUG) Advanced Statistical Analysis 2 Mar 20232 / 25 Introduction Endogeneity Recap Conclusions Is my variable exogenous or endogenous? The fifth OLS assumption which is often forgotten or overlooked: cov (x , ϵ ) = 0 A variable is endogenous if its value is determined or influenced by other variables Remember: Correlated missing independent variables induce bias So does biased sample selection and reverse causality. . . In general, variable x is endogenous if it is correlated with the error term Endogeneity always induces bias (inconsistency and inefficiency) Dr. Mark van Duijn (RUG) Advanced Statistical Analysis 2 Mar 20233 / 25 Introduction Endogeneity Recap Conclusions Causes of endogeneity Correlated missing variables Think of uncontrolled confounders or omitted variable bias, . . . Sample selection What if you select the sample on the basis of something correlated with the error term For example, if you only look at same-sex families and exclude two-sex families, the latter might have a different error term compared to the former Reverse causality What if y causes x? Since y contains the error term, then variation in the error term can show up in x Dr. Mark van Duijn (RUG) Advanced Statistical Analysis 2 Mar 20234 / 25 Introduction Endogeneity Recap Conclusions Correcting for endogeneity Correlated missing variables Include missing (and correct) variables Sample selection Interpretation no longer applies to the population, but rather to a specific group within the population: Narrow the interpretation of your results to a specific group within the population satisfying sample restrictions Reverse causality: difficult Instrumental variables (IV) (Pure) experiments . . . Dr. Mark van Duijn (RUG) Advanced Statistical Analysis 2 Mar 20235 / 25 Introduction Endogeneity Recap Conclusions Test for endogeneity Durbin-Wu-Hausman test is mostly used https://www.stata.com/support/faqs/statistics/durbin-wu-hausman- test/ Or use in STATA after regression: estat endogenous (https://www.stata.com/manuals13/rivregresspostestimation.pdf ) Detailed state-of-the-art information can be found: https://www.schmidheiny.name/teaching/iv2up.pdf Dr. Mark van Duijn (RUG) Advanced Statistical Analysis 2 Mar 20236 / 25 Introduction Endogeneity Recap Conclusions Instrumental variables Endogeneity is basically like pollution in the coefficient of your variable of interest, x A possible solution is to find a variable the explains the variation in your variable of interest, x, that is unpolluted Instruments are variables, let us denote them as H, that are correlated with x, but uncorrelated with the error term by assumption or by construction Properties of valid instruments: cov (H , ϵ ) = 0 cov (H ,x ) ̸ = 0 Dr. Mark van Duijn (RUG) Advanced Statistical Analysis 2 Mar 20237 / 25 Introduction Endogeneity Recap Conclusions 2-Stage Least Squares (2SLS) 1 Regress endogenous variable x 1 on instrument H: x 1 = λ 0 + λ 1H +λ kx k + ewhere k= 2 . . .K Predict ˆ x 1 given the values of the instrument, H This method should be ’clean’ iff H is uncorrelated to the error term and is strongly associated with x 1 2 Regress dependent variable y on predicted value of the endogenous variable ˆ x 1: y = β 0 + β 1 ˆ x 1 + β kx k + ϵ The regression results do not suffer from endogeneity But it does often suffer from having less variance in its predicted value Plus you have to convince your readers that you chose the ’correct’ (strong) instrument Dr. Mark van Duijn (RUG) Advanced Statistical Analysis 2 Mar 20238 / 25 Introduction Endogeneity Recap Conclusions Example: 2SLS results 2SLS results can differ a lot from standard OLS results. . . Dr. Mark van Duijn (RUG) Advanced Statistical Analysis 2 Mar 20239 / 25 Introduction Endogeneity Recap Conclusions Good instruments IV pushes the problem backwards: ”the cure can be worse than the disease” (Bound, Jaeger and Baker, 1993; 1995) Is your instrument actually exogenous? Is it really uncorrelated with the error term? Is there a strong association between your endogenous variable and instrument? Correlation / causal effect? If not, 2SLS estimates may not be consistent and . . . tests of significance have incorrect size, and confidence intervals are wrong Dr. Mark van Duijn (RUG) Advanced Statistical Analysis 2 Mar 202310 / 25 Introduction Endogeneity Recap Conclusions Test for weak instruments Cragg-Donald F-statistic and Kleibergen-Paap Wald F-statistic are mostly used F-statistic with H 0: The instrument(s) is(are) weakly correlated with the endogenous variable(s) Use Stock and Yogo (2005) tables for critival values of the F-statistic Rule of thumb: F-statistic on the excluded instruments in the first stage is greater than 10 Dr. Mark van Duijn (RUG) Advanced Statistical Analysis 2 Mar 202311 / 25 Introduction Endogeneity Recap Conclusions Recap of the first four weeks Recap on exploring relationships Recap on data and modelling issues Recap on linear and logistic regression Recap on model fit statistics Recap on interpretation Dr. Mark van Duijn (RUG) Advanced Statistical Analysis 2 Mar 202312 / 25 Introduction Endogeneity Recap Conclusions Research question Dr. Mark van Duijn (RUG) Advanced Statistical Analysis 2 Mar 202313 / 25 Introduction Endogeneity Recap Conclusions Conceptual thinking and exploring