NLP & Text Analytics Overview PDF
Document Details
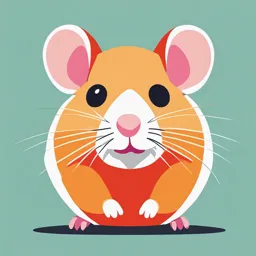
Uploaded by FinerCopernicium5470
University of Rijeka
Sanda Martinčić-Ipšić
Tags
Summary
This presentation provides a comprehensive overview of natural language processing (NLP) and text analytics. It discusses various aspects, including applications in different fields such as marketing, finance, drug discovery, and law enforcement.
Full Transcript
Natural Language Processing (NLP) Sanda Martinčić-Ipšić Full professor [email protected] 2 NLP: NLU + NLG + … NLP NLU Linguistics AI DL ML...
Natural Language Processing (NLP) Sanda Martinčić-Ipšić Full professor [email protected] 2 NLP: NLU + NLG + … NLP NLU Linguistics AI DL ML NLG 3 Web Text Text Analytics Social media News Public Text Text Analytics Reports Statistical Applications reports Marketing Financial investment Private Text Drug discovery Law enforcement Internal … Data e-mail Subscription Data 4 Hidden Values in Text 5 Dream 6 Reality 7 Why NLP is difficult? Different ways of parsing a sentence Word category ambiguity Word sense ambiguity Words can mean more than their sum of parts (The Times of India) Imparting world knowledge is difficult ("the blue pen ate the ice-cream") Fictitious worlds ("people on mars can fly") Language is changing and evolving Complex ways of interaction between the kinds of knowledge exponential complexity at each point in using the knowledge Why NLP is difficult? Meaning Ambiguity Polysemy Sarcasam Irony … … Applications Topic /genre detection Question answering Spam detection Chatbots & Virtual Authorship attribution (sex, age) Assistants Sentiment analysis Text Classification Language identification (multilinguality) Text Extraction Summarization Machine Translation Information retrieval Speech Recognition 10 Is this spam 11 Who wrote which Federalist papers? 1787-8: anonymous essays try to convince New York to ratify U.S Constitution: Jay, Madison, Hamilton. Authorship of 12 of the letters in dispute 1963: solved by Mosteller and Wallace using Bayesian methods James Madison Alexander Hamilton 12 Male of Female author? 1. By 1925 present-day Vietnam was divided into three parts under French colonial rule. The southern region embracing Saigon and the Mekong delta was the colony of Cochin-China; the central area with its imperial capital at Hue was the protectorate of Annam… 2. Clara never failed to be astonished by the extraordinary felicity of her own name. She found it hard to trust herself to the mercy of fate, which had managed over the years to convert her greatest shame into one of her greatest assets… - Females use more pronouns, male more facts.... S. Argamon, M. Koppel, J. Fine, A. R. Shimoni, 2003. “Gender, Genre, and Writing Style in Formal Written Texts,” Text, volume 23, number 3, pp. 321–346 13 Schwartz HA, Eichstaedt JC, Kern ML, Dziurzynski L, Ramones SM, et al. (2013) Personality, Gender, and Age in the Language of Social Media: The Open- Vocabulary Approach. PLoS ONE 8(9): e73791. doi:10.1371/journal.pone.0073791 14 Demographics - age group? 13-18 15 ? 19-23 16 Category of the article? Antogonists MEDLINE Article MeSH Subject Category Hierarchy and Inhibitors Blood Supply Chemistry Drug Therapy Embryology ? Epidemiology … https://www.nlm.nih.gov/mesh/ Which language? detecting language is classification problem many langauges in the world – https://www.ethnologue.com/ 7099 living languages – All small languages Istrorumunjski jezik - istočnoromanski sjever Istre (Žejane, Lanišće, Šušnjevica) danas govori nekoliko stotina Ćiribiraca, dijalekt rumunjskog? 18 Discrepancy between real and virtual 20 Wikipedia: 314 languages (2020) totall entries in top 20 languages https://meta.wikimedia.org/wiki/List_of_Wikipedias Wikipedia by Number of Articles, October 2020 6.000.000 5.000.000 HR 44 4.000.000 SL 52 3.000.000 2.000.000 1.000.000 0 en ceb sv de fr nl ru it es pl war vi ja zh pt uk fa sr ca ar no sh fi hu id Cebuano (Austro-nezijski jezik) 21 Text Analytics Overwiev 27 Text Analytics Intersection 29 From text to knowledge 30 Question answering systems systems that automatically answer questions posed by humans in a natural language wide question types: fact, list, definition, How, Why, hypothetical, semantically constrained, and cross-lingual questions. Closed-domain deals with a specific domain (medicine or geography) use of domain-specific knowledge frequently formalized in ontologies or a limited type of questions are accepted Open-domain deals with questions about anything rely on general ontologies and world knowledge these systems usually have a lot of different data available to extract the answer 33 QA vs IR document search (information retrieval) takes a keyword query and returns a list of documents, ranked in order of relevance to the query keyword QA takes a question expressed in natural language, seeks to understand it in much greater detail, and returns a precise answer to the question. 