QDA Finished Notes PDF
Document Details
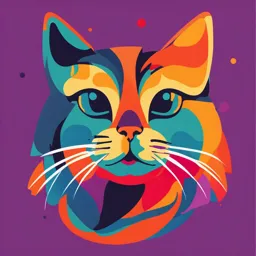
Uploaded by HolySynthesizer9046
Maastricht University
Tags
Summary
This document is summary notes on quantitative data analysis topics, including descriptive and inferential statistics. It discusses measures of central tendency and dispersion, and different types of variables and their measurement levels.
Full Transcript
Quantitative Data Analysis Lecture 1 Descriptive statistics: Techniques by which data are being - organized - Summarize - Represented Numeric - measures of central tendency and measure of dispersion Tabular - frequency tables and cross tabulation / contingency...
Quantitative Data Analysis Lecture 1 Descriptive statistics: Techniques by which data are being - organized - Summarize - Represented Numeric - measures of central tendency and measure of dispersion Tabular - frequency tables and cross tabulation / contingency tables Graphic - plots and graphs Inferential statistics: Techniques by which data are being used to generalize ndings - perform hypothesis tests - Make predictions POPULATION - The entire group of objects, organisms or events we are interested in, including all people or items with the characteristic we wish to understand - The group about which the researcher wishes to draw conclusions - Must be de ned in enough detail to determine whether a given individual or event is included or excluded - Contains all members of the de ned group SAMPLE - Any subset of the population, usually meant to represent the population - A sample is generally selected for study because the population is too large to manage in its entirety - The sample should be representative of the general population, so that the … PROBABILISTIC: Equal probability (greater than 0) for each member in the population to be selected NON - PROBABILISTIC: - strong biases on age (low), education (high) - “Convenience sampling” VARIABLE A variable is a measurable characteristic that varies. It may changes from group to group, person to person, or even within one person over time. If there is no variance, this is not a variable but a constant. Examples: age, gender, IQ, income, party a liation… Every variable has one of four di erent levels of measurement: - Nominal - Ordinal - Interval - Ratio Nominal Variables: Used to name, label or categorize attributes that are being measured. When coding nominal variables with numbers, these numbers have no mathematical meaning. - There is no intrinsic ordering of these categories - Arithmetic operations cannot be performed on the numbers Ordinal variables: - ordinal variable is a type of measurement variable that takes values with an order or rank fi fi ff ffi fi - It os the 2nd level of measurement and is an extension of the nominal variable. - There is an intrinsic ordering of these categories - Arithmetic operations cannot be performed on the numbers Interval variables: - interval scales are numeric scales in which we know both the order and the exact di erences between the values - It is the 3rd level of measurement and is an extension of the ordinal variable - There is an intrinsic ordering of these categories - Arithmetic operations: addition and subtraction (no multiplication and division) The 0 does not represent the complete absence of something and it's not the same for everybody. Ratio variables: - same as interval scales and also have an absolute zero - It os the highest level of measurement Dependent variables: the variables we are interested in, that we try to explain/predict. They DEPEND upon other. The question that you have to answer is “what are we trying to explain?” Outcome is another name for dependent variable Independent variables: these variables are their predictions Tutorial 1 De nition: Variable —> has four di erent levels 1. Nominal: is used yo categorize attributes that are being measured 2. Ordinal: type of measurement that takes values with an order or rank 3. Interval: numeric scales 4. Ratio: same as interval scales, it’s the highest level of measurement and it is an extension of the interval variable ANY VARIABLE THAT ONLY HAS 2 TYPES OF OBSERVATION, IS BY DEFINITION A NOMINAL VARIABLE Vector —> is a sequence of data elements of the same basic type Vector containing numeric values like 21, 23 and 25: c(21,23,25) Vector containing logical values: c( TRUE, TRUE, FALSE, TRUE, FALSE, TRUE, FALSE, FALSE) Vector containing string values: c(“Clinton”, “Bush”, “Obama”, “Trump”, “Biden”) Operation: Addition + —> 5+2 —> 7 Subtraction - —> 5-2 —> 3 Multiplication * —> 5*2 —> 10 Division / —> 5/2 —> 2.5 Exponentiation ^ —> 5^2 —> 25 Modulo* %% —> 5%%2 —> 1 Greater than > Less than < Greater than or equal to >= Less than or equal to mode = 5 84,85,86,86,86,87,88,89,89,89 —> mode = 86 —> we use the rule of thumb, we take the lowest if there are multiple options Male, female, female, female, male —> mode = female MODE NOMINAL YES ORDINAL YES INTERVAL YES RATIO YES THE MEDIAN: The median is the value separating the higher half from the lower half of data. Thus, 50% of the values are larger than the median and 50% of them are smaller than the median. - sort the vector in ascending order - The median is the middle value - The median is not the average 2,3,5,7,12,44,47,51,60 —> median = 14 1,2,6,7,16,41,47,55,58,71 —> media = (16 + 41) /2 = 28.5 