Week 15 Causal Inference BIOE211 PDF
Document Details
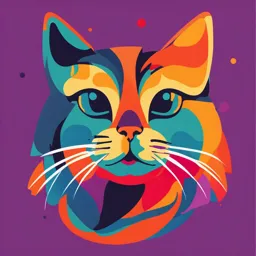
Uploaded by RightfulSydneyOperaHouse7819
Our Lady of Fatima University
2024
null
Ma. Christy V. Gonzales
Tags
Summary
These notes cover week 15 of a Biostatistics and Epidemiology course, focusing on causal inference. They discuss statistical association, internal and external validity, and the goal of epidemiologic studies, along with potential biases and confounding factors.
Full Transcript
BIOE211: BIOSTATISTICS AND EPIDEMIOLOGY WEEK 15: CAUSAL INFERENCE PERIOD: FINALS, 2ND YEAR, A.Y. 2023 – 2024 PROFESSOR: MA. CHRISTY V. GONZALES, RMT, MPH STATISTICAL ASSOCIATION CAUSAL INFERENCE  statistical dependence between t...
BIOE211: BIOSTATISTICS AND EPIDEMIOLOGY WEEK 15: CAUSAL INFERENCE PERIOD: FINALS, 2ND YEAR, A.Y. 2023 – 2024 PROFESSOR: MA. CHRISTY V. GONZALES, RMT, MPH STATISTICAL ASSOCIATION CAUSAL INFERENCE  statistical dependence between two variables Internal Validity  either positive or negative (association)  Validity within the study  process of using statistical methods to characterize  Estimate of effect measure is accurate the association between variables.  Not due to systematic error External Validity CAUSAL INFERENCE  Validity beyond the study  process of ascribing causal relationships to  Estimate generalizable to bigger population associations between variables  Not due to random error Types of association  causal GOAL OF EPIDEMIOLOGIC STUDIES  direct  to estimate the value of the parameter with little  indirect error  noncausal Sources of errors: What is a Cause?  random errors: sampling errors  Factor that plays an essential role in producing an  difference between POPULATION outcome/disease VALUE of parameter being investigated and the ESTIMATE VALUE based on ASSOCIATION VS. CAUSE the different samples Association  systematic errors: biases and confounding  Identifiable relationship between exposure and  distortion in the estimation of the disease magnitude of association between Cause EXPOSURE (E) and DISEASE (D) (over  Presence of mechanism that leads from exposure or under estimation) to disease  deviation from the truth  due to bias CAUSAL VS. NON-CAUSAL  selection Causal  information  Alteration in the frequency or quality of one event is  confounding followed by a change in the other Non-causal BIAS  Association is a result of the relationship of both  Selection Bias: non-representative sample factor and disease with a third variable  Information Bias: inaccurate information collected Causal Non-causal from sample  Misclassification  Differential (non-random) and Non-differential (random)  sources:  instrument  subjects  observers CONFOUNDING  Mixing the effect of the exposure on the disease with that of a 3rd factor  CONFOUNDER  Associated with the exposure  Risk factor of the disease  Can lead to over / under estimation of the association DIRECT VS INDIRECT Direct Indirect METHODS TO CONTROL CONFOUNDING A. Design stage  Randomization: aim is random distribution of confounders between study g roups  Restriction: restrict entry to study of individuals with confounding factor  Matching: aim for equal distribution of confounders B. Analysis stage  Stratified analysis: confounders are distributed evenly within each stratum PROCESS OF CAUSAL INFERENCE  Multivariate analysis: there are a lot of Step 1: Determine the validity of the association statistical tests that may be applied so  rule out chance, bias, confounding as explanation of that you may be able to come up with a the observed association good analysis/result/data Step 2: Determine if observed association is causal  consider totality of evidence taken from a number of sources  HILL’S CRITERIA 11/29/23 BIOE211: BIOSTATISTICS AND EPIDEMIOLOGY WEEK 15: CAUSAL INFERENCE BRADFORD HILL’S CRITERIA FOR CAUSAL INFERENCE  Strength of association  The higher the risk ratio, the higher or it is most likely to be causal  Temporality  Temporal relationship – the exposure precedes diseases  Meaning, when there is exposure, exposure is preceded to disease  Consistency  Consistent findings across different designs, populations, investigators  Whoever investigates it, whatever study design, and whatever population, but with the same exposure data and outcome data, result will be consistent  Example: In diabetes, our risk factor is diet. Whatever study design is used, whoever investigates it, and whatever population is included, if diet is really the cause of diabetes mellitus and is really associated and is really causal, we can establish that diet is the cause of diabetes.  Theoretical plausibility  It does not contradict the natural history of the disease or biology of the disease.  Meaning, whatever the result will be between the exposure and disease, it will not be contradicting to what we already know in science.  Example: Smoking and hypertension – if we say that smoking is causal of hypertension and it is still not known or proven as a study, it contradicts the natural history of the disease. So, it will not be considered as cause of hypertension.  Coherence  Specificity in the causes  Exposure only leads to a single effect  Dose-response relationship  If you increase the dose, outcome is affected  Experimental evidence  Analogy 11/29/23