Audiovisual Translation (AVT) PDF
Document Details
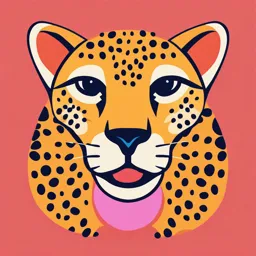
Uploaded by TrendyMulberryTree9740
Malekan Payame Noor University
Tags
Summary
This document provides an overview of audiovisual translation (AVT), exploring its historical context. It discusses theoretical frameworks, categorizations like content-focused and appeal-focused texts, and the significance of multimodal elements in AVT. It also touches upon the development of subtitling technologies and the role of post-editing.
Full Transcript
CHAPTER ONE ((Reconceptualizing Subtitling)) In recent decades, audiovisual translation (AVT) has been, without a doubt, one of the most prolific areas of research in the field of Translation Studies, if not the most prolific one. Although it was ignored in acade...
CHAPTER ONE ((Reconceptualizing Subtitling)) In recent decades, audiovisual translation (AVT) has been, without a doubt, one of the most prolific areas of research in the field of Translation Studies, if not the most prolific one. Although it was ignored in academic and educational circles for many years, audiovisual translation has existed as a professional practice since the invention of cinema at the turn of the 20th century. However, it was not until the mid- 1990s, with the advent of digitization and the proliferation and distribution of audiovisual materials, that it began to gain scholarly prominence and boost its number of acolytes. For years, the activity of translating audiovisual programs was perceived by many academics as falling short of translation proper because of all the spatial and temporal limitations imposed by the medium itself, which in turn constrain the end result. They preferred to talk about adaptation, an attitude that stymied the early debates about the place of AVT in Translation Studies (TS) and may explain why the field was ignored by TS scholars until relatively recently. Jakobson is often cited as being one of the first academics to open up the field of TS (Translation Studies), when he famously established three types of translation, namely: intralingual (or rewording), interlingual (or translation proper) and intersemiotic (or transmutation). One of the early scholars to discuss the significance for translation of the multimodal nature of the source text (ST) is Reiss, who in her text typology for translators distinguishes three initial groups, namely: content-focused texts. form-focused texts, and appeal-focused texts. To these, she adds a fourth, overarching category that she refers to as audiomedial texts, which, in her own words are distinctive in their dependence on non-linguistic (technical) media and on graphic, acoustic, and visual kinds of expression. It is only in combination with them that the whole complex literary form realizes its full potential, as in radio and television scripts, songs, musicals, operas, and stage plays. Audiomedial texts seem then to be aimed at hearers, since they are written to be spoken (or sung) and hence are not read by their audience but heard. From the perspective of audiovisual translation, the term is clearly problematic, and Reiss's taxonomy is also rather wanting because any reference to the main film translation modes, whether dubbing or subtitling, is conspicuously absent in her work and the emphasis is placed, symptomatically, on the hearer rather than the viewer/reader. A decade later, she revisited the term and changed it to multimedial, allowing her to include texts like comics and advertising material, which resort to visual but not acoustic elements. In an attempt to overcome the limitations of Reiss's terminological framework of reference and to dispel any potential confusion, Snell-Hornby later coined four different terms for four different classes of text that all depend on elements other than the verbal: Multimedial texts (in English usually audiovisual) are conveyed by technical and/or electronic media involving both sight and sound (e.g material for film or television, sub-/surtitling); Multimodal texts involve different modes of verbal and nonverbal expression, comprising both sight and sound, as in drama and opera, Multisemiotic texts use different graphic sign systems. verbal and nonverbal (e.g comics or print advertisements); Audiomedial texts are those written to be spoken, hence reach their ultimate recipient by means of the human voice and not from the printed page (e.g. political speeches, academic papers). Finally, interactive software programs, video games, virtual reality and immersive environments keep pushing the nature of audiovisual translation into new territories. The impact of growing practices like cybersubtitling is also being felt in the way subtitles are being produced, distributed and consumed, both in terms of content as well as layout. Cybersubtitles subsume myriad different subtitles found on the internet that can be purposely requested by some collectives, i.c, crowdsourced, or generated on a voluntary basis, and the individuals behind their production can be either amateurs or professionals. Three main sub-groups can be distinguished: fansubs. created by Fans: guerrilla subtitles, produced by people engaged in political causes, and altruist subtitles, usually commissioned and undertaken by individuals with a close affinity to a project. 1. The many instantiations of audiovisual translation Used as an umbrella term, audiovisual translation subsumes a wide variety of translation practices that differ from each other in the nature of their linguistic output and the translation strategies on which they rely. The various ways in which audiovisual productions can be translated into other languages have been discussed by many authors over the years, but the typologies presented by Chaume (2013) and Diaz Cintas (2020) are perhaps two of the most recent and complete. What follows is a panoptic overview of each of the main modes In the main, two fundamental approaches can be distinguished when dealing with the linguistic transfer in AVT. Revoicing: the original dialogue soundtrack is substituted with a newly recorded or live soundtrack in the TL; Timed text: the original dialogue soundtrack is converted into written text that appears on screen..به طور کلی ترجمه های دیداری شنیداری را میتوان به دو دسته تقسیم کرد ترجمه دیالوگها و گفتگوهای زبان صدا ً (مثالً انگلیسی به زبان مقصد (مثالً) فارسی ترجمه میشوند، صداگذاری در این نوع o ًو سپس این ترجمه ها به صوت تبدیل شده و این صوت روی فیلم یا برنامه انداخته میشود؛ یعنی دقیقا ً همان کاری که مثال در دوبله انجام میشود برنامه (مثال صدای آهنگ پرندگان) به صورت نوشتاری/ دیالوگها و تمام صداهای فیلم، در این نوع ترجمه:متن زماندار o.زماندار پایین نمایشگر نمایش داده میشوند یعنی دقیقا همان کاری که در زیرنویس انجام میشود Within these two all-encompassing approaches, further sub-categorizations can be established. Thus, revoicing subsumes interpreting, voiceover, narration, dubbing, fandubbing and audio description. In simultaneous or consecutive interpreting the source speech is transferred by an interpreter, who listens to the original and verbally translates the content. Though currently restricted to the translation of live speeches and interviews, it used to be a fairly common practice during screenings at film festivals, when the film prints arrived too late and there was not enough time to proceed to their subtitling. م به صوت زبان میدا گوش میدهد و آن را به صداگذاری چند دسته دیگر را شامل میشود که یکی از آنها ترجمه شمامی (است که متر صورت شفاهی ترجمه میکندinterpreting) Voiceover (VO) consists in orally presenting the translation of the ST speech over the still audible original voice. Usually, the speaker is heard for a few seconds in the foreign language (FL), after which the volume of the soundtrack is dimmed, so that the original utterances can still be heard in the background, and the translation in the TL is then overlaid. The translation typically concludes whilst the speaker continues talking for a few more seconds, so that the audience can clearly hear the FL once more. Closely associated with the translation of factual genres, such as documentaries and interviews, it is hailed by some authors as a transfer mode that faithfully respects the message of the original text, an assertion that is, of course, highly debatable. Also known as lektoring in some countries - usually done by a man, who reads all the dialogue in a monotone voice, this way of translating audiovisual materials is common for television in Poland, Russia and a few other countries in Eastern Europe, while cinemas prefer to show films subtitled or dubbed. حتما در تلویزیون خصوصا ً در برنامه های مستند دیده اید که در هنگام پخش مستند دو تا صدا شنیده میشود؛ یکی صدای گوینده بالفاصله صدای گوینده فارسی زبان صدای گوینده... The female lion is انگلیسی زبان و یکی دو سه کلمه اولش که مثالً میگوید انگلیسی زبان خیلی واضح نیست جز دو سه کلمه اولش که مثالً می گوید شدت این صدا ضعیف میشود اما هیچ وقت قطع نمیشود و یعنی یکvoiceover به این عمل میگویند...صدای گوینده فارسی زبان با صدای رسا و بلند پخش میشود که مثالً میگوید شیر ماده شود.صدا روی صدای دیگر انداخته می Narration is also used primarily for the translation of non-fiction and differs from voiceover in that the original speech is completely removed from the soundtrack and replaced by a new voice, in translation. The ensuing translation is often roughly synched with the visuals on screen, and the degree of freedom taken in both the translation and the recording of the new TT can differ greatly as TV documentaries, for instance, are often adapted to fit different time slots. است با این تفاوت که صدای زبان اصلی کامالً حذف میشود و با صدای جدید زبان مقصدvoiceover تریشن یا روایت قصه شبیه جایگزین میگردد Dubbing, also known as lip-syne and famously referred to as traduction totale by Cary (1960) because of its many linguistic challenges, consists in the substitution of the dialogue track of an audiovisual production with another track containing the new lines in the TL. It is widely practiced in Brazil, China, France, Germany, Japan, Italy, Tailand, Turkey and Spain, among many others. A fictional world within a broader fictional world that is cinema, dubbing's ultimate fabrication is to make viewers believe that the characters on screen share the same language as the viewer. To achieve this illusion, three types of synchronization need to be respected: همان دوبله است که در آن صدای زبان اصلی حذف میشود و صدای زبان مقصد روی برنامه انداخته میشودdubbing 1. Lip synchrony, to ensure that the translated sounds fit into the mouth of the onscreen characters, particularly when they are shown in close-up, 2. Isochrony, to guarantee that the duration of the source and the target utterances coincide in length, especially when the characters' lip movements can be seen, and 3. Kinetic synchrony, to ensure that the translated dialogue does not enter into conflict with the thespian performance of the actors and that the voices chosen for the new recording are not at odds with the personal attributes and the physical appearance of the onscreen characters. Fandubbing refers to the dubbing or redubbing, usually done by amateurs or enthusiasts rather than by professional actors, of audiovisual productions that have not been officially dubbed or whose available dubbed versions are deemed to be of poor quality Mostly interlingual, some of them are also intralingual, in which case the intent is primarily humorous and they are then known as fundubs, فن باب نوعی دوبله است که توسط افراد اماتور با طرفداران یک برنامه انجام میشود Finally, Audio Description (AD) is an access service that offers a verbal description of the relevant (visual) components of a work of art or media product, so that blind and visually impaired patrons can fully grasp its form and content. The descriptions of essential visual elements have to be inserted into the "natural pauses" in the original soundtrack of the production. ( به انتقال اطالعات دیداری به صورت کلمات اشاره دارد که به نابینایان کمک میکندaudio) description توصیف اتفاقات صحته.