Review Session Midterm 1 PDF
Document Details
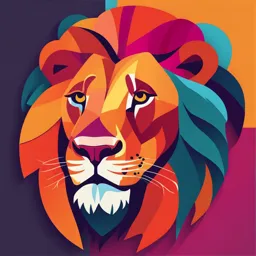
Uploaded by SwiftPrimrose2997
University of California, Berkeley
2024
Jeremy Magruder
Tags
Related
- BMS2043 Statistics & Data Analysis Lecture 3 2024 PDF
- Learning Statistics with R - A Tutorial for Psychology Students PDF
- Homework 3: Multiple Linear Regression
- Week 5 - Simple Regression Model - PART II PDF Lecture Notes
- Applied Econometrics (5AEC) Specimen Test PDF - The London Institute of Banking & Finance
- Linear Regression: Statistical Models and Hypothesis Testing - PDF
Summary
This document is a review session for a midterm exam, covering concepts from statistics, hypothesis testing, simple and multiple linear regressions, and related topics. Key concepts addressed include population vs. sample, estimator characteristics, and different types of regression analysis.
Full Transcript
Review Session Jeremy Magruder 2024 Review Outline 1 Concepts from Statistics 2 Hypothesis Testing 3 simple regression 4 multiple regression Concepts from Statistics 1 Population vs. Sample 2 Random Variable Characteristics Expected Value...
Review Session Jeremy Magruder 2024 Review Outline 1 Concepts from Statistics 2 Hypothesis Testing 3 simple regression 4 multiple regression Concepts from Statistics 1 Population vs. Sample 2 Random Variable Characteristics Expected Value - E [X ] = µ Conditional Expectations E [Y |X ] Variance - E [X 2 − µ2x ] = σ2 , Covariance E [(X − µx )(Y − µY )] Probability Density Function - espec. t and Normal Distributions Estimator Characteristics Unbiasedness - e.g. E [X̄ ] = µ Confidence Intervals e.g.(X̄ − cα/2 ∗ √σn , X̄ + cα/2 ∗ √σ ) n cases for normal vs. t distributions Hypothesis Testing null hypothesis vs. one-sided and two-sided alternate hypotheses How to reject the null? Conclusions from rejecting the null? type 1 versus type 2 error tests of differences in means p-values Simple Linear Regressions First Order Conditions in OLS - derivation and interpretations interpretations of β 1 and β 0 β 1 interpretations with different functional forms of X and Y graphical interpretations - β 0 , β 1 , residuals, predicted values cov\ (X ,Y ) βˆ1 = \ var (X ) R 2 = 1-SSR/SST Simple Linear Regression Assumptions SLR1 - yi = β 0 + β 1 Xi + ui SLR2 - the data are a random sample from the population SLR3 - there is variation in X SLR4 - E [u |X ] = 0 SLR5 = var (u |X ) = sigma2 what results do SLR1-4 provide? SLR1-5? Variance of OLS Estimator with SLR1-5 βˆ1 ∼ tn−1 σu2 var ( βˆ1 = ∑ i −X̄ )2 ( X ∑i uˆi2 var ( βˆ1 ) = (n−2)(∑i (Xi −X̄ )2 ) t-statistics and hypothesis testing Multiple Linear Regressions ceteris paribus interpretations MLR1-MLR6 omitted variable bias effect on parameters ∑ uˆi2 variance of βˆk = (n−k −1) ∑i (xji −x¯j 2 )(1−Rj2 ) β̂ j ∼ tn−k −1 testing in MLR formulating null hypotheses about parameters of interest one and two-sided tests of regression parameters t-tests for individual β j parameters substituting variable changes for some complicated hypotheses (e.g. β 1 = β 2 ) F -tests for tests on multiple parameters (e.g. β 2 = β 3 = β 4 = 0) last extensions (SSRr −SSRu )/q F = SSRu /(n−k −1) (Ru2 −Rr2 )/q F = (1−Ru2 )/(n−k −1) overall F -test unit changes in X and Y - results and derivation E-mailed Questions (1) Q: If the SLR/MLR assumptions are met, can we conclude causality? E-mailed Questions (1) Q: If the SLR/MLR assumptions are met, can we conclude causality? A: if SLR/MLR assumptions 1-4 are met, then E [ β̂ j ] = β j E-mailed Questions (1) Q: If the SLR/MLR assumptions are met, can we conclude causality? A: if SLR/MLR assumptions 1-4 are met, then E [ β̂ j ] = β j Or, in