Research Methodology and Descriptive Statistics Units Summary PDF
Document Details
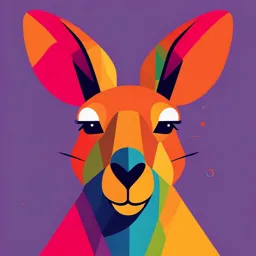
Uploaded by BlissfulDubnium
Universiteit Twente
Tags
Summary
This document provides a summary of research methodology and descriptive statistics, focusing on practical research questions, theoretical/academic research questions, and data collection methods. It's part of a larger course at Universiteit Twente, but isn't a past paper.
Full Transcript
lOMoARcPSD|48636498 Research Methodology and Descriptive Statistics - units summary part I and II Research methodology (Universiteit Twente) Scannen om te openen op Studeersnel Studeersnel wordt niet gesponsord of ondersteund...
lOMoARcPSD|48636498 Research Methodology and Descriptive Statistics - units summary part I and II Research methodology (Universiteit Twente) Scannen om te openen op Studeersnel Studeersnel wordt niet gesponsord of ondersteund door een hogeschool of universiteit Gedownload door Jolein Beute ([email protected]) lOMoARcPSD|48636498 Research Methodology and Descriptive Statistics – Lesson 1 – 08/02/23 R-test (computer program), its also a programming language. two assessments. 24 units. Questions day before at 13:00. Assessments: Two MC tests (graded, 40 q’s & above 5 but average test above 5.5), 1 R test (at the same time as test 2)(pass/fail), 24 assignments (every lesson basically). You could earn a bonus point of 0.5 IF you at least made 20 assignments (units) seriously & if both tests are at least graded with a 5.3 & if you passed the R-test. How do we collect data? How do we know that some method is better than the other? Empirical research questions. Assignment 1 - The social scientist makes a similar, probabilistic prediction—that women overall are likely to earn less than men. Once a pattern like this is observed, the social scientist has grounds for asking why it exists. – - Systematically: excluding the possibility that other answers are better than the answer we give (logic). - The process of going from theory to research is called deduction. And the process of going from data analysis to the answers and knowledge is called induction. - Empirical research questions are often asked in the context of decision making. - ‘How to’ questions can be ‘broken up’ into descriptive and explanatory research questions. So empirical questions can be divided into explanatory and descriptive. Practical research questions process (wheel of science): 1. problem definition and – analysis 2. design (options that have been used) 3. multi criteria analysis, option evaluation 4. decision making rules (preferred alternative) 5. implementation 6. ex post evaluation Theoretical or academic research questions: 1. Think: theory 2. Plan: Research design 3. Observe: data collection 4. Analyze & conclude: Data analysis Phase one: “I ask descriptive questions like ‘how many street robberies did take place between 2000 and 2013?’ This question, asked in the context of decision-making can be answered by doing empirical research.” “‘Why do people rob other people?’, ‘What places are vulnerable for street robberies?’ or ‘Why did the number of street robberies go down in the past 10 years?’ These types of questions can be answered in explanatory research.” These types of questions are used to explain the is-part (status quo) of the problem. Bij empirisch onderzoek voer je zelf een onderzoek uit om nieuwe kennis op te doen. Er bestaan twee varianten: kwantitatief onderzoek & kwalitatief onderzoek Empirisch onderzoek is de op de praktijkgerichte tegenhanger van literatuuronderzoek. Als je empirisch te werk gaat, kun je nieuwe uitspraken doen over de werkelijkheid. De meeste Gedownload door Jolein Beute ([email protected]) lOMoARcPSD|48636498 studenten die voor een empirische scriptie kiezen, gebruiken experimenten, quasi-experimenten, interviews, observaties of enquêtes om data te verzamelen. Je selecteert onderzoeken waarin dit onderwerp al is behandeld en maakt een samenvatting van de resultaten die al zijn gevonden. Uiteindelijk beantwoord je op basis van je literatuurstudie de onderzoeksvraag. (using descriptive questions in the problem definition and problem analysis.) After having described the problem or the opportunity and after analyzing its causes, you may want to have one or more options for solving the problem. Or having a list of demands for a product. This is phase two, design. If there are no previous options, then you need to develop some new ones. You get there by using design techniques. These are never based on logic but on what works better in certain circumstances (example: brainstorming). Phase three, evaluate your options. Do my options meet the criteria I’ve set in phase one (problem analysis)? You answer these types of questions mostly by doing empirical research. But you decide before any choice is made so its called ex ante (before) evaluation. You can base decisions on literature studies, evidence based research or simulation. Now we can make real decisions. Its very hard. It is beyond the scope of this paper to list the ways in which decisions can be made and which methods have been designed to improve decision-making. In phase five you look at the implementation. How will you execute the method or distribute the product? In the final phase you look back at the choices you have made during the six step process. To which extent does the end result meet up with the criteria stated in the first phase? Also, did your plan work out as it was supposed to? This phase is called the outcome evaluation. But it can be very hard to observe whether decisions worked out as intended. That’s why this phase can also turn into a process evaluation. Confirmation bias: frequently made mistakes in politics, business, social relationships, social science, psychology ( conspiracy theories). Je “cherry-pickt” alleen wat je wilt horen. - Normative questions are about ethical dillema’s. What is allowed & what is good? - Conceptional questions are about the proper/useful/efficient meaning of words what is freedom or what is the definition of democracy? ‘should’ at the beginning of a question