Advanced Statistical Analysis Lecture Notes - 27 Feb 2023
Document Details
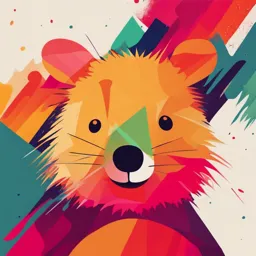
Uploaded by ClearerKoala
University of Groningen
2023
Mark van Duijn
Tags
Summary
These lecture notes cover advanced statistical analysis, specifically focusing on logistic regression. The notes are from a class on advanced statistical analysis and cover topics such as the interpretation of logistic regression results, model fit statistics, and dealing with heteroskedastic errors.
Full Transcript
Advanced Statistical Analysis Week 4 - Lecture 7 Dr. Mark van Duijn Department of Economic Geography Department of DemographyUniversity of Groningen [email protected] 27 Feb, 2023 Introduction Part 0 Part I Part II Conclusion What did we learn last week? Describe logistic regression Apply logis...
Advanced Statistical Analysis Week 4 - Lecture 7 Dr. Mark van Duijn Department of Economic Geography Department of DemographyUniversity of Groningen [email protected] 27 Feb, 2023 Introduction Part 0 Part I Part II Conclusion What did we learn last week? Describe logistic regression Apply logistic regression models and compare them to linear regressions models Critically assess logistic regression models in published academic articles Predict and interpret using logistic regression results Few things are still open:Discussion on model fit statistics robust/clustered standard errors, identification strategies, endogeneity, . . . Dr. Mark van Duijn (RUG) Advanced Statistical Analysis 27 Feb 20232 / 25 Introduction Part 0 Part I Part II Conclusion Agenda Part 0: p, odds, ln(odds) calculations Part I: Model fit statistics Part II: Violating the assumption of homoskedastic errors Dr. Mark van Duijn (RUG) Advanced Statistical Analysis 27 Feb 20233 / 25 Introduction Part 0 Part I Part II Conclusion Probabilities, odds, ln(odds) Dr. Mark van Duijn (RUG) Advanced Statistical Analysis 27 Feb 20234 / 25 Introduction Part 0 Part I Part II Conclusion Probabilities, odds, ln(odds) Math: Let’s call Odds=O O = p 1 − p O ∗(1 −p) = p O −O ∗p = p O =p+ O ∗p O =p∗(1 + O) O (1+ O) = p Dr. Mark van Duijn (RUG) Advanced Statistical Analysis 27 Feb 20235 / 25 Introduction Part 0 Part I Part II Conclusion Probabilities, odds, ln(odds) Math: Let’s call Odds=O O = p 1 − p O ∗(1 −p) = p O −O ∗p = p O =p+ O ∗p O =p∗(1 + O) O (1+ O) = p Dr. Mark van Duijn (RUG) Advanced Statistical Analysis 27 Feb 20235 / 25 Introduction Part 0 Part I Part II Conclusion Probabilities, odds, ln(odds) Math: Let’s call Odds=O O = p 1 − p O ∗(1 −p) = p O −O ∗p = p O =p+ O ∗p O =p∗(1 + O) O (1+ O) = p Dr. Mark van Duijn (RUG) Advanced Statistical Analysis 27 Feb 20235 / 25 Introduction Part 0 Part I Part II Conclusion Probabilities, odds, ln(odds) Math: Let’s call Odds=O O = p 1 − p O ∗(1 −p) = p O −O ∗p = p O =p+ O ∗p O =p∗(1 + O) O (1+ O) = p Dr. Mark van Duijn (RUG) Advanced Statistical Analysis 27 Feb 20235 / 25 Introduction Part 0 Part I Part II Conclusion Probabilities, odds, ln(odds) Math: Let’s call Odds=O O = p 1 − p O ∗(1 −p) = p O −O ∗p = p O =p+ O ∗p O =p∗(1 + O) O (1+ O) = p Dr. Mark van Duijn (RUG) Advanced Statistical Analysis 27 Feb 20235 / 25 Introduction Part 0 Part I Part II Conclusion Probabilities, odds, ln(odds) Math: Let’s call Odds=O O = p 1 − p O ∗(1 −p) = p O −O ∗p = p O =p+ O ∗p O =p∗(1 + O) O (1+ O) = p Dr. Mark van Duijn (RUG) Advanced Statistical Analysis 27 Feb 20235 / 25 Introduction Part 0 Part I Part II Conclusion Example Example: Whether households take or decline an offer of roof solar panels Independent variables: Famsize: size of family household (#) Mortgage: Monthly mortgage (in dollars) y*: take (y=1) or decline (y=0) the offer of roof solar panels y is a limited dependent variable because it can only take the value 0 or 1 