Research Methods Part 2 PDF
Document Details
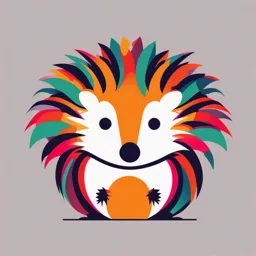
Uploaded by ResoluteAzurite8996
Tags
Related
- Week 2 - Research Methods Notes PDF
- Conducting Psychology Research PDF
- Experimental Research 2024 PDF
- HPCS4031 Research Methods in Psychology Lecture 4: Experimental Design (2) PDF
- HPCS4031 Research Methods in Psychology Lecture 4: Experimental Design (2) PDF
- Experimental Method in Psychology: Experimental Designs ch 7 PDF
Summary
This document covers the topic of research methods, focusing on comparison between and within subjects and discussing confounding variables. It explains the process and features of both between-subjects and within-subjects designs. The document also explores the concept of confounding variables, offering examples and how to minimize their impact on research.
Full Transcript
1 WEEK 7- HYPOTHESES AND VARIABLES HYPOTHESES AND VARIABLES Part 1: Comparing between- and within-subjects designs Process of between-subjects design: 1) Take a sample of participants from a population & they are assigned to a treatment conditions using an assignment process (random samp...
1 WEEK 7- HYPOTHESES AND VARIABLES HYPOTHESES AND VARIABLES Part 1: Comparing between- and within-subjects designs Process of between-subjects design: 1) Take a sample of participants from a population & they are assigned to a treatment conditions using an assignment process (random sampling for example). 2) Each participant only participates in one treatment condition in between subjects design. Features of between subjects design: ↬ Contains 2 or more groups of participants. ↬ Groups go through an intervention & the outcomes are compared between groups. ↬ The groups are also independent from one another. 2 ↬ e.g.: 3 diverse groups with different independent conditions= one group with no physical activity, one group running for 3 weeks & one group doing yoga for 3 weeks. ↬ Usually the average is taken from each group then you compare the independent groups to see which is the most effective. Advantages of between-subjects designs: Opportunity for very little contamination by extraneous factors (allows control of it). Reduced chance of boredom, fatigue, practice effects that can skew results (as participants are only participating in one treatment condition). Disadvantages of between-subjects designs: The required number of participants can be resource heavy ↬ Special populations-might be difficult to find these specific samples. Increased variance across groups ↬ Impossible to maintain homogeneity in age, gender, socio economic, educational levels, intelligence etc. across groups of participants ↬ Must demonstrate that changes in one variable are directly responsible for causing changes in the second variable Environmental influences ↬ E.g. testing one group in the morning and one group in the afternoon 3 Process of within-subjects design: 1) Take one sample of participants from a population. 2) Participants then participate in different conditions of the experiment & they might do so at different times. 3) They can also participate & do everything across the conditions all on one day & do them all at the same time. Features of within-subjects design: ↬ Only one group is participating in the study. ↬ Each individual starts with a baseline measure of the intended outcome. ↬ As the study goes on, subsequent measures are taken & compared to their baseline, ↬ Basically comparing the before & after results of the same group of people. Advantages of within-subjects designs 4 Requires relatively few participants Eliminates problems based on individual differences Reduces variance Increases the chances of detecting a treatment effect A within-subjects design: Has no individual differences between groups; there is only one group of participants. The group in one treatment is exactly the same as the group in every other treatment. Result: no individual differences between groups to confound the study Each subject appears in every treatment condition. Each subject serves as their own control. Disadvantages of within-subjects designs Each participant usually goes through a series of treatment conditions, often with each treatment administered at a different time. ↬ May lead to time-related factors that influence the subjects’ scores. ↬ Their situational & personal factors might differ from week to week & that time related factor then influences the scores. Participant attrition: some of the subjects who start the study will drop out before the study is completed. How do you choose between a between- or within-subjects design? It is often not easy to decide 5 Your research question or IVs may lead you to one or the other Both designs can be possible Between-subjects minimizes transfer and learning effects Less demanding on participants Randomisation more straightforward Within-subjects Fewer participants Cheaper? Minimise variation Part 2: Confounding variables Confounding variables: Extraneous variables that impact results= confounding variables. Factors other than the IV that may influence the DV So…factors other than drinking/not drinking coffee that may influence performance in a reading comprehension test Researchers must attempt to minimise the influence of confounding variable by designing an experiment where all conditions are the same, except the IV: Coffee vs. no coffee. 6 Confounding variables examples: Order effects Participant variability Social desirability effect Hawthorne effect Demand characteristics Evaluation apprehension 1) Order effects Must be careful of these in within-subjects designs Participants could get better with practice Participants could remember something from their first participation that influences their second participation Counterbalancing can control for order effects Half the group do condition 1 first; half do condition 2 first 2) Participant variability The extent to which participants are different can influence results Random allocation can help Matched pairs design can help- age & gender match 3) Social desirability effect People changing their behaviour because they have a desire to be liked by other people. 