اتفاقات فیلم را بهتر درک کنند The second main approach to AVT consists in adding a written text to the original production, for which some players in the industry, like Netflix, have started to use the umbrella term timed text. These flitting chunks of text correspond to usually condensed, synchronized translations or transcriptions of the original verbal input found in the source language (SL). As a superordinate concept, timed text can be either interlingual or intralingual, and it comprises the following related practices; subtitling, surtitling, subtitling for people who are D/deaf or hard-of-hearing, live subtitling and cybersubtitling Interlingual subtitling may be defined as a translation practice that consists in presenting a written text, generally on the lower part of the screen, that aims to recount the original dialogue exchanged among the various speakers, as well as all the other verbal information that is transmitted visually (letters, inserts, graffiti, text messages, inscriptions, placards, and the like) and aurally (songs, voices of, voiceover narration). Surtitling, also known as supertitling in the USA and supratitling, is a close relative of subtitling and refers to the translation, across languages, or transcription, within the same idiom, of dialogue and lyrics in live events such as operas, musical shows, concerts, conferences and theatre performances. The other major assistive service in AVT, subtitling for people who are D/deaf or hard-of- hearing (SDH), also known as captioning, is a practice that consists of presenting on screen a written text that accounts for the dialogue and its paralinguistic dimension, as well as for music, sounds and noises contained in the soundtrack. so that audiences with hearing impairments can access audiovisual material. As opposed to the next category. this type of subtitling is always done for audiovisual programs that have been pre-recorded. Live subtitling is the production of subtitles for live programs or events, which can be achieved by several means. This type of subtitling can be both intralingual and interlingual and be Realtime, as in a sports programme, or semi-live, as in some sections of the news, where a script of the content is usually made available shortly before the broadcast. Traditionally, professionals used stenotype or shorthand techniques and different keyboards to transcribe or translate the original dialogue, but these days respeaking, or speech-based live subtiling, is gaining ground in the industry. 2. Classification of subtitles Subtitles have been grouped according to the following six criteria: (1) linguistic. (2) time available for preparation. (3) display mode, (4) technical parameters. (5) methods of projection, and (6) medium of distribution. 2.1.Linguistic parameters One of the most traditional classifications of subtitles focuses on their linguistic dimension. From this perspective. as illustrated in Table below, the following types exist: Table: Classification of subtitles according to linguistic parameters Intralingual For karaoke and Foreign For hearers singalong language learnin for people who For dialects, g are deaf or hard- accenis, and poor of-hearing quality recordings Telop Tu cao Interlingual Monolingual Bilingual Multilingual Intralingual subtitling, known by some as same-language subtitling (SLS), involves a shift from oral to written but. as it is a monolingual activity that remains within the same language, there has been a reluctance in some quarters to refer to this practice as translation. Though these subtitles can also target hearers. the main practice that is usually associated with intralingual subtitles is that of subtitling for people who are D/deaf or hard-of-hearing. Developed in order to ensure greater democratic access to audiovisual programming for people with hearing impairments, this variety is also known as (closed) captioning in American English. In a nutshell, SDH provides text for any audible information contained in a film or video, also known as audibles. Subtitlers thus convert into written text the dialogue exchanges heard in the soundtrack, indicating who is saying what and ideally incorporating all para linguistic information that accompanies the actors' utterances and is relevant for the appreciation of the message: emphasis, ironic statements, tone, speech impediments, prosody. accents and use of FLs, among others. They also reflect any other relevant sound features that can be heard and are important for the development of the plot, the understanding of the storyline or the creation of an atmosphere and that a hearing-impaired viewer cannot access directly from the soundtrack, e.g. instrumental music, songs, a telephone ringing, laughter, applause, a knock on the door, the revving of an engine and similar sound effects and environmental noises. The second group of intralingual subtitles are those specifically devised as a didactic tool for the teaching and learning of FLs, by students and also an ever-increasing number of migrants round the world who have the opportunity of learning the language of their host countries by watching subtitled programmes broadcast on television, published on DVD or streamed via internet. It has been found in a report by Media Consulting Group (2011) that: o Viewers accustomed to subtitling develop learning strategies more quickly than those accustomed to dubbing. o Depending on the learner's level, either intralingual or interlingual subtitling will be more appropriate. o Intralingual subtitling seems to be better suited to learning grammar and spelling if the learner is not a beginner, whereas interlingual subtitling is more useful for building vocabulary. o Interlingual subtitles seem to be more effective when the languages at work are closely related. A third type of intralingual subtilling that has gained remarkable popularity in recent years is known as karaoke. sing along and sing-a-long-a. It is used to transcribe the lyrics of songs or movie musicals so that the public can join in the singing at the same time as the characters on screen and the rest of the audience. Another example of intralingual subtitling is the use of subtitles, in movies and other audiovisual programs.to account for the utterances of speakers whose prosodic accent is considered difficult to be understood by the audience, despite being the same language. Telop, an acronym of television opaque projector, is used in Japan and other Asian countries to indicate text superimposed on a screen, such as captions, subtitles or scrolling tickers. It makes use of special effects that are added during the post-production phase, typically onto variety shows, and may appear at any time, on any part of the screen. in myriad colors, fonts and sizes that can be disproportionately large and occupy a sizable portion of the screen. In recent years, novel subtitle types have appeared in the Chinese mediascape, which no longer simply translate or transcribe what is being said by the people on screen, but they also incorporate extra information. Especially in cybersubtitling, translator's do on occasions intentionally joke with the audience rather than accurately translate the ST.in a kind of Subtitler’s performance. Borrowing from telop, the Chinese practice of tù cáo, is exemplary of this approach. In countries like Japan, and particularly in China, a new form of subtitles called dàn mù, which literally translates as bullet curtain or bullet fire, has become very popular among young audiences. It consists of Realtime, user-generated comments, which are dynamic. are contextualized and appear overlaid on top of a video. They are essentially snippets of text containing viewers' comments on and reactions to a particular scene. Written in different font sizes and colors, they often contain smileys and emoticons and are presented scrolling on the screen. from right to left, like a bullet. The second major type of subtitles falls under the category of interlingual and, in their most common incarnation they are monolingual, implying the translation from a source to a TL. Gottlieb (1994) calls this diagonal subtitling since it involves a shift from one language to another along with a change of mode, from oral to written. Bilingual subtitles are a variant within the interlingual category and are produced in geographical areas where two or more languages are spoken. The third and final sub-category within interlingual subtitling is that of multilingual subtitles, in which case three or more languages appear simultaneously on screen. 