Dr. Mark van Duijn (RUG) Advanced Statistical Analysis 27 Feb 20236 / 25 Introduction Part 0 Part I Part II Conclusion Example Example: Whether households take or decline an offer of roof solar panels Independent variables: Famsize: size of family household (#) Mortgage: Monthly mortgage (in dollars) y*: take (y=1) or decline (y=0) the offer of roof solar panels y is a limited dependent variable because it can only take the value 0 or 1 Dr. Mark van Duijn (RUG) Advanced Statistical Analysis 27 Feb 20236 / 25 Introduction Part 0 Part I Part II Conclusion Statistical model Example: Whether households take or decline an offer of roof solar panels Independent variables: Famsize: size of family household (#) Mortgage: Monthly mortgage (in dollars) y*: take (y=1) or decline (y=0) the offer of roof solar panels y ∗ = ln( p 1 − p) ln ( p 1 − p) = b 0 + b 1 ∗ Famsize +b 2 ∗ Mortgage +e Dr. Mark van Duijn (RUG) Advanced Statistical Analysis 27 Feb 20237 / 25 Introduction Part 0 Part I Part II Conclusion Linear regression Dr. Mark van Duijn (RUG) Advanced Statistical Analysis 27 Feb 20238 / 25 Introduction Part 0 Part I Part II Conclusion Model fit statistics for linear regression R 2 = 0 .5028: 50.28% of the variance in the dependent variable is explained by the variance in the independent variables F value = 32 .14 and F criticalvalue (2 ,27) = ∼3.35 (at the 95% significance level) F-value is higher than F-critical value so reject or not reject H0? Explicitly specify H0. . . Make sure you are able to read a ”Critical F-value table”! Dr. Mark van Duijn (RUG) Advanced Statistical Analysis 27 Feb 20239 / 25 Introduction Part 0 Part I Part II Conclusion Comparison regression results Dr. Mark van Duijn (RUG) Advanced Statistical Analysis 27 Feb 202310 / 25 Introduction Part 0 Part I Part II Conclusion Interpretation Binomial regression. Dependent variable is a dummy variable: For example, option A = 1 and option B = 0 (reference category) Linear (probability model) regression If x changes by 1 unit, y (the probability of choosing option A) will, on average, increase with β 1 (ceteris paribus) Logistic regression If x changes by 1 unit, ln(odds) of choosing option A (compared to option B) will, on average, increase with β 1 If x changes by 1 unit, the odds of choosing option A be, on average, e β 1 times higher compared to option B (or the odds increase with ( e β 1 − 1) ∗100 %) Remember, interpretation focuses on the change of one independent variable (while keeping all other variables constant) Dr. Mark van Duijn (RUG) Advanced Statistical Analysis 27 Feb 202311 / 25 Introduction Part 0 Part I Part II Conclusion Interpretation Example: Whether households take or decline an offer of roof solar panels Specification 2: ln( p 1 − p) = −18 .627 + 2 .399 ∗Famsize + 0.005 ∗Mortg ∂ ln ( p 1 − p) ∂ Famsize = 2 .399 ∂ p 1 − p ∂ Famsize = exp 2 .399 = 11 .01 If Famsize increase with 1 unit, ln(odds) will increase with 2.399 If Famsize increase with 1 unit, odds will multiply with 11.01 A 1 unit increase in family size increases the odds of taking up the offer with about 11 times on average (compared to not taking up the offer and keeping all other variables constant) Dr. Mark van Duijn (RUG) Advanced Statistical Analysis 27 Feb 202312 / 25 Introduction Part 0 Part I Part II Conclusion Interpretation Example: Whether households take or decline an offer of roof solar panels Specification 2: ln( p 1 − p) = −18 .627 + 2 .399 ∗Famsize + 0.005 ∗Mortg ∂ ln ( p 1 − p) ∂ Famsize = 2 .399 ∂ p 1 − p ∂ Famsize = exp 2 .399 = 11 .01 If Famsize increase with 1 unit, ln(odds) will increase with 2.399 If Famsize increase with 1 unit, odds will multiply with 11.01 A 1 unit increase in family size increases the odds of taking up the offer with about 11 