Lecture 3: Hypothesis Testing (Part I) PDF
Document Details
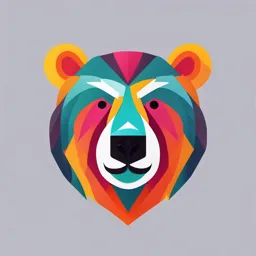
Uploaded by CleverNovaculite
Huddersfield Business School
2023
Dr Artur Semeyutin
Tags
Summary
This is a lecture on hypothesis testing (Part I) from Huddersfield Business School. The lecture covers statistical inference, and regression parameters. Essential reading material is provided and includes Chris Brooks' textbook.
Full Transcript
Lecture 3: Hypothesis Testing (Part I) Essential reading: Chapters 3 and 4 in Brooks. Dr Artur SemeyutinBIE0014: Econometrics Huddersfield Business School w/c 13/02/2023Dr Artur Semeyutin (BIE0014) HYT Business School1 / 46 An Introduction to Statistical Inference We want to make inferences about t...
Lecture 3: Hypothesis Testing (Part I) Essential reading: Chapters 3 and 4 in Brooks. Dr Artur SemeyutinBIE0014: Econometrics Huddersfield Business School w/c 13/02/2023Dr Artur Semeyutin (BIE0014) HYT Business School1 / 46 An Introduction to Statistical Inference We want to make inferences about the likely population values from the regression parameters. Example: Suppose we have the following regression results: ˆ y t = 20 .3 + 0 .5091 x t (14 .38) (0 .2561) ˆ β = 0 .5091 is a single (point) estimate of the unknown population parameter, β. How fleliable” is this estimate? The reliability of the point estimate is measured by the coefficient’s standard error. Dr Artur Semeyutin (BIE0014) HYT Business School2 / 46 Hypothesis Testing : Some Concepts We can use the information in the sample to make inferences about the population. We will always have two hypotheses that go together, the null hypothesis (denoted H 0) and the alternative hypothesis (denoted H 1). The null hypothesis is the statement or the statistical hypothesis that is actually being tested. The alternative hypothesis represents the remaining outcomes of interest. For example, suppose given the regression results above, we are interested in the hypothesis that the true value of βis in fact 0.5. We would use the notation H0: β = 0 .5 H 1: β ̸ = 0 .5 This would be known as a two sided test. Dr Artur Semeyutin (BIE0014) HYT Business School3 / 46 One-Sided Hypothesis Tests Sometimes we may have some prior information that, for example, we would expect β >0.5 rather than β <0.5. In this case, we would do a one-sided test: H0: β = 0.5 H 1: β < 0.5 or we could have had H0: β = 0.5 H 1: β < 0.5 There are two ways to conduct a hypothesis test: via the test of significance approach or via the confidence interval approach. Dr Artur Semeyutin (BIE0014) HYT Business School4 / 46 The Probability Distribution of the Least Squares Estimators We assume that u t ∼ N(0 , σ 2 ) Since the least squares estimators are linear combinations of the random variables i.e. ˆ β = P wty t The weighted sum of normal random variables is also normally distributed, so ˆ α ∼ N(α, Var (α )) ˆ β ∼ N(β , Var (β )) What if the errors are not normally distributed? Will the parameter estimates still be normally distributed? Dr Artur Semeyutin (BIE0014) HYT Business School5 / 46 The Probability Distribution of the Least Squares Estimators (Cont’d)Yes, if the other assumptions of the CLRM hold, and the sample size is sufficiently large. Standard normal variates can be constructed from ˆ αand ˆ β : ˆ α − α √ var (α ) ∼ N(0 ,1) and ˆ β − β √ var (β ) ∼ N(0 ,1) But var( α) and var( β) are unknown, so ˆ α − α √ SE ( ˆα ) ∼ t T −2 and ˆ β − β √ SE (ˆ β ) ∼ t T −2 Dr Artur Semeyutin (BIE0014) HYT Business School6 / 46 Testing Hypotheses: The Test of Significance Approach Assume the regression equation is given by, yt = α+ βx t + u t for t= 1 ,2 , ..., T The steps involved in doing a test of significance are: 1 Estimate ˆ α, ˆ β and SE( ˆα ), SE (ˆ β ) in the usual way 2 Calculate the test statistic. This is given by the formula test statistic=ˆ β − β∗ SE (ˆ β ) where β∗ is the value of βunder the null hypothesis. 