Metrology: Errors and Uncertainties (PDF)
Document Details
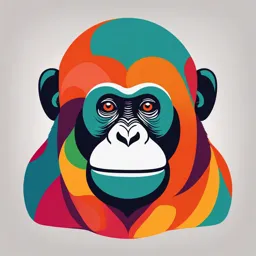
Uploaded by FavorableTiger2547
Riga Technical University
Claudio Rochas
Tags
Summary
These lecture notes cover metrology, focusing on errors and uncertainties in measurements, particularly in energy and environmental engineering. The document explains different types of errors, including measurement blunders, systematic errors, and random errors, along with methods for estimating systematic errors, provides examples, and discusses the use of standard deviation in metrology.
Full Transcript
METROLOGY Applied to energy and environmental engineering ERROR and UNCERTAINTY Claudio Rochas Basic idea Whenever a measurement is made it is unlikely that the measured value will equal the true value; which is the actual or exact value of the measured variable The differe...
METROLOGY Applied to energy and environmental engineering ERROR and UNCERTAINTY Claudio Rochas Basic idea Whenever a measurement is made it is unlikely that the measured value will equal the true value; which is the actual or exact value of the measured variable The difference between the measured value and the true value is the error: ◼ True value X (or also indicated as μ) ◼ Measured (best) value xbest ◼ Absolute error δx=|xbest - X | 𝛅𝐱 𝐱 𝐛𝐞𝐬𝐭 −𝐗 ◼ Relative error 𝛆𝐱 = = 𝐱 𝐛𝐞𝐬𝐭 𝐱 𝐛𝐞𝐬𝐭 True value (X) Measured value (xbest) Voluntary exercise: You are given a set of measured values and 100°C 100.1°C their corresponding true values. Your task is to calculate: 0.5°C 0.0°C Absolute error (δx) 373 K 373.1 K Relative error (𝛆𝐱 ) in percentage 273.5 K 273 K 2 Type of errors ◼ Measurement blunders (big mistakes) ◼ Systematic (bias) errors ◼ Dynamic errors ◼ Random errors Medium of Analysis and interest interpretation Signal Sensor Display Recorder processor 3 Measurement blunders (big mistakes) These errors may arise due to inadequacies of the calibration, instabilities of the transducer, limitations of the data-acquisition system or errors or loss of information Measurement blunders do occur Example: The measurement of liquid temperatures usually involves using an immersed sensor and a For example, a temperature sensor protection tubes or thermowells can be wrongly located or even its location is unknown 20°C The data arising from measurement blunders should generally be ignored 60°C https://www.thermosensors.com/thermowells/ 4 https://www.emerson.com/ Systematic errors - bias ▪ Bias error are a constant offset between the average indicated value and the true value ▪ Bias error remains constant during a series of measurements ▪ Repetition of more and more measurements does not assist in either eliminating or reducing the bias error Measured value, x xത Systematic error μ True value Number of measurements 5 Estimation of systematic errors The level of bias error can only Corrections identified due to a bias be estimated by comparison: error should be incorporated into the data: ◼ by calibration ◼ by concomitant methodology, ◼ If a calibration reveals a bias error of which is the use of different 0.5°C overestimate of temperature then methods to estimate the same all the results should be adjusted by this thing and comparison of the results amount (Nicholas and White, 1994) ◼ by inter-laboratory comparisons of ◼ the uncertainty associated with the measurements of the same calibration itself will remain and quantity propagate into the uncertainty of the result 6 Example of systematic errors ◼ Defects of the instrument uncalibrated instrument = no agreement between the "true value" of the quantity and the response of the instrument ◼ Wrong working conditions some instruments are calibrated to work at certain conditions (temperatures, pressure, moisture, etc.) and provide untrue answers if used at other conditions ◼ Instrument-surveyor interaction parallax error due to a wrong angle of the surveyor with respect to the scale of the instrument ◼ Instrument-medium of interest interaction In measuring the temperature of a fluid with a thermometer what is actually