Levels of Measurement & Frequency Distributions PDF
Document Details
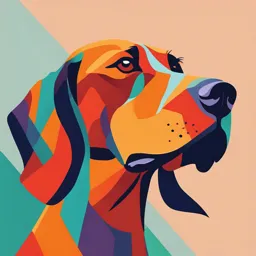
Uploaded by TransparentMusicalSaw1414
Hamilton College
Professor Robinson
Tags
Summary
This document outlines different levels of measurement (nominal, ordinal, interval, and ratio) and frequency distributions in the context of statistics and research methods. It also explains populations vs. samples and parameters vs. statistics.
Full Transcript
Levels of Measurement & Frequency Distributions Statistics and Research Methods Psych/Neuro 201 Professor Robinson Outline I. Quick overview: A. Populations vs. samples B. Parameters vs. statistics C. Descriptive vs. inferential s...
Levels of Measurement & Frequency Distributions Statistics and Research Methods Psych/Neuro 201 Professor Robinson Outline I. Quick overview: A. Populations vs. samples B. Parameters vs. statistics C. Descriptive vs. inferential statistics D. Conceptual vs. Operational definitions II. Levels of Measurement III. Frequency Distributions & Tables Populations vs. Samples Population — set of all individuals of interest in a particular study Sample — set of individuals selected from a population, usually intended to represent the population in a study — for lab experiments, generally NOT a random sample (usually a convenience sample) Parameters vs. Statistics Parameters and statistics both PP and SS refer to values, usually numerical (e.g., means, frequencies, standard deviations) Describe using a parameter. Describe using a statistic. Because it’s usually impossible to study entire populations, we estimate population parameters using sample statistics. Descriptive vs. Inferential Statistics Statistics Purpose: To describe a Purpose: To make inferences sample about populations from samples Types: frequency For example: Do people who counts, means, standard meditate differ significantly deviations from people who do not? That is, do they represent But we’re usually not interested two different populations? in the samples themselves, but rather in what they can tell us about the populations the Types: correlations, t tests, samples come from. ANOVAs, chi-square tests Review of how variables are described Qualitative vs Quantitative data Numbers-based, countable or measurable Interpretation-based, descriptive, relating to language Categorical vs Numeric variables Have values that describe a measurable quantity as a number such as “how many” or “how much” A variable that is not numerical - instead it is based on a qualitative property, such as color, breed, or gender, among others Discrete vs. Continuous variables an infinite number of possible values that can be measured (145.5 pages) A finite number that can be counted (12 naps) Conceptual Variables vs. Operational Definitions Conceptual Variable Fear in (abstract) rats Operational # of seconds Whether or not Definitions # of seconds rat spends a startle reflex (concrete, rat spends against wall of (whole body observable freezing box rather than flinch) is (immobile) indicators) in center observed What Can We Do to Reduce the Amount of Error in our Inferences? 1. Strive for a representative sample (i.e., as much like the broader population as possible). Hard to do this for experiments! Often have to rely on convenience samples. 2. Use relatively large samples BUT: Representativeness is more important than size! Better U.S. election estimate with representative sample of 200 voters than with unrepresentative sample of 1 million voters Outline I. Quick overview: A. Populations vs. samples B. Parameters vs. statistics C. Descriptive vs. inferential statistics D. Conceptual vs. Operational definitions II. Levels of Measurement III. Frequency Distributions & Tables Examples of the four levels of measurement 1. Do you exercise? (circle one: NO YES) Nominal 2. How often do you exercise? Ordinal 1 2 3 4 1. ranked, but not equal intervals 1-2 3-4 5 or more 2. ‘not enough information’ Never days/week days/week days/week 3. How often do you exercise? Interval (rating scale from 1 = not at all to 7 = very frequently) 1 2 3 4 5 6 7 Not at Very all frequently 4.3. Write down the number of minutes you exercise each week: ___ Ratio Take home message (THM): The same variable (exercise) can be assessed at different levels of measurement. It depends on WHAT the experimenter wants to measure and HOW the experiment is designed Four Levels of Measurement Nominal Qualitative Use numbers