3 We need some tabulated distribution with which to compare the estimated test statistics. Test statistics derived in this way can be shown to follow a t-distribution with T-2 degrees of freedom. As the number of degrees of freedom increases, we need to be less cautious in our approach since we can be more sure that our results are robust. Dr Artur Semeyutin (BIE0014) HYT Business School7 / 46 Testing Hypotheses: The Test of Significance Approach (Cont’d)4 We need to choose a “significance level”, often denoted α. This is also sometimes called the size of the test and it determines the region where we will reject or not reject the null hypothesis that we are testing. It is conventional to use a significance level of 5%. Intuitive explanation is that we would only expect a result as extreme as this or more extreme 5% of the time as a consequence of chance alone. Conventional to use a 5% size of test, but 10% and 1% are also commonly used. Dr Artur Semeyutin (BIE0014) HYT Business School8 / 46 Determining the Rejection Region for a Test of Significance 5 Given a significance level, we can determine a rejection region and non-rejection region. For a 2-sided test: Dr Artur Semeyutin (BIE0014) HYT Business School9 / 46 x 95% non-rejection region 2.5% re jection region 2.5% re jection region x f ( ) The Rejection Region for a 1-Sided Test (Upper Tail) Dr Artur Semeyutin (BIE0014) HYT Business School10 / 46x 95% non-rejection region 5% rejection region x f ( ) The Rejection Region for a 1-Sided Test (Lower Tail) Dr Artur Semeyutin (BIE0014) HYT Business School11 / 46x 95% non-rejection region 5% re jection region x f ( ) The Test of Significance Approach: Drawing Conclusions 6 Use the t-tables to obtain a critical value or values with which to compare the test statistic. 7 Finally perform the test. If the test statistic lies in the rejection region then reject the null hypothesis ( H 0), else do not reject H 0. Dr Artur Semeyutin (BIE0014) HYT Business School12 / 46 A Note on the tand the Normal Distribution You should all be familiar with the normal distribution and its characteristic “bell” shape. We can scale a normal variate to have zero mean and unit variance by subtracting its mean and dividing by its standard deviation. There is, however, a specific relationship between the t- and the standard normal distribution. Both are symmetrical and centred on zero. The t-distribution has another parameter, its degrees of freedom. We will always know this (for the time being from the number of observations −2). Dr Artur Semeyutin (BIE0014) HYT Business School13 / 46 What Does the t-Distribution Look Like? Dr Artur Semeyutin (BIE0014) HYT Business School14 / 46normal distribution t-distribution x x f ( ) Comparing the t and the Normal Distribution In the limit, a t-distribution with an infinite number of degrees of freedom is a standard normal, i.e. t(∞ ) = N(0 ,1) Examples from statistical tables: Significance level N(0 ,1) t(40) t(4) 50% 0 0 0 5% 1.64 1.68 2.13 2.5% 1.96 2.02 2.78 0.5% 2.57 2.70 4.60 The reason for using the t-distribution rather than the standard normal is that we had to estimate σ2 , the variance of the disturbances. Dr Artur Semeyutin (BIE0014) HYT Business School15 / 46 The Confidence Interval Approach to Hypothesis Testing An example of its usage: We estimate a parameter, say to be 0.93, and a “95% confidence interval” to be (0.77, 1.09). This means that we are 95% confident that the interval containing the true (but unknown) value of β. Confidence intervals are almost invariably two-sided, although in theory a one-sided interval can be constructed. Dr Artur Semeyutin (BIE0014) HYT Business School16 / 46 How to Carry out a Hypothesis Test Using Confidence Intervals 1 Calculate ˆ α, ˆ β and SE( ˆα ), SE (ˆ β ) as before. 