Statistical Inference – Population Proportion PDF
Document Details
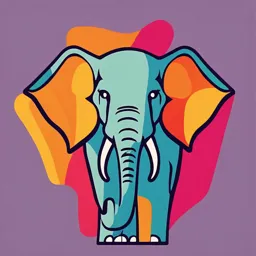
Uploaded by ExceedingChrysoprase7632
Monash University
Tags
Summary
This document details statistical inference, specifically focusing on hypothesis testing for population proportions. It provides examples of how to state hypotheses, check conditions, and calculate test statistics for determining if a claimed proportion is supported by data. Examples for proportions of smooth peas, and whether people prefer a particular drink are included.
Full Transcript
Statistical Inference – Population Proportion Test of Significance for a Proportion 2 Test of Significance for a Proportion, Step 1: Hypotheses The four st...
Statistical Inference – Population Proportion Test of Significance for a Proportion 2 Test of Significance for a Proportion, Step 1: Hypotheses The four steps in carrying out a significance test: Step 1: State the Hypotheses 1. State the null and alternative hypotheses. Null hypothesis H0 : p = p 0 - p0 is called null value. 2. Check conditions and then Calculate the Alternative hypothesis H a: p > p 0 (one-sided, right sided) test statistic. or H a: p < p 0 (one-sided, left sided) 3. Find the P-value using the appropriate distribution. or H a: p ≠ p 0 (2-sided) 4. Make a decision and state your conclusion in the context of the specific setting of the test. AS ! ALWAYS Examples: 1. A parliamentarian will vote for a proposal if there is conclusive evidence that a majority of her constituents favour that proposal. H0 : p = 0.5 vs. Ha: p > 0.5 2. A pharmaceutical company wants to claim that the proportion of patients who experience side effects is less than 20% with a new drug. H0 : p = 0.2 vs. Ha: p < 0.2 3. The proportion of children being raised by grandparents in a particular country has always been 5%. Has this changed? H0 : p = 0.05 vs. Ha: p ≠ 0.5 3 Step 2a: Condition Check, Step 3: Test Statistic Step 2a: Check Conditions 1. The sample should be a random sample from the population. Not always practical, but OK as long as sample is representative of the population for the question of interest. 2. Sample size must be large enough to ensure the approximately normality of the sampling distribution. For tests: check if both np0 and n(1-p0) are at least 10. 4 Step 3: p-value, Step 4: Decision and Conclusion Step 3: P-value Step 4: Decision and Conclusion Whether or not the result is statistically significant is based on the p-value and the chosen level of significance α. If the p-value ≤ α 🡪 Reject null hypothesis concluding there is statistically significant evidence for the alternative hypothesis. If p-value > α 🡪 Cannot reject null hypothesis with conclusion that there is not sufficient evidence to support the alternative hypothesis. 5 Example: Mendel’s peas Pure breed peas were crossed to produce smooth and wrinkled peas. The first-generation peas were all smooth. In the second generation (F2), 5474 smooth and 1850 wrinkled peas were obtained. Do the data agree with the conclusion of 75% dominant trait (here “smooth”) occurrence in F2? Test at a significance level of 0.05. 4) Decision: Since p-value (0.610)>alpha 0.51 -0.51 (0.05), we fail to reject H0. Conclusion: There is no significant evidence at 5% level of significance, that proportion of dominant (smooth) trait occurring in F2 is different to 75%. Data supports H0 and agrees with conclusion of 75% dominant trait in F2. 6 Effect of Sample Size on Statistical Significance Sample 1: fail to reject H0. Sample 2: Reject H0. Larger sample size => easier to reject the null hypothesis! 7 Conditions Summary