7 People might say they drink more coffee than they do to be liked or because it is trendy to do so. 4) Hawthorne effect When people act differently to how they normally do because they are being watched. Change in attention focus on the reading comprehension test because the researcher is watching. ↬ Hence, their results are different to when they are not being watched. Cease checking their phones. 5) Demand characteristics Participants work out how they are supposed to act (according to the demands of the researchers/experiment) Demand characteristics might lead to a participant expectancy effect occurring ↬ Coffee scenario: Coffee condition vs Placebo condition- participants might be given a drink that looks like coffee, tastes like coffee but its decaffeinated. ↬ So they think they are drinking coffee, so expectancy effect influences their performance on their task (they expect to do better in the reading comprehension since they drank coffee) Placebo effect Given participants a cup of coffee that they think is caffeinated Participant belief that they are having caffeinated coffee leads to greater focus on reading comprehension test 8 6) Evaluation apprehension Anxiety about being evaluated changes behaviour Spotlight effect: ↬ E.g. Nervousness on reading comprehension test. Maturation: ↬ Getting better on the second or third question in reading comprehension test. ↬ Counterbalancing it= researcher might mix up the questions in the reading comprehension test they are giving. Information contamination: ↬ Outside information affects performance ↬ E.g. might know details of the text already from previous experience Part 3: Controlled variables The influence of coffee on reading comprehension? You can control variables like Exercise how much exercise was done in the day? Or you could specify no exercise on the morning of test day But it is difficult! Randomised Randomly allocate participants to each condition of the experiment 9 Coffee group or no coffee group Here you are controlling participant variability E.g. limiting influence that exercise could have by limiting the chances that group differences will affect the results. Placebo-controlled One group gets a placebo: Coffee group No coffee group Placebo (decaf coffee) Here you are controlling the placebo effect (participant expectancy effect) Double-blind One group gets a placebo Coffee group No coffee group Placebo (decaf coffee) BUT the researcher and participant are blind to who is in what group Here you are controlling the placebo effect and researcher bias Part 4: Hypotheses Research questions and hypotheses A research question is born out of your interest, your curiosity, your review of the literature. 10 So, for our coffee study ↬ Does drinking coffee influence performance on a reading comprehension test? Hypothesis is a statement that describes or explains a relationship between variables. Assumption/idea built on your understanding of evidence and requires you to prove it via relevant facts and examples. The hypothesis is describing/explaining the expected relationship between the IVs and DV Variables and hypotheses: Hypothesis is drawn from our review of literature in the area of caffeine and cognitive skills. So, for our coffee study Hypothesis- Participants that drink coffee before a reading comprehension test will have better performance on that reading comprehension test compared to participants that did not have coffee before the reading comprehension test. The hypothesis is describing/explaining the expected relationship between variables. A good hypothesis Logical ↬ the logical conclusion of a logical argument-that you can come up with through your readings & research of existing literature. Testable ↬ all the variables, events, and individuals can be defined, observed, and measured Refutable ↬ can be demonstrated to be false- it could be wrong in making a prediction about expected relationship between our variables. Positive ↬ must make a positive statement about the existence of something. 11 The null hypothesis and the alternative hypothesis: Null hypothesis Proposes that no significant effect or relationship between the variables Hypothesis of no difference Coffee will have no effect on performance on a reading comprehension test Alternative hypothesis (non-directional) ↬ Coffee will have an effect on performance on a reading comprehension test If we find that it does, then this will nullify the null hypothesis Alternative hypothesis (directional) ↬ Participants that drink coffee before a reading comprehension test will have better performance on that reading comprehension test compared to participants that did not have coffee before the comprehension test. WEEK 9- ETHICS IN RESEARCH AND DIVERSE POPULATIONS What are research ethics? So why do we need ethical guidelines? Set rules the governs expectations of behaviour. More than simply ‘doing the right thing’ Ethical conduct in research – acting with integrity, with enduring respect for one’s fellow creatures. 