Stat PDF
Document Details
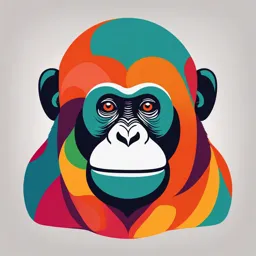
Uploaded by InterestingRetinalite868
Indiana University
Tags
Related
- BA311 Prelim Reviewer PDF
- Business Statistics: A First Course (5th Edition) Chapter 3 - Numerical Descriptive Measures PDF
- M9 - Ch01 Statistics Fundamentals PDF
- Business Statistics Slides PDF
- Topic 2b Descriptive Stats Grouped Data (Student Notes) PDF
- Lecture 3 Descriptive Stats Tabular Graphical PDF
Summary
This document provides an introduction to statistical analysis for business and economics. It covers topics such as data, displaying and calculating descriptive statistics, probabilities, and sampling distributions.
Full Transcript
Chapter 1: Statistics and Data This Class is Useful Because… Other classes use the tools from this class: Econ-E371, Bus-G492, Bus-K303, Bus-M303, Bus-K327, Bus-M346, Bus-G304, Bus-G350, Econ-E471 CFA examination: quantitative methods Abundance of statistical termi...
Chapter 1: Statistics and Data This Class is Useful Because… Other classes use the tools from this class: Econ-E371, Bus-G492, Bus-K303, Bus-M303, Bus-K327, Bus-M346, Bus-G304, Bus-G350, Econ-E471 CFA examination: quantitative methods Abundance of statistical terminology in media: Simple: %, mean, median, distribution Sophisticated: https://www.youtube.com/watch?v=3_aIR- NjYSY&t=44s Abundance of data Need to summarize them for analysis Abundance of statistical results in research S Need to know why these results hold and their limitations, and make correct conclusions Topics Statistics the mathematical science that deals with the collection, analysis, and presentation of data, which can then be used as a basis for inference and induction Topics of our course: Data Displaying and Calculating Descriptive Statistics Introduction to Probabilities Discrete and Continuous Probability Distributions Sampling and Sampling Distributions Confidence Intervals Hypothesis Testing - Regression Analysis An Introduction to Statistical Analysis for Business and Economics Classifications of Data Descriptive and Inferential Statistics Reading: Chapter 1 ~ · O * I Data Definitions Data values assigned to observations or measurements All the data collected in a particular study are referred to as the data set for the study Information data that are transformed into useful facts that can be used for a specific purpose, such as making a decision … it is more than just data Data Raw facts or measurements of interest New and Used Passenger Cars Imported in the US, by country of origin Each individual value is considered a data point Country 1988 2007 Japan 2,123,051 2,300,913 Germany 264,249 466,458 Italy 6,053 5,650 France 15,990 1,746 Source: World Almanac and Book of Facts (2009) Information Analyzing the data can provide information for decision making New and Used Passenger Cars Imported in the US, by country of origin Country 1988 2007 % Change Japan 2,123,051 2,300,913 8.38 Germany 264,249 466,458 76.52 Italy 6,053 5,650 -6.66 France 15,990 1,746 -89.08 Did the imports from country X increase (decrease)? Elements, Variables, and Observations (sales I , Elements are the entities on which data are collected night A variable is a characteristic of interest for the elements The set of measurements obtained for a particular element is called an observation A data set with n elements contains n observations : Elements : plane types variable : Too speed Observation : Boeing (17 the seeed Data Tables - Example Element Variables Sale Customer CC# Item Cost Gender 1 Nathan 1234 Stats Book 130.00 Male 2 Susan 5555 Unscented Lotion 20.00 Female 3 Andrew 8989 Snicker’s Bar 2.00 Male 4 Patrick 8734 Toothpaste 2.99 Male … … … … … … … … … … … … 10,000 Josh 2468 Zinc Supplement 11.00 Male Observation Data Set The Sources of Data Primary data Secondary data Definition: Definition: data that you have collected data collected by someone else for your own use Collection Methods: Advantages: Direct Observations Readily available Experiments more Less expensive to collect extensive Surveys Advantages: Disadvantages: Collected by the person or No control over how the data was organization who uses the data collected Less reliable unless collected and Disadvantages: recorded accurately Can be expensive and time- consuming to gather Types of Data Qualitative Data: Quantitative Data: Classified by descriptive terms Described by numerical values (labels or names used