2.2. Time available for preparation By looking at subtitles from this perspective, the following types can be distinguished: Pre-prepared pre-recorded, offline subtitles Live, online subtitles Semi-live vs. live or realtime subtitles Stenography ,velotype ,group of subtitlers, respeaking Edited vs. verbatim The main difference between pre-prepared/offline and live/online subtitles resides in the fact that the former are done after the program has been shot and ahead of its broadcasting or release, giving translators sufficient time to carry out their work. They are the standard mode in subtitling, allowing for the text to be carefully synchronized with images and soundtrack, with precise in and out timecodes, edited to a reasonable reading speed, and checked for errors. On the other hand, the online type is performed live, i.e. at the same time as the original program is taking place or being broadcast. Within this latter category, two groups can be distinguished: Semi-live subtitling, or as-live subtitling, is a method typically used for live programs which are heavily scripted and have pre-recorded inserts, like news bulletins, theatre plays or opera productions. In these cases. the Subtitler normally creates a list of subtitles, without fixed timecodes, and during the transmission of the program or staging of the performance cues these subtitles manually, following the dialogue as closely as possible. Today, software is being developed that allows for the automatic live broadcasting of prepared surtitles, e. g. for opera and for musicals, which are less prone to improvisation than theatre is. The semi-live approach minimizes the risk of errors that can crop up in live subtitling, though textual accuracy also depends on the amount of material that has been given to the subtitler prior to the event. Nevertheless, temporal synchrony continues to be a challenge. The second type is known as live subtitling, sometimes also called realtime, which is produced for sports. programs, some TV newscasts, live interviews and talk shows as well as for parliamentary proceedings and in educational settings, to provide linguistic support with lectures. In cases like these, the program or speech to be subtitled is not at the disposal of the subtitler beforehand. up to One reason for the advent of intralingual live subtitling for television was the imposition of SDH quotas of 100% in some countries, which made it impossible to provide the required amount of subtitling in pre-prepared mode. Historically, four main approaches can be discerned. First, to achieve the speed and accuracy required for live subtitling, a stenographer or stenotypist-known in North America as stenocaptioner - is in charge of creating the subtitles using a specialist keyboard machine, called a stenotype, to record dictation in shorthand by a series of phonetic symbols, rather than typing letters, and applying writing theories common in court reporting. This was the pioneering method adopted in the early attempts to cope with the provision of subtitles for live programs and, although still in practice, is not widely used because of its labor intensive nature as well as its high costs and required length of training. To overcome the drawbacks of the use of stenography in subtitling, the ergonomic velotype keyboard was invented. A Dutch discovery, it allows velotypists to write complete syllables and words by pressing several keys simultaneously, instead of typing character by character. Yet, mastering velotype involves labor intensive training, which is one of the reasons it is being gradually supplanted by speech recognition. The dual keyboard system, the third approach to live subtitling, which resorted to employing a group of subtitlers, usually three, working on the same program in collaboration has virtually been abandoned in favor of methods based on automatic speech recognition (ASR). Taking turns and typing at very fast speeds on standard keyboards, two of the subtitlers would listen to the audio and produce the subtitles, while the third person revised the text produced by the other two, before authorizing its broadcast. In addition to being too costly, this way of working resulted in significant latency, i.e. the delay between the occurrence of speech and the appearance of the subtitle on screen. The fourth and most common technique today is known as respeaking. It relies on a subtitler/respeaker making use of ASR software to generate the subtitles. It is a process whereby a person listens to the original utterances of a live program and dictates the speech, i.e. respeaks it, including punctuation marks and some specific features for the D/deaf and the hard-of-hearing audiences, to a microphone connected to a computer, to an ASR app and to a subtitling program, which then displays subtitles on screen with the shortest possible delay. The fact that ASR programs can only work with certain major languages, simply because more research effort and capital investment have been put into them, makes their application impractical in the case of some minoritized languages, at least for the time being. Nonetheless, the technology has been around since the 1990s, steadily improving in the number of languages covered as well as in the accuracy of the recognition and the subsequent reduction of spelling mistakes. To conclude, any of the subtitles discussed previously under the various categories can be subdivided further according to their lexical density. Edited subtitles, due to the spatial and temporal limits imposed by the medium, are the most commonly used and consumed when watching a subtitled program, especially in the case of pre-prepared interlingual subtitles. The levels of condensation applied depend on the assumed reading speed of the audience, which has direct impact on the display rate of the subtitles on screen. Verbatim subtitles, on the other hand, are meant to be a full and literal transcription or translation of the spoken words, which risks pushing viewers' reading speed up to uncomfortable levels. 2.3. Display mode Subtitles according to their display mode can be classified into: block, pop-up or pop-on subtitles Cumulative, add-on subtitles Scrolling, scroll-up or roll-up subtitles Subtitles can appear on screen intermittently, in full and in one go, in which case they are known as block, pop-up or pop-on subtitles. They are the standard display mode in pre- prepared subtitling, whether intralingual or interlingual, as a clear consensus exists that block subtitles are the least disruptive of them all, are easier for viewers to read and thus allow them to spend more time looking at images. Cumulative subtitles are sometimes used to permit two- exceptionally three-chunks of speech appearing in the same subtitle but not at the very same time. Each part of the text pops up on screen at a different time, in sync with its speaker, but all text clunks leave the screen at the very same time. The second section of the subtitle appears in syne with the second utterance and is added to the first part, which remains on screen. These cumulative subtitles are normally used for dramatic effect and to avoid advancing information, ic. to present part of a message too early with the risk of diminishing the intended impact of the original message. These subtitles are particularly suitable for jokes (to keep the punch line separate), in quizzes (to separate questions from their answers), to delay a dramatic response or to adhere to the rhythm of a song. Roll-up subtitles have been the typical mode favored when producing live subtitling in most Anglo-Saxon countries, whereas many other nations prefer block subtitles across the board. These subtitles appear as.a constant flow of text and are usually displayed word by word or in blocks containing short phases. They tend to be disliked by viewers because their instability and constant upward movement of the text on screen make the reading a rather challenging and onerous cognitive activity. 2.4. Technical parameters From a technical perspective, the following two types of subtitles can he identified: open, hard subtitles or hard titles, and closed subtitles The basic difference between them is that, in the first case, the subtitles are not encoded into the video signals and are instead irreversibly burned or projected onto the image, which means that they cannot be removed or turned off. As they are merged with the photography, no special equipment or software is required for playback. The audiovisual program and the subtitles cannot be disassociated from each other, giving the viewer no choice Whatsoever as to their presence on screen since they are always visible. Within this category, forced narrative subtitles are those present in the original audiovisual production to provide information necessary to make it comprehensible for the source audience. In the second case, closed or pre-rendered subtitles are separate video frames that are overlaid on the original video stream while playing, which means that they can be turned on and off and become visible on screen only when activated by the viewer by means of a decoder. This formatting makes it possible to have multiple language subtitles and to easily switch between them. As these subtitles are usually encoded as bitmap images, i.e. a series of tiny dots or pixels, they cannot render many colors, which is one of the reasons why SDH on DVD rarely makes use of colors to identify speakers. 2.5. Methods of projection A classification of subtitles from this perspective represents in effect an excursus throughout the history of subtitling: Mechanical and thermal Photochemical Optical Laser Electronic 3D Immersive 2.5.1. Laser subtitling The current method of impression most commonly used in cinema subtitling is laser. Introduced in the late 1980s, it rapidly proved to be much more effective than the previous methods it began to replace. Through a laser ray, the subtitles become an integral part of the film copy, as they have been engraved on the images, and every time the film is projected the subtitles will appear on the lower part of the screen. One of the upsides of this method is that it permits excellent definition of letters, with enhanced contours that facilitate the contrast between the text and the images, enhancing the legibility of the subtitles. Being engraved onto the film's copy eliminates any possibility of the subtitles moving or shaking during the projection of the movie. To laser subtitle a full-length feature film takes about ten times the film’s projection time. 2.5.2. Electronic subtitling Electronic subtitling is the other method frequently used in the profession as an alternative to laser subtitling, its greatest advantage being that it allows subtitles to be superimposed on the screen instead of being hardcoded onto the movie. The preferred type in film festivals, these pre-recorded subtitles, which have been previously translated and synchronized, are then beamed by a projector onto the screen. The technology uses a timecode system to ensure that the text is shown in realtime. in synchrony with the screening of the film. Electronic subtitling permits extremely versatile subtitling of a single film print, making it possible to project the subtitles onto (or below) the image, in any language, in any color (though they