merely as labels for categories (No = 1, Yes = 2) (apples = 10, oranges = 11, grapes = 12) Quantitative Ordinal Categories are ranked in terms of size or magnitude (sml, med, jumbo) You cannot tell if the distance (amount) between categories is equal. Interval Has ordinal properties, PLUS: all categories form intervals that are exactly the same size. No absolute zero point, so ratios of scores aren’t meaningful. Ratio Has interval properties, PLUS: a “true” zero point, ratios of scores are meaningful. Four Levels of Measurement Nominal Qualitative Use numbers merely as labels for categories (No = 1, Yes = 2) (apples = 10, oranges = 11, grapes = 12) Quantitative Ordinal Categories are ranked in terms of size or magnitude (sml, med, jumbo) You cannot tell if the distance (amount) between categories is equal. Interval Has ordinal properties, PLUS: all categories form intervals that are exactly the same size. No absolute zero point, so ratios of scores aren’t meaningful. Ratio Has interval properties, PLUS: a “true” zero point, ratios of scores are meaningful. How I teach: We are going to use this Example of data collected from an interval scale particular example through several lectures. “When I’m going through a very hard time, I give myself the caring and tenderness I need.” 1 2 3 4 5 Almost never Rarely Sometimes Often Almost always 2 3 4 2 4 1 2 3 5 54 participants 1 3 2 5 4 3 4 3 2 These data were filled out a one- 3 3 4 1 2 4 3 1 4 collected using question survey 2 4 2 3 3 5 2 4 3 an interval scale (1-5) 3 4 3 2 4 3 1 4 2 of measurement 5 3 2 3 1 3 3 2 4 Item 6 from the 12-item Short Form of the Self-Compassion Scale (Raes et al., 2011) Examples of data collected using an ordinal scale v Body type Number of naps v slim, average, heavy v Education level 1=0–1 v high school 2=2–4 v some college 3=5–7 v college 4 = 8 or more v masters degree v PhD (doctorate) Note: other than Exam 1 and a few homework assignments, in this statistics course, we will not work with ORDINAL data Anticipating confusion v Education level education code 5 3 4 2 1 4 2 3 3 high school 1 1 3 2 1 2 3 3 3 4 These data were some college 2 2 3 1 5 2 2 3 1 3 collected using an college 3 3 1 2 3 3 5 3 3 5 ORDINAL scale masters degree 4 3 4 3 2 5 3 1 3 2 of measurement PhD 5 4 3 2 3 1 3 3 2 2 v Self compassion 1 2 3 4 5 2 3 4 2 4 1 2 3 5 Almost Rarely Sometimes Often Almost 1 3 2 5 4 3 4 3 2 never always 3 3 4 1 2 4 3 1 4 1 2 3 4 5 2 4 2 3 3 5 2 4 3 never always 3 4 3 2 4 3 1 4 2 5 3 2 3 1 3 3 2 4 These data were collected using an INTERVAL scale of measurement Levels of Measurement Summary Equal & Meaningful order? meaningful Can the categories be RANKED distance between True 0? Type of data intervals? Nominal No Qualitative Ordinal Yes No Quantitative Interval Yes Yes No Quantitative Ratio Yes Yes Yes Quantitative Minutes spent waiting for your Grubhub delivery Concentration at Hamilton Ranking of favorite food Clumsiness (1 = not at all clumsy, 9 = very clumsy) Here is an example of a type of question you could see on Exam 1 Studying the behavior of rodents can help us understand anxiety disorders. In the “HOLE BOARD TEST” a rat is placed into an enclosure with a flat floor that has a number of holes. Rats dip their heads into the holes to investigate them. Rats that explore fewer holes are considered to have greater anxiety-like behavior. Data on the number of holes explored by a rat were collected using the following scale. 0 – 2 holes 3 – 5 holes 6 – 8 holes 9 – 11 holes 12 – 14 holes \ Level of measurement: ____________ Why? (explain): ________________________________________________ ________________________________________________________ Here is a strong answer (notice how complete and detailed the answer is…) Studying the behavior of rodents can help us understand anxiety disorders. In the “HOLE BOARD TEST” a rat is placed into an enclosure with a flat floor that has a number of holes. Rats dip their heads into the holes to investigate them. Rats that explore fewer holes are considered to have greater anxiety-like behavior. Data on the number of holes explored by a rat were collected using the following scale. 