measured is the temperature of the thermometer-fluid system after reaching thermodynamic equilibrium 7 Instrument-medium of interest interaction Example of systematic error in temperature measurement ▪ The measurement of liquid temperatures usually involves using an immersed sensor and a protection tubes or thermowells Cylindrical fin for inserting temperature sensor x L Flow Tf Pipe wall https://www.badotherm.com/products/thermowells/ 8 Cylindrical fin ◼ Cylindrical fin provides a conduction path between the support and the transducer, which affect temperature measurement ◼ Can be modelled as a fin of constant cross-section Conduction in x-direction Coveting cooling/heating from external surface 9 Steady-state analysis, assuming no changes in the internal energy of the fin element Applying the principle of conservation of energy to the finite element and the Fourier’s law: qx = qx+dx + dqs dT qx = −kA dx The heat rate (𝐪𝐱+𝐝𝐱 ) at the right side of the The differential heat transfer rate dqs element can be approximated using a 1st-order due to convection is determined by the Taylor series expansion Newton's law of cooling p is the perimeter of the cross dq x d2 q x q x+dx = qx + dx + dx 2 + ⋯ section 1! dx 2! dx 2 dqs = h dAs T − Tfluid dAs p= dAs = 𝑃dx dx Higher order terms that can be neglected qx = qx+dx + dqs dT dT d dT −kA = −kA − kA dx + hP T − Tf dx dx dx dx dx 10 ◼ Cylindrical fin provides a conduction path between the support and the transducer, which affect temperature measurement ◼ Can be modelled as a fin of constant cross-section in steady state conditions d2 T hP 2 − T − Tf = 0 Where: dx k As T = local fin temperature (K) x = location away from the fin base (m) ◼ Under transient conditions, the balance As = the cross-sectional area (m²) equation becomes: P = fin perimeter (m) K = thermal conductivity of the fin (W/m K) h = heat transfer coefficient (W/m²K) dT d2 T h P Tf = temperature of the surrounding fluid (K) = 2− T − Tf dt dx k As 11 ◼ Solution assuming negligible loss from the tip of the fin, gives: T − Tf cosh m(L − x) Where: = L = length of the fin (m) Tb − Tf cosh mL Tb = temperature at the base of the fin (K) ℎ𝑃 m= At the tip, x = L, the error can be defined as = Tf –TL 𝑘As Tf − Tb ◼ For a cylindrical fin, P = 2πr and Ac = π r² 𝐸𝑟𝑟𝑜𝑟 → Tf − TL = cosh mL 2ℎ Sensors with this response are known as first- m= 𝑘𝑟 order systems 12 If you are interested in the solution d2 T dx2 − hP k As T − Tf = 0 1. Rewrite the Equation: Simplify the equation as: where 2. Let θ=T−Tf, where θ represents the temperature difference between the fin and the fluid: 3. The general solution for θ(x) is: 4. Since θ=T−Tf : 5. The constants C1 and C2 are determined by the boundary conditions of the specific problem, such as: 1. Temperature at the base of the fin (x=0) T=Tb 2. Temperature at the tip of the fin (x=L) T=Tf T − Tf cosh m(L − x) At x=0 → T=Tb → = Tb − Tf cosh mL ℎ𝑃 m= 𝑘As At x=L → T=Tf → 13 Systematic error in temperature measurement Calculation example Determine analytically the temperature indicated by the transducer under the following measurement set-up ◼ A 10 mm external diameter stainless steel sheathed thermocouple is inserted into a pipe containing a fluid ◼ The heat transfer coefficient is h=140 W/m²·K ◼ The fluid temperature is Tf=90°C ◼ Length of the sensor protruding from the wall is L=60mm ◼ The transducer is located 5 mm from the tip of the sheath ◼ The pipe wall temperature is 30°C. ◼ The thermal conductivity of the sensor is 16 W/m·K ◼ The effects of heat transfer by radiation can be neglected 14 T − Tf cosh m(L − x) = X = L-5=60-5=55mm=0.055m Tb − Tf cosh m L Tf − Tb 2ℎ 𝐸𝑟𝑟𝑜𝑟 → Tf − TL = m= 2ℎ 2×140 cosh mL 𝑘𝑟 m= = = 59.2 𝑘𝑟 16×0.005 T − 90 cosh 59.2(0.06 − 0.055) = = 0.06 30 − 90 cosh 59.2 × 0.06 So the temperature indicated by the transducer will be T = 86.4°C. 