to identify (how many or how much) an attribute of elements) 1. Counted Examples: Examples: Number of Children Marital Status Defects per hour Political Party (Counted items) Eye Color (Defined categories) 2. Measured Examples: Weight words Voltage (Measured characteristics) numbers Classifying Data by Level of Measurement Types of Data Qualitative Quantitative Nominal Ordinal Interval Ratio Classifying Data by Level of Measurement The Four Levels of Data Measurement: A Summary Level Description Example Nominal Arbitrary labels for data Eye Color, Zip Codes gender No ranking allowed (19808, 76137) Ordinal Ranking allowed Education level No measurable meaning (Master’s degree, to the number differences doctorate degree) , height Interval Meaningful differences Calendar& year No true zero point (2009, 2010) (zero does not mean absence) Ratio Meaningful differences & Income True zero point ($48,000, $0) (zero means absence) Time Series vs. Cross-Sectional Data Time Series Data Values that correspond to specific measurements taken over a range of time periods Data can include hourly, daily, weekly, monthly, quarterly, or annual observations · same subject and variable measured over time Cross Section Data Values collected from a number of subjects during a single time period Subjects might include individuals, households, firms, industries, regions, countries, etc, many subjects measured at one point in time Time Series vs. Cross-Sectional Data Time Series Graph of U.S. & Unemployment Rates, 2006–2010 rates across us 5 years in Cross-Sectional Graph & of 2010 Unemployment Rates rats across countries in "year Time Series vs. Cross-Sectional Data New and Used Passenger Cars Imported in the US, by country of origin Country 1988 2007 Time Japan 2,123,051 2,300,913 Series Data Germany 264,249 466,458 Italy 6,053 5,650 France 15,990 1,746 Cross- Sectional Data Source: World Almanac and Book of Facts (2009) Descriptive vs. Inferential Statistics Descriptive statistics Collecting, summarizing, and displaying data (reported based on observations) - Averages (mean , median , mode) - visual statistics Inferential statistics Making claims or conclusions about the population based on a sample of data - requires descriptive stats do be good Recall the structure of our course… Population vs. Sample Population Represents all possible subjects that are of interest in a particular study Sample Refers to a portion of the population that is representative of the Every ju population from which - Student it was selected 2 Student from every Class Parameter vs. Statistic Parameter – a described characteristic about a population pop Statistic – a described characteristic about a sample Sang Population Sample Values calculated using Values computed from population data are sample data are called called parameters statistics The Need for Sampling Reasons for sampling from the population: Too expensive to gather information on the entire #) population I Too time-consuming to gather information on the entire population Often impossible to gather information on the entire population Inferential Statistics described Characteristic & Sample statistic is calculated from the sample data and is used to make inferences about the unknown population parameter described characteristic Observed Estimated population sample parameter (unknown, but can statistic Inference be estimated from sample (known) & evidence) -made atter taking tons of Example: sample stats and making estimations A statistics professor asked students in the class about their ages. On the basis of this information, the professor states that the average age of ALL the students in the university is 24 years. Practice Time Identify the type of data (quantitative/qualitative): 1. The average monthly rainfall in Bloomington Quan 2. The education level of survey respondents (High School, Bachelor’s Degree, Master’s Degree) Qual (0) 3. The marital status (Single, Married, Divorced) Qual (0) 4. The ages of the respondents in the survey Quan 5. iPhone price Quar 6. The number on the mailbox in the post office Qual (NY Can you identify the level of measurement for the above data? Practice Time The Department of Transportation of a city has noted that on the average there are 17 accidents per day. The average number of accidents is an example of descriptive or inferential statistic? Based on a survey, it was concluded that households with children