0 – 2 holes 3 – 5 holes 6 – 8 holes 9 – 11 holes 12 – 14 holes \ Level of measurement: _Ordinal____ Why? (explain): These data can be ranked (so that eliminates nominal LOM), and there is SOME evenness in the intervals, but because the five options contain a range to choose from (e.g., 3-5), the experimenter does not know the specific distance between intervals (so that eliminates interval and ratio LOM). In addition, there is no true zero (so that again eliminates ratio LOM). OYO: Worksheet 1: Levels of Measurement Outline I. Quick overview: A. Populations vs. samples B. Parameters vs. statistics C. Descriptive vs. inferential statistics D. Conceptual vs. Operational definitions II. Levels of Measurement III. Morling textbook study guide (1 slide) IV. Frequency Distributions Statistics and Research Methods – Morling Text study pointers for Exam 1. Robinson For the most part, the exams in this statistics course will be centered on material that is directly covered in lecture. Exam 1 is an exception. Specifically, Exam 1 will include questions from the Morling textbook reading that I DO NOT COVER IN LECTURE. To help guide your studying, here are some broad areas from the Morling textbook that you should focus on while studying for Exam 1. Please start early. Please work with a buddy. Chapter 1 - Know the definitions and differences between applied, basic and translational research Chapter 2 - pgs 23 – 26, pgs 36-53 - Know the importance of a comparison group - Know the limitations of authority figures and personal experience - Know the terms confederate, confounds, probabilistic, availability heuristic, present/present bias, confirmation bias - Know the different ways that scientists share their work. For example, know the differences between empirical articles and review articles, and be able to describe meta analyses - Be able to describe and identify features of the different sections of an empirical article (Abstract, Introduction, Method, Results, Discussion, References) Chapter 3 – pgs 56-59, Chapter 5 – pgs 117 – 124 - Know what construct validity is and how to describe it - Know about variables and their levels, and be able to come up with your own examples -measured, manipulated, conceptual, operational - Know about types of measures – observational, physiological - Know about levels of measurement (nominal, ordinal, interval, ratio) - Know the terms qualitative vs. quantitative; discrete vs. continuous Chapter 6 – pgs 153 – 165 - Know how to make a good survey, and what makes a bad survey - Know what a Likert-type survey is - Know how to write good questions (for a survey or an experiment) - key terms: open ended questions, forced choice questions, leading questions, double barrel questions, negative wording, question order, acquiescence, fence sitting, desirable responding, faking bad Frequency Distributions Imagine that you are a psychology major who is interested in studying the transition to college. One of your main interests is the development of independence. For your first project, you collect data from 50 first-year students and 50 college seniors. In your first survey, you ask only one question: “How many times do you text your parent/guardian during a 24-hour period?” Raw data: First-year students Raw data: College seniors 9 8 9 7 7 8 9 8 10 9 2 6 3 0 4 3 5 7 3 1 8 8 9 8 8 10 6 9 10 How 7 I teach: 7 3 5 1 2 5 3 2 4 4 You’ll work with these 8 8 7 9 8 9 7 8 8data in 7 some of4your2 0 6 4 1 1 2 3 7 practice worksheets, too 7 9 9 7 8 8 8 7 9 8 5 2 6 3 4 6 5 4 3 2 9 7 7 8 8 9 7 8 8 9 1 6 4 5 4 2 3 3 0 5 These numbers are the ”x-values” These are the data that we want to analyze We have a few different ways to visualize the raw data: “How many times do you text your parent/guardian during a 24-hour period?” Frequency histogram Frequency tables 20 1st yrs seniors x f x f 0 0 0 3 15 1 0 1 5 2 0 2 8 Frequency 3 0 3 10 10 4 0 4 9 5 0 5 7 6 1 6 5 7 12 7 3 5 8 20 8 0 9 14 9 0 10 3 10 0 0 11 0 11 0 0 0 1 2 3 4 5 6 7 8 9 10 Number of text messages Frequency tables can be Frequency histograms let us see the expanded to provide different ’shape’ of our data. types of information. Let’s have a closer look at shapes… Let’s add some columns next. Shape of a distribution: Many variables (e.g., number of text messages sent to parent) are ‘normally distributed.’ 