is a conceptional question. - Empirical questions are answered by observations based on descriptive, relational (correlation) and explanatory questions. Own observations + theory/thinking are necessary. Research in the context of decision-making may be applied research, but that is not necessarily the case. Applied research is ‘using existing knowledge (theories and methods) to better understand a particular case or problem’ In explanatory research you first think about potential answers. The results of this thinking, in many cases based on a literature study, are summarized in a preliminary answer to this question called a theory. Sometimes, but definitely not always, these theories are tested to see whether they indeed offer an explanation of the is-part of the problem. there will always be discussion about the exact difference between units and setting (and even between variables and setting, but that is less often mentioned). For example, if we are studying Dutch teenagers, ‘Dutch teenagers” can be seen as the units or “teenagers” Gedownload door Jolein Beute ([email protected]) lOMoARcPSD|48636498 can be seen as the units and “The Netherlands” the spatial aspect of the setting. We can even have a discussion about the nuanced differences between Dutch teenagers, non- Dutch teenagers living in The Netherlands, and Dutch teenagers living abroad. But in most cases this is not very relevant. Assignment 2 Social science is about Units of analysis, variables and attributes. Units are a logical set of variables. The variables Moroccan, Dutch, Russian or Turkish all are part of the unit ethnicity. There are two types of variables, dependent (effect) and independent (cause). Example: your education level (effect) is most likely to be higher when your parents had a lot of education (cause). Units: the answer to the question "Who/what is characterized by a variable? (a group of) person, organization or countries. Variable: the answer to the question "What characteristic(s) does the unit have?” Characteristics of those units. There are independent and dependent variables. Dependent variables are related to intended outcome and effect measures. In this RQ the variable is "hobby's", the units are "students at the UT". Football, running, gym, tennis etcetera are the attributes(=values) of the variable hobby's. An empirical research question is only correct if it refers to a clear unit (of analysis) and variables. Explanations, we have all given them. But in what way? There is an idiographic way of explaining, which means to explain the case fully by making the other understand all the aspects of what happened. In a research they try to fully understand the subject or group. Example, because I studied at home, I got a lower grade because there were distractions. And my cat kept asking to play with her and I still had housework to do, etc.. Nomothetic explanations are characterized by generalizing. It only speaks about the relation between two variables in the story. Example, I get a better grade if I study in the library then at home. In social science there are also two types of reasoning. When you move from a specific (a good grade on a test) to a general (cause: studying with others) its called induction. You move your way from an observation to a possible cause. It doesn’t tell you why the pattern exist or why its related to each other. It just tells you that it does. This second mode of inquiry, deduction, moves from the general to the specific. It moves from (1) a pattern that might be logically or theoretically expected to (2) observations that test whether the expected pattern actually occurs. However, both present equally valid results. Social researchers deal with questions and answers in a probabilistic way. They almost never use definite words like, always or never. You’ll see words like most likely or the majority. Determinism is a philosophical view, where all events are determined completely by previously existing causes. In social science, agency is the capacity of individuals to have the power and resources to fulfill their potential. Another complex definition: tolerance for ambiguity. It means to have the ability to hold conflicting ideas in your head a t the same time without denying or dismissing any of them. There are three most common purposes of research; exploration, description and explanation. Exploration study could help you find out answers to some widespread Gedownload door Jolein Beute ([email protected]) lOMoARcPSD|48636498 questions, like how a certain group moves or what motivates them. Exploratory studies are most typically done for three purposes: to satisfy the researchers curiosity, to test the usefulness of a more extensive study and to develop methods to be employed in any later study. But many social science studies have as a goal to describe situations and events. They observe and then describe what happened. Many qualitative studies aim primarily at description. And then there is the third purpose of social science research, to explain things. Where descriptive studies answer questions of what, where and how – explanatory research tries to answer the question of why. Causal (or explanatory) means that you want to explain something that you have observed (e.g. cancer), the reason why it happened (e.g. unheallty life style). In a causal RQ you want to find out whether e.g. an unheallty life style causes cancer' (= whether the cause causes the effect). How can we formulate empirical research questions? Start with a topic that interests you but narrow it down. Don’t have a topic that’s too broad, it should be relevant answerable. There are two context of research question, practical or theoretical. Practical research questions can be broken up in the six step circle (assignment 1). Theoretical/academic research is a five step circle (assignment 1). Is the question causal/explanatory? A clearly formulated research question should include units, variables (treatments and observations) and setting (time and place, relevance). Assignment 3 After this unit, you will be able to... recognize the units of analysis and the units