under the age of 18 are more likely to have access to the Internet than family households with no children. Is it an example of descriptive or inferential statistic? Practice Time The table represents the results from a survey that collected annual household income in 2012. What type of data was used to construct this table - time series or cross-sectional data? Household Income ($000) # of Households Under $30 67 $30 to under $40 111 $40 to under $50 125 $50 to under $60 21 $60 to under $70 38 Over $70 40 Chapter 2: Displaying Descriptive Statistics Displaying Descriptive Statistics Displaying Quantitative Data Single Variable Displaying Qualitative Data Displaying Two Variables (discuss in Chapter 3) Reading: Chapter 2 (Sections 2.1 – 2.3) Skip Polygons and Pareto Charts Optional Reading: An Economist's Guide to Visualizing Data. Jonathan A. Schwabish. The Journal of Economic Perspectives, Vol. 28, No. 1 (Winter 2014), pp. 209-233 (posted on Canvas) Displaying Quantitative Data Recall the types of data: Qualitative and Quantitative Summarizing Quantitative Data Frequency Distribution Relative Frequency Distribution Tabular Cumulative Relative Frequency Distribution Histogram Graph Frequency Distribution A frequency distribution shows the number of data observations that fall into specific intervals (classes) - Example: Number of iPads sold per day cant Hell much class descriptive Relative Frequency Distributions Relative frequency distribution displays the proportion of observations in each class relative to the total number of observations Shows the fraction of observations in each class Found by dividing each frequency by the total number of observations /5 Fractions in a relative frequency distribution add up to 1 - = relative Relative Frequency Distributions Example: I Two iPads were sold on 28% of the days Cumulative Relative Frequency Distributions Cumulative relative frequency distribution totals the proportion of observations that fall below the upper limit of each class Shows the accumulated proportion as class values vary from low to high Cumulative relative frequency for the highest class is equal to 1 Cumulative Relative Frequency Distributions 3 Example: > - - = = Cuf = CofofC a if of 3 923 Three iPads or less were sold on 80% of the business days Histogram to Graph a Frequency Distribution Histogram is a graph showing the number or % of observations in each class It is a graphical representation of a frequency - distribution or the relative frequency distribution Classes of a variable of interest are placed on the horizontal axis things you're studying A rectangle is drawn above each class interval with its height corresponding to the frequency or relative frequency Histogram to Graph a Frequency Distribution ↑ A histogram for the iPad example: 16 14 Number of Days 12 10 8 6 4 2 0 0 1 2 3 4 5 Number of iPads Sold Per Day Excel Exercise >> Discrete vs. Continuous Data Discrete data are typically represented by integer numbers based on observations that can be counted (how many) take on whole numbers such as 0, 1, 2, 3 - ex : Plopie , buildings , iPads Continuous data are values that can take on any real numbers, including numbers that contain decimal points based on observations that can be measured (how much) take on any numbers such as 1, 3.1, 5.07, 4.941, etc. - ex : Money , time · opportunity to have decimals Discrete vs. Continuous Data Examples of Discrete data Number of children per family Number of cars listed per insurance policy Vacation days per month Examples of Continuous data Time required to read Chapter 2 Thickness of paint applied to a car body Person's height - Frequency Distribution Using Grouped Quantitative Data Ideally, the number of classes in a frequency distribution should be between 4 and 20 Some data sets, particularly those with continuous data, require several values to be grouped together in a single class This grouping prevents having too many classes in the frequency distribution, which can make it difficult to detect patterns Class Width There are methods to determine the number of classes k in a frequency distribution. But they are just a recommendation. You can always adjust! ↑ - Once k is known, the width of each class can be found as: Maximum data value Minimum data value Estimated class width = · The width is the range of numbers to put into each class Round this estimate to a useful whole number that makes