Lecture 14: Exploring Relationships Between Variables PDF
Document Details
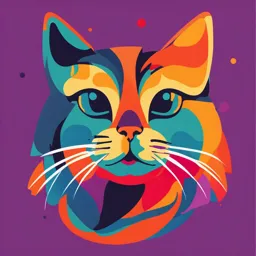
Uploaded by InstructiveProsperity
University of Michigan - Ann Arbor
Tags
Summary
This document introduces concepts in statistics, exploring relationships between variables. It delves into independent samples and demonstrates different scenarios. The objective is to analyze the relationship between quantitative and categorical variables.
Full Transcript
Lecture 14 Page 01 Exploring Relationships Between Variables Objective: We aim to examine a relationship between a quantitative variable and a categorical variable. Research Question: We will be answering research questions that take the form: Do two groups defined by the binary categorical var...
Lecture 14 Page 01 Exploring Relationships Between Variables Objective: We aim to examine a relationship between a quantitative variable and a categorical variable. Research Question: We will be answering research questions that take the form: Do two groups defined by the binary categorical variable 𝑋 show differences in the quantitative outcome 𝑌? Parameter of Interest: We will focus on measuring and comparing the average values of 𝑌 between the two groups determined by 𝑋. Lecture 14 Page 01 Try It! Research Questions In each of the scenarios, re-express the given research question in terms of an association between a categorical explanatory variable and a quantitative response variable. How large is the gender pay gap in the United States? annual Is a persons salary associated with their gender identity ? Coutcome) (predictor) Lecture 14 Page 02 Two Independent Samples data collected must represent this in order for associations to be made Independent samples: Measurements in one sample are unrelated to those in the other sample Ways that independent samples can occur: Random samples are taken separately from two populations and the same response variable is recorded for each observation. One random sample is taken and a variable is recorded for each observation, but then observations are categorized as belonging to one population or another (e.g. old/young, undergrad/graduate student). Participants are randomly assigned to one of two treatment conditions and the same response variable is recorded for each participant. Lecture 14 Page 03 Try It! Scenario 1 In a study, researchers examined whether caffeine increases the rate at which people can tap their fingers. A total of 82 students were randomly divided into two groups of 41 students each, with one group receiving caffeinated coffee and the other receiving decaffeinated coffee. After a few hours, each student was tested to measure finger tapping rate (taps per minute) Does the previous scenario result in two independent samples of measurements? If so, identify the two independent samples. yes. Sample I tap rates of 1 coffinated students : sample z: tap rates of 1 uncoffinated students Lecture 14 Page 03 Try It! Scenario 2 Before the first lecture, a Calculus instructor gives their students a pretest to determine their Calculus readiness. At the end of the course, the instructor gives a post-test to the same students and compares the results with the pretest. Does the previous scenario result in two independent samples of measurements? If so, identify the two independent samples. scores of calc students Sample 1: Precourse No of the same students ↳ paired data Sample 2 : Post course scores analysis (not suitable for our tests) Lecture 14 Page 03 Try It! Scenario 3 A study compared the GPA of sophomores who live in campus dormitories with the average of sophomores who live off campus. 20 sophomores were randomly selected from campus dormitories at a college, and 20 other sophomores were randomly selected from students who live off campus. Does the previous scenario result in two independent samples of measurements? If so, identify the two independent samples. Group Work Question 2.3 Recall the Sampling Distribution of Sample Means The distribution of all possible sample mean values has the following properties: Center = population mean 𝞵 𝜎 Standard deviation = 𝑛 Shape = Result 1: When the population distribution is Normal, the distribution of all possible sample mean values is Normal. Result 2: When the population distribution is non-Normal, and the sample size is large enough, the distribution of all possible sample mean values is approximately Normal. How do we update these findings when we are comparing data from two independent groups? 