of observation in a study; tell how mixing up units of analysis and units of observation may sometimes lead to the ecological fallacy; identify variables with their attributes / values used in a study; determine if the attributes / values of a variable are complete (a.k.a. exhaustive) and mutually exclusive; differentiate between different levels of measurement; explain what a data matrix looks like. Units of analysis: the subject being studied (what/who). Usually individuals. Which seems weird because in a research question you often see groups of people. But if the researcher is interested in exploring, describing, or explaining how different groups of individuals behave as individuals, the unit of analysis is the individual, not the group. Any type of individual can be the unit of analysis for social research, this also applies to ant Gedownload door Jolein Beute ([email protected]) lOMoARcPSD|48636498 social group. While doing research and drawing conclusions from the results, two pitfalls can occur in regards to units of analysis. The ecological fallacy (something larger than individuals, misvatting) and Reductionism. The ecological fallacy is the assumption that something learned about an ecological unit says something about the individuals making up that unit. Reductionism involves attempts to explain a particular phenomenon in terms of limited and/ or lower-order concepts. It involves more than the researcher is including. Units of observation are usually different than the units of analysis. Units of obersvation are the perspective of the date. Example, we are studying married couples (unit of analysis) and we formulate hypotheses about them and ask them questions. But we collect data by talking to them individually (units of observations). What perspective does the data have? When these two concepts are different, we should be careful with drawing conclusions. Its not necessarily correct to draw conclusions about lower level units (individuals) with aggregate data (regions). Sociobiology = a paradigm based on the view that social behavior can be explained solely in terms of genetic characteristics and behavior. All variables are composed of attributes, but there are five levels of measurement we can distinguish. Dichotomy, nominal, ordinal, interval and ratio. Dichotomy measures (dichotomous) o Variables whose attributes are different from one another o Only two attributes (yes/no) o In SPSS: nominal o In R: Logical/Factor Nominal measures o Variables whose attributes are different from another o More than two, but not ordered. At least three o In SPSS: nominal o In R: Logical/ Factor Gender (male, female, other). Occupation (fireman, social worker) Ordinal measures o Variables with attributes we can logically rank or order o The distance between the values is unknown o In SPSS: ordinal o In R: Ordered Prejudice or income or IQ level Ranking (high, medium, low or words like very and more) Interval measures o Variables with attributes who are equally spaced from one another o known distance between them o Not age! Because there is no meaningful zero point o In SPSS: Scale o In R: Numeric (this can be discrete (1,2,3,4) or continuous (1.2,1.3,1.4) Fahrenheit or Celsius Scales or scores Ratio measure o Variables with attributes who meet all the requirements of previous levels of measurement. o You can say twice as much Gedownload door Jolein Beute ([email protected]) lOMoARcPSD|48636498 o Meaningful zero point o In SPSS: Scale o In R: Numeric (this can be discrete (1,2,3,4) or continuous (1.2,1.3,1.4) Can be nominal, ordinal, interval This data can go back to a true zero (age) The words values and attributes both refer to the same thing. However, values usually refer to numerical factors. In SPSS attributes (like, color) have numbers that are labelled. In R attributes are often stored as words. When conceptualizing the variables and attributes we must keep two concepts in mind. Exhaustive/complete and mutually exclusive. The term complete refers to that the unit is characterized by one of its attributes/values. So the values should "match" the unit. This means that you should make the attributes applicable to the unit as well as having them defined in a way that facets are also captured if needed. All the options should be noted as an attribute, leave no option out. For example, you cannot ask if someone is rich/poor, but it can also be the middle so you need to capture these values in between as well. For mutually exclusive, you should pay attention that units are characterized by only one attribute/value. Two things cannot happen simultaneously. So, you cannot ask if someone is nice and has good grades because these two attributes are not possible to be summarized and are not excluding each other. So, a person can be nice but also has good grades, but it can also be that only one of the two applies. The attributes need to be exhaustive/complete and mutually exclusive. Wide/broad format: Long format: Gedownload door Jolein Beute ([email protected]) lOMoARcPSD|48636498 Assignment 5 We all have a different meaning of words, certain mental images that come up. In social research, we try to come to an agreement of what certain terms (or constructs) mean. We call that conceptualization, with the result of a clear concept. A construct is a more difficult term than a concept. Concepts, constructs and terms all mean more or less the same thing, except that concepts are more generally-accepted characteristics, such as age or grade on an exam, and constructs are more complicated ideas, such as democracy or personality. Indicator = an observation that is an reflection of a variable we are studying. Dimension = an aspect of an concept. There are four types of correlation between terms and facets. Let´s assume we have the concept of Aggression- - in the sense maybe of an aggressive person- where we have the facets verbal aggression, physical aggression, and hostile aggression. AND-relationship: you would only be classified as an aggressive person, if you show all three kinds of aggression. You need to check all boxes to qualify. NOT-relationship: different