Stats Study Guide PDF
Document Details
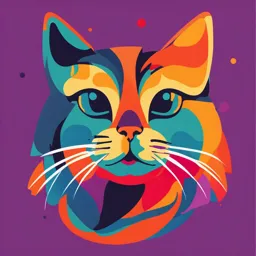
Uploaded by DeadOnMaxwell8869
Tags
Summary
This document is a study guide on statistics, covering topics such as population, samples, parameters, and statistics. It discusses descriptive and inferential statistics, different types of sampling, and data distributions, including quantitative and qualitative data. The guide also explains concepts such as skewness, standard deviation, percentiles, and the interquartile range (IQR).
Full Transcript
xpopulation: entire group to be studied census: collect data for every individual in a population parameter: numerical summary of the population sample: subset of the population for which we actually collect data statistic: numerical summary of a sample. used to estimate parameter...
xpopulation: entire group to be studied census: collect data for every individual in a population parameter: numerical summary of the population sample: subset of the population for which we actually collect data statistic: numerical summary of a sample. used to estimate parameter individuals: entities that we measure in a study (people of objects descriptive statistics: methods for summarizing the collected data. describe data through tables, graphs, & numerical summaries such as averages or percentages. allows researcher to obtain overview of the data & can help detemrine type of statistical mehtods researcher should use Inferential statistics: method that takes a result from a sample, extend it to the population, and meausre the reliablity of result. The accuracy of a generalization always contain uncertainty. this is why results should inculde measure of reliablity. process of statistics: 1) identify research objective, 2) collect data needed to answer ?s posed, 3) describe data, 4) perform inference variable: any characteristics of individuals distribution: tells what values it takes & how often it takes those values Quantitative: numerical measures of individuals - continuous: measured data, can have infinity values within possible range (temp, height, weight, speed, etc) - discrete: observation can only exist at limited values, of ten counts (# of things, no decimals!) - histogram: the hieght of each rectnagle is the frequnecy or relative frequency of the class. (class width= (largest data value-smallest data value)/# of classes) dot plot stem and leaf plot: stem is all digits except last box plot Qualitative: categorical - classification of individuals based on characteristic nominal: name of things ordinal: represent categories and order/ranking) binary: “yes or no” frequency distribution: lists each category of data & the number of observation in each relative frequency distribution: lists each category of data together with the relative frequency, the proportion of observation in each category. the relative frequency is found by taking the frequency for a particular category & dividing by the total # of observations pie charts, bar graph, pareto chart experiment: researcher assigns the individuals in the study to certain treatments & then observes outcomes on the response variable of interest observational study: researcher observes & records the behavior of the individuals in the study without imposing treatments on participants. (lurking variables can affect results, leading to misinterpretation simple random sampling: gives each subject in population the same chance to be in the sample stratified sampling: divides the population into separate groups, called Strats, then selects a random sample from each stratum, Individuals within each stratum should be homogenous. cluster smapling: divides popualtion tino large # of clusters, such as city blocks. Then a simple random of cluster is selected, & all individuals in selected clusters are included systematic sampling: done by selecting every kth individual from a popualtion. the first individual selcted corresponds to a random # between 1 & k bad sampling methods convience smapling: smaple done based on convinces (includes self-selected individuals) Bias sampling bias: favor one part of the population nonrepsonse bias: individuals in sample to no respond response bias: exists when the answers on a survery do not reflect the true opinions of the respondent, perhaps because of the questions or because the way in which the interviewer asks the quesition is confusing or misleading Left skewed - mean is greater than the median Right skewed - median is greater than the mean use median and IQR for skewed unimodal: if a graph contains a single peak bimodal: if a graph contains two peaks uniform: each class is relatively the same height bell-shaped: symmetric use mean and standard deviation or range for symmetric describe distribution shape: symmetry or skewness, # of peaks, clusters or gaps, and outliers center: mean or median. (median is most resistant to outliers) spread: the spread (or dispersion) of the ditribution describes the variablity in the data. clusters or spread out standard deviation: a measure of how spread out the numbers in a data vlaue set are the sqaure root of the variance = STDV if S=0 then all data is the same number percentiles: the p-th percentile means that p% of the observations fall below this value, and (100-p)% fall above it IQR Q1: 25th percentile Q2: 50th percentile Q3: 7th percentile order data from smallest to largest find the median find the median of the data below the median (Q1) and again for data above the median (Q3) IQR = Q3 - Q1 1.5(IQR) rule for outliers lower fence: Q1 - 1.5(IQR) upper fence: Q3 + 1.5(IQR) Five number summary minimum Q1 median Q3 maximum scatter diagrams response variable: (y) measures the outcome explanatory variable: (x) explains