Statistical Analysis of Data - Fall 2020
Document Details
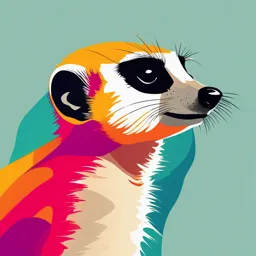
Uploaded by PerfectClearQuartz
2020
Tags
Summary
This presentation covers various statistical concepts, including individual differences, descriptive statistics, cross-tabulation, measures of central tendency, and distributions. It's suitable for students in an undergraduate research methods course.
Full Transcript
Statistical Analysis of Data Graziano and Raulin Research Methods: Chapter 5 Individual Differences ON THE COUNT OF 3… everyone share their height (in inches). A fact of life People differ from one another People differ from one occasion to another Statistics give us a way to detect...
Statistical Analysis of Data Graziano and Raulin Research Methods: Chapter 5 Individual Differences ON THE COUNT OF 3… everyone share their height (in inches). A fact of life People differ from one another People differ from one occasion to another Statistics give us a way to detect subtle effects in a sea of individual differences Descriptive Statistics Are used to describe the data Many types of descriptive statistics Frequency distributions Summary measures such as measures of central tendency, variability, and relationship Graphical representations of the data A way to visualize the data The first step in any statistical analysis Cross-Tabulation A way to see the relationship between two nominal or ordinal variables Can be done with interval and ratio scales, but often the size of the table is very large Create a set of cells by listing the possible values of one variable at the top of columns and the values of the other along the rows Cross-Tabulation Example Males Females Total Democrats 4 5 9 Republican s Other 6 1 7 7 1 8 17 7 24 Total Shapes of Distributions Many variables in psychology are distributed normally The distribution is skewed if scores bunch up at one end Illustrate on the left are symmetric and skewed distributions Measures of Central Tendency Mode: the most frequently occurring score Median: the middle score in a distribution Easy to compute from frequency distribution Less affected than the mean by a few deviant scores Mean: the arithmetic average Most commonly used central tendency measure Used in later inferential statistics Finding the Mode The easiest way to find the mode is to construct a frequency distribution first Look for the score with the largest frequency If there are two or more scores that are tied for the largest frequency, report each of them Computing the Median Arrange the scores from smallest to largest Determine the middle score {(N+1)/2} If 7 scores, the middle is the fourth score (7+1)/2=4 4,5,6,7,8,9,10– the median is 7 If 8 scores, the middle score is half way between the two middle scores 4,5,6,7,8,9,10, 11- the median is 7.5 Computing the Mean Compute the mean of 3, 4, 2, 5, 7, & 5 Sum the numbers Count the numbers 26 6 Plug these values into the equation at the right X X N 26 X 4.33 6 Measuring Variability Range: lowest to highest score Variance: average squared distance from the mean Used in later inferential statistics Standard Deviation: square root of variance expressed on the same scale as the mean The Range Computing the Range Find the lowest score Find the highest score Subtract the lowest from the highest score Easy to compute, but unstable because it relies on only two scores The Variance Computing the Variance Compute the mean Compute the distance of each score from the mean and square that distance Sum those squared distances and divide by the degrees of freedom (N-1) Good statistical properties, but this measure of variability is in squared units The Standard Deviation Computing the Standard Deviation Compute the variance Take the square root of the variance This measure, like the variance, has good statistical properties and is measured in the same units as the mean EVERY TIME YOU REPORT A MEAN, YOU MUST ALSO REPORT A SD! FAKE Exam 1 Data 103 101 99 93 90 90 90 90 90 88 87 85 84 82 80 80 78 78 76 70 60 55 FAKE Class results Mean: 84.05 Median: 86 Mode: 90 Range: 48 What happens if… … we add a new low score of 25. How does it change our mean, median, and mode? Which one is most affected? Mean was: 84.05 becomes ? Median: 86 becomes? Mode: 90 becomes? Range: 48 becomes? Mean 81.48 (somewhat different) Median 85 (very close) Mode 90 (stayed the same!) Range 78 (BIG increase) Measures of Relationship Pearson product-moment correlation Spearman rank-order correlation Used with interval or ratio data Used when one variable is ordinal and the second is at least ordinal Scatter plots Visual representation of a correlation Helps to identify nonlinear relationships Correlations Range from –1.00 to +1.00 -1.00 means a perfect negative relationship (as one score decreases, the other increases a predictable amount) +1.00 means a perfect positive relationship 0.00 means that there is no relationship Regression Using a correlation (relationship between variables) to predict one variable from knowing the score on the other variable Usually a linear regression (finding the best fitting straight line for the data) Best illustrated in a scatter plot with the regression line also plotted Reliability Indices Test-retest reliability and interrater reliability are indexed with a Pearson product-moment correlation Internal consistency reliability is indexed with coefficient alpha (Cronbach’s alpha) Inferential Statistics Used to draw inferences about populations on the basis of samples from the populations The “statistical tests” that we perform on our data are inferential statistics Provide an objective way of quantifying the strength of the evidence for our hypothesis Populations and Samples Population: the larger groups of all participants of interest to the researcher Sample: a subset of the population Samples almost never represent populations perfectly (termed “sampling error”) Not really an error; just the natural variability that you can expect from one sample to another The Null Hypothesis States that there is NO difference between the population means Compare sample means to test the null hypothesis Population parameters & sample statistics Population parameter is a descriptive statistic computed from everyone in the population Sample statistics is a descriptive statistic computed from everyone in your sample Statistical Decisions We can either Reject or Fail to Reject the null hypothesis Rejecting the null hypothesis suggests that there is a difference in the populations sampled Failing to reject suggests that no difference exists Decision is based on probability (reject if it is unlikely that the null hypothesis is true) Alpha: the statistical decision criteria used in Traditionally alpha is set to small values (.05 or .01) Always a chance for error in our decision What’s worse??? Convicting an innocent person? Failing to convict a guilty person? THERE IS A RIGHT ANSWER! Statistical Decision Process Null Hypothesis is True Null Hypothesis is False Reject Null Hypothesis Retain Null Hypothesis Type I Error Correct Decision Correct Decision Type II Error Testing for Mean Differences t-test for independent groups: tests mean difference of two independent (different) groups (in only one condition) t-test for paired samples: tests mean difference of two groups that are in both conditions Analysis of Variance: tests mean differences in two or more groups Groups may or may not be independent T test for indep samples, t test for paired samples, or an ANOVA? You want to compare which type of cracker people prefer Cheese Nips vs. Cheez Its. You randomly assign people to try 1 type. You do the same study but you add in a 3rd type of cracker, Cheesy Ritz. You have people try both types of crackers and make ratings. You compare men vs. women on exam scores.