tend to be white or yellow), and without damaging the original copy. It is cheaper than laser engraving and is used mainly in film festivals where a single copy of the film can be shown with various sets of subtitles in several countries. Another great advantage over laser is that, since electronic subtitles are independent of the audiovisual program, they can easily be revised and modified from projection to projection. This system is also used to provide better access to movies screened in the cinema for people with hearing loss, without imposing on the hearing population as the subtitles can be projected onto a screen adjacent to the movie screen. In addition, it is a solution for cinema theatres that want to use the same film print for alternative screenings with and without SDH, at different times of the day. Electronic subtitling is also preferred on television and DVD. 2.6. Distribution Although not as neat as the previous categories, a sixth and last taxonomy can also be somewhat discerned. according to the medium used for the distribution of the audiovisual production, which may affect the way in which subtitles are produced. Thus, subtitles can be made for the following: Cinema Video, VHS DVD, VCD, Blu-ray Television Internet CHAPTER ONE ((Human and Machine Translation)) 1. What makes translation difficult? Inter-linguistic differences or non-isomorphism between languages Discontinuous dependencies, where two words that belong together are separated by one or more intervening words, as in the following example Send your certificate of motor insurance back Idioms, 1.e., phrases whose meaning cannot be inferred on the basis of their constituent parts. Idioms, in other words, are non-compositional. A good example is old hat 2. Translation memory In the 1990s translators working in the growing software localization industry found themselves translating texts that were either extremely repetitive in themselves or that repeated verbatim whole sections of earlier versions of a document. This was the case, for example, with software manuals that had to be updated any time there was a new release of the software. Rather than translate each sentence from scratch, as if it had never been translated before. they invented a tool that would store previous translations in a so-called translation memory, so that they could be reused. The tool, known as a translation memory tool would take in a new source text divide it into segments sentences or other sensible units like headings or cells in tables and then compare each of these segments with the source- language segments already stored in memory. If an exact match or a very similar segment was found, then the corresponding target-language segment would be offered to the translator for re-use, with or without editing. As translators worked their way through a new translation assignment, they would get hits from the translation memory, accept, reject or edit the existing translation and update the memory as they went along, adding their own translations for the source language segments for which no matches existed. Over time, the translation memories grew extremely large. Some companies who were early adopters of the technology built up translation memories containing hundreds of thousands and then millions of translation units that is source-language segments aligned with their target-language segments. Private translation enterprises also accumulated large translation memories, which came to be regarded as valuable linguistic assets that could help control translation costs and enhance competitiveness. International organizations such as the Institutions of the European Union adopted the technology and built up huge multilingual translation memories, which they in turn made freely available to computer scientists in the knowledge that they could support research agendas in natural language processing. While translation memory was originally conceived as a way of improving, among other things, the productivity of human translators, it also eventually supported efforts to increase automation in the translation industry: on the one hand, translation memory tools enabled translation data to be created in great quantities and in a format that could be easily used in machine translation development, on the other hand, the tools used to manage them provided an editing environment in which machine translation outputs could later be presented to human translators for editing alongside human translations retrieved from conventional translation memory. Translation memories can be seen as a special type of parallel corpus, that is a collection of source texts aligned at sentence level with their target texts. 3. What is machine translation? Machine translation can be briefly defined as translation performed by a computer program, like Google Translate. Machine translation was one of the first non-numerical applications of the digital computers that emerged in the aftermath of the Second World War. Despite the undoubted usefulness of machine translation, it comes with some health warnings: First, just like human translators, machine translation systems can make mistakes. Errors might range from the amusing but trivial to the extremely serious. Machine translation also raises a surprising number of moral and legal issues. Machine translations can be used for assimilation and dissemination purposes: If you are simply using machine translation to get the gist of a text, to understand the basic contents of a web page, then we can say you are using machine translation for assimilation. Such uses generally involve low-stakes, private use of the translated text in question, with little risk of reputational or other damage. If, however, you want to use machine translation for dissemination, for example to publish your blog in a second language, or to advertise your business, then it is wise to understand the risks involved and even to take measures to mitigate them. The ability to do so is a component of what is now known as machine translation literacy. 4. Artificial intelligence, machine learning and machine translation Contemporary machine translation is frequently mentioned alongside a number of other related concepts, including artificial intelligence, machine learning, artificial neural networks and deep learning. Artificial intelligence (AI) is the most general category. It is often defined as the branch of computer science that aims to create machines - or more specifically computer programs that can solve problems of the kind that would normally require human intelligence. The machines in question don't necessarily have to think like humans, rather they need to act like an intelligent human would. They might be designed to solve fairly narrowly defined problems, like recognizing faces. Such goals are the stuff of narrow Al, also known, somewhat unkindly. as weak Al. So- called strong Al is a more aspirational undertaking. It would involve either general Al-in which machines would have human-like intelligence, be self-aware, able to learn and plan for the future – or superintelligence, which would involve intelligence that exceeds the abilities of any human. It is fair to say that translation, as practiced by professional, human translators, requires the kind of intelligence that strong Al aspires to, but that such intelligence still remains beyond the capacity of machine translation systems. 4.1. Rule-based machine translation One way to tackle the challenges of Al is to attempt to give a computer program all the knowledge it would need to solve a particular problem, and rules that specify how it can manipulate this knowledge. In the case of machine translation, for example, you can give the program a list of all the words in each of the source and the target languages, along with rules on how they can combine to create well-formed structures. You can then specify how the words and structures of one language can map onto the words and structures of the other language, and give the machine some step-by-step instructions (an algorithm) on how to use all this information to create translated sentences. This approach, known as rule-based machine translation (RBMT), dominated machine translation up until the early part of this century. When free online machine translation first became available in 1997, for example, it was based on RBMT. RBMT was beset by a number of problems, however: It was very expensive to develop, requiring highly skilled linguists to write the rules for each language pair. Like other knowledge-based approaches to AI, it suffered from knowledge bottlenecks it was simply impossible in many cases to anticipate all the knowledge necessary to make RBMT systems work as desired. This applies both to knowledge about language and knowledge about the wider world, so-called real-world knowledge. 4.2. Data-driven machine translation This is where machine learning comes in. Machine learning is based on the premise that rather than telling a machine or, more precisely, a computer program everything it needs to know from the outset, it is better to let the machine acquire its own knowledge. The machine does so by observing how the problem it is intended to solve has been solved in the past. We have already seen how translation problems and their solutions can be captured at segment level in the translation units stored in translation memories and other parallel corpora. These translation units constitute the training data from which contemporary machine translation systems learn. This is why such systems are usually categorized as data-driven. And learning from data is what distinguishes machine learning from other types of AI Data-driven machine translation is divided into two types: statistical machine translation and neural machine translation, each of which is addressed below. 