𝑇 = 0.06 × 30 − 90 + 90 = 86.4°C This is 3.6°C below the true temperature of the fluid The error is proportional to Tf – Tb so insulating the wall or pipe and elevating Tb will help minimize the error. If sensor selection is an option, choosing one with a small radius, low thermal conductivity, and a large immersion length is preferable. Note: this analysis did not take into account the detailed assembly of the sensor. In practice most of the sensors consist of a multi-core assembly with the transducer isolated electrically from the sheath by a ceramic insulator with low thermal conductivity medium/material 15 Voluntary exercise Perform a sensitivity analysis on the error in temperature measurement based on the given statement: The error is proportional to Tf – Tb so insulating the wall or pipe and elevating Tb will help minimize the error. If sensor selection is an option, choosing one with a small radius, low thermal conductivity, and a large immersion length is preferable Define the Parameters: Identify the key variables affecting the error. Consider sensor properties (included in m) and immersion length (L) Mathematical Model: Express the error as a function of these variables Propose an equation or system of equations describing how the error changes with sensor properties, and temperature differences. Sensitivity Analysis: Vary each parameter systematically while keeping others constant Determine how sensitive the error is to changes in each parameter Present the results in a graph showing the impact of each factor Discussion and Interpretation: Interpret the results: Which parameters have the highest influence? Suggest practical recommendations based on your findings. Discuss any assumptions made and potential limitations. Note: Implement the analysis using a computational tool such as Excel 16 60cm 55cm https://www.minco.com/using-thermal-ribbon-sensors-for- non-invasive-temperature-measurement-of-liquid-within-a- pipe/ 17 Dynamic errors Dynamic error: the difference between the true value of the The deviations that occur in a system's output measured quantity to the value shown by the measuring due to its inability to instantly respond to changes instrument under varying conditions in the input. These errors arise in dynamic systems where the response is time-dependent, Response time: the rapidity of the measurement system that often due to inertia, delays, or the system's responds to the changes in the measuring variable inherent dynamics NOTE: ◼ In many applications the temperature of an object is being measured is not constant but varies with time ◼ Even if the object's temperature were constant, introducing a transducer into thermal contact with it would cause heat transfer between the two if their temperatures were initially different. This heat exchange alters the object's temperature and continues over a certain period until thermal equilibrium is reached ◼ No heat transfer process results in instantaneous temperature changes. The time required for a temperature sensor to adjust to a value approaching that of the application is known as its response time 18 Main types of Dynamic Errors Lag Error Imagine placing a thermometer at 20°C in hot water. Instead of instantly displaying the water temperature (e.g., 80°C), the When a system does not immediately follow input thermometer takes time to adjust. This delay happens because changes, causing a time delay heat transfer from the water to the thermometer probe requires time Overshoot and Undershoot Consider a digital temperature sensor with a control system that adjusts a heater. When the heater is turned on to reach 100°C, the When a system initially exceeds or falls short of its sensor may initially measure 105°C before stabilizing at 100°C final steady-state value (overshoot). Similarly, if cooling is applied, the temperature might momentarily drop below the setpoint (undershoot). A bi-metallic strip thermometer in an industrial furnace may show Hysteresis Error 250°C when heating up but only return to 245°C when cooling When output depends not only on the current input down, even if the furnace temperature is the same. This is but also on past inputs because the sensor response depends on the direction of temperature change. 