2 Choose a significance level, α, (again the convention is 5%). This is equivalent to choosing a (1- α)× 100% confidence interval, i.e. 5% significance level = 95% confidence interval 3 Use the t-tables to find the appropriate critical value, which will again have T-2 degrees of freedom. 4 The confidence interval is given by ( ˆ β − t crit × SE (ˆ β ), ˆ β + t crit × SE (ˆ β )) 5 Perform the test: If the hypothesised value of β(β ∗ ) lies outside the confidence interval, then reject the null hypothesis that β= β∗ , otherwise do not reject the null. Dr Artur Semeyutin (BIE0014) HYT Business School17 / 46 Confidence Intervals Versus Tests of Significance Note that the Test of Significance and Confidence Interval approaches always give the same answer. Under the test of significance approach, we would not reject H 0 that β = β∗ if the test statistic lies within the non-rejection region, i.e. if −t crit ≤ˆ β − β∗ SE (ˆ β ) ≤ +t crit Rearranging, we would not reject if −t crit × SE (ˆ β ) ≤ ˆ β − β∗ ≤ +t crit × SE (ˆ β )) ˆ β − t crit × SE (ˆ β ) ≤ β∗ ≤ ˆ β + t crit × SE (ˆ β )) But this is just the rule under the confidence interval approach. Dr Artur Semeyutin (BIE0014) HYT Business School18 / 46 Constructing Tests of Significance and Confidence Intervals: An Example Using the regression results above, ˆ y t = 20 .3 + 0 .5091 x t , T= 22 (14 .38) (0 .2561) Using both the test of significance and confidence interval approaches, test the hypothesis that β= 1 against a two-sided alternative. The first step is to obtain the critical value. We want t crit = t 20;5% Dr Artur Semeyutin (BIE0014) HYT Business School19 / 46 Determining the Rejection Region Dr Artur Semeyutin (BIE0014) HYT Business School20 / 46x 95% non-rejection region 2.5% re jection region 2.5% re jection region –2.086 +2.086 x f ( ) Performing the Test The hypotheses are: H0: β = 1 H 1: β ̸ = 1 Test of significance approach Confidence interval approach test stat =ˆ β − β∗ SE (ˆ β ) = 0 .5091 −1 0 .2561 = −1.917 Find t crit = t 20;5% = ±2.086 ˆ β ± t crit · SE (ˆ β ) = 0 .5091 ±2.086 ·0 .2561 = ( −0.0251 ,1 .0433) Do not reject H 0 since test statistic Do not reject H 0 since 1 lies lies within non-rejection region within the confidence interval Dr Artur Semeyutin (BIE0014) HYT Business School21 / 46 Testing other Hypotheses What if we wanted to test H 0: β = 0 or H 0: β = 2? Note that we can test these with the confidence interval approach. For interest (!), test H0: β = 0 vs. H 1: β ̸ = 0 H 0: β = 2 vs. H 1: β ̸ = 2 Dr Artur Semeyutin (BIE0014) HYT Business School22 / 46 Changing the Size of the Test But note that we looked at only a 5% size of test. In marginal cases (e.g. H 0: β = 1), we may get a completely different answer if we use a different size of test. This is where the test of significance approach is better than a confidence interval. For example, say we wanted to use a 10% size of test. Using the test of significance approach, test stat=ˆ β − β∗ SE (ˆ β ) = 0 .5091 −1 0 .2561 = −1.917 as above. The only thing that changes is the critical t-value. Dr Artur Semeyutin (BIE0014) HYT Business School23 / 46 Changing the Size of the Test: The New Rejection Regions Dr Artur Semeyutin (BIE0014) HYT Business School24 / 46 Changing the Size of the Test: The Conclusion t 20;10% = 