the frequency distribution more readable Class Width There is no one correct answer for the class width The goal is to create a histogram to clearly and usefully show the pattern in the data Often there is more than one acceptable way to accomplish this Class Boundaries Class boundaries represent the minimum and maximum values for each class Choose class boundaries that are easy to read: 3.21 to less than 6.21 minutes vs. 3 to less than 6 minutes 6.21 to less than 9.21 minutes 6 to less than 9 minutes Class Frequencies Find class frequencies by counting and recording the number of observations in each class: Each class is represented by a range of values Example: Excel Exercise >> The Consequences of Too Few/Many Classes Wide classes results in few class intervals Can obscure important patterns Gives a “blocky” distribution graph Tells little about the distribution shape Too many narrow classes in a histogram also has consequences Results in a “jagged” histogram Some classes may be empty Displaying Qualitative Data Qualitative data are values that are categorical Can be nominal or ordinal measurement level Describe a characteristic, such as gender or level of education Summarizing Qualitative Data Frequency Distribution i Tabular Relative Frequency Distribution Bar and Pie Charts Graphs Frequency Distributions Frequency distribution: Indicates the number of occurrences of various categories Techniques are similar to frequency distributions with quantitative data We can construct a relative frequency distribution (same idea as for the quantitative data) Cumulative relative frequency distribution does not really make sense (specifically for nominal data) Bar Charts Can be arranged in a vertical or horizontal orientation On one axis (usually, horizontal), we specify the labels that are used for each of the classes A frequency or relative frequency scale can be used for the other axis (usually, vertical) Using a bar of fixed width drawn above each class label, we extend the height appropriately Bar Charts Vertical bar chart Horizontal bar chart Excel Exercise >> Pie Charts Pie charts are a tool for comparing proportions for qualitative data Each segment of the pie represents the relative frequency of one category All categories in the data set must be included in the pie Use a pie chart to compare the relative sizes of all possible categories Bar charts are more useful when you want to highlight the actual data values Pie Charts Example: Excel Exercise >> Excel Time: Exercise 2.7 A major U.S. airline records the number of no-shows on a flight that operates each day from Philadelphia to Paris. A no- show is a passenger who purchases a ticket but fails to arrive at the gate at time of departure. The data for no-show during 70 flights can be found in the Excel file no-shows.xlsx (Excel Files Ch 02 on Canvas) a. Construct a frequency distribution for these data. b. Using the results from part a, calculate the relative fre- quencies for each class. c. Using the results from parts a and b, calculate the cumulative relative frequencies for each class. d. Construct a histogram for these data. Back Data Analysis > Histogram > OK Note: If you do not see the Data Analysis option under Data, you must add in this option: see the next three slides If you have a frequency distribution or a relative frequency distribution Select the classes and the frequencies and use Insert > Charts > Insert Column or Bar Chart > 2-D Column and choose the graph on the top left Excel Time: Data Analysis Tool 1. In Excel, click on the File tab 2. Click Options shown in the drop- down menu (may be hidden in the More… menu). This will open Excel Options dialog box 3. Select Add-Ins in the left margin of the Excel Options dialog box Excel Time: Data Analysis Tool 4. Click on Go… at the bottom of the form 5. Check boxes for Analysis ToolPak and Analysis ToolPak - VBA in the popup menu and click OK Excel Time: Data Analysis Tool Select the Data tab. Click on Data Analysis on the right side of the application bar The Data Analysis pop-up menu should appear in the spreadsheet Excel Time: Constructing a Histogram 1 1. Select the Data tab, and click on Data 1 Analysis in the upper right corner 2. In the pop-up menu, select 2 Histogram and click OK… 2 Excel Time: Constructing a Histogram 3. In the Input Range, highlight the data Check «Labels» if you selected the data with the column name 4. In the Bin