times on average (compared to not taking up the offer and keeping all other variables constant) Dr. Mark van Duijn (RUG) Advanced Statistical Analysis 27 Feb 202312 / 25 Introduction Part 0 Part I Part II Conclusion Interpretation Example: Whether households take or decline an offer of roof solar panels Specification 2: ln( p 1 − p) = −18 .627 + 2 .399 ∗Famsize + 0.005 ∗Mortg ∂ ln ( p 1 − p) ∂ Famsize = 2 .399 ∂ p 1 − p ∂ Famsize = exp 2 .399 = 11 .01 If Famsize increase with 1 unit, ln(odds) will increase with 2.399 If Famsize increase with 1 unit, odds will multiply with 11.01 A 1 unit increase in family size increases the odds of taking up the offer with about 11 times on average (compared to not taking up the offer and keeping all other variables constant) Dr. Mark van Duijn (RUG) Advanced Statistical Analysis 27 Feb 202312 / 25 Introduction Part 0 Part I Part II Conclusion Interpretation Example: Whether households take or decline an offer of roof solar panels Specification 2: ln( p 1 − p) = −18 .627 + 2 .399 ∗Famsize + 0.005 ∗Mortg ∂ ln ( p 1 − p) ∂ Famsize = 2 .399 ∂ p 1 − p ∂ Famsize = exp 2 .399 = 11 .01 If Famsize increase with 1 unit, ln(odds) will increase with 2.399 If Famsize increase with 1 unit, odds will multiply with 11.01 A 1 unit increase in family size increases the odds of taking up the offer with about 11 times on average (compared to not taking up the offer and keeping all other variables constant) Dr. Mark van Duijn (RUG) Advanced Statistical Analysis 27 Feb 202312 / 25 Introduction Part 0 Part I Part II Conclusion Comparing models Example: Whether households take or decline an offer of roof solar panels Dr. Mark van Duijn (RUG) Advanced Statistical Analysis 27 Feb 202313 / 25 Introduction Part 0 Part I Part II Conclusion Comparing models Example: Whether households take or decline an offer of roof solar panels Specification 1: ln( p 1 − p) = 0 .134 Specification 1: Odds=p 1 − p = exp 0 .134 = 1 .143 Specification 1: p= 1 .143 1+1 .143 = 0 .533 Specification 2: ln( p 1 − p) = −18 .627 + 2 .399 ∗Famsize + 0.005 ∗Mortg Specification 2: Odds =p 1 − p = exp ( − 18 .627+2 .399 ∗Famsize +0.005 ∗Mortgage )Specification 2: p= exp ( − 18 .627+2 .399 ∗Famsize +0.005 ∗Mortgage ) 1+ exp ( − 18 .627+2 .399 ∗Famsize +0.005 ∗Mortgage )Dr. Mark van Duijn (RUG) Advanced Statistical Analysis 27 Feb 202314 / 25 Introduction Part 0 Part I Part II Conclusion Comparing models Example: Whether households take or decline an offer of roof solar panels Specification 1: ln( p 1 − p) = 0 .134 Specification 1: Odds=p 1 − p = exp 0 .134 = 1 .143 Specification 1: p= 1 .143 1+1 .143 = 0 .533 Specification 2: ln( p 1 − p) = −18 .627 + 2 .399 ∗Famsize + 0.005 ∗Mortg Specification 2: Odds =p 1 − p = exp ( − 18 .627+2 .399 ∗Famsize +0.005 ∗Mortgage )Specification 2: p= exp ( − 18 .627+2 .399 ∗Famsize +0.005 ∗Mortgage ) 1+ exp ( − 18 .627+2 .399 ∗Famsize +0.005 ∗Mortgage )Dr. Mark van Duijn (RUG) Advanced Statistical Analysis 27 Feb 202314 / 25 Introduction Part 0 Part I Part II Conclusion Pseudo R-squared Pseudo R-squared (McFadden’s) Same interpretation as R-squared from linear regression (but calculated differently) 58% of the variance in the dependent variable can be explained by the variance in the independent variables Dr. Mark van Duijn (RUG) Advanced Statistical Analysis 27 Feb 202315 / 25 Introduction Part 0 Part I Part II Conclusion Model chi-square Difference between -2LL of specification 2 and -2LL of specification 1 is referred to as the model chi-square H 0 : The model of specification 1 is a good fitting model H 1 : The model of specification 1 is not a good fitting model (i.e. the independent variables have a significant effect) Dr. Mark van Duijn (RUG) Advanced Statistical Analysis 27 Feb 202316 / 25 