34 Types of Questions Factoid questions Who wrote “The Universal Declaration of Human Rights”? How many calories are there in two slices of apple pie? What is the average age of the onset of autism? Where is Apple Computer based? Complex (narrative) questions: In children with an acute febrile illness, what is the efficacy of acetaminophen in reducing fever? What do scholars think about Trump’s position on dealing with immigrants? 35 Watson demo https://www.youtube.com/watch?v=_Xcmh1LQB9I 36 Watson’s architecture 37 Intelligent personal assistant is a software agent that can perform tasks or services for an individual. based on user input, location awareness, and the ability to access information from a variety of online sources (weather or traffic conditions, news, stock prices, user schedules, retail prices, etc.). The combination of : – Automatic Speech Recognition. – Artificial Intelligence. – Natural Language Processing. – Question answering – Inter Process Communication. 38 Features Speaks Naturally. Communicate with surroundings and other objects. Georeferencing and Event based services. Grows with you. Get smarter every day. Will entertain you. 39 Features Some of the services for day by day use: Make Phone Calls Schedule meetings & Appointments Get Direction Send Messages Set Reminders Ask Questions Play Music & Videos Wake me up at 6.30AM 40 Google asistant vs Amazon Alexa demo https://www.youtube.com/watch?v=jrpaQN8TN6o ALEXA: voice interaction, music playback, making to-do lists, setting alarms, streaming podcasts, playing audiobooks, and providing weather, traffic, and other real time information... Google Asistant: provides answers, entertainment (music,...), task planing, managing the house, personal planning, finance and flight information, shopping,... 41 Chatbot on-line chat conversation text or text-to-speech / speech-to-text dialog systems for various purposes: customer service, request routing, information gathering, telecom, product or service sell some use extensive natural language processoring and sophisticated AI /DL or scan for general keywords and generate responses using predefined responses 42 Types Pedefined Button bot Conversational Keyword spotting Intent-based Autonoumos https://www.getjenny.com/what-is-a-chatbot 43 Chatbot examples https://www.wordstream.com/blog/ws/2017/10/04/chatbots A Companion for Dementia Patients Helping Insomniacs Get Through the Night Making Medical Diagnoses Faster Help/ self diagnose in Covid Pandemics https://andrija.ai/ (in Croatian) 44 https://www.wbb.ai/ 60% less emails and phone calls. Reduce inbound contact centre traffic by up to 60% intelligent bots - can execute complex processes and respond to simple customer queries Free thousands of hours - so you can focus on less repetitive, more rewarding work. 26% more time to focus on the real work. A OnePoll survey found that 26% of staff time is eaten up by tasks that a non core to their role. I bots can execute these processes in a fraction of the time and your teams can get back to the real work. Choose from 100s of pre-built bots wide range of pre build bots that you can drag and drop into place, quickly edit and away you go! you can be live in minutes, not weeks. 