0 10 20 30 40 50 60 70 80 90 100 Anxiety score (x) Modified from Privitera: Fig 6.1 This graph displays three examples of normal distributions, but with two different means and three different standard deviations (not drawn). Notice: AUC is equivalent for all three curves In stats, we have a specific name for the shape of a curve: Kurtosis: A measure that describes the shape of a distribution leptokurtic f mesokurtic platykurtic X platy- (flatness, broad) meso- (middle, intermediate) lepto- (fine, thin) platypus m for leap up! plateau middle Note: We’ll return to this when we discuss variability and the standard deviation… Frequency histogram Frequency tables 20 x f x f 0 0 0 3 15 1 0 1 5 2 0 2 8 Frequency 3 0 3 10 10 4 0 4 9 5 0 5 7 6 1 6 5 7 12 7 3 5 8 20 8 0 9 14 9 0 10 3 10 0 0 11 0 11 0 0 0 1 2 3 4 5 6 7 8 9 10 Number of text messages Frequency tables can be Frequency histograms let us see the expanded to provide different ’shape’ of our data. types of information. Let’s have a closer look at shapes… Let’s add some columns next. Let’s examine the data from the college seniors… This column describes the percent of This is the participants who data column. selected each Folks, we’ll use these first two We call this response. You can also columns (and add some other columns the find the same “x column” information that we will add later) A LOT this here semester x f rf % cf crf 20 11 0 0.00 0 50 1.000 For example, 20% of college seniors in this sample 10 0 0.00 0 50 1.000 15 send 3 texts a day to their 9 0 0.00 0 50 1.000 parent 8 0 0.00 0 50 1.000 Frequency 7 0 0.06 6 50 1.000 10 6 5 0.10 10 47 0.940 5 7 0.14 14 42 0.840 5 4 9 0.18 18 35 0.700 3 10 0.20 20 26 0.520 2 8 0.16 16 16 0.320 0 1 5 0.10 10 8 0.160 0 0 1 2 3 4 5 6 7 8 9 10 0 3 0.06 6 3 0.060 Number of text messages And finally, in this sample, 52% of This is the frequency (f) column. If you sum college seniors send 3 or fewer text this column, you will know exactly how many messages/day to their parents. college seniors participated in the study. Here are some terms for you to review OYO X = the raw data (if interval data, the scale units) f = the frequency (how often each x value occurs) x f rf % cf rcf 11 0 0.00 0 50 1.000 rf = the relative frequency 10 0 0.00 0 50 1.000 9 0 0.00 0 50 1.000 how often each x value occurs divided by N 8 0 0.00 0 50 1.000 7 0 0.06 6 50 1.000 % = the relative frequency expressed as a percentage 6 5 0.10 10 47 0.940 5 7 0.14 14 42 0.840 4 9 0.18 18 35 0.700 cf = the cumulative frequency (the total of all the 3 10 0.20 20 26 0.520 frequencies. You add the frequencies “up” the 2 8 0.16 16 16 0.320 column 1 5 0.10 10 8 0.160 0 3 0.06 6 3 0.060 cf = the sum of all the cumulative frequencies in the data set. SPSS Printout of Frequency Tables First-year students College seniors In SPSS, the values are listed from lowest to highest. It doesn’t matter, as long as you add the cumulative frequencies and percentages in the right direction. (so they represent those AT OR BELOW a certain value). QUESTION: If the data are qualitative in nature (i.e., nominal), would cf, crf, and cumulative % be meaningful? Valid %: Denominator is # of people who answered the question (excludes missing data). Same as % in this case b/c only 1 missing data point out of 2,867 No! Nominal scores are not ordered, so seeing scores at or “below” a given value (cumulative %) doesn’t make sense. (e.g., % of scores below widowed) Note: SPSS will produce a frequency table with cumulative % for nominal data, but it should not! Question: Can we still construct a frequency table if the number of possible values is very large? e.g., scores on a test (0 – 100%), SAT scores, GPA, household incomes, ages of people in a large data set Answer: Yes! Use a grouped frequency distribution Usually between 5 and 15 groups You have to have enough categories to group data. THUS, if you only have four or fewer groups, you would not make a grouped frequency distribution Just know that these exist. I won’t ask you to create one. Advantages of Frequency Tables Aid in identification of: Outliers: Cases with extreme scores Ceiling/floor effects: When a large portion (~75%) of your sample is at the top/bottom of the distribution) This distribution seems OK (no ceiling or floor effects), though it’s a bit skewed. A little more about depicting data visually When do we use a bar graph vs. a histogram? Marital Status Test scores Use a bar graph for Use a histogram for qualitative data quantitative data (nominal) (ordinal, interval, ratio) Bars should not touch Bars should touch (but when you make yours in SPSS they will not quite touch, don’t worry about it) Skewness: looking at histograms tells a lot. Negatively Skewed ~Symmetric Positively Skewed Frequency Frequency Frequency Nitty picky but real: don’t say “this is skewed to the left”. Instead, say “this distribution is negatively skewed”