4.3. Statistical Machine Translation Statistical Machine Translation (SMT) systems basically build two types of statistical models based on the training data. The first model, known as the translation model, is a bilingual one in which words and so-called phrases found in the source-language side of the training data appear in a table alongside their translations as identified in the target-language side of the training data, and each source-target pairing is given a probability score. The ensuing structure is known as a phrase table. Note: The term "phrase" is something of a misnomer here however, as the strings in question don't necessarily correspond to phrases as commonly understood in linguistics. Rather they are n-grams, that is, strings of one, two, three or л words that appear contiguously in the training data. In the previous sentence, appear contiguously is a bigram, for example, and "appear contiguously in" is a trigram. The second model, known as the language model is a monolingual model (or combination of models) of the target language. Again, it is based on n-grams. A trigram target language model, for example, would give the probability of seeing a particular word in the target language, given that you had already seen the two words in front of it. In SMT systems, the translation model is supposed to capture knowledge about how individual words and n-grams are likely to be translated into the target language, while the language model tells you what is likely to occur in the target language in the first place. What is really important from the current perspective, is that linguists don't have to handcraft these models. Rather they are learned directly from the data by the machine in a training phase. In a second phase, called tuning, system developers work out the weight that should be assigned to each model to get the best output. Once the system is trained and tuned, it is ready to translate previously unseen source sentences. Translation (as opposed to training) is called decoding in SMT. It generally involves generating many thousands of hypothetical translations for the input sentence, and calculating which one is the most probable, given the particular source sentence, the models the system has learned, and the weights assigned to them. SMT was state-of-the-art in machine translation for at least a decade up to 2015. It represented a huge advance compared to the RBMT systems that preceded it, but suffered from a number of deficiencies, most of them due to the fact that: Relatively short -grams were used to build models and that -grams in the same sentence were translated almost as if they were independent of each other. SMT performed particularly poorly on agglutinative and highly inflected languages. Other problems included word drop, where a system simply failed to translate a word, and inconsistency, where the same source-language word was translated two different ways, sometimes in the same sentence. By 2015, SMT was already being displaced by a competing approach to data-driven machine translation, the above-mentioned neural approach. Within a matter of two years the transition to neural machine translation was complete 4.4. Neural Machine Translation SMT had its heyday between 2004 and 2014. Most major users and suppliers of machine translation, including Google Translate (from 2007) and the European Commission (from 2010) were using the technology. Until 2015, that is. That year a neural machine translation (NMT) system developed at Stanford University beat a number of SMT systems by a wide margin and on what was considered a difficult language pair. The Stanford success heralded the beginning of what Bentivogli et al. call "the new NMT era." The excitement was palpable among researchers and especially in the press. Grand claims were made about the new technology, for example, that it was as good as professional, human translation and had thus reached human parity It was also claimed, with some justification, that NMT could learn "idiomatic expressions and metaphors", and "rather than do a literal translation, find the cultural equivalent in another language”. But while there is some truth in such claims, they should not be over-interpreted. An NMT system might indeed produce an idiomatic translation, but this is generally because the data it has learned from contain hundreds or maybe thousands of examples of that very translation. An NMT system (in this case Google Translate) does not know it is being idiomatic, or using a cultural equivalent, when it correctly translates an idiom. Rather it is outputting what it has learned from data. But why is NMT so much better that SMT, if it is simply learning from data? Is that not what SMT was already doing? The answer lies in the kind of representations that NMT systems use and in the kind of models they learn. 4.4.1. Models in NMT Let's start with models. A computer model is an abstract, mathematical representation of some real-life event, system or phenomenon. One use of such a model is to predict an answer to a previously unseen problem. A computational model of translation, for example, should be able to predict a target-language sentence given a previously unseen source-language sentence We have already seen that SMT systems use probabilistic models of translation and the target language that are encapsulated in phrase tables and n-gram probabilities. NMT systems, in contrast, use models that are inspired. even if only loosely, by the human brain. They use artificial neural networks, in which thousands of individual units, or artificial neurons, are linked to thousands of other artificial neurons (let's just call them neurons from now on). In such a network, each neuron is activated depending on the stimuli received from other neurons, and the strength or weight of the connections between neurons. As Forcada explains, the activation states of individual neurons do not make much sense by themselves. It is, instead, the activation states of large sets of connected neurons that can be understood as representing individual words and their relationships with other words. The trick in training an NMT system is to learn precisely those weights that will result in the best performing model of translation, that is, the model whose activation states allow it to predict the best translations. So how is this done? Like in all machine learning, the system learns from data. A neural model of translation is built step by step by exposing a learning algorithm to vast quantities of parallel data. In successive passes, the algorithm learns weights and keeps adjusting those weights, so that the predictions of the model it builds get closer and closer to a desired correct answer It suffices to say here that data-driven machine translation is typical of machine learning in that it involves technologies that are developed to solve problems to which humans already know the answer and to which, in fact, humans have already supplied at least one, if not several correct answers. Such correct answers may be present în the training data or they may be arrived at through generalization from the training data. When a machine translation system is tested to see whether it is improving during training or to compare it to another system once training has finished, we also test by giving it a problem to which we already know the answer. Typically, we ask it to predict the translation of several sentences it has never seen before but for which we already have good (human) translations that we set aside specifically for this purpose. When an NMT system has been trained to our satisfaction1. it can be put into use in a real translation scenario. We no longer talk about "testing the system, and instead talk about using it. When an NMT system is in actual use, most people say that the system is "translating". As with SMT, computer scientists also use the term decoding for the moment when an NMT system produces an output in the target language. 4.4.2.Representing words in NMT In NMT this type of representation is used: the vector, which is a fixed-sized list of numbers. The word apple could be represented by a vector like [1.20.2.80.6.10] for example. Vectors are quite good at representing relationships between words. Vectors have other interesting properties that make them particularly attractive to computer scientists. You can add a vector to another vector, for example, or multiply them and so on. The vector-based representations of words that the machine leams are called word embeddings. The reason why embeddings for related words end up lookcing similar to each other is that they are built up on the basis of where particular words are found in the training data. Word embeddings are not built in one go, but rather in successive layers. An artificial neural network that has multiple layers sandwiched between its external layers is known as a deep neural network Deep learning, in turn, is simply the branch of machine learning that uses multiple layers to build representations. In a deep neural network the external layers correspond to inputs and outputs of the network and are visible to the human analyst. The intermediary, or hidden, layers have traditionally been less open to scrutiny. however, giving deep learning a reputation for opacity, and encouraging some commentators to misleadingly use the word "magic" to describe the internal workings of deep neural networks. 5. The advantages and disadvantages of neural machine translation NMT is generally considered the best performing type of machine translation invented so far. It performs better than SMT, for example, because it can build up very rich representations of words as they appear in a given source text, taking the full source sentence into account, rather than mere n-grams. When it produces translations, an NMT system considers both these rich representations and the emerging target sentence at the same time. Because NMT handles full sentences, it is better at dealing with tricky linguistic features like discontinuous dependencies and it handles all sorts of agreement phenomena better than SMT. But while contemporary NMT systems certainly handle full sentences, until recently, they did not look beyond the current sentence. This meant that they could not use information from a previous sentence to work out what a pronoun like "it" refers to in the current sentence. This restriction to sentence-level processing can cause lots of other problems that only become apparent when users translate full texts rather than isolated sentences. The problem is currently being tackled by researchers working in the area of document-level machine translation, however. NMT can also output words that don't actually exist in the target language. Far more seriously, NMT output can be fluent but inaccurate. And when a translation looks anad sounds good, one might neglect to check that it is compatible with the source text. Like other technologies trained on large quantities of existing text, it can also amplify biases encountered in the training data. NMT systems take much longer and much more computing power to tm in than their predecessors and use up vast quantities of energy in the process. They usually require dedicated, expensive hardware in the form of graphical processing units. They also need massive quantities of training data, which are not available for every language pair. Note: Improvements in the technology have also led some people to question the wisdom of learning foreign languages: if a machine can translate anything anyone else says or writes in a foreign language into your language, why go to all the trouble of learning their language? Such arguments are based on a very limited understanding of the benefits of second or foreign language learning, however, and ignore the fact that machine translation is viable for only a small number of the world's languages. They also tend to see machine translation as being in competition with language learning, rather than possibly being an aid in the process. 