45 BUT Microsoft Tay example Tay AI bot , 2016 the bot began to post inflammatory and offensive tweets through its Twitter account, causing Microsoft to shut down the service only 16 hours after its launch. https://en.m.wikipedia.org/wiki/Tay_(bot) 46 Name-entity recognition: NER entity identification, entity chunking, and entity extraction is a subtask of information extraction that seeks to locate and classify named entities mentioned in unstructured text into pre-defined categories such as person names, organizations, locations, medical codes, time expressions, quantities, monetary values, percentages, etc. 47 NER Example: Location extraction (example Hamburg: Balke et al. 2018) NER – Bilbao Evans 2003. Example TOP locations: London 12 New York 8 Barcelona 8 BUT paper is related to Guggenheim Museum in Bilbao SOLUTION: ??? annotation 49 Deep Neural Models Use a deep neural network Deep = many layers Various architectures Many parameters 50 Number of parametars January 2020 2019 51 Number of parametars July 2020 GPT3 January 2020 2019 52 Foundations Models in 2022 53 Deep learning vs machine learning 54 Example predicting movie genres (Lev sci-fi Konstantinovskiy) romance 55 Example movie genres - corpus 56 Example movie genres – doc2vec vectors P(vIN|vOUT, Genre) = P(over| fox, comedy) The fox jumped over the lazy dog. comedy vOUT vIN vOUT vIN 57 Vectors in 2D space 58 Relations between words are distances between vectors 59 Semantic space simmilar words are close (they have simmilar contexts) we can calculate semantic simmilarity 60 Multilingual, Machine translation 61 Sentiment 62 OpenAI GPT-2 + GPT-3: language foundation model GPT-2 text generation demo https://www.newyorker.com/magazine/2019/10/14/can-a- machine-learn-to-write-for-the-new-yorker https://talktotransformer.com/ New GPT-3 (2020) https://github.com/openai/gpt-3 63 Wu-Dao 2.0: pre-trained multimodal foundation model https://en.m.wikipedia.org/wiki/Wu_Dao https://gpt3demo.com/apps/wu-dao-20 Beijing Academy of Artificial Intelligence GPT-3 - 175 billion parameters Wu Dao -1.75 trillion parameters multimodal learning from text and images trained on 4.9 terabytes of images and texts 1.2 TB Chinese + 1.2 TB English texts 2.5TB Chinese graphic data 64 DALL-E: multimodal foundation model https://en.m.wikipedia.org/wiki/DALL-E https://openai.com/blog/dall-e/ OpenAI creates images from textual descriptions 12-billion parameter version of the GPT-3 Transformer model trained on a dataset of text–image pairs Demo: https://huggingface.co/spaces/flax- community/dalle-mini 65 EXAMPLES https://daleonai.com/dalle-5-mins takes a text caption “an armchair in the shape of an avocado” generates images to “a snail made of a harp.” 66 GitHub Copilot https://copilot.github.com/ https://openai.com/blog/openai-codex/ AI tool -powered by OpenAI Codex — that functions as a pair programmer, helping human developers write code Codex - a descendant from GPT-3 trained on huge amounts of coding data publicly available, from GitHub repositories and other sites complete lines of code, write whole functions, transform descriptive comments into code, autofill repetitive code, or create unit tests for your methods works best with Python, JavaScript, TypeScript, Ruby, and Go, but “understands dozens of languages.” 67 Copilot – big picture https://copilot.github.com/ 68 Copilot Demo: https://youtu.be/vLWQ9_uKNSs https://copilot.github.com/ Skip the docs and stop searching for examples. GitHub Copilot helps you stay focused right in your editor. Convert comments to code. Write a comment describing the logic you want, and let GitHub Copilot assemble the code for you. Autofill for repetitive code. GitHub Copilot works great for quickly producing boilerplate and repetitive code patterns. Feed it a few examples and let it generate the rest! Tests without the toil. Tests are the backbone of any robust software engineering project. Import a unit test package, and let GitHub Copilot suggest tests that match your implementation code. Show me alternatives. Want to evaluate a few different approaches? GitHub Copilot can show you a list of solutions. 69 BUT: ethics, security, privacy, bias,… legal https://www.facebook.com/about/privacy “There’s a lot of public code in the world with insecure coding patterns, bugs, or references to outdated APIs or idioms. When GitHub Copilot synthesizes code suggestions based on this data, it can also synthesize code that contains these undesirable patterns.” „ questions about intellectual property, licenses, and copyright infringement” GPT3 Creating fake news in many languages, gender bias, racial bias…, trained on private data…. Who is the author of the contect AI system, AI system programmers/company or user? 70 Conclusion Text analytics & NLP can be usefull in many applications Your start-up projects maybe can benefit with NLP Good luck! 71