12 Human research: research conducted with or about people, or their data, or tissue. Human research has led to MANY breakthroughs in science, medicine, and psychology. ↬ Vaccines ↬ Cancer treatment ↬ Understanding effects of starvation Impacts of authority on behaviour ↬ Ethical considerations have sometimes fallen by the wayside in the pursuit of knowledge. ↬ Up until the end of WW2, researchers predominantly established their own ethical standards & safeguards for human participants in research. ↬ It was assumed that researchers guided by their own moral & compass, would protect their participants from harm. 13 ↬ However, not all researchers were committed to the ethical treatment of human participants. ↬ The shift for this started after the uncovering of the very brutal experiments that were performed on prisoners in Nazi concentration camps during WW2. ↬ Belmont Report was created which summarises the basic ethical principles identified by the National Commission & these are used as the foundation upon which federal regulation for protecting human participants are based, even to this day. Ethical considerations ↬ Ethical considerations in research are a set of principles that guide your research designs and practices. Scientists and researchers must always adhere to a certain code of conduct when collecting data from people. ↬ The goals of human research often include understanding real-life phenomena, studying effective treatments, investigating behaviours, and improving lives in 14 other ways. What you decide to research and how you conduct that research involve key ethical considerations. These considerations work to: protect the rights of research participants enhance research validity maintain scientific integrity National Health and Medical Research Committee: Ethical Conduct in Human Research (NHMRC) Justice – fair treatment of participants and distribution of benefits. Beneficence – the benefits should outweigh the risks. Respect – privacy, confidentiality, and consent. Research merit and integrity – honesty, transparency and justified. ↬ The creation of the research itself, the initial conception of an idea right through to the conducting of research. ↬ Also, how those research results are disseminated or how the results are given out to the community. Beneficence Risks: ↬ Physical= Injury, illness, pain. - E.g.: a part of research is drawing blood- what will the potential harm & how we might manage or minimise that risk. 15 ↬ Social= Damage to networks/relationships, stigmatisation, prejudice. - Social media posts or something like that might be damaging to the individual’s social networks or their relationships. - Social stigma,prephaps if it is revealed against a client's will that they have a particular diagnosis. ↬ Psychological=Feelings of worthlessness, distress, guilt, anger, fear, devaluation of personal worth. ↬ Economic= Direct/indirect ↬ Legal=Prosecution of criminal conduct ↬ Discomfort=Side effects from medication, data collection ↬ Inconvenience Time Benefits: Direct benefits (to the participant) Educational- people can learn something about themselves/receive psychoeducation. Acquisitional Treatment- take part in a controlled trial where they receive a treatment or a particular type of therapy. 16 Monetary- monetary compensation or gift vouchers ↬ General community Increased scientific understanding- of a particular phenomenon, diagnosis, behaviour, mental health issue. Improved applied procedure Justice: Procedural ↬ Fair treatment in the recruitment of participants – The exclusion and inclusion of categories of research participants must be fair – The inclusion and/or exclusion of participants based on age, ethnicity, gender, or other criteria must be justified on scientific grounds= there needs to be a reason you are choosing to exclude a certain group of people. Distributive ↬ The fair distribution of the benefits and burdens of research= no unfair burden. – There is fair distribution of and fair access to the benefits of research – There is no unfair burden of participation in research on groups Respect Privacy and confidentiality 17 Confidentiality-ensures that information obtained from a research participant will be kept secret & private. ↬ Benefits both the participants & researcher. – Ensured through anonymity – Includes: ↬ Attitudes and opinions ↬ Measures of performance ↬ Demographic characteristics ↬ Any of those items can be considered private & personal by some people & it is reasonable if some participants would not want this information to be made public. Informed consent Participants must be informed of all available information about the study so they can make a rational decision to participate (or not). ↬ Information ↬ Understanding ↬ Voluntary-decide whether they wish to take part. ↬ It is difficult to provide participants with complete information about the research study prior to their participation. ↬ They might be blinded to the actual purpose of research, which makes results less valid. ↬ So, it is important that at the end of the study that the participants are fully debriefed or informed about the full reason for the study. 18 Research merit and integrity “Unless proposed research has merit, and the researchers who are to carry Merit out the research have integrity, the involvement of human participants in the Justifiable by its potential benefit research cannot be ethically justifiable.” Uses methods appropriate for achieving the research aims Based on a thorough study of the relevant existing literature Conducted by persons with appropriate competence Uses appropriate facilities and resources Designed to ensure that respect for participants is not compromised Searching for knowledge and understanding Following recognised principles of research conduct Conducting research honestly Disseminating and communicating results, whether favourable or unfavourable, in ways that permit scrutiny and contribute to public knowledge and understanding. 