combinations of the dimensions would have different names and result in a new concept (e.g. summarizing verbal and physical aggression and NOT hostile aggression and call this "sub-scale" common aggression). Also, typology. Missing: Each dimension is treated as a separate variable without a relationship to each other. So in this case that would be verbal aggression, physical aggression and hostile aggression seperately as three variables. The combination will not be useful in a research. OR-relationship: in this case a high score on of the three dimension would be enough to be classified as an aggressive person, so you do not necessarily need to score high on all three dimensions. A high score on one facet is enough to be classified as a (insert term). Constructs can refer to both units and variables. And all do have a certain measurement level. We usually begin with a construct, this we divide into facets and we can learn something about facets if we look at the indicator. An indicator is a sign of the presence or absence of the concept. They measure the construct while holding into account the facets (=dimensions aswell). This is the deductive approach to science. These steps all have names: 1. Construct divided into facets a. Conceptualization 2. Facets into indicators a. Operationalization b. The way youre gonna measure something 3. Indicators into observations a. measurement If we do this the other way around, from observations to a construct, then this is called inductive. Gedownload door Jolein Beute ([email protected]) lOMoARcPSD|48636498 There are three ways to combine indicators: 1. index a. create a ordinal variable by adding up answers (grading). Your grade is the index. 2. Typology a. Creating a nominal variable. b. By using dimensions (facets) of the construct 3. Scale a. Ordinal variable by combining answers but it is not a scale if you check the empirical relations between these variables. Factor analysis. Both indexes and scales are ordinal measures of variables, they rank order the units of analysis. Both are also composite measures of variables. A scale usually shows us a logical or numerical structure between serval indexes. But it can also be used interchangeably. A typology is creating a set of categories or types, which we call a typology. The term content validation means that all aspects of the construct are included in the operationalization (measurement planning). Assignment 6 Data collection methods: - survey - diary methods - (in depth) interview - Coding documents - Psychical measures - Focus group recordings - Observing behaviour To classify them we can divide these methods into primary and secondary data. Primary data is the data you collect and secondary data already exist. There are obtrusive and unobtrusive methods. The first one affects the human you’re interested in, the second one does not. Also verbal and non-verbal methods. Bivariate relationships: You have two variables and you want to know how they connect. Assignment 7 Content analysis steps: 1. Research question including variables and a unit of analysis 2. Select primary documents 3. Develop an operationalization 4. Coding 5. Conclusion 6. Reporting the method and finding Gedownload door Jolein Beute ([email protected]) lOMoARcPSD|48636498 Content analysis is a data collection method. In this method we look at variables by coding a set of primary documents to say something about the unit of analysis. It is an unobtrusive method, it doesn’t affect anything or anyone. There are two different aims of coding and content analysis: 1. Creating concepts or better understanding how concepts are used. a. No arguments about generalizing. 2. Descriptive and comparative research. Primary documents: Primary sources provide raw information and first-hand evidence. Content analysis is a method often used when starting at the information of primary documents. So coding is both inductive and deductive (from variables to data & other way around). When you’re not comparing units in a study it is called a non comparative content analysis. Three ways of coding: 1. Deductive coding a. Creating variables from data (theoretical constructs) 2. Inductive coding a. Creating theoretical constructs using primary documents 3. Combination of the two a. Going back and forth A way to come up with a new dimension or facet is to construct interviews. This is called conceptualization using interviews. After this you can validate in research. Sampling and saturation are closely connected. Code saturation refers to how we can use code to understand the text. Theoretical saturation refers to additional people do not add new meanings. Manifest coding/ analytic coding: Measure a content by choosing a certain word and counting it, for example. Manifest coding is more reliable than latent coding because the word is either there or is not (word counting), and different results can only come in error by missing a word. Latent coding/holistic scoring: Latent coding goes beyond the descriptive level of the data and attempts to identify hidden meanings or underlying assumptions, ideas, or ideologies that may shape or inform the descriptive or semantic content of the data. Inter coder reliability = if a group has different variables Assignment 8 Sometimes we have a perfect unit of analysis and there’s no mistake in our sampling but observation may be imperfect. Two types of observation mistakes: - Random errors o These can happen accidently o When there’s no random error, this is called: measurement/construct reliability o Same result every time you measure? - Systematic errors Gedownload door Jolein Beute ([email protected]) lOMoARcPSD|48636498 o Bias or measuring the wrong construct o When there’s no systematic error, this is called: measurement validity. So, the validity tells us if the result is correct or true. This can only be tested/measured if you already have the answer to your question. Then you can test if it is correct. But this is not always the case. So when the result is not known, there are three ways to approach measurement validity. 1. Content validity a. Does it cover all the aspects of the concept? i. BMI, so weight AND length. 