6. Four last things you need to know about machine translation Many readers are likely to use only free, online inachine translation and so will encounter only generie engines buiht for the language pair that interests thein. But even these readers should be interested to learn that: different systems may output different translations; different engines in the same system may output different translations; a single system may output different translations for the same input depending on the co-text; a single system's outputs may change over time. 7.Conclusions In one way. NMT is just the latest in a line of technologies designed to automate translation, albeit one that has risen to prominence remarkably quickly. Its success could lead to policy makers and ordinary citizens questioning the value of learning foreign languages or training human translators. But such positions would ignore the fact that NMT still relies on human translations or at least translations validated by humans as training data. And because NMT, like other types of machine translation. is not invincible, its outputs still need to be evaluated and sometimes improved by people who can understand both source and target texts. There is also a pressing need for machine translation literacy among even casual users of the technology, so that they do not suffer unnecessarily because of ignorance of how the technology works. Given the right conditions, NMT can be a vital pillar in the promotion and maintenance of multilingualism, alongside language learning and continued translation done or overseen by humans. CHAPTER TWO ((Selecting and preparing texts for machine translation: Pre-editing and writing for a global audience)) Neural machine translation (NMT) is providing more and more fluent translations with fewer errors than previous technologies, Consequently, NMT is becoming a real tool for speeding up translation in many language pairs. However, obtaining the best raw MT output possible in each of the target languages and making texts suitable for each of the target audiences depends not only on the quality of the MT system but also on the appropriateness of the source text. This chapter deals with the concept of pre-editing, the editing of source texts to make them more suitable for both machine translation and a global target audience. Put simply, pre-editing involves rewriting or editing parts of source texts in a way that is supposed to ensure better quality outputs when those texts are translated by machine. It may involve applying a formal set of rules, sometimes called controlled language rules, which subulate the specific words or structures that are allowed in a text, and prohibit others. Alternatively, it can involve applying a short list of simple fixes to a text, to correct wrong spellings, or impose standard punctuation, for example. Another way to ensure that a text is translatable is to write it that way in the first place. Writers whose work will ultimately be translated into multiple languages are thus often asked to write with a global audience in mind As well as applying principles of "clear writing", they are asked for example, to avoid references that may not be easily understood in cultures other than their own. This applies also to writers whose work will be read in the original language by international readers who are not native speakers of that language. 1.Pre-editing and NMT In the past, when rule-based systems produced obvious, and often systematic, errors in adequacy and fluency. pre-editing was often necessary to get the best out of MT. Even after the transition to statistical MT (SMT), researchers sully found pre-editing to be useful. Some, however, believe that pre-editing is not an effective strategy with NMT systems, because these systems make fewer errors. 2. Genre and domain-based advice Based on their own experience with MT, many language service providers recommend restricting the use of MT. and by extension, NMT, to the translation of: Certain types of technical documentation: These usually involve already standardized texts, in which terminology use is already strict in itself, the style is direct and simple, and the use of linguistic resources closely resembles the application of controlled language rules. The conceptual framework that underpins such technical documentation may also be identical in both the source and target locales. Low-risk internal documentation: These are texts which have very low visibility and where the consequences of less-than-optimal translation are not serious. They may even be limited to use within the user's or client's company. A priori, considerations such as naturalness or fluency in the target language are less relevant than would otherwise be the case (although NMT generally produces quite fluent output anyway). but companies may still wish to control lexical selection and lexical variability. Low-risk external documentation: This refers to texts that are consulted only occasionally or sporadically. Or texts that are used as a help database or similar, and that are often not produced by the client, but by the community of users of its service or product. In many such cases, the MT provider may explicitly deny liability for any losses caused by faulty translations. Note: MT is not usually recommended for texts of a more visible nature whose purpose is not just to inform for give instructions but also to be appellative. that is, to arouse a particular interest in the reader, for example, in a certain brand, or to elicit a certain behavior. In other words, the more informative a text is, the more it limits itself to the literalness of its message, the less implicit information it contains and the less it appeals to references linked to the reader's culture or social reality. the greater the expected success of MT. 3. Writing for a global audience Sometimes the objective of pre-editing is not a matter of avoiding errors in the translated text, but rather of ensuring that the translation, beyond conveying a meaning consistent with that of the source text. also achieves the same or a similar effect on the reader of the target text as the source text did on its reader, to the extent that this. is possible. It is a question of making a text available to a global audience and attempting to have the same effect on readers in each target language Whether the text is to be translated with an MT system or not, from a communication perspective, for years it has been considered advisable to have the translation already in mind during the drafting phase of the source text. In fact, the preparation of documentation for translation forms part of their training for technical writers. NMT allows users to obtain translations with fewer and fewer errors of fluency or adequacy. It enables translations to be completed very quickly. Moreover, it seems to a achieve excellent results when translating many different text genres. But a text written grammatically in the target language and without translation errors may still not be an appropriate translation. Pre-editing makes it possible to ensure the appropriateness of the transition with a global audience in mind. Currently, this phase is seldom used in the translation industry. In the past, some global companies using SMT or RBMT pre- edited their original texts to avoid recurring translation errors using their own systems. With NMT pre- editing may become widespread in the industry as pant of a strategy that not only avoids translation errors but also contributes to making the raw MT output appropriate to the contexts of use of the target translation. 4. Pre-editing guidelines Pre-editing is based on applying a series of specified strategies to improve MT results when preparing content for a global audience or in controlled domains. Pre-editing helps to ensure clear communication in controlled domains targeting global audiences. In this context, the predominant textual type is informational, where there is no creative or aesthetic use of language but a literal and unambiguous use with the intention of either informing or instructing the text's recipient. The following are the most common guidelines used in communication for a global audience. and are the basis for pre-editing strategies. The aim of most of these guidelines is to increase MT effectiveness in producing grammatically correct translations that reproduce the source text "message" and also to obtain translations that are appropriate to the communicative situation of the receiver according to the text function and the context in which it is used. These guidelines can be grouped into three different categories: - Lexical choice - Structure and style - Referential elements Whatever the case, the success of pre-editing will be determined by two considerations: The function of the (source and target) text: the greater the predominance of the informative or instructive function over the phatic or aesthetic functions, the more sense it makes to pre-edit the original text. The kind of errors in the raw MT output that the chosen MT system provides and that should he avoided or minimized by pre-editing the source text. Pre-editing has two objectives: To prepare the original text so that the most error-free possible raw MT output can be obtained, and also To prepare the original text so that its translation through MT is suitable for a global audience. The pre-editing guidelines presented in this section respond to these two objectives. 