1.725. So now, as the test statistic lies in the rejection region, we would reject H 0. Caution should therefore be used when placing emphasis on or making decisions in marginal cases (i.e. in cases where we only just reject or not reject). Dr Artur Semeyutin (BIE0014) HYT Business School25 / 46 Some More Terminology If we reject the null hypothesis at the 5% level, we say that the result of the test is statistically significant. Note that a statistically significant result may be of no practical significance. E.g. if a shipment of cans of beans is expected to weigh 450g per tin, but the actual mean weight of some tins is 449g, the result may be highly statistically significant but presumably nobody would care about 1g of beans. Dr Artur Semeyutin (BIE0014) HYT Business School26 / 46 A Special Type of Hypothesis Test: The t− ratio Recall that the formula for a test of significance approach to hypothesis testing using a t-test was test statistic=ˆ β i− β∗ i SE (ˆ β i) If the test is H 0: β i = 0 H 1: β i ̸ = 0 i.e. a test that the population coefficient is zero against a two-sided alternative, this is known as a t-ratio test: Since β∗ i = 0, test stat =ˆ β i SE (ˆ β i) The ratio of the coefficient to its SE is known as the t-ratio or t -statistic. Dr Artur Semeyutin (BIE0014) HYT Business School27 / 46 The t-ratio: An Example Suppose that we have the following parameter estimates, standard errors and t-ratios for an intercept and slope respectively. Coefficient 1.10 -4.40 SE 1.35 0.96 t -ratio 0.81 -4.63 Compare this with a t crit with 15-3 = 12 d.f. (2 1 2 % in each tail for a 5% test) = 2.179 5% = 3.055 1%Do we reject H 0: β 1 = 0? (No) H 0: β 2 = 0? (Yes) Dr Artur Semeyutin (BIE0014) HYT Business School28 / 46 What Does the t-ratio tell us? If we reject H 0, we say that the result is significant. If the coefficient is not “significant” (e.g. the intercept coefficient in the last regression above), then it means that the variable is not helping to explain variations in y. Variables that are not significant are usually removed from the regression model. In practice there are good statistical reasons for always having a constant even if it is not significant. Look at what happens if no intercept is included: Dr Artur Semeyutin (BIE0014) HYT Business School29 / 46 yt xt Testing Multiple Hypotheses: The F-test We used the t-test to test single hypotheses, i.e. hypotheses involving only one coefficient. But what if we want to test more than one coefficient simultaneously? We do this using the F-test. The F-test involves estimating 2 regressions. The unrestricted regression is the one in which the coefficients are freely determined by the data, as we have done before. The restricted regression is the one in which the coefficients are restricted, i.e. the restrictions are imposed on some βs. Dr Artur Semeyutin (BIE0014) HYT Business School30 / 46 The F-test: Restricted and Unrestricted Regressions Example The general regression is yt = β 1 + β 2x 2t + β 3x 3t + β 4x 4t + u t We want to test the restriction that β 3 + β 4 = 1 (we have some hypothesis from theory which suggests that this would be an interesting hypothesis to study). The unrestricted regression is (31) above, but what is the restricted regression? yt = β 1 + β 2x 2t + β 3x 3t + β 4x 4t + u t s.t. β 3 + β 4 = 1 We substitute the restriction ( β 3 + β 4 = 1) into the regression so that it is automatically imposed on the data. β3 + β 4 = 1 ⇒β 4 = 1 −β 3 Dr Artur Semeyutin (BIE0014) HYT Business School31 / 46 The F-test: Forming the Restricted Regression yt = β 1 + β 2x 2t + β 3x 3t + (1 −β 3) x 4t + u t y t = β 1 + β 2x 2t + β 3x 3t + x 4t − β 3x 4t + u t