19 ↬ Some of the ethical considerations that we need to take part in or need to consider are things like cultural sensitivities, different social norms & accepted practises & different cultural groups. ↬ So, it is important that at the end of the study that the participants are fully debriefed or informed about the full reason for the study. ↬ As a part of the ethics process or the research design process, we need to think about things such as potential misunderstandings. Aboriginal and Torres Strait Islander Peoples- Respect for & valuing of cultural & language diversity. Core Principles Reciprocity Respect Equality Responsibility Survival and protection Spirit and integrity 20 WEEK 10: CORRELATIONAL RESEARCH STRAREGY Introduction to Correlational Research Part 1 - The relationship between the two variables X and Y. - Could be done when variables cannot always be manipulated (where u live or income), so it could describe but not explain. Comparing research strategies 1. Correlational Research: - Establishes a relationship between two variables - E.g., “What is the relationship between household income & age?” - Does not determine that one variable cause another 21 “Correlation does not equal causation!” ↬Age of Miss America & the number of murders that involves steam/hot objects throughout the years. ↬ These variables are correlated but not casually influencing one another. Hence, we can expect that the age of Miss America to have anything to do with the murders. ↬ Known as a spurious correlation= just because two variables' correlates do not mean that they causally influence each other. 2. Experimental Research ↬ The experimenter assigns the participants to the independent group. Demonstrates a cause-and-effect relationship between variables. The researcher actively manipulates a variable while measuring another variable E.g., “Does Drug X improve headache pain?” 22 ↬ Actively manipulated who is going in which groups and the measuring the outcome that, making this experimental research. ↬ More confident in finding the difference between groups. 3. Differential Research ↬ Involves two groups of scores and focuses on the difference between the groups. ↬ Not assigning people to groups, they come assigned as groups. E.g., Average household income for different suburbs ↬ Not assigning people to suburb they live in, participants just come assigned to a suburb and then we want to compare the average household income across those suburbs. - Does not determine the cause-and-effect relationship - Correlational research is about individuals, where differential research is about groups! The Data and Statistical Analysis for Correlational Studies: Part 2 23 24 A correlation coefficient measures and describes the relationship between two variables: 1. Direction 2. Form 3. Consistency or strength 1. Direction can be positive or negative. Ask yourself: what happens to Y as X increases? ↬ Here, Y increases as X increases (same direction). ↬ So it’s a positive correlation. 25 2. Form is about the shape of the relationship ↬ Linear relationship = straight line. - For linear relationships, we calculate a Pearson Correlation Coefficient. - Pearson’s r describes and measures the linear relationship when both variables are numeric scores from interval or ratio scales. - E.g.: age correlated with height=both numerical. ↬ Monotonic relationship = still a one-directional trend, but not a straight line. Spearman’s Rank-Order Correlation ↬ Measures the relationship between the ranked X and ranked Y scores. 26 ↬ Organised al of the X values into the right order and the Y values in their rank order. We could compare how similar the order of those list is. Whatever type of Correlation Coefficient you use: ↬ The sign (+/–) indicates the direction of the relationship. ↬ The numerical value (0.0–1.0) indicates the strength or consistency of the relationship. ↬ You can assess the form by visualising the data with a scatterplot. This will help guide you in choosing which correlation coefficient to use. 27 ↬A) Strong Positive Correlation: If a straight line is drawn through those points, it would fit them tightly around the line= strong relationship. ↬B) Weak Negative Correlation: The fit of those lines is not good, those points are more spread out = weak relationship. ↬ C) Prefect correlation: R has the value of one, so the fit is prefect. Every single dot is on the line= negative correlation. ↬ D) No correlation= no relationship between X and Y. Evaluating and reporting a correlation By squaring r, we get the percentage of variability in one variable that is explained by its relationship with the other variable. R2 = Coefficient of determination. Higher is better! 28 ↬ Relationship between the R value (correlation coefficient) and the coefficient of determination. ↬For a very large correlation (greater than 0.5), we are explaining about 25% of the variability in the day. Evaluating and reporting a correlation What about the statistical significance of the correlation? - In addition to the strength of a correlation, we also need to evaluate whether r value could have been produced by random variation. ↬ We need a p-value -With a small sample, it is possible to obtain what appears to be a very strong correlation when, in fact, there is no relationship between the two variables. -Increasing the sample size makes it more likely that a correlation represents a real relationship ↬ More confident about the line we draw and the correlation coefficient we produce is likely to represent real relationship between those two variables. 