2. Criterion validity a. Predictive validity b. Example: drivers test 3. Construct validity a. Is it correctly related to theoretically related variables? b. Which method does predict or days something the best about *obesitas Bias: systematic error introduced into sampling or testing by selecting or encouraging one outcome or answer over others Face validity: The quality of an indicator that makes it seem to be a reasonable measure of some variable. That it’s a logical way to measure something. Common agreement. Assignment 9 So, a Pie Chart is easier if you want to see the percentage easily. A Bar Graph is easier for the numbers. And when you have a high number of categories, use the BG. Dotplot: placing dots on a chart. The picture below are all histograms. Gedownload door Jolein Beute ([email protected]) lOMoARcPSD|48636498 Assignment 10 Mean = average Variance = each difference, square it, and then average the result Standard deviation = the square root of variance Data can be shown in a graph, but also a center. There are three ways; the mode, the median and the mean. These are called, the measures of central tendency. Mode = the value that occurs most often Median = the middle value of your observations when arranged from low to high. Order them and then choose the middle. Mean = sum of all the values divided by the number of observations These methods are used to provide data about the middle or center of your observations. In the picture, we can see the guidelines. There are many measures of variability. The range (lowest to highest) and the interquartile range (middle half) for example. But the frequently used are: 1) Variance Gedownload door Jolein Beute ([email protected]) lOMoARcPSD|48636498 The larger the variance, the larger the variability. This means the values are more spread out. 2) Standard deviation This is the average distance of an observation from the mean. To visualize the distribution of quantitative variables (a.k.a. interval and ratio variables) you could use a boxplot. It contains are a box, two whiskers and an outlier. The box has a median, Q1 and Q3. The range between Q1 and Q3 is called the interquartile range. IQR is Q3 – Q1. End of an whisker is 1.5 times IQR. The outcome of that equation is used to step from Q1. Usually there are no observation at that point, so then you take the closest observation in the direction of the median. The values outside the whiskers are called the outliers. Assignment 11 Z-scores =number of standard deviations removed from the mean. Mostly its used by researchers when trying to answer the question if something is common or exceptional. This is about one data point, while a standard deviation calculates the average. Standardized values are values scaled by population data. It is basically comparing data to a certain group or whatever you want to compare it with. It is called standardization. Z-scores are telling us a lot about the distribution curve (histogram). In a bell shaped distribution there are guidelines: Gedownload door Jolein Beute ([email protected]) lOMoARcPSD|48636498 So that means scores larger than 3 or less than -3 are exceptional. When you have a distribution skewed to the right, then larger z-scores are more common. When a distribution is skewed to the left, larger negative z-scores are more common. - 1Sd = 68% - 2Sd = 95% - 3Sd = 99.7% Lecture – 10/3/23 You should be able to calculate the following for the exam: - Mode, mean, median - IQR, variance, standard deviation - Z-score - Empirical rule - Normal distribution - Standard normal distributions - Non normal distributions Part two Assignment 13 Aims: - differentiate between bivariate and univariate graphs and tables (and you know when to use what kind of display); Gedownload door Jolein Beute ([email protected]) lOMoARcPSD|48636498 - create a scatterplot (using statistical software and by hand) with the independent variable on the X-axis and the dependent variable on the Y-axis; - create a contingency table (using statistical software and by hand) with the independent variable in the columns, the dependent variables in the rows, and column percentages in the cells; - interpret results that are displayed in scatterplots and contingency tables. There are frequency tables and contingency tables. The difference are the variables. In a frequency table, only one variable is displayed. In a contingency table there are two variables. When data is put in this table it doesn’t tell you much yet about the correlation between two or more variables. That’s why we convert it into column percentages. When we have the percentages, you can display them as conditional proportions as well. So then, 40% becomes 0.40. When you only use the data in the marge for the proportions, then you’re displaying marginal proportions. Assignment 24 Aims: - select the correct measure of association to quantify the association between two variables given the measurement levels of the variables - compute different measures of association using statistical software. One of the measures of correlation is Pearson’s R. It describes the direction and the strength of a linear correlation with one number. When you draw a line in the middle of the scatterplot it goes up, down or stays horizontal. Up = positive correlation. Down = negative correlation. The closer the dots to the line, the stronger the connection. When the line is not linear, but a curve, we call this a curvilinear relationship. Pearson’s R is never a number below -1 and never a number above 1. To calculate, we use the formula below. First we change the original scores to Z-scores, we standardize the data. Then multiply X’s Z score with Y’s Z score. Further apply the formula. --------------------------------------------------------------------------------------------------- Gedownload door Jolein Beute ([email protected]) lOMoARcPSD|48636498 ‘Measures of association’ refers to a wide variety of coefficients that measure (the direction and) the strength of an association between two variables (bi-variate) in a dataset. Most of the coefficients can take