Topic 4 Descriptive Statistics for Economic Data PDF
Document Details
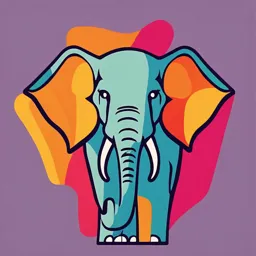
Uploaded by FerventChlorine
Camm, Cochran, Fry, Ohlmann, Anderson, Sweeney, Williams
Tags
Summary
This document covers descriptive statistics, focusing on categorical and quantitative data, frequency distributions, relative/percent frequency, numerical measures, variability (range, interquartile range, variance, standard deviation, coefficient of variation), skewness, outliers, and z-scores.
Full Transcript
Data Analytics in Economics Topic 4 Descriptive Statistics for Economic Data Camm, Cochran, Fry, Ohlmann, Anderson, Sweeney, Williams, Statistics for Business & Economics, 15th Edition. © 2024 Cengage Gro...
Data Analytics in Economics Topic 4 Descriptive Statistics for Economic Data Camm, Cochran, Fry, Ohlmann, Anderson, Sweeney, Williams, Statistics for Business & Economics, 15th Edition. © 2024 Cengage Group. All Rights Reserved. May not be scanned, copied or duplicated, or posted to a publicly accessible website, in whole or in part. Topic Contents 4.1 Summarizing Categorical Variable 4.2 Summarizing Quantitative Variable 4.3 Numerical Measures 4.4 Measuring Variability 4.5 Measuring Distribution Shape and Outliers Detection 4.1 Summarizing Categorical Variable Examples of categorical data – race, sex, age group, educational level, etc. Frequently asked questions related to categorical data: 1. How do you make a summary of these data? 2. Is there a way to summarize them numerically? 4.1 Summarizing Categorical Variable Frequency Distribution A tabular summary of data showing the number (frequency) of observations in each of several non-overlapping categories or classes. To develop a frequency distribution for the sample of 50 soft drink purchases, we count the number of times each soft drink appears. The frequency distribution highlights Coca- Cola as the leader, followed by Pepsi, Diet Coke, Dr. Pepper, and Sprite. 4.1 Summarizing Categorical Variable Relative Frequency and Percent Frequency Distributions The relative frequency of a class is the fraction or * In the example proportion of the total number of data items belonging here, n=50 to the class. Frequency of the class Relative Frequency = 𝑛 The percent frequency of a class is the relative frequency multiplied by 100. The relative frequency distribution for the soft drink data shows a relative frequency of 19/50 = 0.38 for Coca-Cola, 13/50 = 0.26 for Pepsi, and so on. The percent frequency distribution, shows 38% Coca- Cola purchases, 16% Diet Coke purchases, and so on. 4.2 Summarizing Quantitative Variable Frequency Distribution To build a frequency distribution for quantitative data, we must be more careful in defining the non- overlapping classes to be used in the frequency distribution. Three steps to define the classes for a frequency distribution with quantitative data are: 1. Determine number of classes: from 5, for small data sets, up to 20 for large data sets. 2. Determine width of the classes: Largest Data Value − Smallest Data Value Approx. class width = Number of classes 3. Class limits: chosen so that classes do not overlap, and each data item belongs to only one class. Example Audit Time (days) Frequency The data shows the time in days required to complete 10-14 4 year-end audits for a sample of 20 clients of a small 15-19 8 public accounting firm. Five classes were chosen, with class width = 20-24 5 (33 − 12)⁄5 = 4.2 ≈ 5. The resulting frequency 25-29 2 distribution is shown to the right. 30-34 1 Total 20 © 2024 Cengage Group. All Rights Reserved. 4.2 Summarizing Quantitative Variable Relative Frequency and Percent Frequency Distributions We define the relative frequency and percent frequency distributions for quantitative data in the same manner as for categorical data. Frequency of the class Relative Frequency of a class = 𝑛 Remember that the percent frequency of a class is the relative frequency multiplied by 100. Based on the class frequencies for the 20 Audit Time (days) Relative Frequency Percent Frequency audit times, the table shows the relative 10-14 0.20 20 frequency distribution and percent 15-19 0.40 40 frequency distribution. 20-24 0.25 25 Note that 40% of the audits required 25-29 0.10 10 between 15 and 19 days, and only 5% of the audit required more 30 or more days. 30-34 0.05 5 Total 1.00 100 © 2024 Cengage Group. All Rights Reserved. 