29 What about when your variables are non-numerical? e.g., How does suburb relate to household income? Evaluating relationships with non-numeric scores from nominal variables One numeric and one non-numeric score: - Use the non-numerical variable to organize the scores into separate groups (i.e., categories) - If you have just two categories (e.g., Penrith & Parramatta) — numerically code the categories as 0 and 1 ↬ Calculate the Pearson correlation ► point-biserial correlation e.g., How does suburb relate to household income? Evaluating relationships with non-numeric scores from nominal variables If both variables are non-numeric: - Organise your data into a MATRIX (contingency table). ↬ Make a count data for the number of people who support Parramatta Eels and those who support the Penrith Panthers as function of whether they live in Penrith or Parramatta. 30 - Use a chi-square test to know whether the counts in each cell are different to what we would expect by chance. ↬ Inspecting whether the frequencies in these cells are different from the frequencies we would expect by chance. ↬ E.g.: If we had 100 people & simply asked them, do you support the eels or the panthers? 50% to say the eels & 50% to say the panthers. So, we're interested here to see whether the counts we get in these cells are different from those 50% values. e.g., How does suburb relate to football team? Correlation in the wild (applications): Part 3 Used for making predictions: -The correlational strategy is all about demonstrating a relationship between two variables: If you know the relationships between variables, you can use knowledge about one variable to help predict or explain the second variable. -One predictor variable - one criterion variable (being explained) e.g., the relationship between peak daily temperature and bushfire severity. 31 ↬ Linear Regression: to find the equation that produces the most accurate predictions of Y (the criterion variable) for each value of X (the predictor variable). ↬ Can be used for multiple variables, with many predictors. E.g.: inspect how bush fire severity is varying or predicted by daily temperature & add a term in the model like region or state. Used for inspecting validity & reliability ↬ Validity and reliability are important aspects of research -Are you really measuring what you think you’re measuring? -How reliable is your test? Will I get the same results on your test if I do it again next week? Next month? ↬ Correlation often helps us answer these questions. For example: - Correlate this score with another, established measure (e.g., correlate a newly developed memory scale with scores on an existing, well-validated memory test) = strong correlation is found. -Correlate scores across occasions (test-retest reliability) or raters (inter-rater reliability). ↬ Test-retest = a follow up test, people are invited to research then are asked to return 6 months later for a follow-up test. So, when you correlate individuals scores from time one to time two, we should expect to find a strong correlation. ↬ Raters= Rating something, so it does not matter who does the rating, they should always produce very similar results. Strengths & Weaknesses of Correlational Research: Part 4 32 Correlation: The good and the bad Strengths Weaknesses Describes relationships between Cannot assess causality variables Nonintrusive — gets at natural Low internal validity behaviours High external validity Directionality problem ↬We believe that there might be a third variable that is driving both variables that we are measuring. ↬ X=temperature as it goes up, has a casual influence over ice cream sales and crime rates. So, temperature is moderating both. ↬ No causal link of the relationship between ice cream sales and crime rates. ↬ Difficulty in research, where we will not see the influence of a third variable unless we know to actively look for it. 33 Relationships with More Than Two Variables - Correlational research is bi-directional. Multiple regression: a statistical procedure for studying multivariate relationships – Can be used to examine the relationship between two specific variables while controlling the influence of other, potentially confounding variables. ↬ Suburbs and household income but with controlling the age. ↬ It could be that age explains part of the relationship. ↬ People who are in the mid/late stage of their career tend to live in one suburb and people who are in the early stage of their career tend to live in another suburb. Multiple regression= examine unique contributions of that a given variable makes for some variable. – Predictor variables only predict – Relationships can be described—not explained 34 WEEK 11- DESCRIPTIVE STATISTICS Part 1: Introduction, descriptive statistics for ratio and interval data, and frequency distributions Two categories of statistic: Descriptive statistics Inferential statistics Methods that help researchers Methods that use the results obtained organise and summarise data from samples to help make generalisations about populations Help simplify and interpret the Help the researcher answer the inferential statistics general questions that initiated the research. Population vs. Sample Population The collection of all things having one or more specified characteristics A single thing having the specified characteristic/s is called an element of the population. Elements can be people, objects, events, or observations based on measurable characteristics of people, objects, or events (e.g., dieting behaviour, weight, gender, cortisol levels). Sample It is rarely possible to study all the elements in a population (& costly) 35 Hence, research in psychology usually involves the study of a sample drawn from the population of interest A subset of elements within a population Researchers make generalisations from what they observe in the sample elements to all the elements in the population Whether the sample is a sound basis to inform generalisations to the population depends on how the sample was drawn from the population (i.e., the sampling method – see Validity lecture) Functions of descriptive statistic: Describe the characteristics of the sample – both numerically and graphically. Provide an indication of where a sample tends to sit (central tendency) and how diverse it is on any given characteristic/element. Can provide suggestion of whether the sample is representative of the population. 