Normal Distributions, Z Scores & Unit Normal Table (PDF)
Document Details
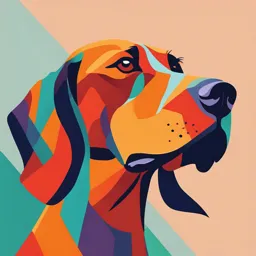
Uploaded by TransparentMusicalSaw1414
Hamilton College
Tags
Summary
This document provides an overview of normal distributions, z-scores, and the unit normal table. The text introduces the concept of normal distributions and their properties, emphasizing the use of z-scores to analyze and interpret data. It also highlights the importance of these concepts for statistical analysis.
Full Transcript
Normal distributions, The Standard Normal Distribution Z scores, and the Unit Normal Table (z table) Putting Today’s Material in Context What we’ve done before: l Described an entire set of scores using measures of central tendency and variability. What we’re doing now: l Learning more...
Normal distributions, The Standard Normal Distribution Z scores, and the Unit Normal Table (z table) Putting Today’s Material in Context What we’ve done before: l Described an entire set of scores using measures of central tendency and variability. What we’re doing now: l Learning more about normal distributions. l Learning about the relationships between the mean, the standard deviation and normal distributions. l Interpreting a single score (or a chunk of scores) within a distribution using z scores. note: we are still talking about descriptive statistics. It turns out that a lot of data (populations and samples) are normally distributed. Today, we’ll dig into what that means and why it matters so much for statistics. ~11,000 participants 60 90 120 150 Finish time - minutes BIG PICTURE: In this course we will do lots of fun things with normally distributed data. First, though, we need to learn about important properties of normal distributions Why are many variables normally distributed? l Each score in a distribution is influenced by a number of factors, and each factor is random. Runner time is influence by: age, gender, experience, fitness level, state of mind, weather conditions l The combination of these random factors makes the scores that are closest in value to the center of the distribution most common. Frequency l And the opposite is also true: extreme scores (e.g., scores far from the mean) are unlikely. l Question: Why are extreme scores unlikely? l Answer: very few people have ALL of the random factors “going their way” or ‘going against them” 𝑥̅ An unusual race score: lots of experience, high fitness Left and right level, in perfect state of mind, excellent weather. halves are mirror images l When a score is not a middle score, there are equal odds that random factors cause the score to be either above or below the mean, making the curve symmetrical. More key properties of normal distributions 50% (i.e.,.5000) 50% (i.e.,.5000) of scores are located of scores are located ABOVE the mean Frequency BELOW the mean LAMAL: 4-digit numbers Theoretical, but many variables are approximately normally distributed. Bell-shaped: mean = median = mode (and all are located at the 50th percentile). AUC = 1. [1.0 =.5000 scores are above &.5000 scores are below the mean). Symmetrical (equal chance random factors increase or decrease scores from the mean of the distribution). The mean can equal any value. (20, -5, 0.45, etc.) The standard deviation can be any positive number. (2, 1.33, 0.07, etc.) The curve has no upper or lower limits (e.g., tails are asymptotic - they don’t touch the x-axis). Scores closer to the mean are more likely than scores further from the mean. In a SPECIAL distribution, the Standard Normal Distribution, the mean is 0 and the standard deviation is 1 (too be continued...) OBSERVATION: In a normal distribution, the mean can be ANY positive or negative number, and the standard deviation can be any positive number. PROBLEM: As statisticians, one of our main activities is to find the probability (likelihood) that a particular score or sample mean will occur. With an infinite number of normal distributions, that task is very, very hard. SOLUTION: Statisticians, found the area under ONE NORMAL CURVE called “THE STANDARD NORMAL DISTRIBUTION” and figured out the exact proportions of scores that are located within each segment of the curve. We use “the standard normal transformation” (or z transformation) to convert raw scores into z scores. TRANSLATION: We can take any set of normally distributed raw scores and convert them into z scores. When raw data are normally X−X# distributed, the proportions of scores in each area of the curve of the Z score = transformed data will be the same as the proportions in the SND. s DETAILS: 1. The standard normal distribution (or z distribution) has a Q: What are all mean (!