4.2 Summarizing Quantitative Variable Cumulative Distributions A cumulative frequency distribution shows the number of items with values less than or equal to the upper limit of each class. A cumulative relative frequency distribution shows the proportion of items with values less than or equal to the upper limit of each class. A cumulative percent frequency distribution shows the percentage of items with values less than or equal to the upper limit of each class. The distributions in the table show that 85% of the audits were completed in 24 days or less, 95% of the audits were completed in 29 days or less, and so on. Audit Time (days) Cumulative Frequency Cumulative Relative Frequency Cumulative Percent Frequency Less than or equal to 14 4 0.20 20 Less than or equal to 19 12 0.60 60 Less than or equal to 24 17 0.85 85 Less than or equal to 29 19 0.95 95 Less than or equal to 34 20 1.00 100 © 2024 Cengage Group. All Rights Reserved. 4.3 Numerical Measures For data sets consisting of a single variable (e.g., income), we may develop numerical summary measures – mean, mode and median. When a data set contains more than one variable (e.g., income and expenditure), the same numerical measures can be computed separately for each variable. However, in the two-variable case, we will also develop measures of the relationship between the variables. If the measures are computed for data from a sample, they are called sample statistics. If the measures are computed for data from a population, they are called population parameters. A sample statistic is referred to as the point estimator of the corresponding population parameter. Statistical software packages and spreadsheets can be used to develop the descriptive statistics presented in this chapter. Source: Household Income Survey Report 2022, DOSM Source: Household Expenditure Survey Report 2022, DOSM 4.3 Numerical Measures Mean Mean, the average of all the data values is the most important measure of central location. For a sample with n observations, the formula for the sample mean is as follows. ∑ 𝒙𝒊 A= 𝒙 where 𝑥" is the 𝑖th observation in a data set of size 𝑛 𝒏 Example: consider the class size data for a sample of five college classes: 46, 54, 42, 46, 32. Using the introduced notation, we have: 𝑥# =46, 𝑥$ =54, 𝑥% =42, 𝑥& =46, and 𝑥' =32. To compute the sample mean, we write: ∑ 𝑥" ∑ 𝑥# + 𝑥$ + 𝑥% + 𝑥& + 𝑥' 46 + 54 + 42 + 46 + 32 𝑥̅ = = = = 44 𝑛 𝑛 5 A is the point estimator of the population mean, 𝜇. The sample mean 𝒙 ∑ 𝒙𝒊 𝝁= where 𝑁 is the size of the population 𝑵 © 2024 Cengage Group. All Rights Reserved. 4.3 Numerical Measures Mode The mode of a data set is the value that occurs with the greatest frequency. Example: consider the starting monthly salary for 12 business graduates. 5710 5755 5850 5880 5880 5890 5920 5940 5950 6050 6130 6325 $5,880 is the mode because it occurs more than once. It may happen that the greatest frequency occurs at two or more different values. In the presence of multiple modes, we define the following two cases: If the data have exactly two modes, the data are said to be bimodal. If the data have more than two modes, the data are said to be multimodal. © 2024 Cengage Group. All Rights Reserved. 4.3 Numerical Measures Median The median of a data set is the middle value when the data set is arranged in ascending or descending order. In the case of an even number of values, the median is the average of the two middle values. Let's consider the starting monthly salaries for 12 business graduates again: 5710, 5755, 5850, 5880, 5880, 5890, 5920, 5940, 5950, 6050, 6130, 6325 To find the median, we first rearrange the data set in ascending order: 5710, 5755, 5850, 5880, 5880, 5890, 5920, 5940, 5950, 6050, 6130, 6325 Since we have 12 values, which is an even number, we take the average of the two middle values. The two middle values are 5890 and 5920. Adding them together and dividing by 2 gives us (5890 + 5920) / 2 = 5905. Therefore, the median of this data set is $5,905. It's important to note that the median is not affected by extreme values in the data set, as it only considers the middle value(s). Additionally, the median is a useful measure of central tendency when dealing with skewed distributions or data sets with outliers. © 2024 Cengage Group. All Rights Reserved. 