Introduction Part 0 Part I Part II Conclusion Hosmer and Lemeshow test (H-L Statistic) H-L Statistic Alternative to model chi-square Compares observed and predicted number of successes for different groups based on their estimated probability H 0: There is no difference between observed and model-predicted values (i.e. model’s estimates fit the data at an acceptable level) H 1: There is a difference between observed an model-predicted values (i.e. model’s estimates do not fit the data well) Basically, you do not want to reject the null hypothesis Dr. Mark van Duijn (RUG) Advanced Statistical Analysis 27 Feb 202317 / 25 Introduction Part 0 Part I Part II Conclusion Classification tables for spec. 1 and spec. 2 Dr. Mark van Duijn (RUG) Advanced Statistical Analysis 27 Feb 202318 / 25 Introduction Part 0 Part I Part II Conclusion Check out the Excel-file for the exact calculations Show Excel-file in class. It is available on Brightspace! Take the opportunity to study it so you are able to perform such tests and checks yourself and be able to understand and discuss the outcomes. Dr. Mark van Duijn (RUG) Advanced Statistical Analysis 27 Feb 202319 / 25 Introduction Part 0 Part I Part II Conclusion Violating constant error variance: σ2 ϵ Consequences on consistency? on efficiency? (Chapter 7.2.2) How to test? Breusch-Pagan test (Gujarati, 2012). What’s the null-hypothesis? How to check in Stata? ”regcheck” BP test tells us to reject the null-hypothesis. How to solve the heteroskedasticity in the error term? Dr. Mark van Duijn (RUG) Advanced Statistical Analysis 27 Feb 202320 / 25 Introduction Part 0 Part I Part II Conclusion Robust standard errors vs. cluster standard errors Heteroskedasticity-robust standard errors (Eicker, 1963; Huber, 1967; White, 1980) reg y x i.region, r Cluster-robust standard errors (Liang and Zeger, 1986; Arellano, 1987) reg y x i.region, cluster(region) Academic discussion is twofold: i) adjust or not adjust? Discussion is on: Cluster sampling. ii) Which technique to use? See Abadie et al. (2023) or follow tweets by Wooldridge Dr. Mark van Duijn (RUG) Advanced Statistical Analysis 27 Feb 202321 / 25 Introduction Part 0 Part I Part II Conclusion Misconceptions I. Need for clustering standard errors hinges on the presence of a nonzero correlation between residuals for units belonging to the ”same” cluster. II. There is no harm in using clustering standard errors when they are not required. III. Researchers have only two choices: either fully adjust for clustering or use robust standard errors Abadie et al. (2023). When should you adjust standard errors for clustering? The Quarterly Journal of Economics, 138(1). Dr. Mark van Duijn (RUG) Advanced Statistical Analysis 27 Feb 202322 / 25 Introduction Part 0 Part I Part II Conclusion What did we learn? Distinguish between ln(odds), odds, odds ratios and probabilities and transform them Interpretation of logistic regression results Describe and interpret model fit statistics Describe how to deal with heteroskedastic errors How to account for heteroskedastic errors in Stata Dr. Mark van Duijn (RUG) Advanced Statistical Analysis 27 Feb 202323 / 25 Introduction Part 0 Part I Part II Conclusion Literature + Data Background literature: Burns and Burns (2008): Chapter 24: Logistic Regression, in Business Research Methods and Statistics Using SPSS. Data and *.do file can be found on Nestor! Dr. Mark van Duijn (RUG) Advanced Statistical Analysis 27 Feb 202324 / 25 Introduction Part 0 Part I Part II Conclusion Next... Next lecture: Thursday from 11h00-13h00 on remaining issues + Q&A - Send me an email with potential questions and topics to recap! Next Computer labs: Thursday from 15h00-17h00 Dr. Mark van Duijn (RUG) Advanced Statistical Analysis 27 Feb 202325 / 25