4.1. Lexical guidelines The way each word or unit of meaning is processed in NMT is determined by its content and vice versa. A lexical choice in a text is linked to the range of texts and contexts in which the same choice is used. Let's take the case of a source text to be translated by MT and, consequently, to be published in several target languages in the shortest time possible. An appropriate choice of words in the source text can contribute not only to avoiding translation errors, but also to complying more effectively with the linguistic uses in accordance with the function of the text and the reason for its publication. Table below contains typical guidelines related to the lexicon. Guideline Avoid lexical shifts in register Avoid uncommon abbreviations Avoid unnecessary words Be consistent Table:Typical lexical pre-editing guidelines Explanation Avoid words that can change the style of the text orthe way it addresses the receiver. This facilitates understanding the text and normalizes the way the receiver is addressed. Only use commonly-found abbreviations. Avoid abbreviated or reduced forms that cannot be easily translated from their immediate context. Avoid unnecessary words for transmitting the information required. Using more words than needed means that the NMT system handles more word combinations and has more opportunities to propose an inappropriate or erroneous translation. Use terminology in a consistent and coherent way. Avoid introducing unnecessary word variation (that is, avoid synonymy). 4.2.Structure and style The way a text is formulated in general, and its individual sentences in particular, are as important in terms of comprehensibility as the lexicon used. The order in which ideas are interrelated. at the sentence level, throughout a text, or even intertextually, contributes to the reader's comprehension and interpretation. In the case of NMT. the options adopted in the source text activate or inhibit translation options. An unnecessarily complex and ambiguous text structure that allows objectively different interpretations increases the possibility of the NMT system proposing correct translations of microstructural elements (terminology, phrases or syntactic units) which, when joined together in the same text, generate texts that are internally incoherent, suggest a different meaning to the source text Table(a) plow. Gives premediating guidelines regarding the style and structure of the text. Most of them are not only aimed at optimizing the use of NMT systems, but also at the success of the translated text in terms of comprehensibility and meaning. Most of the guidelines listed in Table (a) are aimed at producing a simple text that can be easily assimilated by the reader of the source text. In the case of NMT engines trained with data sets already translated under these criteria, source text pre-editing helps to obtain the best raw MT output possible. Note, however, that if an engine is trained on "in-domain" data, that is, using a specialized and homogeneous dataset, based on texts of a particular genre and related to a particular field of activity, then the best possible pre-editing, if needed, will involve introducing edits that match the characteristics of that genre and domain. In addition to this general advice, in many cases it is also necessary to take into account guidelines that are specific to the source or target language. This might mean avoiding formulations that are particularly ambiguous, not only for the MT system, but also for the reader. If we take English for instance, avoiding ambiguous expressions means, for example, avoiding invisible plurals. A noun phrase such as "the file structure' could refer to both "the structure of files" and "the structure of a particular file". Although this ambiguity is resolved as the reader moves through the text, the wording of the noun phrase itself is not clear enough to provide an unambiguous translation. Another example of ambiguous structures in many languages, not only in English, is often the way in which negation is expressed. Sentences such as "No smoking seats are available." are notorious for giving rise to different interpretations and, consequently, incorrect translations. Verb tense forms are another aspect that may be simplified for the sake of intelligibility for the reader and error-free translations. Although the translation of the different verb tense forms and modes does not necessarily pose a problem for MT, an inappropriate use of verb tenses in the target language, despite resulting in well-formed sentences, can lead to target translation text comprehension errors. Typical guidance related to verb forms is given in Table (b). Table (a): Aspects related to structure and style in pre-editing Guideline Short and simple sentences Avoid unnecessarily complex sentences that introduce ambiguity. This makes it easier to understand the source text and avoids confusion. Anaphoric or cataphoric references may not be correctly handled by the NMT system and may lead to omissions or mistranslations. Avoid syntactic ambiguities subject to interpretation. Complete sentences Avoid cliding or splitting information. The compensation mechanisms for the non-typical structure of sentences do not necessarily work in the target language. For instance, a sentence written in passive form which does not take the agent explicitly into account can lead to misunderstanding in target texts. The same can happen when one of the sentence complements is presented as a list of options (e.g., in a bulleted list). In such cases, the sentence complement is broken down into separate phrases which the NMT system may process incorrectly. Remember that NMT systems only use the sentence as a translation unit, i.e., the text between punctuation marks such as full stops or paragraph breaks. Use the same syntactic structure in sentences in a list or that appear in the same context (e.g., section headings, direct instructions). This kind of consistency usually makes it easier to understand the text, both during the source publishing phase and post-publishing corrections. Active voice Where appropriate, use mainly the active voice or other structures that make “participants” in an action explicit (taking into account the conventions of the text genre and the languages involved). Homogeneous style Maintain a homogeneous style. This facilitates understanding the text, both the source and target language. This is particularly related to preparing texts for a global audience. Table (b): Aspects related to pre-editing verb forms Guideline Use the active voice Whenever possible, adopt or prioritize the active voice. Prefer simple verb forms Depending on your language pair and the MT engine, you may wish to simplify or avoid compound verb forms. For example, avoid unnecessarily concatenated verbs as they might make the sentence ambiguous or hard to follow. 4.3. Referential elements Referential elements are all those which substitute for or make reference to another element, whether in the same text (in the case of intertextual references) or outside of the text (in the case of extratextual references). The most illustrative example of this is pronouns (e.g., I, he, she, him, her, etc.) and the related category of possessive determiners such as my, his, her, etc. Pronouns with the referent in the same sentence are not usually problematic from an NMT point of view. When they are within the same sentence, there are usually no gender or number agreement problems between possessive determiners and nouns. This is also the usual case when successive pronouns throughout the text maintain the same referent (e.g., in the case of the same subject in consecutive sentences). In other cases, however, pronouns may be translated according to the way in which the training corpus treats them most frequently. This issue is particularly sensitive when the text alternates between different referents. In these cases, even though the human reading of the text leaves no doubts about who or what is the referent of each pronominal form, MT systems are usually unable to maintain this consistency and tend to homogenize the pronominal forms, using those that are most common. Pre-editing and Extratextual References Consequently, in the case of languages that reflect relationships between referent and pronominal forms through mechanisms such as gender and number agreement, the agreement may be lost. This particular kind of problem can usually be minimized by the use of simple sentences. As regards extratextual references, there are two types of references that need to be taken into account during the pre-editing phase: 1. Those that address the reader, and 2. Any cultural references the reader identifies with. From a stylistic point of view, it is best to compose simple, short sentences and use a direct style with the active voice or passive sentences that include the agent and patient. This style is especially appropriate for instructive texts. In the case of instructive texts, the direct style and active voice ensure that the text always addresses a reader directly. Machine Translation (MT) tends to reflect the most frequent usage found in the corpus, so if the target language allows the reader to be addressed in more than one way, it could alternate between different options — more or less formal or explicit — causing cohesion problems throughout the text. These issues can often be avoided through pre-editing. Extratextual references to cultural aspects with which the reader of the source text particularly identifies are difficult to deal with generically. In many cases, pre-editing the text involves making all implicit information related to these cultural references as explicit as possible while keeping the global audience in mind. In both cases (references to the reader and cultural references), MT pre-editing should take into account the target reader’s language and profile. 5. Pre-editing Tools and Resources As can be seen from the previous sections, pre-editing has to be carried out within the framework of a translation (or multilingual publication) project. The same conditioning factors that guide a translation project need to be taken into consideration in pre-editing. A style guide is used to detail how to pre-edit the original texts with and without pre-editing. It is comparable to a post-editing guide, but examples of sentences are typically provided in advance. The purpose of pre-editing guides is to provide orientation about language use when preparing text contents. These writing guidelines aim to avoid MT errors when translating the source text to different languages and to ensure that the best possible text is produced for a global audience. For this reason, they include: 1. Micro-structural writing: Recommended or required words and syntactic structures. 