36 Descriptive Statistics for Ratio and Interval Data: Numerical Can be ranked (e.g., 50cm is longer than 20cm) Evenly spaced (e.g., the space between 1cm and 2cm is the same as the space between 2cm and 3cm) Ratio data has a true zero value (e.g., height or length), whereas interval data does not (e.g., temperature on the Celsius scale, year of birth) Examples: body weight, temperature, frequency of behaviours such as binge eating episodes, scores on psychological measures Frequency Distributions: Frequency distributions are a method of simplifying and organizing a set of scores by grouping them into an organized display. A frequency distribution table consists of two columns of information: – First column ► the scale of measurement – Second column ► the frequency of individuals located in each category 37 ↬ X= the number of possible scores that someone could get on a quiz. ↬ F= the number of people who scored at each of those scores. ↬ Polygon= line graph/dot are inserted. 38 Distribution characteristics Kurtosis: The property of being peaked (leptokurtic), flat (platykurtic), or in between (mesokurtic). Skewness: The property of being symmetrical or asymmetrical. “Positively skewed” is where the longer tail extends away from the X origin (to the right). “Negatively skewed” is where the longer tail extends toward the X origin (to the left). The normal distribution: A mesokurtic and symmetrical frequency curve. Most values fall in the central region and the frequency of scores fall off fairly rapidly on either side. Many (but not all) of the numerical phenomena we wish to measure are normally distributed. ↬ Mesokurtic= not to flat or peaked, symmetrical as it does not have the long tail towards and away from the X axis. 39 Part 2: Measures of central tendency and dispersion -Measures of Central Tendency – Central tendency is a statistical measure used with ratio or interval data. ↬ A measure of central tendency identifies a single score that defines the centre of the distribution of scores in the sample ↬ Goal: to identify the value that is most typical or most representative of the entire group– The mean, median, and mode 40 41 42 Dispersion Range = (highest score) – (lowest score)– The scores from 12 participants are: 0– The range is: 12 – 0 = 12, 1, 1, 1, 2, 2, 3, 3, 3, 4, 4, 12 Interquartile range (IQR) = range of the middle half of the distribution – Divide the distribution into 4 quartiles: Q1, Q2, Q3, Q4 43 Dispersion Similar to the mean, the SD is often preferred when reporting descriptive statistics (vs the range) as it accounts for every score in the distribution. ↬ Greater sampling stability. ↬ Works well for relatively normally distributed (mesokurtic, symmetrical) data. However, SD is sensitive to extreme scores (“outliers”) so not recommended for markedly skewed distributions. In such instances, convention is to report the IQR. Range rarely used as sensitive to outliers and only relies on two most extreme data points (50% of scores). 44 Part 3: Descriptive statistics for nominal and ordinal data and descriptive vs inferential statistics. Descriptive Statistics for Nominal and Ordinal Data Categorical data In nominal data, there is no ranking between categories (e.g., one gender is not superior to another) In ordinal data, there is ranking between categories, however the spacing between the categories is not even or is unknown (e.g., military ranks) The most common way to describe these data are via: – Frequency distributions: Histogram & polygon – Percentages: (number of observations within a category/number of total observations) *100 – Proportions: number of observations within a category/number of total observations – Mode: most frequently endorsed category (e.g.: most chosen pizza toppings) – Illustrated graphically 45 ↬ Calculate the % of adolescents that meet the diagnosis for different eating disorders. ↬ Prevalence of each of the eating disorder diagnoses in the sample. ↬ The least prevalent or lowest % was the subthreshold binge eating disorder. 46 ↬ The highest prevalent % was for the subthreshold probable bulimia nervosa with 4.6% ↬ The % of adolescents who met the criteria for any of the eating disorders was 22.2% which is quite high. Statistical Terminology Statistic: A summary value that describes a sample – Example: the average score for a sample Parameter: A summary value that describes a population – Example: the average score for a population 47 48 WEEK 12-HYPOTHESIS TESTING & SAMPLING What is a hypothesis? Part 1: - A precise, testable statement of a prediction (educated guess) ↬ I predict that it will rain by 4pm today -In research, a hypothesis proposes a possible relationship between the independent variable and the dependent variable. ↬ I predict that Drug A will significantly improve participants’ headache pain ↬ A hypothesis is stated at the start of the study – before you know anything about the results! ↬ Making up a hypothesis about what will happen after it has already happened is not very scientific. 49 What is a hypothesis? -A fundamental requirement of a hypothesis is that it is testable (so that it can be supported or rejected). -Testing predictions require us to think about the null hypothesis: ↬ Hypothesis testing is all about finding statistical evidence that you should reject this null hypothesis. ↬ Null hypothesis is important to us because it’s the aspects of that we are going to statistically test. ↬ Rejecting the null hypothesis, finding evidence. This allows us to reject the default assumption (that there’s in fact an effect). 