other words, our estimate from the sample will on average be the same as the true value in the population the true value in the population is causal So, our estimate is still a random variable, and won’t be exactly the same as the causal relationship - if the standard errors are large, it may be a lot different! but we have an unbiased estimate of the causal relationship E-mailed Questions (2) σu2 Q: What is the difference between var ( βˆ1 ) = ∑i (xi −x̄ )2 and σ2 var ( β̂ j ) = (1−Rj2 ) ∑i (Xi −X̄ )2 ? E-mailed Questions (2) σu2 Q: What is the difference between var ( βˆ1 ) = ∑i (xi −x̄ )2 and σ2 var ( β̂ j ) = (1−Rj2 ) ∑i (Xi −X̄ )2 ? A: The first is from a Simple Linear Regression, the second from a multiple linear regression E-mailed Questions (2) σu2 Q: What is the difference between var ( βˆ1 ) = ∑i (xi −x̄ )2 and σ2 var ( β̂ j ) = (1−Rj2 ) ∑i (Xi −X̄ )2 ? A: The first is from a Simple Linear Regression, the second from a multiple linear regression Notation would be more clear if the second piece read 2 var ( βˆj ) = (1−R 2 ) σ (X −X̄ )2 j ∑i ji j In a multiple linear regression, the variance used to identify the coefficients is the part not explained by the other variables This is captured by Rj2 , the R 2 from a regression of Xj on the other X variables E-mailed Questions (3) Q: What does it mean to specify β 1 in terms of r as in y = β 0 + β 1 x1 + β 2 x2 + u (1) x1 = δ0 + δ1 x2 + r (2) cov (rˆ, y ) βˆ1 = (3) var (rˆ) E-mailed Questions (3) Q: What does it mean to specify β 1 in terms of r as in y = β 0 + β 1 x1 + β 2 x2 + u (1) x1 = δ0 + δ1 x2 + r (2) cov (rˆ, y ) βˆ1 = (3) var (rˆ) A: this is the "residualing-out" interpretation of MLR. If the true model features both x1 and x2 then our estimate for β 1 should be based on the residuals from a regression of x1 on x2 , not the full variation in x1 E-mailed Questions (4) Q: How much detail is expected? If the question asks for a test-statistic do we need to complete the hypothesis test, or just write down the test statistic? E-mailed Questions (4) Q: How much detail is expected? If the question asks for a test-statistic do we need to complete the hypothesis test, or just write down the test statistic? A: You don’t need to answer questions that aren’t asked. So, if it asks for a test statistic, but does not ask for a hypothesis test, you do not need to complete the hypothesis test. If we’re interested in a hypothesis test, and it’s feasible to complete with the tools you have in front of you, we typically will ask for one; if we don’t it may not be feasible. E-mailed Questions(5) Q: Could you go over omitted variable bias? In particular, the direction of bias with cov (y , xov ) and cov (x, xov )? E-mailed Questions(5) Q: Could you go over omitted variable bias? In particular, the direction of bias with cov (y , xov ) and cov (x, xov )? A: Let’s suppose yi = β 0 + β 1 xi + β 2 xovi + ui. But, xov is omitted, so you regress yi = β 0 + β 1 xi + ui. Consider xovi = δ0 + δ1 xi + ri Then yi = ( β 0 + β 2 δ0 ) + ( β 1 + β 2 δ1 )xi + ui + β 2 ri E-mailed Questions(5) Q: Could you go over omitted variable bias? In particular, the direction of bias with cov (y , xov ) and cov (x, xov )? A: Let’s suppose yi = β 0 + β 1 xi + β 2 xovi + ui. But, xov is omitted, so you regress yi = β 0 + β 1 xi + ui. Consider xovi = δ0 + δ1 xi + ri Then yi = ( β 0 + β 2 δ0 ) + ( β 1 + β 2 δ1 )xi + ui + β 2 ri cov (y ,x1 ) var (x1 ) = β1 + β 2 δ1. β 2 δ1 is the omitted variable bias. cov (x,xov ) δ1 = var (xov ) β 2 δ1 > 0 if β 2 and δ1 have the same sign. cov (y ,x ) β 2 δ1 > 0 ⇒ var (x ) > β 1 β 2 δ1 < 0 if β 2 and δ1 have opposite signs. cov (y ,x ) β 2 δ1 < 0 ⇒ var (x ) < β 1