values between -1 (perfect negative association) and +1 (perfect positive association), with 0 meaning no relationship at all (values close to zero can be seen as weak associations). The number of coefficients that can be used to describe relationships between variables is very large. The choice between these measures depends to a large extent on the level of measurement of the variables that are being used. In this course we will only discuss % difference E, Pearson’s r, Spearman’s rho, Kendall’s tau-b, Kendall’s tau-c and Cramér’s V. In Table 1 you can see when to use these coefficients. To start from the right bottom of Table 1 we encounter Pearson’s r. Pearson’s r can be used to look at the association between two scale variables. It is a standardized measure of strength for the linear relationship between two scale variables only. What also should be noted, is that Pearson’s r is not robust (that means: Pearson’s r is sensitive to extreme values). Spearman’s rho can be used as a more robust coefficient to look at the relationship between two quantitative variables (ordinal or scale). Also, it can be used for consistently increasing or decreasing non-linear associations. Raw scores are sorted from high to low and replaced by the ranks of values. The highest value of a variable is given rank 1, the second highest value is given rank 2, etcetera. Because of that, it can also be used to look at the bivariate association between two ordinal variables. Kendall’s tau can also be used as a measure of association for a consistently increasing or decreasing relationship between two ordinal variables, but only when the number of categories is relatively small so the relationship can be displayed in a contingency table. Kendall’s tau-b can be used for squared tables (3x3, 4x4, for example), whereas Kendall’s tau-c can be used for rectangular tables (2x3, 3x4 etc..). To measure the association between two nominal variables or between a nominal and a ordinal variable Cramér’s V can be used. Unlike the previous coefficients, Cramér’s V cannot take a value lower than 0, since the categories are not ordered and it therefore does not make sense to talk about a positive or negative association. In case of two dichotomous variables, we use the % difference E (Epsilon. The counts of two dichotomous variables are shown in a squared contingency table (2x2) and the column percentages for the independent variable are calculated. The percentages are compared horizontally and expressed as % difference E. Assignment 12 Gedownload door Jolein Beute ([email protected]) lOMoARcPSD|48636498 Aim: - explain that causal statements play a big role when trying to answer empirical explanatory research question; - explain what is meant by the three basic implications of a bivariate causal statement: cause precedes consequence, cause is associated with consequence, and there is no third variable producing the observed relationship - distinguish linear from non-linear (causal) relationships between variables; - explain why relationships are often probabilistic and not deterministic Causal relationships are important because it explains how and why an effect occurs, and consequently, provides information regarding when and where the relationship can be replicated. If we make a causal statement about why things happen, we need to test three things: - Correct time order o This is do-able because we can check if X precedes Y in time o Cause precedes consequence - Association o Correlation between X and Y - Non- spuriousness o There is not a third variable upsetting the relationship. Like always, X is independent and Y is dependent. To check the time order, we can collect data at different points in time. But what happens when there’s a third variable (spurious relations)? There are two important keywords: - Explanation/confounding - Specification/interaction/modification Confounding is that the original relation disappears (full explanation) or that the original becomes weaker (partial explanation). The third variable explains the independent variable. Interaction is about weakening or letting the original relation disappear but it only applies to the dependent variable. X is not affected. Earlier, I mentioned that we more often use probabilistic relationships in social sciences. This is because of a few reasons: - Measurement error o It is difficult to have perfect measurement - We aim for simple models: parsimonious o We want a simple view of the world, so variables are left out. Deterministic relationship: ‘if this happens, this ALWAYS happens’. Probabilistic relationship: ‘if this happens, it is most likely or less likely that the other thing will happen.’. Assignment 15 Aim: - explain in what way studies with a correlational (cross-sectional) design, an interrupted time-series design, and an experimental design differ in their ability to test bivariate causal relationships; (wat is het verschil tussen deze drie?) Gedownload door Jolein Beute ([email protected]) lOMoARcPSD|48636498 - assess the internal validity of the three basic research designs (correlational, interrupted time-series, experiments); (the risks) - describe basic features of a classical experiment (including random assignment, posttest, pretest, treatment, placebo, and observation); - explain the basic idea of a double blind experiment; - use standard research design notation (R, N, X, O). There are three different research designs to test for causal relationships: cross sectional research design (correlation), interrupted time series and (classical) experiments. Research design is a way of answering an explanatory (causal) RQ. In Unit 12 we learned the three criteria of a causal relationship. The answer is listed above, by using one of the three research designs. Cross sectional research needs a unit and two variables. Its also required that the variables are measured in the same moment of time. But there are some possible threats. You can’t check if the time order is correct because theyre both measured in the same moment. And there's also not a third variable. So here we can establish that there is a correlation, but