50 Formulating a hypothesis statement “If I… (do this to an independent variable) … Then… (this will happen to the dependent variable).” A good hypothesis should: ↬ Include an “if” and “then” statement ↬ Include both the independent and dependent variables. -Be testable by experiment, survey or other scientifically sound technique. -Be based on prior information (e.g., existing literature, your own observations) Hypothesis Testing: Part 2 Testing a hypothesis - “Smokers weigh more, on average, than non-smokers” We get a sample of smokers, and a sample of non-smokers, and we weigh them. 51 ↬ IndepVar = smoking status ↬ Dependent Var = weight (kg) ↬Null hypothesis:the distributions overlap= no effect. ↬ Alternative hypothesis: distributions do not overlap & are different= there is an effect. Is there a difference between smokers and non-smokers? 52 Yes, looks like it! But how much can we trust the observed difference? The all-important p-value* * - Assuming the null hypothesis is true, how likely is the observed difference in our data? ↬ “How likely is it that we got this result just by chance?” - The p-value given by a statistical test answers this question. It gives you the probability of obtaining a difference of this size or larger, assuming that the null hypothesis is true ↬ i.e., the chances that the result is a false positive -Smaller p-values = good! Result “unlikely” to be due to chance - But what counts as “small enough”? The p-value * - Indicates the probability of incorrectly rejecting the null hypothesis ↬ Misclassifying a result-due-to-chance as a ‘real effect’) - making a false positive -Rejecting the null hypothesis when it is actually true = Type 1 Error ↬ Type 1 error occurs when you say there’s a real effect, but there is no effect. ↬ This becomes a problem as we do not want to draw important conclusions based on patterns of data that are simply due to chance. Experimenter decides on the Type 1 error rate: - An alpha of 0.05 (5%) indicates that you are willing to accept a 5% chance that you are wrong when you reject the null hypothesis. 53 ↬ If alpha = 0.05, then p-values (less than) <.05 are “statistically significant”. ↬ We are confident that there is a 95% chance that it’s a real result. Thinking about Samples: Part 3 Sampling -You want to know something about a population (e.g., over 55s) -Since you cannot feasibly test every person over 55, instead you get yourself a sample of people aged over 55. ↬ Obviously, sample < population -How you select your sample matters! -The outcome of a study may depend on the method used to select participants -The sample must represent the population – otherwise we cannot generalize from our results 54 1. General question about a population such as is cycling or running better for people who are aged 55? The population is everyone in the age group. 2. Select a sample people aged over 55. The sample is drawn from a population & is smaller than the population. 3. Research is conducted with this sample. Find some interesting effects & in the discussion part, we must generalise the findings to people all over 55. 4. Findings can be that cycling is better than running for people over 55 over this kind of time period. Also, mention the effects of it & benefits we can expect to see. We expect that for everyone over 55, not just the sampled that was tested. ↬ Target Population: People over 55 worldwide. ↬ Accessible Population: The members of the target population that we reasonably have access to e.g.: people from Sydney over 55, so will not expect other people living in other countries. - We also think about the relationship between the relationship between the target population and accessible population. 55 ↬ Findings may have important cultural caveats to be made. ↬ E.g.: testing particpants,where findings research effects the Western cultures. ↬ So may not be generalised to the Asian or Indigenous cultures. ↬ Must be careful about what we consider as representative & think carefully about our sample characteristics in terms of conclusions we can draw from. Representative Samples (1 of 2) -Our goal in experimental research is always to conclude something meaningful about the population under study ↬ e.g., cycling provides more health benefits than running does for over 55s. - This means that the sample we test must reflect or “represent” the population we want to conclude something about ↬ E.g., imagine we recruit our sample of over 55s exclusively from expensive, women’s only fitness centres in Sydney’s eastern suburbs - Would we really be able to say anything about “over 55s” in general? ↬ Not appropriate as it has a largely female sample from a very affluent & high socioeconomic status. Representative Samples (2 of 2) -Bias is a major threat. ↬ A biased sample’s characteristics are noticeably different from those of the population. ↬ Some individuals in the sample may be older, faster, smarter, etc. ↬ Selection bias (also called sampling bias) 56 ↬ Participants or subjects are selected in a manner that increases the probability of obtaining a biased sample. ↬ E.g.: only putting flyers in one section or one suburb of Sydney. This means that we will only recruit people from that suburb or local to that area. Sample Size Sample size affects representativeness - Law of large numbers: a large sample will probably be more representative than a small sample. ↬ A sample size of 25 to 30 individuals for each group or treatment condition is a good target for many studies. Research ethics governs sample size - Overly large samples are unnecessarily using extra participant. ↬ If a sample is too small, then it is unlikely that the study will be successful, which is also an unethical waste of resources What are two ethical aspects to consider when planning your sample? 