19 Dynamic errors Example in temperature measurement Transient conduction can be modelled in several ways. The simplest is to assume that temperature gradients within a body are negligible and performing an energy balance for the system (lumped capacitance method) ◼ The temperature variation of a body exposed to a convective boundary condition can be evaluated with respect to time by: Where: T − T∞ h As ρ = density (kg/m³) = exp − t As = surface area of the body (m²) Ti − T∞ ρ V cp cp = specific heat at constant pressure (J/kg K) V = volume of the body (m³) ρ V cp h = heat transfer coefficient (W/m²K) τ= t = time h As T∞ = bulk fluid temperature (K) Ti = initial temperature (K) h Lc τ = defines the characteristic speed of response of the system Bi = < 0.1 Bi = Biot number k Lc = characteristic length V/As (m) K = thermal conductivity (W/m K) 20 If you are interested in the solution This derivation is based on the lumped capacitance model, which applies when the Biot number (Bi) is much less than 1, the body is considered thermally thin, and the temperature is assumed uniform throughout the body 1. Energy conservation: ℎ 𝐿𝑐 𝐵𝑖 = 99% 23 ✓ Time constants help determine the relationship between a continuously varying process temperature and the temperature indicated by a sensor ✓ If the application temperature fluctuates, the sensor in thermal contact will also register changes ✓ Due to the sensor's thermal characteristics, there will be: A time delay between the actual temperature change and the sensor's response. A temperature difference between the application and the sensor reading 24 Example – Sinusoidal Temperature Variation ◼ When the application temperature varies AIN sinusoidally, the sensor's response will lag due to AOUT = thermal inertia. ω2 τ2 + 1 attenuation factor ◼ As the application temperature reaches its maximum, the sensor continues registering an ϕ = arctan(ωτ) increase before catching up. ◼ Similarly, as the temperature begins to decrease, Where: the sensor may still indicate a rising temperature. ◼ AOUT = output amplitude ◼ First-Order System Model: A thermometer can be ◼ AIN = input amplitude modelled as a first-order system, where the sensor's ◼ Φ = phase lag (rad) response to a sinusoidal input follows a phase- ◼ ω = angular frequency of a sine wave (rad/s) shifted sinusoidal output. ◼ τ = time constant 30 25 20 15 10 5 0 0 2 4 6 8 10 12 14 16 18 20 22 24 26 28 30 32 34 36 38 -5 -10 25 Example A temperature transducer is used to measure the temperature of a liquid in a pipe. The indicated temperature varies approximately sinusoidally between 60.0°C and 100.0°C, with a time period of 16s. The transducer has a time constant of 2.6s. Determine: ◼ The temperature variation of the application ◼ The time delay between corresponding points on the cycle of the actual and indicated temperatures 110 16 s Time period 100 20° C 90 Amplitude (AOUT) 80 Recorded temperature 70 60 50 0 2 4 6 8 10 12 14 16 18 20 22 24 26 28 30 32 34 36 38 26 1 1 ◼ Frequency of a cycle is given by: f= = = 0.06250𝑠 T 16𝑠 ◼ Angular frequency: ω = 2πf = 2π ∙ 0.06250 = 0.3927rad/s Tmax − Tmin 100.0 − 60.0 ◼ Output Amplitude: AOUT = = = 20.0°C 2 2 ◼ Input amplitude: AIN AIN = AOUT ∙ ω2 τ2 + 1 AOUT = ω2 τ2 + 1 = 20.0 ∙ 0.28562 3.82 + 1 = 28.6°C Tmax = Tshift + AIN = 80.0 + 28.6 = 108.6°C ◼ Temperature of the application: Tmin = Tshift − AIN = 80.0 − 28.6 = 51.4°C 180 ◼ Phase lag: Degree = Radians π ϕ = arctan ωτ = arctan 0.3927 ∙ 2.6 = 0.796rad = 45.6° ϕ 0.796 ◼ Delay: Delay = ∙ T= ∙ 16 = 2.0s 2π 2π 27 Example – summary of results 115 16 s 110 Time period 105 100 28.6° C 95 Amplitude (AIN) 90 2s 85 Delay 80 75 70 65 Recorded Actual process 60 temperature temperature 55 50 0 1 2 3 4 5 6 7 8 9 10 11 12 13 14 15 16 17 18 19 20 21 22 23 24 25 26 27 28 29 30 31 32 33 34 35 36 37 38 7.0% 25.00 6.0% Absolute error, °C Relative error, % 20.00 5.0% 4.0% 15.00 3.0% 10.00 2.0% 5.00 1.0% 0.0% 0.00 0 2 4 6 8 10 12 14 16 18 20 22 24 26 28 30 32 34 36 38 What if your readings are more complex 40 °C 30 20 10 0 0 4 8 12 16 20 24 28 32 36 40 44 48 52 56 60 64 time -10 -20 -30 -40 29 The Fourier Transform Any signal can be represented as a sum A mathematical tool that decomposes of sinusoidal components (sines and a time-domain signal into its cosines) with different frequencies, constituent frequencies amplitudes, and phases Where: f(t): Time-domain signal f[n]: Discrete-time signal F(ω): Frequency-domain representation ω: Angular frequency j is the imaginary unit (j²=−1) 30 Discrete-time Fourier transform A,°C Frequency domain 25 0.0625 Hz 20 15 10 0.25 Hz 5 0 0.00 0.05 0.10 0.15 0.20 0.25 0.30 0.35 0.40 0.45 0.50 0.55 0.60 0.65 0.70 0.75 0.80 0.85 0.90 0.95 1.00 f, Hz Generate a sine wave using 64 sample points with identified frequencies and amplitude y n = sin 2π ∙ f ∙ n 31 30 20 10 y1 n = sin 2π ∙ 0.0625 ∙ n 0 0 4 8 12 16 20 24 28 32 36 40 44 48 52 56 60 64 -10 -20 -30 + 20 10 y2 n = sin 2π ∙ 0.25 ∙ n 0 0 4 8 12 16 20 24 28 32 36 40 44 48 52 56 60 64 -10 -20 = 40 30 20 10 0 f(t) -10 0 4 8 12 16 20 24 28 32 36 40 44 48 52 56 60 64 -20 -30 -40 32 Random errors Random errors in measurements are caused by unknown and unpredictable changes occurring in the measuring instrument or in environmental conditions. Random errors show up as different results for the same repeated measurement. The concept of random error is closely related to the precision. The higher the precision of a measurement instrument, the smaller the variability (standard deviation) of the fluctuations in its readings Random (precision) error in xi Example: Measured value, x ◼ Electronic noise in the circuit of an electrical instrument ◼ Instabilities of the transducer Systematic ◼ Changing in environmental error conditions (for example irregular changes in heat transfer due to True value wind) Number of measurements 33 Error | uncertainty | accuracy ◼ The difference between the measured value and the true value ◼ Accuracy is a qualitative term a measurement can be accurate or not accurate (maximum difference that will exist between the true value and the measured values) ◼ Uncertainty is a quantitative term The bounds placed on a measurement defines the uncertainty associated with that measurement Uncertainty of a measurement expresses the doubt that exists about the result of the measurement In order to quantify the uncertainty of a measurement it is necessary to identify how large the margin is and how bad the doubt is. The margin can be expressed by means of the width of the margin, or interval. The doubt can be expressed by means of a confidence level, which states the certainty that the true value is within the margin 34 Error | uncertainty Lower limit Upper limit Mean Unknown measured true value value Error Range of uncertainty 35 Example ◼ The measurement of a room temperature might be stated as 20°C ± 1.5°C at the 95% confidence level ◼ This statement indicates 95% sure that the temperature in the room is between 18.5°C and 21.5°C interval is 3°C. Mean Unknown measured true value Upper limit Lower limit value 20.5°C 18.5°C 20°C Error 21.5° Range of uncertainty – 95% sure that room temperature is in this range 36 Determination of the uncertainty ◼ Evaluated by statistical methods The measurement results are random variables. Repeated measurement data available ◼ Evaluated by other means Statistical data are not available. Use other types of information, such as instrument accuracy, class, etc. 37 Statistical background – average value ◼ An average provides an estimate of the true value 𝑛 Where: 1 𝑥ҧ = mean value of x 𝑥ҧ = 𝑥𝑖 n = total number of measurements 𝑛 𝑥𝑖 = individual measurements 𝑖=1 ◼ Example: determine the average flue gas flow rate for the following measurement (m³/h) Measurement 1 2 3 4 5 6 7 8 9 10 11 number Flow rate reading 191.0 191.5 191.7 179.5 191.0 190.3 179.3 182.7 178.8 181.0 180.3 (m³/h) 1 𝑥ҧ = σ11 1 𝑥𝑖 = 1 191.0 + 191.5 + 191.7 + 179.5 + 191.0 + 190.3 + 179.3 + 182.7 + 178.8 + 181.0 + 180.3 =185.2 m³/h 11 11 In Excel use the function: =AVERAGE(cell.range) 38 Statistical background – quantify the spread of data ◼ One option is the range as difference between the highest and lowest