if the numbers are even you need the average MEDIAN NOMINAL NO ORDINAL YES INTERVAL YES RATIO YES THE MEAN: Mean = … = sum of all observations / number of all observations MEAN NOMINAL NO ORDINAL NO INTERVAL YES RATIO YES RANGE: re ection between the di erence of the highest value and the lowest The range is useful for all 4 variables But the range only looks at the extreme values so it doesn’t take in consideration the values in the middle. Range = Max - Min Standard deviation from the mean formula σ = sigma —> standard deviation symbol μ = Average N = total number of observations Σ = capital sigma —> sum of … Xi = individual observation values depending on (i) The smaller sigma is the more homogenous the sample is, the greater the sigma is the more heterogenous the sample is. If sigma = 0 all values identical to mean (constant) Variance formula fl ff FREQUENCY TABLES - a frequency is a method of organizing raw data in a compact form by displaying a series of scores in ascending or descending order, together with their frequencies - the number of times each score occurs in the respective data set - In addition to the count, we look at the percentages , valid percentages and cumulative percentages Marginal frequency = the total Contingency tables / cross tables - a cross table is a two way table consisting of columns and rows displaying the (multivariate) frequency distribution of two variables - We can also use xtabs to eyeball relationships between variables Crosstable: LECTURE 3 Graphical Boxplot: used for continuous variable (so no ordinal and no nominal) Examples: Political position and Bucks NBA players INFERENTIAL STATISTICS Inferences about what? Population —> sample —> data —> statistics —> infer —> parameter Sample: a part of the population (subset) In this case the results given by the sample are generalized to the entire population. Example: if from the entire population of a country you take a sample and the results show that 40% of the sample would vote for party X, then also the 40% of the entire population is going to vote for party X. PROBABILITY Probability is the branch of mathematics concerning numerical descriptions of how likely a particular outcome is to occur. In an in nitely a very long sequence of like observations Poutcome = Nitem1 / N Examples Probabilities keep changing and you must be able to estimate a probability, but if you can’t your ndings are unclear. How likely a particular outcome is to occur, in a very long sequence of like observations: “ANY” fi fi How likely a particular outcome is to occur, in a very long sequence of like observations: “OR” How likely a particular outcome is to occur, in a very long sequence of like observations Central limit theorem The sampling distribution of the sample means approaches a normal distribution as the sample size gets larger. ???? Normal distribution -theoretical distribution of scores with mean (μ) and standard deviation (σ) -Perfectly symmetrical - Bell-shaped - Mean = median = mode - Unimodal —> one mode - Tails extend in nitely in both directions (asymptotic = the tails never reaches the value of zero, they stretch to in nity ) The 68.3-95.4-99.7 rule AKA the Empirical rule This rule tells you where most of the values lies in a normal distribution. Around 68% of the values are within 1 standard deviation of the mean (+/- 1) , around 95.4% of the values are within 2 standard deviations of the mean (+/- 2) and around 99.7% of the values are within 3 standard deviations of the mean (+/- 3). Anything that goes beyond the 3 standard deviation lines it’s an outliner. In a boxplot the outliners are indicated with circles. HYPOTHESIS TESTING “In statistics a hypothesis is a statement about a population. It takes the form of a prediction that a parameter takes a particular numerical value or falls in a certain range of values”. fi fi - The general goal of a hypothesis is to rule out the chance (sampling error) as a plausible explanation for the results from a research study. - The purpose of hypothesis testing is to determine whether there’s enough statistical evidence in favor of a certain belief or expectation, about a parameter - A hypothesis test evaluates two mutually exclusive statements about a population to determine which statement is best supported by the sample data “The null hypothesis, denoted as H0, is a statement that the parameter takes a particular value. The alternative hypothesis, denoted as Ha, states that the parameter falls in some alternative range of values. H0 = No association between the samples Usually the value in H0 corresponds, in a certain sense, to no e ect. The values in Ha then represent an e ect of some type”. (Agresti 2018, 152) In Hypothesis testing we are mainly going to use two types of testing: Z-Test and T-Test But when do you use Z-test and when do you use T-test? Formulas to nd Z and T: The Vincent’s example: We use the t.test to see if the average that Vincent told us (150) was the correct one based on his recent games. The p-value shows us that there is a signi cant di erence between Vincent’s hypothesis and the real mean (we can see that because the value is below 0.05). In fact the real mean is lower than the one that Vincent told us, since the t has a - in front of the number. (Check also Grandma’s example) fi fi ff ff ff Chi Squared hypothesis testing - Assessing the goodness of t of a statistical model involving comparisons of actual data with those expected under our model - Allows testing the signi cance of di erences between a set of observed frequencies and expected frequencies - Simple example —> we expect each exam answer (a, b, c, d) to be right 25% of the case. If this is not so, how big is the deviance? Example made with Chi Squared on R: SIGNIFICANCE LEVEL The signi cance level (denoted by alpha or p) is the probability that the test statistic will fall in the critical region when the null hypothesis is actually true. In other words, it’s the probability of making the wrong decision when the null hypothesis is true. Alpha levels (sometimes just called “ signi cance levels”) are used in hypothesis tests. Usually these tests are run with an alpha level of 0.05 (5%) !!!! To determine whether the result is signi cant we need two more pieces of information: 1. What signi cance level do we want? 0.05 2. How many degrees of freedom are there in the variable? DF = Ncases -1 M&M’s Example on R: The p-value is above 0.05, that means that the di erence between the M&M’s observed and the M&M’s expected is not signi cant. fi fi fi fi fi fi ff fi ff In this case the degree of freedom is 5 because the Number of cases is 6 ( six types of M&M’s) -1 = 5. LECTURE 4 Central limit theorem: The sampling distribution of the sample means approaches a normal distribution as the sample size gets larger. Levels of measurement, summary table Two-samples hypothesis testing Dependent variables - the variables we are interested in, that we try to explain/predict. They DEPEND, by some law or rule, upon other, independent variables. Independent —> dependent Which factors —> what are we cause this? trying to explain? Hypothesis testing phases: 1. Assumptions: type of data, randomization, population distribution, sample size condition 2. Hypothesis: null hypothesis, H0 (parameter value for “no e ect”), alternative hypothesis, Ha (alternative parameter values) 3. Test statistic: compares point estimate to H0 parameter value 4. P-value: weight of evidence against H0, If it’s smaller than 0.05 then it’s signi cantly di erent. 5. Conclusion: report and interpret P-value, make formal decision Measures of association: Measures of association - a single summarizing number that re ects how strongly related two variables are. There are several characteristics of a good measure of association. - They range from a value of 0 ( i.e, no relationship) to 1 (i.e, the strongest possible relationship) - For variables that have an underlying order from low to high they can be positive or negative - Provides the strength of a relationship, indicates the usefulness of predicting Choosing appropriate MoA: 1. Level of measurement? - nominal —> Chisq test example m&m’s - Ordinal —> Chisq test - Continuous —> t.test/ z.test example the political choice of students 2. Table dimension? - r=c=2 - R=c>2 - R!=c>2 - R, c > 5 Chi - Squared When having one sample —> Two-samples chi squared ff fl fi ff Examples of two samples chi squared test: Limitations of Chi-Squared Chi-sq tells us whether the relationship is statistically signi cant or not (two samples). Or whether the di erence between O and E is statistically signi cant or not (one sample) But Chi-sq does NOT tell us how strong the relationships are and this is because Chi-sq is highly sensitive and in uenced by the sample size. Implications: 1. Reasonably strong association may not come up as signi cant if the sample size is small, and conversely. 2. In large samples, we may nd statistical signi cance when the ndings are small and uninteresting. Example: Solution: remove e ect of sample size ! How? By dividing by X and creating the “children” of Chi-sq ff fl ff fi fi fi fi fi fi First: The Phi Coe cient It can only be used to test relation between variables in two-by-two tables—> R = C = 2 It tells us how strong the association between two variables is and obviously is only for nominals variables since it can be used only is 2x2 tables. If the result of Phi is 0 that means that there is no association, if the result is -/+ 1 there’s a weak association, if the result is -/+2 moderate , if the result is -/+3 the association is strong and if the result is more than 3 is very strong. The Phi removes the e ect of the sample size on the Chi-sq by dividing the result by the sample size. Second: Contingency Coe cient Table is larger than a 2x2 but the number of the rows and the number of columns is always the same —> R = C > 2 Used for nominal variables. It takes the Chi-sq and manipulates it; if the result is 0 there is no relationship between variables, if the result is -/+ 1 there’s a weak association, if the result is -/+2 medium , if the result is -/+3 the association is strong and if the result is more than 3 is very strong. ffi ff ffi Third: Cramér’s V It’s used for more than 2x2 and when the number or rows and columns is not the same—> R! = C Based on Chi-sq, we also have n so we get rid of the sample size. If the result is 0 then there is not relationship, if the result is -/+ 1 there’s a weak association, if the result is -/+2 medium , if the result is -/+3 the association is strong and if the result is more than 3 is very strong. Proportional Reduction of Error: PRE Statistical criterion which quanti es