Gather terms in β’s together and rearrange ( y t − x 4t) = β 1 + β 2x 2t + β 3( x 3t − x 4t) + u t This is the restricted regression. We actually estimate it by creating two new variables, call them, say, P t and Q t. P t = y t − x 4t Q t = x 3t − x 4t So P t = β 1 + β 2x 2t + β 3Q t+ u t is the restricted regression we actually estimate. Dr Artur Semeyutin (BIE0014) HYT Business School32 / 46 Calculating the F-Test Statistic The test statistic is given by test statistic =RRSS −URSS URSS × T −k m where URSS =RSS from unrestricted regression RRSS =RSS from restricted regression m = number of restrictions T = number of observations k = number of regressors in unrestricted regression including a constant in the unrestricted regression (or the total number of parameters to be estimated). Dr Artur Semeyutin (BIE0014) HYT Business School33 / 46 The F-Distribution The test statistic follows the F-distribution, which has 2 d.f. parameters. The value of the degrees of freedom parameters are mand ( T-k) respectively (the order of the d.f. parameters is important). The appropriate critical value will be in column m, row ( T-k). The F-distribution has only positive values and is not symmetrical. We therefore only reject the null if the test statistic >critical F-value. Dr Artur Semeyutin (BIE0014) HYT Business School34 / 46 Determining the Number of Restrictions in an F -test Examples : H0 : hypothesis No .of restrictions ,m β 1 + β 2 = 2 1 β 2 = 1 and β 3 = −1 2 β 2 = 0 , β 3= 0 and β 4 = 0 3 If the model is y t = β 1 + β 2x 2t + β 3x 3t + β 4tx 4t + u t, then the null hypothesis H 0 : β 2 = 0, and β 3 = 0 and β 4 = 0 is tested by the regression F -statistic. It tests the null hypothesis that all of the coefficients except the intercept coefficient are zero. Note the form of the alternative hypothesis for all tests when more than one restriction is involved: H 1 : β 2 ̸ = 0, or β 3 ̸ = 0 or β 4 ̸ = 0 Dr Artur Semeyutin (BIE0014) HYT Business School35 / 46 What we Cannot Test with Either an For a t-test We cannot test using this framework hypotheses which are not linear or which are multiplicative, e.g. H0 : β 2β 3 = 2 or H 0 : β 2 2 = 1 cannot be tested. Dr Artur Semeyutin (BIE0014) HYT Business School36 / 46 The Relationship between the tand the F -Distributions Any hypothesis which could be tested with a t-test could have been tested using an F-test, but not the other way around. For example, consider the hypothesis H0 : β 2 = 0 .5 H 1 : β 2 ̸ = 0 .5 We could have tested this using the usual t-test: test stat =ˆ β 2− 0.5 SE (ˆ β 2) or it could be tested in the framework above for the F-test. Note that the two tests always give the same result since the t -distribution is just a special case of the F-distribution. For example, if we have some random variable Z, and Z∼ t(T −k) then also Z2 ∼ F(1 ,T −k) Dr Artur Semeyutin (BIE0014) HYT Business School37 / 46 F -test Example Question: Suppose a researcher wants to test whether the returns on a company stock ( y) show unit sensitivity to two factors (factor x 2 and factor x 3) among three considered. The regression is carried out on 144 monthly observations. The regression is y t = β 1 + β 2x 2t + β 3x 3t + β 4x 4t + u t –What are the restricted and unrestricted regressions? –If the two RSS are 436.1 and 397.2 respectively, perform the test. Solution: Unit sensitivity implies H 0: β 2 = 1 and β 3 = 1. The unrestricted regression is the one in the question. The restricted regression is ( y t − x 2t − x 3t) = β 1 + β 4x 4t + u t or letting z t = y t − x 2t − x 3t, the restricted regression is z t = β 1 + β 4x 4t + u t In the F-test formula, T=144, k=4, m=2, RRSS =436.1, URSS=397.2Dr