2. Macro-structural indications: Guidelines for guaranteeing intratextual and extratextual cohesion. When the text is embedded in a digital product or documentation linked to a consumer good, consistent use of language and referential elements (e.g., terminology) ensures extratextual cohesion. A guide oriented to content preparation contributes to extratextual cohesion by detailing the steps to follow for content to be published and translated. Such guides are also comparable to quality assurance (QA) instructions for translation. The actions required to control the quality of the source language content are typically as follows: Pre-editing Quality Assurance (QA) Task Explanation Proofing for spelling Ensure that the source text is free of spelling errors that could create and grammar comprehension difficulties for readers or MT. Using established Check that the glossary has been applied correctly and avoid unnecessary lexicon synonym variations. Use specialized and non-specialized lexicons consistently. References to the Verify that references to the reader maintain the same style as the source text. reader Style Maintain consistent language style throughout the text. Avoid style shifts. 6. Who Should Pre-edit? And When? Texts to be translated by MT have traditionally been pre-edited in-house by large corporations as part of technical writing phases, which require high proficiency in the source language. Today, with the accessibility of Neural Machine Translation (NMT), translators increasingly incorporate MT into their workflows. MT-literate translators can determine whether to use MT based on their experience or by analyzing small samples of output. If the text is unnecessarily complex, incoherent, or fails to meet the author's intentions, pre-editing may help ensure the necessary cohesion, style, and use of rhetorical devices. Translators who are skilled in the source and target languages can adapt the content to make it functional and understandable for a global audience, while minimizing errors. Pre-editing is valuable whether the content is written solely to generate a translation or is also to be published in the source language. In both cases, pre-editing ensures the quality of the original text and optimizes the use of MT. 7. Concluding Remarks The main objective of MT as a resource in translation projects is to increase productivity and reduce the time required to produce high-quality translations. Pre-editing helps minimize errors in the translated text by optimizing the source text. This ensures that translations are aligned with the original intentions and comprehensible for the target audience. When NMT can produce texts with no fluency or adequacy errors (e.g., in instructive texts), the challenge extends to translating texts with other communicative functions, such as games or marketing materials. In such cases, pre-editing ensures that translations match the intent and tone of the source text, providing added value. Pre-editing is also useful for multilingual publishing, where translators contribute their skills to refine the source text. This practice continues the long tradition of focusing on the text's intended meaning while leveraging modern technologies. Post-Editing in Machine Translation: Comprehensive Study Guide Chapter 3: How to Deal with Errors in Machine Translation: Post-editing Overview Machine Translation (MT) is an imperfect technology. While it may provide accurate translations for some sentences, others might contain: Errors in meaning Omissions Additions Stylistic issues Post-editing (PE) is the process of identifying and fixing these errors, ensuring the text is suitable for publication or distribution. Definition of Post-Editing Post-editing is a bilingual language-processing task, primarily performed by experienced professional translators, known as post-editors. It involves: 1. Understanding the source language sentence. 2. Evaluating the MT output. 3. Identifying errors and implementing revisions. Post-editing is fundamentally a revision task. Types of Errors in MT Outputs MT systems produce various error types, influenced by factors like language pair, direction, and content type. Examples include: Grammatical Errors: E.g., The cat scratches anyone which tries to touch it. Lexical Errors: E.g., The cat is very protective of her pups. Syntactic Errors: E.g., They to school went. Collocation Errors: E.g., quick food instead of fast food. Levels of Post-Editing 1. Light Post-Editing o Focus: Essential fixes for a merely comprehensible text. o Speed: Rapid implementation. o Characteristics: ▪ No stylistic improvements. ▪ Retain as much of the raw MT output as possible. 2. Full Post-Editing o Focus: Comprehensive error correction for quality comparable to human translation. o Characteristics: ▪ Stylistic changes allowed. ▪ Adherence to grammatical, syntactical, and semantic accuracy. ISO 18857:2017 Definitions Light Post-Editing: Produces a comprehensible text without aiming for human-level quality. Full Post-Editing: Produces output comparable to human translation. TAUS Guidelines for Post-Editing Light Post-Editing Full Post-Editing Ensure grammatical, syntactic, and semantic Ensure semantic accuracy. accuracy. Correct key terminology. Correct key terminology and maintain consistency. Edit offensive/inappropriate content. Edit offensive/inappropriate content. Retain as much raw MT output as Restructure sentences for improved natural flow. possible. Correct spelling where basic rules apply. Correct spelling, punctuation, and hyphenation. No stylistic changes required. Implement stylistic changes for natural flow. Objectives of Post-Editing According to ISO 18857, the goals of post-editing are: 1. Comprehensibility: Ensure the target text is understandable. 2. Content Correspondence: Align source and target language content. 3. Compliance: Adhere to requirements specified by the Translation Service Provider (TSP). Key criteria: Terminology consistency. Adherence to syntax, spelling, and orthographic conventions. Compliance with client agreements and audience suitability. Types of Post-Editing Approaches 1. Traditional MT: o Static suggestions edited or discarded by the post-editor. 2. Adaptive MT: o The MT system learns from edits in real time (e.g., Trados Studio). o Saves revisions in translation memory (TM) databases for future use. 3. Interactive MT: o MT adjusts output dynamically as the translator edits (e.g., Lilt). o Functions similarly to predictive text tools. Measuring Post-Editing Effort Effort in post-editing is measured across three dimensions: 1. Temporal Effort: Time taken to complete post-editing versus translation. 2. Technical Effort: o Number of keyboard/mouse actions. o Edit distance metrics: ▪ Levenshtein Distance: Measures minimum character changes. ▪ Translation Edit Rate (TER): Quantifies percentage of edited content. 3. Cognitive Effort: o Mental processes like reading, understanding, and decision-making. o Techniques for measurement: ▪ Think-aloud protocols. ▪ Task recordings. ▪ Eye-tracking technology. Attributes of a Post-Editor According to De Almeida & O'Brien, a good post-editor: 1. Identifies and resolves issues in MT output effectively. 2. Maintains productivity to meet daily expectations. 3. Adheres to guidelines while balancing speed and quality. Summary Post-editing is an essential task for improving MT output, ensuring it meets specific quality requirements. It involves a mix of linguistic expertise, technical know-how, and adaptability to various tools and standards. By understanding different error types, levels of post-editing, and associated efforts, translators can enhance their efficiency and the quality of the final product. Okay, here's another draft, focusing on a more concise and structured approach with bullet points and clear headings, suitable for quick review and memorization: Post-Editing in Machine Translation: A Summary I. Introduction MT Limitations: MT is imperfect; errors occur in meaning, omissions, additions, and style. 1 Post-Editing (PE): The process of identifying and correcting these errors for publishable-quality text. 2 Post-editor: The person performing PE, typically an experienced translator. PE Process: o Understand source and MT output. o Identify errors. o Develop correction strategies. o Implement revisions. Common MT Error Types: o Grammatical: Incorrect grammar (e.g., subject-verb agreement). 3 o Lexical: Wrong word choice (e.g., incorrect term). o Syntactic: Incorrect sentence structure. o Collocation: Unnatural word combinations. Error Factors: Language pair, content type, MT training data. 4 II. Levels of Post-Editing Light Post-Editing: o Focus: Essential corrections for basic comprehension. 5 o Goal: Comprehensible text, not necessarily human-quality. o Speed: Prioritized. Full Post-Editing: o Focus: Comprehensive error correction. o Goal: Human-quality output. o Speed: Less prioritized than light PE. ISO 18857:2017 Definitions: o Light: "Merely comprehensible." o Full: "Comparable to human translation." TAUS Guidelines: Offered more practical guidance on stylistic changes. ISO 18857 Objectives: o Comprehensibility o Source-target equivalence o Compliance with TSP requirements. Achieving Objectives (Key Criteria): o Terminology consistency o Correct grammar, spelling, punctuation o Standard compliance o Proper formatting o Target audience/purpose suitability o Client-TSP agreements. Core Principle: Maximize reuse of MT output. Conceptual Challenges: Linking levels to quality perceptions, translator resistance to "merely comprehensible" output. III. Types of Post-Editing Traditional PE: Editing static MT output within a TM environment. Adaptive MT: MT system learns from post-editor's edits in real time. 6 Interactive MT: MT output adjusts dynamically based on the translator's real-time input (similar to predictive text). Blurs the line of "post"-editing. 7 IV. Measuring Post-Editing Effort (Krings' Dimensions) Temporal Effort: Time spent on PE compared to translation. Technical Effort: Measurable actions (keystrokes, mouse movements). 8 o Tools: Translog (keyboard logging), Edit Distance Metrics. o Levenshtein Distance: Character-level edit distance. 9 o Translation Edit Rate (TER): Percentage of changes. Cognitive Effort: Mental processes (understanding, decision-making). Difficult to measure directly. o Methods: Think-aloud protocols, retrospective reporting, eye-tracking. V. Attributes of a Good Post-Editor (De Almeida & O'Brien) Error identification and correction skills. Efficient speed. Adherence to guidelines (minimizing over-editing). Strong translation skills and a positive attitude toward MT. This version uses more concise language, bullet points for key information, and clear headings to make it easier to scan and remember the essential concepts. This format is more suitable for quick revision before an exam.