values Example before: Max 191.7 | Min 178.8 | Range 191.7-178.8=12.9 Information about the range on its own can, however, be misleading, especially if a single reading is markedly different from the rest ◼ A useful way is to use the standard deviation (square of the variance) The standard deviation (s or σ) of a sample of values provides an indication of how the readings typically differ from the average Low standard deviation: Where: The values are close to the σni=1 xi − x 2 s = estimated standard deviation mean, meaning less s= = 5.8 m3 / h n = total number of measurements variability in the dataset n−1 𝑥ҧ = mean value of x High standard deviation: 𝑥𝑖 = individual measurements The values are spread out over a wider range, meaning more variability in In Excel use the function: =STDEV.S(cell.range) the dataset 39 Statistical background – data distribution ◼ For a set of data it is possible for the Measurement number Ti (°C) 1 699.2 spread of data to take many forms 2 701.1 ◼ This data can be plotted in the form 3 702.4 4 703.6 of a frequency distribution curve 5 704.7 indicates the number of occurrences for 6 703.2 7 702.6 which data with the same value occurs 8 698.9 ◼ Example: temperature measurement 9 705.3 in a furnace 10 11 707.5 706.8 Average 703.4°C 12 705.3 13 S=4.9°C 712 14 706.1 15 696.3 16 695.5 17 701.3 18 707.2 19 713.4 20 695.2 40 ◼ Frequency distribution curve for the data Example of data distributed with the majority of values nearer to the mean than further away. Here: T = 703.4°C s = 4.9°C 41 Normal distribution (Gaussian distribution) ◼ For data following normal distribution the frequency distribution is defined as Where: 1 1 x−μ 2 σ = standard deviation f x = exp − ∞ < x < +∞ μ = mean σ 2π 2 σ 𝑓(𝑥)= probability density function of the continuously random variable x 99.73% of the values falls within a bound of ±3 σ 95.44% of the values falls within a bound of ±2 σ 68.26% of the values falls within a bound of 1± σ The normal distribution models sets of data arising from a large number of applications. If a set of data follows the normal distribution then frequency equation can be used to determine the frequency with which a particular range of data values will occur. It is basically a probability function to determine the frequency with which a particular range of data values will occur 42 Standard deviation in metrology Standard deviation provides a way to define confidence intervals, helping to ◼ The value of the estimated standard deviation, understand how much a measurement 5.8 m³/h can be used to provide an indication of might deviate from the true value the spread of the data low standard deviation means that repeated measurements are consistent, ◼ For normal distribution: which is essential for high-precision 99.73% of the values falls within a bound of ±3 σ instruments 95.44% of the values falls within a bound of ±2 σ 68.26% of the values falls within a bound of 1± σ high standard deviation in repeated measurements suggests systematic errors, instrument drift, or external influences affecting precision This facility for bounding data makes the standard deviation a useful concept in metrology Used to determine acceptable measurement limits in industry standards 43 Standard deviation in metrology ◼ The standard deviation, is directly proportional to the width of variation ◼ It can therefore be used to characterise the uncertainty associated with a value and using a bilateral tolerance the measurement can be stated as: A measurement stated in this way is known as the standard uncertainty and accounts for 68.26% of Value = mean ± standard deviation measurements provided the data is normally distributed. That is, we could be confident that 68% of measurements would lie within the range – to +. 