𝒙) of 0 and a standard deviation (s) of 1. these four digit numbers? 2. Scores on the x-axis in the standard normal distribution are called z scores. 3. We will practice converting raw scores (x) into z scores (using the z transformation) so that we can interpret the meaning of a single score (or a chunk of scores) within a distribution. (Is the score typical? Is it extreme?) 𝑥̅ Z Score Formula Sample: X−X# s Z= z score s x X Population: X−𝜇 s Z= € z score σ x µ Definition of a z score: how many standard deviations away from the mean a particular raw score falls – expressed in standard deviation units. Big picture: You are not trying to change the meaning of the raw score, you are converting each raw score into a different format (“a z score”). SIDE NOTE about when working with data that are not normally distributed: If your raw data are skewed, they will stay skewed even after you convert the raw scores. We call these “standard scores” instead of z scores. Keep in mind: The standard normal distribution is used to determine the probability of a certain outcome in relation to all other outcomes. After we learn more about the properties of normal distributions and z scores, we’ll use the standard normal distribution to answer these types of questions: l What percentage of scores lies at or above a z score of 2.3? l What proportion of scores falls between a z of -2.4 and a z of 0.70? Solve this problem two different ways. l If the mean on a test was 82, the standard deviation was 5, and you knew that your z score was 2.30, how do you figure out your raw score on the test? With our big goal in mind, now let’s learn more about normal distributions… Last lecture we learned about 𝑥̅ s the standard deviation (s): 𝑥̅ It represents the average amount that scores in a distribution deviate from the mean (𝑥). ̅ 0 10 20 30 40 50 60 70 80 90 100 Anxiety score (x) But the standard deviation is even more informative for data that are NORMALLY DISTRIBUTED: First notice the x-axis on this distribution. 𝑥̅ It displays raw scores (0 – 24) and also standard deviations from the mean (-3 to 3) This example: A normal distribution displaying the number of feet Let’s replace the three normal distributions 𝑥̅ that students moved from a podium when making a class presentation. with a single normal distribution to explain s the next few concepts. 𝑥̅ Now let’s bring those proportions back… 0 10 20 30 40 50 60 70 80 90 100 In this example, it For normal distributions with any mean would extremely and any variance, we can make the In this example, unusual/unlikely to see a score of 25 following three statements: ~95% of scores in this data set. will be between 4 and 20 feet. The Empirical Rule (aka: the 68, 95, 99.7 rule) Approx. 68% of all scores lie within 1 sd of the mean 𝑥̅ Approx. 95% of all scores lie within 2 sd of the mean Approx. 99.7% of all scores lie within 3 sd of the mean Let’s add up the proportion of scores in a normal distribution that fall within 2 standard deviations of the mean (aka between 4 and 20 feet)..3413 +.3413 +.1359 +.1359 =.9544 We are VERY interested in the tails! In this example, it RELEVANCE: Scientists compare their would extremely In this example, experimental data to statistical norms ~95% of students unusual/unlikely to (proportions in the normal curve) to moved between see a score of 25 in this data set. determine if they have ‘unusual’ scores 4 and 20 feet from the podium The Empirical Rule (aka: the 68, 95, 99.7 rule) Approx 68% of all scores lie within 1 sd of the mean 𝑥̅ Approx 95% of all scores lie within 2 sd of the mean Approx 99.7% of all scores lie within 3 sd of the mean Properties of z scores lThe mean of a set of z scores = 0 l The SD of a set of z scores will always equal 1. Let’s see why… Assume 𝑥̅ = 50, s = 10 When you standardize And the standard deviation a set of scores, the becomes 1 because any mean is converted value that is 1 SD above Frequency to 0 because the the mean will become value that represents X−X# 1 when you convert the mean (e.g., 50), it to a Z score. minus itself (50), s will equal zero. X- 𝑥̅ z score A visual explanation of why the standard deviation of a set of z scores equals 1. Below is a distribution of raw scores X−X# Frequency 3 2 Z = s 10 19 − 8 −16 Z= 23 Mean (raw Mean (raw score) score) == 8 19 16 10 22 12 Z=1 MeanMean (stndrd score) == (z score) 00 11 22 The x value (raw