the extent that knowledge about one variable can help us predict another variable. Idea to reduce the error. Gamma: only used for ordinal level of measurement. It is based on a distribution; the problem tho is that it in ates the relationship, so we need tau. fi fl Gamma looks at concordant pairs and not at observations. In ates the size of its own weight. Concordant pairs - all possible pairs where one individual is higher on both variables than the other. We multiply the highest cell value with the concordance call value together and we obtain the number of concordances. Discordances pairs - all possible where one individual is higher on one variable but lower on the other. Tau-B —> R = C Very similar to gamma. For ordinal level of measurement. When there is the same amount of rows and columns. fl Tau-C —> R! = C Number of rows and columns is not the same. For ordinal level of measurement. SUMMARIZING ASSIGNMENT 3: Summarizing Histograms will show data for 1 variable, uses continuous variables (it will understand already what numbers you will use and wont need additional information) Bar chart ( rst you need to create a summary of the variables, you need to rst group the numbers and create frequencies) Boxplot Chi-square test (expected, p cannot be higher for one cause its the proportion / probability and observed values). If there’s a signi cance then we reject the null hypothesis. The mu stand for population mean. scatterplot -> plot (you can see what kind of a relationship there is ) cor.test - (to get the Pearsons coe cient) rcorr (correlation tables are printed as a matrix, df -> name of the dataset, we exclude the nominal variables and the type was the pearson's coe cient). MoA divided in 2 sections: 1. Categorical data —> Nominal and Ordinal 2. Continuous data —> Interval and Ratio CATEGORICAL DATA: each of them also divided in families. the head of the families are: Nominal —> Chi-Sq Ordinal —> Gamma Each of these heads have the role to nd the p-value. Each of these heads have children: The children of Chi-sq are: Phi, CC, and Cramer’s V The children of Gamma are: Tau-b and Tau-C LECTURE 5 Dependencies One sample distribution: we will have an observed distribution and an hypothesized distribution Two samples distribution: we have an independent variable and a dependent variable The more the circles share the greater the strength is. fi fi ffi fi ffi fi Example. WHAT IS THE RELATIONSHIP BETWEEN CITY-POPULATION AND THE NUMBER OF CONCERTS PLANNED HELD IN 2023? Dependent variable —> always in the X axes —> # concerts Independent variable —> always in the Y axes —> population size The greater the population the greater the number of concerts SCATTERPLOTS - a graph that shows the relationship between two continuous variables. Scatterplots allow us to visually inspect associations between variables. Each individual (x,y) pair is plotted as a single point with the Cartesian coordinates (x,y). Explanatory (independent) variable-predictor variable; plotted to the horizontal axis (x-axis), outcome (dependent) variable - a value explained by the explanatory variable; plotted on the vertical axis (y-axis). Relationships Negative relation: The higher x is, the lower y is (pic) Positive relation: the higher x is, the higher y is (pic) No relation: our null hypothesis pertains to this scenario —> orthogonal relationship CORRELATION: - correlation is a statistical measure of the relationship between two variables - Used to test hypotheses pertaining to relationship between variables - Best used in variables that demonstrate a linear relationships, between each other using a scatterplot, we can eyeball the relationship between variables - Can take any values from -1 to 1 Correlation Coe cients is the value indicating: 1. The strength of relationship: -1≤ r ≥ 1 2. The direction of relationship -/+ 3. The statistical signi cance p < 0.05 Spearman’s rho formula —> for ordinal variables Pearson’s rho formula —> for continuous variables SPEARMAN’S RANK CORRELATION COEFFICIENT Example: what is the relationship between distance from city center and price of beer? PEARSON PRODUCT - MOMENT CORRELATION COEFFICIENT Pearson product - moment correlation coe cient: Non parametric measure of the strength and direction of association that exists between two variables measured on at least an interval scale. Shows the linear relationship between two sets of data. In simple terms, it answers the question “can I draw line graph to represent the data?” Denoted by the symbol rp or the Greek letter p (rho) LIMITATIONS OF PEARSON’S: - correlation is only useful to identify LINEAR relationships - Correlation does not allow us to go beyond the given data - A strong correlation does NOT imply cause and e ect relationship CORRELATION ≠ CAUSATION - Y CAUSES X (INVERSE CAUSATION) - Z CAUSES BOTH X AND Y - X CAUSES Y AND Y CAUSES X (BIDIRECTIONAL) - X CAUSES Z AND CAUSES Y (CAUSATION PATH) ffi fi ffi ff LECTURE 6 Pearson and Spearman are part of the PRE family - what if we have more than one variable that a ects a dependent variable? Examples: - health situation: genetics, diet exercise, accessibility to doctors and medications, wealth(?) - Internet penetration in society: modernization, economic performance, democracy level, corruption, social con ict. REGRESSION ANALYSIS It’s an approach used to determine