68% limits are not suitable for all measurements Value = mean ± kσ Common values used for the coverage factor, k, are 1, 2 and 3, corresponding to 68.26%, 95.44% and 99.73% of measurements 44 Standard deviation in metrology ◼ Example: determine the average flue gas flow rate for the following measurement (m³/h) Measurement 1 2 3 4 5 6 7 8 9 10 11 number Flow rate reading 191.0 191.5 191.7 179.5 191.0 190.3 179.3 182.7 178.8 181.0 180.3 (m³/h) 1 𝑥ҧ = σ11 1 𝑥𝑖 = 185.2 m³/h 11 Value = 185.2 ± 11.6 𝐦𝟑 / 𝐡 (95%) σni=1 xi − x 2 s= = 5.8 m3 / h n−1 45 Independent, uncorrelated random variables ◼ Let y be a function of several independent, uncorrelated random variables Temperature sensor Heat meter unit y = 𝑓 𝑥1 , 𝑥2 …. 𝑥𝑛 (supply side) ◼ Example Q = 𝑓 𝑉, 𝑇1 , 𝑇2 Source Load Q = ρ V cp T2 − T1 Flow meter Temperature sensor (return side) Other simpler example: If you measure length (L) and width (W) to calculate area (A =L∙W), uncertainties in L and W contribute to uncertainty in A. If we measure voltage (V) and current (I) to calculate power (P=V⋅I), uncertainties in V and I contribute to uncertainty in P. 46 Statistical mean and standard deviation of a function CASE: Measure physical quantities and perform calculations with those measurements. Always some degree of uncertainty associated with each single measurements and therefore with the results of the calculations PROPAGATION: estimate the uncertainty in the outcome of a function that depends on several measured variables METHOD: total differential of a function like f(x, y, z,...) where the variables x, y, z, etc. are independent. This is a mathematical expression that represents how the function changes with small changes in those variables. Mathematically, it is given by: Where: df is the change in the function f 𝜕f 𝜕f 𝜕f dx, dy, dz, etc. are the changes in the variables (basically the uncertainties in the df = dx + dy + dz… respective measurements) 𝜕x 𝜕y 𝜕z 𝜕f 𝜕f 𝜕f , , , etc. are the partial derivatives of the function f(x,y,z…) with respect to 𝜕x 𝜕y 𝜕z each variable, which represent how f changes with a change in that particular variable, holding the other constant When each variable (x, y, z,...) has an uncertainty (Δx, Δy, Δz,...), the uncertainty in the function f, denoted as Δf, can be approximated by propagating these uncertainties through the total differential Basically dx = Δx as the uncertainty in x 2 2 𝜕f 2 𝜕f 2 𝜕f 2 𝜕𝑓 1 𝜕2 𝑓 ∆f 2 ≅ ∆x 2 + ∆y 2 + ∆z 2 … 𝜎𝑓2 = σ𝑛𝑗=1 𝜎𝑥2𝑗 + σ𝑛𝑗=1 𝜎𝑥4𝑗 … 𝜕x 𝜕𝑦 𝜕z 𝜕𝑥𝑗 2 𝜕𝑥𝑗2 𝑛 2 This formula gives the combined uncertainty in f resulting 2 𝜕𝑓 Can be neglected from the uncertainties in each of its variables. 𝜎𝑓 ≈ 𝜎𝑥2𝑗 𝜕𝑥𝑗 𝑗=1 47 Example 1 ◼ For the function f(x,y,z)=x+y−z with the measured values: x=2.0±0.2 (68%) y=3.0±0.6 (68%) z=4.52±0.02 (68%) ◼ The uncertainty of f can be calculated using the formula for error propagation: 𝜕f 2 𝜕f 2 𝜕f 2 ∆f 2 ≅ ∆x 2 + ∆y 2 + ∆z 2 =[12x0.22+12x0.62+(-1)2x0.022] 𝜕x 𝜕𝑦 𝜕z ◼ Given the partial derivatives: 𝜕f = 1 𝜕x 𝜕f = 1 𝜕𝑦 𝜕f = -1 𝜕z ◼ Substituting the values and uncertainties into the formula yields a propagated uncertainty in f of approximately Δf = 0.633 ◼ Thus, the result for ∆f with the given values and uncertainties is f(x,y,z) = 0.5±0.6 (68%) where 0.5 comes from calculating f(x,y,z)=2.0+3.0−4.52=0.48 48 Example 2 ◼ For the function f(x,y)=x∙y with the measured values: x=4.52±0.02 (68%) y=2.0±0.2 (68%) ◼ The uncertainty of f can be calculated using the formula for error propagation: 2 2 2 𝜕f 2 𝜕f ∆f ≅ ∆x + ∆y 2 =[4.522x0.022+2.02x0.22] 𝜕x 𝜕𝑦 ◼ Given the partial derivatives: 𝜕f =y=4.52 because f(x,y) varies linearly with x when y is constant. 𝜕x 𝜕f =x=2.0 because f(x,y) varies linearly with y when x is constant 𝜕𝑦 ◼ Substituting the values and uncertainties into the formula yields a propagated uncertainty in f of approximately Δf = 0.905 ◼ Thus, the result for ∆f with the given values and uncertainties is f(x,y) = 9.0±0.9 (68%) where 9.0 comes from calculating f(x,y)=4.52 ∙ 2.0=9.04 49 Example 3 x ◼ For the function f x, y = with the measured values: y x=2.0±0.2 (68%) y=3.0±0.6 (68%) ◼ The uncertainty of f can be calculated using the formula for error propagation: 2 2 2 𝜕f 2 𝜕f ∆f ≅ ∆x + ∆y 2 𝜕x 𝜕𝑦 ◼ Given the partial derivatives: 𝜕f 1 = y = 0.33 because f(x,y) varies inversely with y when x is changing 𝜕x 𝜕f 𝑥 = − 𝑦 2 = −0.22 because f(x,y) decreases as y