relationships Studying existing literature, what theories are out there?, literature review, formulate hypotheses to be tested using quantitative methods! Hypotheses need to be based on existing literature. Tip: Do not formulate a null-hypothesis in a theoretical chapter! Dr. Mark van Duijn (RUG) Advanced Statistical Analysis 2 Mar 202314 / 25 Introduction Endogeneity Recap Conclusions Data Explore data: Histograms, scatter plots (bin scatter), correlation matrices, descriptive statistics . . . Data ethics: Transformations vs. manipulation Transformations: Taking the natural logarithm of skewed variables Data issues: Measurement error + Missing values + Missing variables Data issues: Multicollinearity + influential observations/outliers Data management: Where to save? Transparent process and reproducable results Dr. Mark van Duijn (RUG) Advanced Statistical Analysis 2 Mar 202315 / 25 Introduction Endogeneity Recap Conclusions Methods and techniques Overview empirical methodologies so far: Linear regression models: OLS Parametric estimation procedure: linear or any other specific functional form (Instrumental variables: 2SLS) Discrete choice/event models: Binary, multinomial, ordered, count Logistic regression Probit regression . . . Note: Methodologies focussed on cross-sectional data! Dr. Mark van Duijn (RUG) Advanced Statistical Analysis 2 Mar 202316 / 25 Introduction Endogeneity Recap Conclusions Modelling Model assumptions: Always formally test error term assumptions and put results in an Appendix! Consistency: Correctness of the estimated coefficient Efficiency: Correctness of the estimated standard error Modelling issues: Violating model assumptions Modelling issues: Endogeneity issues Modelling issues: Functional form Polynomials: e.g. include age and age-squared Splines: e.g. transform age into four or five age categories (dummy variables) Dr. Mark van Duijn (RUG) Advanced Statistical Analysis 2 Mar 202317 / 25 Introduction Endogeneity Recap Conclusions Model fit statistics Linear regression models: OLS Joint sign. F-test. Null-hypothesis: b1=b2=b3=0. Compare F-value to critical F-value and conclude R-square and Adjusted R-square: How much variation in the dependent variable is explained by the variation in the independent variables included in the model? Logistic regression: Maximum likelihood Likelihood-Ratio test / Model Chi-square. Null-hypothesis: Model with constant only is a better fitting model. Compare Chi-square value with Chi-square critical value and conclude Pseudo R-square: Similar interpretation as above Hosmer and Lemeshow test. Null-hypothesis: No difference between observed and model-predicted values. Compare Chi-square value with Chi-square critical value and conclude Dr. Mark van Duijn (RUG) Advanced Statistical Analysis 2 Mar 202318 / 25 Introduction Endogeneity Recap Conclusions Interpretation Standard interpretation without transforming variables or using interaction variables Linear regression models: OLS A unit increase in x, changes y with . . . Logistic regression: Maximum likelihood A unit increase in x, changes ln(odds) with . . . Dr. Mark van Duijn (RUG) Advanced Statistical Analysis 2 Mar 202319 / 25 Introduction Endogeneity Recap Conclusions Interpretation OLS regression coefficients Linear OLS: y= b 0 + b 1x + . . . +e b 1 : level-effect +1 x increases y with b 1 Log-linear OLS: ln(y ) = b 0 + b 1x + . . . +e b 1 : growth rate +1 x increases y with exp( b 1) times or +1 x increases y with (( exp( b 1) − 1) ∗100) % Log-log OLS: ln(y ) = b 0 + b 1ln (x ) + . . .+e b 1 : elasticity +1% in x, increases y with ( b 1)% Assuming that x is a continuous variable! Dr. Mark van Duijn (RUG) Advanced Statistical Analysis 2 Mar 202320 / 25 Introduction Endogeneity Recap Conclusions Interpretation Logit regression coefficients Logistic regression equation: ln ( P 1 − P ) = b 0 + b 1x 1 + . . . +ϵ If x1 increase with 1 unit, ln(odds) will increase with b1 If x1 increase with 1 unit, odds will multiply with exp(b1) A 1 unit increase in x1 increases the odds of Y=1 with about exp(b1) times (compared to Y=0 and keeping all other variables constant) Dr. Mark van Duijn (RUG) Advanced Statistical Analysis 2 Mar 202321 / 25 Introduction Endogeneity Recap Conclusions Interpretation polynomials y = b 0 + b 1x + b 2x 2 + e First derivative gives us the interpretation (if x increases with 1, y increases with . . . ) ∂ y ∂ x = . . . ∂ y ∂ x = b 1 + b 22 x Note: Slope is a linear line increasing(decreasing) in x if b2 is positive(negative) Dr. Mark van Duijn (RUG) Advanced Statistical Analysis 2 Mar 202322 / 25 Introduction Endogeneity Recap Conclusions Interpretation interaction variables Dr. Mark van Duijn (RUG) Advanced Statistical Analysis 2 Mar 202323 / 25 Introduction Endogeneity Recap Conclusions Q&A Any other questions? Dr. Mark van Duijn (RUG) Advanced Statistical Analysis 2 Mar 202324 / 25 Introduction Endogeneity Recap Conclusions Next... Computer practical from 15-17 Lecture 9 Monday 11-13: Multinomial logistic regression - Ch.8 MJ(2022) Example from https://stats.oarc.ucla.edu/stata/dae/multinomiallogistic-regression/ and DeMaris (1995) and Reczek et al. (2014) (if you want to prepare for the next lecture) Dr. Mark van Duijn (RUG) Advanced Statistical Analysis 2 Mar 202325 / 25