Range, highlight the bins (create if not already created before step 1) 3 7 5. For Output options, 4 select where you want to see the results 5 Specifying one cell indicates the upper left corner of the output 6. Choose “Chart 6 Output” option if you want the histogram to be displayed 7. Click OK Excel Time: Exercise 2.5 Excel file college_credit_card.xlsx (Excel Files Ch 02 on Canvas) contains the results of a survey that collected the current credit card balances for 36 undergraduate college students. a. Construct a frequency distribution for these data. b. Using the results from part a, calculate the relative fre- quencies for each class. c. Using the results from parts a and b, calculate the cumulative relative frequencies for each class. d. Construct a histogram for these data. Back > Excel Time: Exercise 3.42 (Modified) Excel file city_populations.xlsx ( ) lists 12 largest U.S. cities from the 2010 Census. Using the data in the file: 1. Determine descriptive statistics using Data Analysis add-in. 2. Using Excel functions, find descriptive statistics for this sample: mean, median, mode, range, variance, standard deviation. Provide units of measurement for these values. 3. Describe the shape of this distribution. 4. Additionally, find 60th and 85th percentile, 1st quartile and the coefficient of variation. Interpret calculated values. 5. Calculate the z-scores for the first and the last city in the table. 6. Are there any outliers in the sample? 7. What measure of central tendency would best describe this data? Back correlation coeff ) ↳ scatter dist.. has plats info and what rule empirical - - rel. F. dist. it tells US Ch4 : - - Cumulative rel I dist.. - Basics probability of - Charts (bar a pie) · complement - disctle v Continuous data · Mion - Scatter plots · intersection of events -Histograms · conditional probabilities # full multiplication Discrete · sendem independent) dependent Expect variables · events ~ Disciple probability dist -. defining function of a probability · · calculations discrete prob, dist (au). interpreting - mean and SDs of discre define · · · light or calculations pd's · reles · No excel - Binomial random variables · representation as a table , graphe, or · Similar to HW Q's and distributions (characteristies , formula examples - mean , variance , and SD & binomial random variables - (*./ [ 1 1100) : CV Y : Sonare weal - shows how much spread is present in data measures of variability - Gender is an example... of Normal data Qualitative data descriptive to data uses terms classify - of interest - collecting , summarizing , displaying data... descriptive stats Chapter 6: Continuous Probability Distributions (Normal Dist.) Continuous Probability Distributions Continuous Random Variables Normal Probability Distribution Uniform Probability Distribution Don’t discuss Exponential Probability Distribution Reading: Chapter 6 (Sections 6.1-6.2) Probability Distributions Probability Distributions Discrete Continuous Probability Probability Distributions Distributions Continuous Probability Distributions A continuous random variable can assume any value in an interval on the real line or in a collection of intervals It is not possible to talk about the probability of the random variable assuming a particular value Because there is an infinite number of possible values, the probability of one specific value occurring is theoretically equal to zero P(x = x0) = 0 Instead, we talk about the probability of the random variable assuming a value within a given interval P(x > x1), P(x x1), P(x < x2), P(x x2) < < P(x1 x x2) Probability Density Function Probability density function f(x) (people also call it pdf) is used to specify the probability of the random variable falling within a particular range of values, as opposed to taking on any one value f(x) is used to calculate probabilities BUT the value of f(x) is not a probability per se The probability that x takes on a value between some lower value x1 and some higher value x2 can be found by computing the integral of the probability density function f(x) over the interval from x1 to x2 Graphically, it is equivalent to computing the area under the graph of f(x) over the interval from x1 to x2 Probability Density Function The probability of the random variable assuming a value within some given interval from x1 to x2 is defined to be the area under the graph of the probability density function f(x) between