value) that is one standard deviation above the mean is always going to be equal to a z score of 1. When you standardize any distribution (regardless if it is a normal or skewed distribution of scores), what properties are affected? l Mean? l YES (the mean becomes 0; the value changes) l Standard deviation? l YES (the sd becomes 1; the value changes) l Skewness? l NO (distribution will stay the same shape) l Kurtosis? l NO (distribution will stay the same shape) THM: Just by knowing the MEAN, the STANDARD DEVIATION, and that this variable is NORMALLY DISTRIBUTED, we know A LOT about this population (or sample) 4 key characteristics of the standard deviation 1. SD is always positive. 2. SD describe quantitative data (i.e., in this class, we’ll calculate the SD only when we work with ratio or interval data). 𝑥̅ 3. Since the SD represents the average distance from the mean, the SD is most often reported WITH the mean. 4. SD is affected by the value of EACH SCORE in a distribution. OYO: READ PRIVITERA!! (ch 3, ch 4, ch 6) A z score is a value on the x-axis of a standard normal distribution. The numerical value of a z score specifies the distance or the number of standard deviations that a value is above or below the mean. The standard normal distribution, or z distribution, is a normal distribution with a mean equal to 0 and a standard deviation equal to 1. The standard normal distribution X−X# Z score = is distributed in z score units along the x-axis. s Reaching each learner: Because students learn in different ways, we will use three different examples to practice working with raw data, means, standard deviations, z scores and distributions. 1. Exam scores 2. Race times (swimmers) 3. Text messaging (Worksheet 5) Example 1 – exam scores - Calculating z scores and using the z table - Determining probabilities and percentages - Working ‘backwards’ from z scores to raw scores I got a I got a 76% on 76% on the exam the exam! You just learned that you scored a 76% on an Spanish exam Q: What else would you want to know? - the class mean (𝑥)̅ - the standard deviation (𝒔) # ) and Okay, let’s have a look at the class mean (𝒙 standard deviation (s) to determine if a 76% is worth a celebration… Let’s transform! Spanish grades X−X# 76 − 70 6 =2 Frequency 3 s 3 3 In this data set, a score of 76 is 2 z scores above the mean. 𝑥̅ = 70 76 Question: How does your exam score compare to the rest of the class? 1359 +.3413 +.3413 +.1359 +.0215 +.0013 =.9772.0298 Answer: BUENO! You scored equal to or higher than 97.72% of the class. Answer: You could also say that only 2.98% of the class scored equal to or higher than you did. Next: Let’s learn how to use the Unit Normal Table (i.e., the z table) using this example. a score of 76 is equal to or higher than 97.72% of the other scores. #$%#& z= ' = + 2.0 Or, only 2.28% of scores are equal to or higher than a score of 76. Step 1: locate the z score (2.00) in column A Step 2: Find the proportion in the C column (.0228) or use the b column and add.5000 NEXT: Converting from a z score (z) to a raw score (x) On the next exam in your Spanish class, the mean on the exam is 92 and the standard deviation of the mean is 2.3. Spanish grades Your prof tells you that you earned a Frequency z score of -0.33. 2.3 What is your exam score? x Solution: 92 Step 1: Always draw the distribution! X−X# (%)* Z = -0.33 = *.' Step 2: Let’s do some simple math s (2.3) * -0.33 = 𝑥 − 92 Bueno! You got an A! -0.759 = 𝑥 − 92 92 - 0.759 = 𝑥 𝑥 = 91.24 % OYO: Below are the data (mean, s) generated by two other sections of Spanish class. For each class compute the z transformation and use the z table - Calculate a the z score for an exam grade of 76 in each class - State the proportion of scores that are higher than 76 (use z table and this should be in the form.xxxx) X−X# - State the percentage of scores that are lower than 76 s - Which class would you rather get a 76% in and why? Section 1 Section 2 Section 3 s = 3 9 1.7 𝑥̅ = 70 72 83 #$%#& z= = + 2.0 ' Higher =.9772 proportion Lower = 2.98% percent What’s the benefit of transforming raw scores to z scores? Allows us to compare raw scores from different distributions Example 1: comparing SAT (800) and ACT (36) scores Example 2: comparing athletic race times from different decades Reaching each learner: Because students learn in different ways, we will use three different examples to practice working with raw data, means, standard deviations, z scores and distributions. 1. Exam scores 2. Race times (swimmers) 3. Text messaging