increases, with a rate of change that depends inversely on the square of y 𝜕𝑦 ◼ Substituting the values and uncertainties into the formula yields a propagated uncertainty in f of approximately Δf = 0.149 ◼ Thus, the result for ∆f with the given values and uncertainties is f(x,y) = 0.7±0.2 (68%) where 0.7 comes from calculating f(x,y)=2.0 / 3.0=0.67 50 Example 4 x∙𝑦 2 ◼ For the function f x, y = with the measured values: A x=4.52±0.02 (68%) y=3.0±0.6 (68%) A= 2.0±0.2 (68%) ◼ The uncertainty of f can be calculated using the formula for error propagation: 2 2 2 𝜕f 𝜕f 𝜕f ∆f 2 ≅ ∆x 2 + ∆y 2 + ∆A2 𝜕x 𝜕𝑦 𝜕A ◼ Given the partial derivatives: 𝜕f 𝑦2 = = 6.36 captures how f changes with x while keeping y and A constant 𝜕x 𝐴 𝜕f 2𝑥𝑦 = = 19.18 captures how f changes with y, accounting for the squared relationship between f and y 𝜕𝑦 𝐴 𝜕f 1 𝑥𝑦2 =− = −7.19 captures how f changes with A, noting the inverse square root relationship 𝜕𝐴 2 32 𝐴 ◼ Substituting the values and uncertainties into the formula yields a propagated uncertainty in f of approximately Δf = 11.596 ◼ Thus, the result for ∆f with the given values and uncertainties is f(x,y) = 28.8±11.6 (68%) x∙𝑦2 where 28.8 comes from calculating f x, y = = 28.77 A 51 Example 5 ◼ For the function f x, y, z = xy + z 2 with the measured values: x=4.52±0.02 (68%) y=2.0±0.2 (68%) z= 3.0±0.6 (68%) ◼ The uncertainty of f can be calculated using the formula for error propagation: 2 2 2 𝜕f 𝜕f 𝜕f ∆f 2 ≅ ∆x 2 + ∆y 2 + ∆𝑧 2 𝜕x 𝜕𝑦 𝜕z ◼ Given the partial derivatives: 𝜕f = 𝑦 = 2.0 captures how f changes with x while keeping y and A constant 𝜕x 𝜕f = 𝑥 = 4.52 captures how f changes with y, accounting for the squared relationship between f and y 𝜕𝑦 𝜕f = 2𝑧 = 6.0 captures how f changes with A, noting the inverse square root relationship 𝜕𝐴 ◼ Substituting the values and uncertainties into the formula yields a propagated uncertainty in f of approximately Δf = 3.712 ◼ Thus, the result for ∆f with the given values and uncertainties is f(x,y,z) = 18.0±3.7 (68%) where 18.0 comes from calculating f x, y = xy + z 2 = 18.04 52 Practical example 1 ◼ A temperature difference is determined using two different PT100 sensors For the function f x, y = x − y with the measured values: ◼ The average measurements of the two sensors are T1=52.0°C and T2=92.0°C x=52.0±2.0 (95%) ◼ The 95% confidence limit uncertainties for each device are ±2.0°C and ±1.2°C y=92.0±1.2 (95%) 𝑓 𝑇1 , 𝑇2 = T2 − T1 ◼ Given the partial derivatives: 𝜕f = -1 𝜕𝑇1 𝜕f = 1 𝜕𝑇2 ◼ Substituting the values and uncertainties into the formula yields a propagated uncertainty in f of Δf=2.332 ◼ Thus, the result with the given values and uncertainties is f(T2-T1)= 40.0±2.3°C (95%) n 2 2 𝜕f where 40.0 comes from calculating f(T2-T1)=92.0-52.0=40.0 °C σy ≈ σ2xj 𝜕xj j=1 ◼ NOTE: 95% limits (k = 2), then the standard deviation 2.0 σT1 = = 1.0°C 2 1.2 𝜎Δ𝑇 = 𝜎12 + 𝜎22 = 1.17°𝐶 ΔT = T2 − T1 =92-52=40.0 ±2.3°C (95%) σT2 = = 0.6°C 2 53 Practical example 2 For the function f x, y, z = a ∙ b ∙ x ∙ (y − z) ◼ Heat power is determined using the following relationship: with the measured values: x=0.00312±0.00007 (95%) Q = ρ V cp ΔT Q = ρ V cp T2 − T1 y=92.0±1.0 (95%) z=52.0±1.0 (95%) ◼ The volumetric flow rate is determined using an ultrasound flow meter ◼ A temperature difference is determined using two PT100 sensors ◼ Average measurements of the flow meter V=0.00312 m³/s 95% confidence limit uncertainty is ±0.00007 m³/s ◼ Average measurements of PT100 sensors T1=52.0°C T2=92.0°C 95% confidence limit uncertainties ±1.0°C For simplicity the density ρ (998kg/m³) and the specific heat capacity cp (4.186kJ/kg K) are considered constants and determined without uncertainty 54 ◼ Given the partial derivatives: 𝜕f = ρcp T2 − T1 = 167105.1 𝜕V 𝜕f = ρcp V = 13.0 𝜕T1 𝜕f = −ρcp V = −13.0 𝜕T2 ◼ Substituting the values and uncertainties into the formula yields a propagated uncertainty in f of Δf = 21.831 ◼ Thus, the result for ∆f with the given values and uncertainties is Q=521.4 ± 21.8 kW (95%) where 521.4 comes from calculating Q = 1000 ∙4.186 ∙ (92-52) =521.4 kW Q = ρ V cp T2 − T1 55