x1 and x2 Uniform f (x) Exponential f (x) Normal f (x) x1 x2 x x1 x2 x x1 x2 x Note: All area under the graph of f(x) is equal to 1 Cumulative Probability Cumulative probability at a given point x0 is the probability that a random variable x takes a value less than or equal to x0 P(x x0 ) Graphically, cumulative probability is the area under the graph of f(x) on the left of x0: Normal f (x) e(X(X0) x x0 Useful Notes The probability of a random variable falling within a particular range of values can always be expressed in terms of cumulative probabilities E.g., P(x > x1) can be expressed as a function of P(x x1) It is useful because most software allow computing only cumulative probabilities For a continuous random variable, inclusion of the boundary does not affect calculations of probability: For example, P(x x1) = P(x < x1) P( 1) = P(x = x1) + P(x < x1) = 0 + P(x < x1) Same holds for other types of intervals Normal Probability Distribution The normal probability distribution or normal distribution is the familiar bell-shaped distribution Most extensively used distribution Closely approximates the distribution for a wide range of random variables (heights and weight of newborns; scores on the SAT; advertising expenditure of firms; rate of return on an investment) Various ways to determine if the normal distribution is appropriate for a given applications For example, histogram In this chapter, we simply assume that it is known that the random variable is normally distributed Normal Probability Distribution < < = 0.5 < < = 0.5 f(x) x = mean save as median < < =1 Normal Probability Distribution 1. Bell-shaped and symmetric around its mean 2. The highest point on the normal curve is at the mean, which is also the median and the mode 3. The mean can be any numerical value 4. Asymptotic: tails get closer and closer to the horizontal axis but never touch it 5. Fully described by two parameters: mean and standard deviation 6. The standard deviation determines the width of the curve: larger values result in wider and flatter curves Ishape) 7. Probabilities are calculated as an area under the normal curve. The total area under the curve is 1 (0.5 to the left of the mean and 0.5 to the right) 8. Values near the mean, where the curve is the tallest, have a higher likelihood of occurring than values far from the mean, where the curve is shorter Normal Probability Distribution A mean ( ) and standard deviation ( ) completely describe distribution’s shape and location on a real line Changing shifts the Changing increases distribution left or right or decreases the spread n = M 5 = a f(x) f(x) x x > < & Normal Probability Distribution Example: Ages of employees in Industries A, B and C Industry A Industry B Industry C = 42 years = 36 years = 42 years = 5 years = 5 years = 8 years Standard Normal Probability Distribution Characteristics A random variable having a normal distribution with a mean of 0 and a standard deviation of 1 is said to have a standard normal probability distribution The letter z is designated for the standard normal random variable Relationship with Normal Distribution Any normally distributed variable x (with any mean and standard deviation) can be transformed into the standard normal distribution z Need to find a z-score for all values of x Converting to Standard Normal Distribution Normally distributed variable can be transformed into : = where: x = value of interest = the distribution’s mean # = the distribution’s standard deviation Recall: We can think of z as a measure of the number of standard deviations x is from The Standard Normal Distribution When a random variable x follows the normal distribution z-scores also follow a normal distribution with = and = or, in other words, follow the standard normal distribution z f(z) =1 =0 z Example The time customers spend on the phone for service follows the normal distribution with a mean of 12 minutes and a standard deviation of 3 minutes. What is the probability that the next customer who calls will spend 14 minutes or more on the phone? Solution Steps: 1. Determine what’s given 2. Determine what probability you need to find 3. Find z-scores for the numerical boundaries of the interval of interest and rewrite probability in terms of a z-score 4. Rewrite probability using a cumulative probability 5. Calculate the answer Example Given: = 12 and =3 Question: P(x > 14)? z-transformation: Find the z-score