the strength and character of the relationship between one dependent variable and one or more independent variables. It's also useful to predict the value of the DV based on the value(s) of other variables. - one explanatory (independent) variable: simple regression - Mare than one explanatory (variable) : multiple regression We have a constant and a multiplier – In order to calculate the dependent variable – the constant plus the multiplier times the independent variable (x). Sometimes plus E, the error. Linear regression estimates the degree to which the variation in one variable x, is related to or can explained by the variation in another variable y. Two motions to use linear regression: 1. Test hypothesis 2. Forecast Dependent variable (ÿ) = constant + multiplier x independent variable (x) When calculating (y) = constant + multiplier x independent variable (x) + error. REGRESSION ANALYSIS : EXAMPLE 3 The error term (e) is the di erence between the observed value of the dependent variable and the value of the dependent variable predicted by a regression line. This line minimizes the sum of the squared error terms —> this line is BLUE. fl ff ff Finding Beta 1 Finding Beta 0: DV —> dependent variable IV —> independent variable The error term is the di erence between the observed value of the dependent variable and the value of the dependent variable predicted by a regression line (blue line). Blue is the line that we can possibly draw in a scatterplot. This line will reduce the error that’s why we call it a PRE model. B = best L = linear U = unbiased E = estimate How strong is the model? We need a numeric, to determine the strength – We look at adjusted R- squared to nd out whether it is weak or strong. ( adjusted R-squared = is a statistical measure used to evaluate the goodness of t of a regression model, particularly when comparing models with di erent numbers of predictors (IVs) ) F-statistics tell us whether our model is better than just guessing – based on chi-sq, it gives us the signi cance but not how strong the relationship is – We look at p-value – if statistically signi cant then it is meaningful to us. fi ff fi fi ff fi Coe cient of determination. (Adjusted R-squared). - asymmetric PRE measure of association which indicates how well data points t a line or curve. - Simply: the proportion of the variance in the dependent variable that is predictable from the independent variable. - provides a measure of how well observed outcomes are replicated by the model. - By looking at R-squared we can determine the strength of the model – weak, moderate, strong, very strong. - A perfect prediction would be 1 – 100%. - It illustrates how much of the variable we can actually explain and if we use it, we can say something meaningful about it. - It is also called ‘explained variable’ – every variable has a certain dispersion – there is a way of variance around the mean, some sort of dispersion. 1. Look at the p-value of the predictor– in order to check whether the predictor is statistically signi cant or not. 2. Look at the relationship through adjusted R-squared. 3. Look at the entire model (F-squared) and check whether or not the model is statistically signi cant or not – if it is a good prediction or not. How good is it? 4. Look at the percentage of the adjusted R-squared that indicates the percentage of the predictor’s variance. MULTIPLE REGRESSION ANALYSIS Multivariate analysis – based on more than two variables – highly in uenced by the level of measurement. Multiple regression – using the OLS Add another piece of information to the equation for the regression analysis. Y = constant + multiplier x Independent variable + another multiplier x its Independent variable and so on The di erence between multiple regression and simple regression is that in this one we have an extra coe cient and another variable – when using multiple regression, we are checking if having more information in the equation would improve the result. There is no limit for the number of predictions – but it better to try explaining things in the simplest way. ffi fi ff ffi fi fl fi In order to nd out which variable is the most important between the two – they are all measured with di erent scales – it is necessary to look at the level of measurement – compare the standardized coe cient on R. EXAMPLE 3: BIVARIATE RELATIONS: A bivariate relation refers to a relationship between two variables. In statistical and mathematical contexts, it involves examining how changes in one variable correspond to changes in another. Here are some key aspects: - Variables: In a bivariate relation, you typically have two variables, often denoted as X and Y - Types of Relationships: Positive Relationship: When one variable increases, the other also tends to increase (e.g., height and weight). Negative Relationship: When one variable increases, the other tends to decrease (e.g., hours spent studying and number of errors on a test). No Relationship: Changes in one variable do not a ect the other. In statistical analysis techniques such as correlation and regression analysis are used to quantify the strength and nature of the relationship between the two variables. In graphical representation they can be visualized using scatter plots, where each point represents a pair of values for the two variables. ff fi ffi ff