Artur Semeyutin (BIE0014) HYT Business School38 / 46 F -test Example (Cont’d) F -test statistic = 6.68. Critical value is an F(2,140) = 3.07 (5%) and 4.79 (1%). Conclusion: Reject H 0. Dr Artur Semeyutin (BIE0014) HYT Business School39 / 46 Data Mining Data mining is searching many series for statistical relationships without theoretical justification. For example, suppose we generate one dependent variable and twenty explanatory variables completely randomly and independently of each other. If we regress the dependent variable separately on each independent variable, on average one slope coefficient will be significant at 5%. If data mining occurs, the true significance level will be greater than the nominal significance level. Dr Artur Semeyutin (BIE0014) HYT Business School40 / 46 Goodness of Fit Statistics We would like some measure of how well our regression model actually fits the data. We have goodness of fit statistics to test this: i.e. how well the sample regression function (srf ) fits the data. The most common goodness of fit statistic is known as R2 . One way to define R2 is to say that it is the square of the correlation coefficient between yand ˆ y. For another explanation, recall that what we are interested in doing is explaining the variability of yabout its mean value, , i.e. the total sum of squares, TSS: TSS =X t ( y t − ¯ y )2 We can split the TSSinto two parts, the part which we have explained (known as the explained sum of squares, ESS) and the part which we did not explain using the model (the RSS).Dr Artur Semeyutin (BIE0014) HYT Business School41 / 46 Defining R2 That is, TSS=ESS +RSS X t ( y t − ¯ y )2 = X t ( ˆ y t − ¯ y )2 + X t ˆ u 2 t Our goodness of fit statistic is R2 = ESS TSS But since TSS = ESS + RSS , we can also write R 2 = ESS TSS = TSS −RSS TSS = 1 −RSS TSS R 2 must always lie between zero and one. To understand this, consider two extremes RSS =TSS i.e . ESS = 0 soR2 = ESS /TSS = 0 ESS =TSS i.e . RSS = 0 soR2 = ESS /TSS = 1Dr Artur Semeyutin (BIE0014) HYT Business School42 / 46 The Limit Cases: R2 = 0 and R2 = 1 Dr Artur Semeyutin (BIE0014) HYT Business School43 / 46y – y t xt yt xt Problems with R2 as a Goodness of Fit Measure There are a number of them: 1 R 2 is defined in terms of variation about the mean of yso that if a model is reparameterised (rearranged) and the dependent variable changes, R2 will change. 2 R 2 never falls if more regressors are added. to the regression, e.g. consider: Regression 1 :y t = β 1 + β 2x 2t + β 3x 3t + u t Regression 2 :y t = β 1 + β 2x 2t + β 3x 3t + β 4x 4t + u t R 2 will always be at least as high for regression 2 relative to regression 1. 3 R 2 quite often takes on values of 0.9 or higher for time series regressions. Dr Artur Semeyutin (BIE0014) HYT Business School44 / 46 Adjusted R2 In order to get around these problems, a modification is often made which takes into account the loss of degrees of freedom associated with adding extra variables. This is known as ¯ R 2 , or adjusted R2: ¯ R 2 = 1 − T −1 T −k(1 −R2 ) So if we add an extra regressor, kincreases and unless R2 increases by a more than offsetting amount, ¯ R 2 will actually fall. There are still problems with the criterion: 1 A “soft” rule 2 No distribution for ¯ R 2 or R2 Dr Artur Semeyutin (BIE0014) HYT Business School45 / 46 Essential Reading Please read (finish relevant parts) the textbook chapters: Chris Brooks - Introductory Econometrics for Finance, 4th Edition (2019) Cambridge University Press, Chapters 3 and 4. Or read: Jeffrey Wooldridge - Introductory Econometrics, 7th Edition (2019) Cengage, Chapter 4. Dr Artur Semeyutin (BIE0014) HYT Business School46 / 46