4.3 Numerical Measures Percentile A percentile provides information about how the data are spread over the interval from the smallest value to the largest value. Admission test scores for colleges and universities are frequently reported in terms of percentiles. The 𝑝th percentile of a data set is a value such that at least p percent of the items take on this value or less and at least (100– 𝑝) percent of the items take on this value or more. To calculate the 𝑝th percentile of a data set, we must first arrange the data in ascending order so that the smallest value in the data set is in position 1, the next one in position 2, and so on. The location of the 𝑝th percentile, denoted 𝐿( , is computed using the following equation: 𝑝 𝐿( = 𝑛+1 100 Now, we are ready to calculate the 𝑝th percentile. Let us do that with an example. © 2024 Cengage Group. All Rights Reserved. 4.3 Numerical Measures Percentile Example Compute the 80th percentile for the sample of 12 business graduates’ starting salaries. With the data rearranged in ascending order, we indicate the position of each observation directly below its value. 5710 5755 5850 5880 5880 5890 5920 5940 5950 6050 6130 6325 Position 1 2 3 4 5 6 7 8 9 10 11 12 The location of the 80th percentile is 𝑝 80 𝐿)* = 𝑛+1 = 12 + 1 = 10.4 100 100 The interpretation of 𝐿)* = 10.4 is that the 80th percentile is 40% between the values in position 10 and 11. To get the exact value: 80+, percentile = 6050 + 0.4 6130 − 6050 = 6050 + 0.4 80 = 6082 © 2024 Cengage Group. All Rights Reserved. 4.3 Numerical Measures Quartile Quartiles are specific percentiles that divide the data set into four parts, with each part containing approximately 25% of the observations. Quartiles are defined as follows: 𝑄# = first quartile, or 25th percentile 𝑄$ = second quartile, or 50th percentile (also the median) 𝑄% = third quartile, or 75th percentile The procedure for computing percentiles can be also used to compute quartiles. Let us calculate 𝑄# and 𝑄% for the sample of 12 business graduates’ starting salaries. First, we calculate the locations 25 75 𝐿$' = 12 + 1 = 3.25 and 𝐿-' = 12 + 1 = 9.75 100 100 The calculations of 𝑄# and 𝑄% follow: 𝑄# = 5850 + 0.25 5880 − 5850 = 5857.5 𝑄% = 5950 + 0.75 6050 − 5950 = 6025 © 2024 Cengage Group. All Rights Reserved. 4.3 Numerical Measures Decile Deciles are specific percentiles that divide a data set into ten equal parts, with each part containing approximately 10% of the observations. Deciles provide a way to understand the distribution of data by splitting it into ten segments. The deciles are defined as follows: D1 = first decile, or 10th percentile D2 = second decile, or 20th percentile... D9 = ninth decile, or 90th percentile D10 = tenth decile, or 100th percentile To calculate deciles, we can use the same procedure as calculating percentiles. Let's calculate D₁ and D₉ for the sample of 12 business graduates' starting salaries. © 2024 Cengage Group. All Rights Reserved. 4.3 Numerical Measures Decile First, we calculate the locations: 𝑝 𝐿( = 𝑛+1 100 L₁ = 10/100 * (12 + 1) = 1.3 L₉ = 90/100 * (12 + 1) = 11.7 Now we can calculate D₁ and D₉: D₁ = 5710 + 0.3 * (5755 - 5710) = 5729 D₉ = 6130 + 0.7 * (6325 - 6130) = 6234.5 Therefore, the first decile (D₁) of the starting salaries is approximately $5,729, and the ninth decile (D₉) is approximately $6,234.50. Deciles provide additional insights into the distribution of data beyond quartiles. They divide the data into smaller segments, allowing for a more detailed understanding of the distribution's shape and spread. © 2024 Cengage Group. All Rights Reserved. 4.3 Numerical Measures Quintile Quintiles are specific percentiles that divide a data set into five equal parts, with each part containing approximately 20% of the observations. Quintiles provide a way to analyze the distribution of data by dividing it into five segments. The quintiles are defined as follows: Q₁ = first quintile, or 20th percentile Q₂ = second quintile, or 40th percentile Q₃ = third quintile, or 60th percentile Q₄ = fourth quintile, or 80th percentile Q₄ = fifth quintile, or 100th percentile © 2024 Cengage Group. All Rights Reserved. 