or influences change in the variable a graph showing relationship between two QUANTITATIVE variables a scatter diagram with a straight line trend shows a linear relationship between x and y positive association: as x increases, y also tends to increase (/) negative association: as x increases, y tends to decrease (\) no association: as x increases, there is no clear pattern in the changes of y linear correlation coefficient the linear coefficient, denoted by r, is a number between -1 & 1 that describes the direction, form, and strength of the relationship between two quantitative variables 1. direction: r>0 --> x&y have positive association (0.5). r x&y have negative association (-0.5) 2. form: the correction r measures only linear relationships. a value of r close to 0 indicates that the relationship id not linear 3. Strength: the closer r is to +/ 1, the stronger the linear relationship. the closer r is to 0, the weaker the relationship. the stronger the relationship, the easier it is to see a straight line trend in the scatter diagram corretion between x&y = rxy values of x w/in the sample = Xi same with Yi Correlation coefficient determine the absolute value of the correlation coefficient if the absolute value of the correlation coe. is greater than the critical value, we say a linear relation exists between the two variable. otherwise no linear relation exists correlation: measures the strength and direction of relationship between 2 variables however, correlation alone doesn’t imply that one variable cause the other causation: caustion means that one variable directly affects or causes a chnage in another vaible. this is a stronger relationship than correlation and suggests a cause-and-effect connection line that has small residuals minimize the sum of magnitudes (absolute values) of residuals minimize the sum of squared residuals - least squares Least squares regression on scatterplot & linear correlation coefficient show that two variables have a linear relationship, we can find a linear equation (called regression line) to describe this relationship. the least square regression line minimizes the sum of the squared residuals, making it the best-fitting line for the data regression line: used to predict the value of the response variable (y) based on a given value of the explanatory variable (x). this means we can estimate the value of y for any known value of x. the regression line should not be used to predict values of y for x-values outside the collected data or is outside the observed data range least-squares regression line equation ○ Bo=the y-intercept, representing the predicted vlaue of y when x=0 ○ Bi= the slope, indication how much y changes for each unit increase in x (include on aver. when inter.!!) y intercept limitations: the y-intercept might not be meaingful if x=0 is not reasonable or is outside the observed data range residuals a residuals is the predicitoon error for any goven value of x formula: residual=observation(y)-predicted(y) every data point has a residual. some residuals are postitive, some are negative in a scatterplot, the residual is the vertical distance between a data point and the regression line the smaller this distance, the better the prediction limitations of regression models approximation: the regression model predicts the average value of y, not the exact value, for given x influence of other variables: the value of y may also be influenced by other factors, such as gender, age, or activity level, in addition to x random variation: even with multiple variables in the model (multiple regression), there will still be some unexplained random variation in y due to factors that cannot be modeled line of means: the regression line predicts the mean value of y for all individuals with a specific x value probability: refers to the proportion of times a particular outcome would occur in a long run of observations probability is concerned with measuring the chances of possible outcomes for random phenomena, the many things in life for which the outcome is uncertain small samples can misleading with only a few trials, the outcome might seem surprising for example, flipping a coin four times may result in four heads in a row, but this doesn’t mean the coin is unfair the description of a probability experiment (called probability model) includes two parts: a list of all the possible outcomes and a probability for each outcome. sample space: of a probability experiment (denoted s) is the set of all possible outcomes the law of large numbers: the more times you do it the closer the average results will be to the expected probability tree diagram: lisitng all possible outcomes with branches showing what can happen on each of the different tasks replacement & without replacement examples: jar with 1 red, 1 black, 1 white marble ○ with replacement: (3^2) = 9 outcomes n^? ○ without replacement: (3(2)) = 9 outcomes n(n-1) listing outcomes - order of selection What if an experiment invovles selcting objects from a collection? this is a common probability experiment, one example being selecting a random sample of people from a population ○ first, take not of whether the selection is done with replacement or without replacement, as this will affect the number of ways an individual task can be done ○ second, take not of whether the order selection is important, that is, whether we care which object was selected first, which was selected second, and so on (if order is important, the AB & BA are considered different outcomes) event: any collection of outcomes from a probability experiment events with only 1 outcome are called simple events and are denoted e in general, events are denoted using capital letters