we can not say that there is a causal relationship. With interrupted time series we have a unit, two variables and timeline that goes like this: Y variable (dependent) is measured X variable (independent) is changed Y variable is measured again. This usually happens a lot of times in the interrupted time series. This example listed above is more like a before-after study. But this research design isn’t strong in excluding the influence of a third variable (spuriousness). Classical experiments include two groups: the experimental group and the control group, these are identical. The control group also exists of a before and after measurement but without something changing (placebo). With this research design there is no possible threat of missing the effect of a third variable, because these groups are the same. Nothing can change, so there is no third variable. Check, check, check. So why not always use experiments? Because theyre not always do-able and they require relatively simple RQ’s. With a design method such as cross sectional studies you have the risk of the time order problems. However, it van be used to collect data on confounders. To assess the research designs we look at validity. These four validities are grouped into two: - correct conclusions drawn in the study itself o statistical conclusion validity correct handling of data and using the right statistical tests association/correlation between variables o internal validity time order non-spurious relationship - Generalize conclusion for the greater group o Measurement and sampling validity Assessing the variables critically The way data is processed o External validity Gedownload door Jolein Beute ([email protected]) lOMoARcPSD|48636498 Can we generalize the conclusion Random assignment = randomization = important element of classical/randomized experiments. The placebo effect is the concept of giving the experimental group a certain factor, while you give the control group something else (but similar) (political show vs reality show). After measuring you see the difference (or not) between the two groups and you can make a comparison. The interval validity in the three different research designs: - Cross sectional research o Reverse causation cannot be rules out o Confounding third variables may affect the relation - Interrupted time series o Reversed causation cannot be ruled out o The composition in the group changes Selective attritions Panel mortality There are specific notations to use. Interrupted time series: - Observation/outcome/dependent variable = O - Change/independent variable / intervention = treatment = X Cross sectional/correlation research: - There are no treatments, only observations = O Classical experiment: - Two groups using randomization then measurements, treatment and another round of measurements - R = groups - X= the treatment, the number stands what number the change is. Is it the first or the third? - N = comparison group not created by random assignment Assignment 14 Aims: - explain how to analyze the effect of a third dichotomous variable on a bivariate relationship between two dichotomous variables (a trivariate relationship) using the elaboration model; - differentiate between various elaboration models, including: full or partial explanation, interpretation, specification, addition, and replication; - tell what type of ‘third’ variable is associated with these elaboration models: confounding variable, moderator or integration variable, intervening variable. Gedownload door Jolein Beute ([email protected]) lOMoARcPSD|48636498 When you're testing a bivariate hypothesis, you also need to look at the effect of a potential third variable. You do this by theorizing the variable, formulating a trivariate hypothesis and testing the trivariate hypothesis. There are four possible effects of introducing a third variable: 1. another independent variable/ addition a. only affecting the dependent variable 2. confounder/ explanation a. affecting both or letting the relation disappear b. if the x variable is explained by the third variable 3. intervening variable / interpretation a. trying to interpret the original relationship b. why? c. If the independent variable precedes (voorafgaan) the third variable in time 4. moderator variable/ interaction/specification a. modifying variable causes an interaction effect Assignment 16 Aims: - construct clear tables to confirm or reject a hypothesis; - explain why a 'confirmation' of the hypothesis only relates to the 'association' aspect of the hypothesis and somewhat to the 'third variable aspect'; o understand that a 'confirmation' of the hypothesis only relates to the 'association' aspect of the hypothesis and somewhat to the 'third variable aspect' ('somewhat' because there may be other 'third variables' affecting the relationship), NOT to the time order aspect - understand causality in the context of replication and addition models; Gedownload door Jolein Beute ([email protected]) lOMoARcPSD|48636498 - create a contingency table with two layers (in columns and/or in rows) with the correct percentages. This means you have to be able to create a 2x2x2 table. You test the hypothesis partly by testing the trivariate hypothesis by creating a trivariate table (2x2x2). A causal diagram is an arrow based model with plusses and minuses to indicate the relationship it has in comparison to one another. Assignment 17 Aims: - construct clear tables to confirm or reject a hypothesis - understand that a 'confirmation' of the hypothesis only relates to the 'association' aspect of the hypothesis and somewhat to the 'third variable aspect' ('somewhat' because there may be other 'third variables' affecting the relationship), NOT to the time order aspect When the original bivariate relationship disappears after adding a test variable, it is called spurious. Gedownload door Jolein Beute ([email protected]) lOMoARcPSD|48636498 Assignment 18 Aim: - display multivariate relationships using graphs and tables Assignment 19 Aims: - differentiate between non-probability sampling and probability sampling; - give examples of sampling methods; - explain