4.3 Numerical Measures Quintile To calculate quintiles, we can use the same procedure as calculating percentiles. Let's calculate Q₁ and Q₄ for the sample of 12 business graduates' starting salaries. First, we calculate the locations: L₂₀ = 20/100 * (12 + 1) = 2.2 L₈₀ = 80/100 * (12 + 1) = 9.8 Now we can calculate Q₁ and Q₄: Q₁ = 5710 + 0.2 * (5755 - 5710) = 5722 Q₄ = 5940 + 0.8 * (6050 - 5940) = 6028 Therefore, the first quintile (Q₁) of the starting salaries is approximately $5,722, and the fourth quintile (Q₄) is approximately $6,028. Quintiles provide further insights into the distribution of data, beyond quartiles and deciles. They divide the data into smaller segments, allowing for a more nuanced understanding of the distribution's characteristics and identifying potential outliers or differences between various sections of the data. © 2024 Cengage Group. All Rights Reserved. 4.3 Numerical Measures Quintile Source: Household Income Survey Report 2022, DOSM © 2024 Cengage Group. All Rights Reserved. 4.4 Measuring Variability It is desirable to consider measures of variability (dispersion), as well as measures of location. Consider the histograms for the number of days required to fill orders for two suppliers. Although the mean number of days is 10 for both suppliers and the supplier to the right is able to fill some orders in as little as 7 to 8 days, the supplier to the left has a lower dispersion and demonstrates a higher degree of reliability in terms of making deliveries on schedule. © 2024 Cengage Group. All Rights Reserved. 4.4 Measuring Variability Range and Interquartile Range Range Interquartile Range The range is the simplest measure of The interquartile range (IQR) overcomes the variability, and it is defined as dependency on extreme values considering Range = Largest Value – Smallest Value the range for the middle 50% of the data. For the example of the business graduates’ The interquartile range is calculated as the starting salaries, the range is difference between the third quartile, 𝑄% , and the first quartile, 𝑄#. 6325 − 5710 = 615 𝑰𝑸𝑹 = 𝑸𝟑 –𝑸𝟏 However, the range sensitivity to extreme data values makes it a poor choice to For the example of the business graduates’ measure the dispersion in a data set. starting salaries, the IQR is 6025 − 5857.5 = 167.5 © 2024 Cengage Group. All Rights Reserved. 4.4 Measuring Variability Variance The variance is a measure of variability that utilizes all the data. The variance is based on the difference between the value of each observation (𝑥" ) and the mean (𝑥̅ for a sample, 𝜇 for a population). The difference between each 𝑥" and the mean is called a deviation about the mean. In the computation of the variance, the deviations about the mean are squared. Population Variance Sample Variance ∑ 𝒙𝒊 3𝝁 𝟐 𝒙𝟐 ∑ 𝒙𝒊 36 𝝈𝟐 = 𝒔𝟐 = 𝑵 𝒏3𝟏 Where N is the population size. Where n is the sample size. © 2024 Cengage Group. All Rights Reserved. 4.4 Measuring Variability Variance Let us calculate the sample variance of the class size for the sample of five college classes. A summary of the data, including the computation of the deviations about the mean and the squared deviations about the mean, is shown in the table below. The sum of squared deviations about the mean is ∑ 𝑥" − 𝑥̅ $ = 256. With 𝑛 − 1 = 4, the sample variance is Number of Mean Class Squared Deviation ∑ 𝑥" − 𝑥̅ ! 256 Students in Class Size (" 𝒙) About the Mean 𝑠! = = = 64 (𝒙𝒊 ) " 𝟐 𝒙𝒊 − 𝒙 𝑛−1 4 46 44 4 Note that, because the variance is a squared 54 44 100 operator, its units are also squared. 42 44 4 In this case, the units are students $ , and not 46 44 4 students. 32 44 144 ∑ 𝑥# − 𝑥̅ $ = 256 © 2024 Cengage Group. All Rights Reserved. 4.4 Measuring Variability Standard Deviation The standard deviation is defined to be the positive square root of the variance. Sample standard deviation: 𝒔 = 𝒔𝟐 Population standard deviation: 𝝈 = 𝝈𝟐 In the previous example, we calculated the sample variance of the class size for the sample of five college classes as 𝑠 $ = 64. Thus, the sample standard deviation of the class size is 𝑠 = 64 = 8 students Because the standard deviation is the square root of the variance, the units of the variance, students $ , are converted to students in the standard deviation. Thus, the standard deviation is more easily compared to the mean and other statistics that are measured in the same units as the original data. © 2024 Cengage Group. All Rights Reserved. 4.4 Measuring Variability Coefficient of Variation The coefficient of variation, usually expressed as a percentage, measures how large the standard deviation is relative to the mean. Standard Deviation ×100 Mean For the class size of the sample of five college classes, we found a sample mean of 44 and a sample standard deviation of 8. The coefficient of variation is 8 ×100 = 18.2% 44 In other words, the coefficient of variation tells us that the sample standard deviation is 18.2% of the value of the sample mean. © 2024 Cengage Group. All Rights Reserved. 