57 Answer: 1. If a sample is too large, then it is unnecessarily using extra subjects or participants. 2. If a sample is too small, then it is unlikely that the study will be successful, which is also an unethical waste of resources. Sampling Methods: Part 4 Two categories of sampling methods Probability sampling -The exact size of the population must be known, and it must be possible to list all the individuals (e.g., all students enrolled at WSU) -Each individual in the population must have a specified probability of selection -The selection process must be unbiased; it must be a random process Non-Probability sampling ↬ Odds of selecting a particular individual are unknown 1. Simple random sampling Participants are selected from a list containing the total population: ↬ Equality: each individual has an equal chance of selection. ↬ Independence: choice of one individual does not influence or bias the probability of choosing another individual. ↬ e.g., we get a list of all enrolled WSU students, and we randomly pick 1000 of those to be in our sample Two methods: 58 ↬ Sampling with replacement – we pull one individual out (a name out of a box), record their data, then put them back into the population before making the next selection. ↬ Sampling without replacement – each selected individual is removed from the population before the next selection is made. ↬ Do not want the same person to win in the raffle for example. Concerns about this approach: -Since chance determines each selection, it is possible (although usually unlikely) to obtain a very distorted sample. ↬ Example: coin toss – you *might* get 10 heads in a row = not a representative sample of the possible coin tosses in the world. ↬ Do not have control over how the selection plays out. Additional restrictions on random sampling techniques help avoid a non- representative sample. ↬ E.g., instead of a fully randomised selection, we decide to ensure that the ratio of M:F students in the sample matches the M:F ratio in the total population. 2. Systematic sampling -Every nth participant is selected from a list containing the total population. ↬ A random starting position is chosen - Violates the principle of independence – because the selection of one individual does have an impact on which individual is chosen next -Ensures a high degree of representativeness. 59 ↬ e.g., Instead of a fully randomized selection, we get the full list of enrolled students, randomly choose a starting point, and then take every 50th student in the list. ↬ The individual then does have an impact on which individual is chosen next. 3. Stratified random sampling -The population is divided into subgroups (strata), then equal numbers are randomly selected from each of the subgroups. - Guarantees that each subgroup will have adequate representation. - Overall sample is usually not representative of the population. ↬ e.g., Instead of a fully randomized selection, we decide to sample 100 students from each WSU campus. ↬ The final sample (n = 1,300) represents each campus equally ↬ but it does not reflect the actual enrolment rates at each campus 3A. Proportionate random sampling -The population is subdivided into strata, number of participants from each stratum is selected randomly (not equally). ↬ E.g.: instead of taking an equal number of subjects from every single campus, the number of subjects is now for a given campus should be proportional to the enrolment rates the campus. ↬ The proportions in the sample correspond to the proportions in the population. ↬ Ensures the sample will be representative of the population 60 ↬ Requires a lot of work and may make it difficult or impossible to compare subgroups within strata. 4. Cluster sampling - Clusters (preexisting groups) are randomly selected from a list of all the clusters that exist within the population. ↬ An easy method for obtaining a large, relatively random sample - Selections are not really random or independent. ↬ e.g., Instead of a fully randomized selection of all WSU students, we decide that our sample will be all students enrolled at three randomly selected WSU campuses. ↬ Then put all the names of the WSU campuses in a hat,randomly draw out three and that is our sample. 5.Combined Strategy sampling -Two or more sampling strategies are combined to select participants. ↬ Optimizes the chances that a sample is representative of a widely dispersed or broad-based population. ↬ Examples of applications: a wide market survey or a political poll. ↬ Pseudo randomisation, which is where you generally want things to be randomised but with some constraints. 61 Non-Probability Sampling; 1. Convenience sampling Sample is made up of individuals who are available and willing. ↬ An easy method for obtaining a sample, but a weak form of sampling. ↬ The sample is probably biased Selecting a reasonably representative sample and clearly describing the selection process helps combat problems with this method. ↬ e.g., Instead of a fully randomized selection, we send all enrolled WSU students an email and ask them to sign up online. Our sample consists of whoever is willing to do so. ↬ The sample is biased towards students with the time/inclination to sign up. 2. Quota sampling Subgroups to be included are identified. ↬ Quotas are established for individuals to be selected through convenience from each subgroup. Allows a researcher to control the composition of a convenience sample ↬ The sample is still probably biased. ↬ e.g., We email all WSU students and ask them to sign up for our study. We take the first 100 students from every campus who respond. Our final sample consists of an equal number of students from each WSU campus who have the time/inclination to sign up 62 63