10 Lecture 14 Page 04 ෝ𝟏 − 𝝁 Sampling Distribution of 𝝁 ෝ𝟐 2) N(12 2) N(10 , Population 1: , Population 2: 𝑁𝑜𝑟𝑚𝑎𝑙 𝜇1 , 𝜎1 Independent 𝑁𝑜𝑟𝑚𝑎𝑙 𝜇2 , 𝜎2 where 𝜇1= 12 Populations where 𝜇2= 10 Draw a sample Draw a sample from population 1 2 from population 2 = = 10 8 of size 𝑛1 and of size 𝑛2 and compute 𝜇Ƹ 1 compute 𝜇Ƹ 2 Compare the difference in means: 𝜇Ƹ 1 − 𝜇Ƹ 2 What should be the center of the , = 14 M distribution? = 8 2 My M , My - = How does increasing the sample sizes 𝑛1 and 𝑛2 , affect the variability in -M of the sampling distribution? d Lecture 14 Page 04 ෝ𝟏 − 𝝁 Sampling Distribution of 𝝁 ෝ𝟐 Sampling Distribution of the Difference in Two (Independent) Sample Means Result 1: If the two populations of responses are normally distributed, then the distribution of all possible values of the difference in sample means, 𝜇Ƹ 1 − 𝜇Ƹ 2 , is of - sum - individual ders 𝜎12 𝜎22 Standard 𝜇ො1 − 𝜇ො2 ~𝑁 𝜇1 − 𝜇2 , + ~ 𝑛1 𝑛2 I unknown difference in independent pop means Result 2: If the two populations of responses are not normally distributed and sample sizes are both large enough, then the distribution of all possible values of the difference in sample means, 𝜇Ƹ 1 − 𝜇Ƹ 2, is approximately 𝜎12 𝜎22 𝜇ො1 − 𝜇ො2 ~𝑁 𝜇1 − 𝜇2 , + 𝑛1 𝑛2 Lecture 14 Page 06 Try It! Are movies getting longer, on average? We took a random sample of 105 movies released in the 1980s (group 1) and another random sample of 45 movies released in the 2000s (group 2). Let’s explore the data descriptively. Are movies getting longer on aug? Explain why these two samples of movies are considered independent. of a movie sample in one knowing the run time provides no information about the runtime Of ANY movie in the other sample There is no way to meaningfully link observational units accross samples ↳ can treat them as two samples of ind. Observations Lecture 14 Page 06 Try It! Are movies getting longer, on average? M. - My > aggregate(runtime~decade, data=movies, FUN = quantile) decade runtime.0% runtime.25% runtime.50% runtime.75% runtime.100% 1 1980 25.00 91.25 100.00 109.00 160.00 2 2000 78.00 102.50 115.00 127.00 219.00 > aggregate(runtime~decade, data=movies, FUN = mean) decade runtime 1 1980 101.67 2 2000 116.64 What is a point estimate value of the unknown true population parameter 𝜇1 − 𝜇2 ? X Change inrea M , - My = 116 64 101 67. - 14 97 - = -. mins Lecture 14 Page 07 Assumptions When performing statistical inference to compare two means, we have the following assumptions: 1. Random samples: A random sample of responses that was drawn from each population of interest. 2. Independence between the two groups: This implies no relationship between the observations in one group and the observations in the other. 3. Normality: The data in each group was drawn from population data that is normally distributed. This assumption can be relaxed with large sample sizes due to the CLT. Lecture 14 Page 07 Checking Normality Assumption Approach: If the sample data appear to follow a normal distribution, then we may assume that the sample data was drawn from a normally distributed population. Graphical Methods to Check Normality: Histograms: Visualize the distribution of sample data. If sample data seems approximately symmetric and unimodal, then we can suggest that the sample data was drawn from a normally distributed population. QQ Plots (Quantile-Quantile Plots): Compare the quantiles of sample data against the quantiles of a theoretical normal distribution. If sample data points fall along the identity line, then we can suggest the sample data was drawn from a normally distributed population Lecture 14 Page 08 Try It! Are movies getting longer, on average? Sample 1: 105 movies released in the 1980s A n. = 105 linear more d or - closer the movies fall to identity line, the more normally distributed o they appear to be - - Observations from Q-Q plot: ↳ hypothetically where the movies should fall if they were normally distributed The observed sampled data points roughly follow along and around the identity line of a perfectly normal distribution. Conclusions from Q-Q Plot: → Sample of 105 movie lengths seems to be approximately normal → We can suggest that the population of movie lengths from the 80s from which this sample was drawn seems to be normally distributed. Lecture 14 Page 09 Try It! Are movies getting longer, on average? Sample 2: 45 movies released in the 2000s Observations: Data roughly follow identity line of gq plot Conclusion: can assume population of all runtimes of movies released in 2000s is nearly normal p have checked all 3 ↳ assumptions Lecture 14 Page 09 Confidence Intervals for Unknown