4.5 Measuring Distribution Shape and Outliers Detection Skewness Skewness is an important numerical measure of the shape of a distribution. Because of its complexity, skewness is usually calculated with the help of statistical software. Panel A: a distribution moderately skewed to the left has negative skewness. Panel B: a distribution moderately skewed to the right has positive skewness. Panel C: a symmetric distribution has the mean equal to the median, and skewness = 0. Panel D: a distribution highly skewed to the right has a larger positive skewness. © 2024 Cengage Group. All Rights Reserved. 4.5 Measuring Distribution Shape and Outliers Detection Skewness Key information obtained from data skewness includes: Shape of the distribution: Skewness allows us to understand the departure of the data from a symmetric distribution. (+)ve or (-)ve skewness indicates asymmetry in the data. Central tendency: Skewness affects the location of the mean, median and mode. In positively skewed data, the mean tends to be greater than the median, while in negatively skewed data, the mean is usually less than the median. Outlier detection: Skewness can reveal the presence of outliers. Extreme values in the higher or lower tail of the distribution can contribute to the skewness. Data transformation: Skewness helps determine if data transformation techniques like logarithmic or power transformations are necessary to achieve a more symmetric distribution. This transformation can be beneficial for certain statistical analyses or modeling purposes. © 2024 Cengage Group. All Rights Reserved. 4.5 Measuring Distribution Shape and Outliers Detection z-scores In addition to measures of location, variability, and shape, measures of relative location help us determine how far a particular value is from the mean. The z-score, often called the standardized value, denotes the number of standard deviations, s a data value x8 is from the mean, xd. 𝐱𝐢 3;𝐱 𝐳𝐢 = 𝐬 Number of Deviation About " 𝒙𝒊 − 𝒙 The z-scores for the class size data from the Students in the Mean z−Score 𝒔 previous section are computed to the right. Class (𝒙𝒊 ) " 𝒙𝒊 − 𝒙 For example, for 𝑥# = 46, the z-score is 46 2 2 / 8 = 0.25 54 10 10 / 8 = 1.25 42 2 ‒2 / 8 = -0.25 𝑥# − 𝑥̅ 46 − 44 2 46 2 2 / 8 = 0.25 𝑧# = = = = 0.25 𝑠 8 8 32 12 ‒12 / 8 = ‒1.50 © 2024 Cengage Group. All Rights Reserved. 4.5 Measuring Distribution Shape and Outliers Detection Detecting Outliers with z-scores An outlier is an unusually small or unusually large value in a data set. Care should be taken when handling outliers, as they might be: Ø an incorrectly recorded data value Ø a data value that was incorrectly included in the data set Ø a correctly recorded data value that belongs in the data set A data value with a z-score less than –3 or greater than +3 might be considered an outlier. For this example, refer to the starting monthly salary for the 12 business graduates, with mean, 𝑥̅ = 5940, and standard deviation, 𝑠 = 165.65. The smallest value in the data set, 5710, has 𝑧 = 5710 − 5940 ⁄156.65 = − 1.39, and the largest value in the data set, 6325, has 𝑧 = 6325 − 5940 ⁄156.65 = 2.32. Because all the values are within three standard deviations of the mean (z-scores within ±3), we conclude that there is no evidence of outliers. © 2024 Cengage Group. All Rights Reserved. 4.5 Measuring Distribution Shape and Outliers Detection Alternative Method for Detecting Outliers Another approach to identifying outliers is based upon the values of the first and third quartiles (𝑄) and 𝑄* ) and the interquartile range (IQR). For this example, refer to the starting monthly salary for the 12 business graduates. To use this method, we first compute the following lower and upper limits: Ø Lower Limit = 𝑄) − 1.5 𝐼𝑄𝑅 = 5857.5 − 1.5 167.5 = 5606.25 Ø Upper Limit = 𝑄* + 1.5 𝐼𝑄𝑅 = 6025 + 1.5 167.5 = 6276.25 Looking at the starting monthly salary for the 12 business graduates, we see that: Ø There are no starting salaries lower than the Lower Limit = 5606.25. Ø There is one starting salary, 6325, that is greater than the Upper Limit = 6276.25. Thus, using this alternate approach, 6325 is considered to be an outlier. © 2024 Cengage Group. All Rights Reserved.