the relationship between the population, sampling frame, and sample for probability sampling; - explain the consequences of sampling error, sampling bias, and non-response; - compute the response rate. When do we need sampling? We sample if not all units in our RQ can be studied. For example: ‘all Dutch people’, you cant collect data from all Dutch people. Sampling frame is the boundary withing you're looking for your target group. Then you sample etc. All these steps are called the sampling process and are displayed in the picture. But in a process errors occur. Sampling error or sampling bias. Non response or refusals. As shown in the picture. Everyone you interviewed divided by the people you selected is called response rate. The step between the sampling frame and the sample is called sampling. You have to ask yourself the question: is the chance that a specific unit from the sampling frame is included in the study, known? No non-probability sampling. Yes probability sampling. Non-probability sampling (strong bias): - Convenience - Purposive - Snowball sampling o Ask one person for other individuals who want to answer - Quota Probability sampling (size): Gedownload door Jolein Beute ([email protected]) lOMoARcPSD|48636498 - Simple randomly selecting from the sampling frame - Systematic random but systematic o The first column of the sampling frame o - Stratified - (multi-stage) cluster sampling We always make mistakes sampling, these are called sampling bias and sampling error. In a bias you're studying the wrong group of people. In the error it is about the sample size and the characteristics of the population. Assignment 20 Aims: - explain the relationship between difference (in a table) or effect size (in a regression equation) (which are both indications of the effect), sample size, and significance; - compute the difference between two percentages in a contingency table that represent the effect of one variable on the other. In a research you draw a sample from a population. It is very unlikely that the sample will differ a lot from the population. If you draw an infinite number of samples from your population, you create a perfect bell shaped distribution with a mean equal to the population mean. This is called the sampling distribution of the sample mean. The mean that would be equal to the population mean is called sampling distribution. We know some things about a normal distribution, for instance, 95% of all samples lie between -2*Sd (of the sampling distribution) and +2*Sd (of the sampling distribution). That space between these two points is called the confidence interval. If we are ‘pretty confident’ that mju (mean) of the population lies in this interval. Central limit theorem = the sampling distribution of the sample mean is always around normal. A condition to that rule is that n should be large enough (30 or larger). It means that even if the distribution of the population isnt normal, the central limit theorem tell us that the sampling distribution of the sample mean is always normally distributed. An then you have the standard deviaton of the sampling distribution. It is affected by the standard deviation of the population and the sample size. A larger sample size (n) leads to a smaller Sd of the sampling distribution. Inference is the process that describes the extent to which sample statistics can say something (through a random sample) about the population. This is the basic question of inferential statistics. In the formula to calculate the Sd of the sampling distribution we do not know the Sd of the population. So then we look at the Sd of the sample size. Gedownload door Jolein Beute ([email protected]) lOMoARcPSD|48636498 Assignment 21 Aims: - explain the relationship between difference (in a table) or effect size (in a regression equation) (which are both indications of the effect), sample size, and significance; - compute the difference between two percentages in a contingency table that represent the effect of one variable on the other. What is a T-distribution? There is a 95% chance that the mean of the sample lies between -2.06 and 2.06. Causal research is about finding a slope. We use Greek letters for the intercept and the slope; β0 & β1. slope (de helling): geeft aan hoe stijl de lijn is; intercept: geeft aan wat het startpunt van de lijn is bij x = 0 (dus het punt waar de lijn de y-as kruist, daarom ook wel de y-intercept genoemd). The sampling distribution of the slopes is normal. The samples (b’s) are all within 95%. We need the Sd of a sampling distribution of the slope. The standard error is a statistical term that measures the accuracy with which a sample distribution represents a population by using standard deviation. Assignment 22 Aims: - understand and explain examples of unethical behavior in social science research; - Classify unethical behavior in research in categories; - Explain why these categories of behavior are deemed unethical. Research ethics is to systemize or fight the wrong factor in social science research. When you're a researcher you have to deal with units, principals/sponsors, other researchers and society. Units of observation: - No harm to objects o By providing anonymity o Confidentiality (identity is hidden by the researcher) - Informed consent o Using consent forms o Debriefing (telling them after the experiment) Principals: - Quality research and not overstretching claims Gedownload door Jolein Beute ([email protected]) lOMoARcPSD|48636498 o Sponsor non-disclosure o Confidentiality of result - Other researchers: o Plagiarism o Data fabrication, making up data o Transparency and replicability Data storage, giving access o Peer review Research proposals (for funding) Submitting papers for publishing Anonymity (so people wont get blamed when rejected) Society: - Relevance of research - No harm to society (hard to tell) Ethical dilemmas occur when the interests of the groups differs. Overall organization of research ethics: - Review boards - Competing interest disclosure - Company standards - If you violate, complaints procedures. Gedownload door Jolein Beute ([email protected])