for x = 14: 14 12 = = = 0.6667 3 This says that x = 14 is 0.6667 standard deviations above the mean of 12 Note: the step of calculating z-scores for the boundary can be skipped if you have Excel to calculate probabilities Example =3 12 14 x 0 0.6667 z Note that the shape of distribution is the same, only the scale has changed Instead of original units (x), we expressed the problem in standardized units (z) Example Upper tail probability The area under the normal curve equals 1.0: 67 % that = 50 14 P(x > 14) = P(z > 0.6667) cumulative under = 1 – P( 0.6667) E probability Ar = 1 – 0.7475 that = 0.2525 25 % was mor call 1 – 0.7475 minutes then 14 = 0.2525 Note: Finding P( 0.6667) 0.7475 requires Excel 12 14 x 0 0.6667 z Example Lower tail probability Let’s go back to the original example and ask: What is the probability that the next customer who calls will spend 10 minutes or less on the phone? P( 10) = = 0.2525 = P( -0.6667) = 0.2525 10 12 x -0.6667 0 z Note: the distribution is symmetric around 0. So, if we know P(z know P(z -0.6667) or area on the left of -0.6667 Example Probability between two values What is the probability that the next customer who calls will spend between 10 and 13 minutes on the phone? Question: P x Convert x = 10 and x = 13 to z-scores: Probability = ? 10 12 = = = 0.6667 3 ? -0.6667 0 0.3333 z 13 12 = = = 0.3333 10 12 13 x 3 Example P x = P(- z M 0.6306 e = P( 0.3333) - P( -0.6667) -0.6667 0 0.3333 z 10 12 13 x = 0.6306- - – 0.2525 = 0.3781 - 0.2525 mun -0.6667 0 0.3333 z 10 12 13 x 0.3781 -0.6667 0 0.3333 z 10 12 13 x Inverse Probability Calculations A reverse exercise: Finding z or x value In our example, the time on the phone follows the normal distribution with = 12 and = 3. What is the wait time so that 95% of calls have a shorter wait time? X-12 - Find x0 value so that P(x x0) = 0.95? 3 1. Find the necessary z-score: the z value needed to include 95% of the area under the curve to the left of the z-score Note 1: This step requires Excel. In this example, z = 1.645. 0.05 Note 2: With Excel, this and the next 0.95 steps may be combined: x can be found without z value 0 ? z Inverse Probability Calculations A reverse exercise: Finding z or x value 2. Find x value that is 1.645 (or z) standard deviations above the mean: = = + 0.05 = 12 + 1.645 × 3 = 16.94 0.95 1 scor for a5% 0 1.645 z 12 16.94 x In words, 95% of calls have a wait time less than 16.94 min Excel Exercise >> Binomial Distribution Approximation The binomial distribution can be approximated by the normal distribution under some conditions In general, it is tedious to compute binomial probabilities Excel makes it easy BUT The normal distribution approximation of the binomial distribution is important when making inferences for the population proportion, p (we’ll see in later chapters) The normal distribution approximation can be used when: np nq When using the normal approximation, we set: = and = Binomial Distribution Approximation Example: Suppose that 15% of people are left-handed. What is the probability of finding 9 left-handed people in a random sample of 50 individuals? Exact probability: Calculated from the binomial distribution Using Excel, we obtain: P(x = 9, p = 0.15, n = 50) = 0.1230. Approximated probability: To approximate the binomial probability, we use the normal distribution with the mean and the SD as follows: = = 50 × 0.15 = 7.5 = = 50 × 0.15 × 0.85 = 2.525 We don’t cover the steps of approximation FYI... Approximated probability is 0.1319 Approximation results depend on whether np nq Revisiting the Empirical Rule For a normal distribution: ± 1 encloses about 68% of the data values ± 2 covers about 95% of the data values ± 3 covers about 99.7% of the data values f(x) 2 2 x 95% x -1 +1 3 3 68% x 99.7% Revisiting the Empirical Rule For a standard normal distribution: [-1; 1] encloses about 68% of the data values [-2; 2] covers about 95% of the data values [-3; 3] covers about 99.7% of the data values f(x) 2 2 0 x 1 1 95% x -1 0 1 3 3 68% 0 x 99.7% Excel Time: Exercise 6.8, I According to a recent survey by Smith Travel Research, the average daily rate for a luxury hotel in the United States is $237.22. Assume the daily rate follows a normal probability distribution with a standard deviation of $21.45. What is the probability that a randomly selected luxury hotel’s daily rate will be a. Less than $250 per night? b. More than $260? c. Between $210 and $240? Back