Confidence Parameters Intervals for Unknown Parameters General Structure: - margin of error + margin of error Sample Statistic ± Margin of Error point estimate One Population Proportion (𝝅):O ෝ ± 𝑧∗ 𝝅 / Pointest. ෝ (𝟏−ෝ 𝝅 multiplier 𝝅) 𝒏 ] - Stand err. One Population Mean (𝝁):8 ෝ 𝝈 ෝ ± 𝑡∗ 𝝁 𝒏 Difference in Two Population Means (𝜇1 − 𝜇2 ): ??? Lecture 14 Page 10 CI for a Difference in Means (μ1 – μ2) Confidence intervals follow the same basic logic: Observed sample statistic ± (multiplier) ×(standard error) ෝ 𝟐𝟏 𝝈 𝝈 ෝ 𝟐𝟐 𝝁 ෝ 𝟐 ± (𝒕∗ ) × ෝ𝟏 − 𝝁 + 𝒏𝟏 𝒏𝟐 Note: To calculate t* use the qt() function in R: qt(___________, cutoff ___________, of lower.tail = _________) TIF - degrees of freedom either use technology or smaller of (𝑛1 − 1, 𝑛2 − 1) Lecture 14 Page 10 Try It! Are movies getting longer, on average? 105 n =. n= = 45 Interpretation of the 90% confidence interval: Based on the two samples of movies, we estimate, with 90% confidence, that the difference in population mean lengths for all movies released in the 1980s versus the 2000s is between -19.72 minutes and -10.20 minutes. btwn 805-2000s , we are 90% confident that movies got btwn 10-20 mins longer Lecture 14 Page 10 ෝ 𝟐𝟏 𝝈 𝝈 ෝ 𝟐𝟐 𝝁 ෝ 𝟐 ± (𝒕∗ ) × ෝ𝟏 − 𝝁 + 𝒏𝟏 𝒏𝟐 0 05 qt(___________,. 44 ___________, T lower.tail = _________) Verify how posit.cloud arrived at the bounds of the 90% confidence interval presented above. 𝟏𝟒. 𝟐𝟏𝟐 𝟏𝟔. 𝟕𝟒𝟐 2) 𝟏𝟎𝟏. 𝟔𝟕 − 𝟏𝟏𝟔. 𝟔𝟒 ± (𝒕∗ ) × 𝟏𝟎𝟓 + 𝟒𝟓 = (19. 72 , = 10. Lecture 14 Page 10 Try It! Are movies getting longer, on average? 15 181 - movies got shorter (- >, 6) sinconclusive outcome 90 % confident that runtimes We are on average are longer among 2000s movies released the movies than in among 1980s Lecture 14 Page 11 Try It! Are movies getting longer, on average? Based on the two samples of movies, we estimate, with 90% confidence, that the difference in population length for all movies released in the 1980s versus the 2000s is between -19.72 minutes and -10.20 minutes. There is a 90% probability that the difference in the population mean lengths for all movies released in the 1980s versus the 2000s is between -19.72 minutes and -10.20 minutes. What can you say about the above statement? A. The statement is correct. B. The statement is incorrect. Lecture 14 Page 11 Try It! Are movies getting longer, on average? Based on the two samples of movies, we estimate, with 90% confidence, that the difference in population length for all movies released in the 1980s versus the 2000s is between -19.72 minutes and -10.20 minutes. If we repeat the sampling process many times, about 90% of the resulting confidence intervals will contain a difference in the population mean lengths for all movies released in the 1980s versus the 2000s which is between - 19.72 minutes and -10.20 minutes. What can you say about the above statement? A. The statement is correct. B. The statement is incorrect. Lecture 14 Page 11 Four General Steps When Performing a HT 1. Determine appropriate null and alternative hypotheses. 2. Check the assumptions for performing the test. 3. Calculate the actual statistic and corresponding test statistic, and determine the 𝒑-value. 4. Two-Step Process: 1. Evaluate the 𝒑-value to determine amount of evidence against the null hypothesis. 2. Make a conclusion about the context of the problem. Lecture 14 Page 11 tailed right left tailed non directional Lecture 14 Page 12 𝑶𝒃𝒔𝒆𝒓𝒗𝒆𝒅 𝑺𝒂𝒎𝒑𝒍𝒆 𝑺𝒕𝒂𝒕𝒊𝒔𝒕𝒊𝒄 − 𝑵𝒖𝒍𝒍 𝑽𝒂𝒍𝒖𝒆 𝑇𝑒𝑠𝑡 𝑆𝑡𝑎𝑡𝑖𝑠𝑡𝑖𝑐 = 𝑺𝒕𝒂𝒏𝒅𝒂𝒓𝒅 𝑬𝒓𝒓𝒐𝒓 ෝ𝟏 − 𝝁 ൫𝝁 ෝ𝟐ቁ − 𝟎 𝑡= ෝ 𝟐𝟏 𝝈 𝝈 ෝ 𝟐𝟐 + 𝒏𝟏 𝒏𝟐 Lecture 14 Page 13 Try It! Coursera enrollment tailed right hyp test above su 5 1. o & - some evidence - Lecture 14 Page 13 Try It! Coursera enrollment i. Question: Identify the assumption about normality. A. The enrollments for the sample of beginner and intermediate courses were each drawn from a normally distributed population of enrollments. B. The sample size for each sample is larger than 25, so the distribution of enrollments for each population of courses is normal in shape. Lecture 14 Page 13 Try It! Coursera enrollment ii. Which plots would you use to check this normality assumption? Lecture 14 Page 13 Try It! Coursera enrollment Explain what the t-test statistic, 𝑡 = 1.5, measures. Lecture 14 Page 13 Try It! Coursera Claim/Research Question: Is enrollment in beginner-level courses higher compared to intermediate-level courses, on average? Results: 𝑡 − 𝑡𝑒𝑠𝑡 = 1.5 and 𝑝 − 𝑣𝑎𝑙𝑢𝑒 = 0.07 Write a few sentences that include: - Use the 𝑝-value to evaluate the evidence against 𝐻0 - Draw an overall conclusion referencing the original question.