PSCI 2702 Chapter 3: Measures of Central Tendency and Dispersion PDF
Document Details
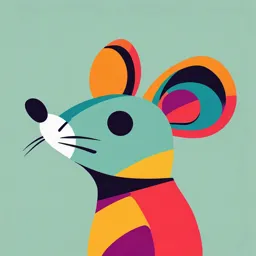
Uploaded by ConscientiousEvergreenForest1127
Toronto Metropolitan University
Tags
Summary
This is a chapter on measures of central tendency and dispersion in the field of statistics. It explores how frequency distributions, graphs, and charts summarize the shape of data distributions and the importance of reporting detailed information. The chapter also introduces examples to demonstrate the concept of dispersion.
Full Transcript
Book Title: eTextbook: Statistics: A Tool for Social Research and Data Analysis, Fifth Canadian Edition Chapter 3. Measures of Central Tendency and Dispersion 3.1. Introduction 3.1. Introduction 80 One clear benefit of frequency distributions, graphs, and charts is...
Book Title: eTextbook: Statistics: A Tool for Social Research and Data Analysis, Fifth Canadian Edition Chapter 3. Measures of Central Tendency and Dispersion 3.1. Introduction 3.1. Introduction 80 One clear benefit of frequency distributions, graphs, and charts is that they summarize the overall shape of a distribution of scores in a way that can be quickly comprehended. Often, however, you will need to report more detailed information about the distribution. Specifically, two additional kinds of statistics are almost always useful: some idea of the typical or average case in the distribution (e.g., “the average starting salary for social workers is $49,000 per year”), and some idea of how much variety or heterogeneity there is in the distribution (“In this province, starting salaries for social workers range from $43,000 per year to $55,000 per year”). These two kinds of statistics, the subjects of this chapter, are referred to as measures of central tendency and measures of dispersion, respectively. The three commonly used measures of central tendency—mode, median, and mean—are all probably familiar to you. All three summarize an entire distribution of scores by describing the most common score (the mode), the middle score (the median), or the average of the scores (the mean) of that distribution. These statistics are powerful because they can reduce huge arrays of data to a single, easily understood number. Remember that the central purpose of descriptive statistics is to summarize or reduce data. Nonetheless, measures of central tendency by themselves cannot summarize data completely. For a full description of a distribution of scores, measures of central tendency must be paired with measures of dispersion. While measures of central tendency are designed to locate the typical and/or central scores, measures of dispersion provide information about the amount of variety, diversity, or heterogeneity within a distribution of scores. The importance of the concept of dispersion might be easier to grasp if we consider an example. Suppose that a sociology professor wants to evaluate the different styles of final exams that they administered last semester to students in the two sections of the Introduction to Sociology course. Students in Section A of the course received an essay-style final exam, while students in Section B received a multiple-choice final exam. As part of the investigation, the professor calculated that the mean exam score was 65% for students who wrote the essay-style exam and 65% for those who wrote the multiple-choice exam. The average exam score was the same, which provides no basis for judging if scores for one exam style were less or more diverse than scores for the other exam style. Measures of dispersion, however, can reveal substantial differences in the underlying distributions even when the measures of central tendency are equivalent. For example, consider Figure 3.1, which displays the distribution of exam scores for students in each section of the course in the form of histograms (see Chapter 2). 81 Figure 3.1 Exam Scores for Two Styles of Exams Compare the shapes of these two figures. Note that the histogram of exam scores for students who wrote the multiple-choice exam (Section B) is much flatter than the histogram of exam scores for students who wrote the essay exam (Section A). This is because the scores for students who wrote the multiple-choice exam are more spread out or more diverse than the scores for students who wrote the essay exam. In other words, the scores for the multiple- choice exam are much more variable and there are more scores in the high and low ranges and fewer in the middle than there are on the essay exam. The essay exam scores are more similar to one another and are clustered around the mean. Both distributions have the same “average” score, but there is considerably more “variation” or dispersion in the scores for the multiple- choice exam. If you were the professor, would you be more likely to select an exam style for which students receive similar exam results (essay exam) or one for which some students receive very low scores and some other students very high scores (multiple-choice exam)? Note that if we had not considered dispersion, a possibly important difference in the performance of students writing the two different types of exams might have gone unnoticed. Keep the two shapes in Figure 3.1 in mind as visual representations of the concept of dispersion. The greater clustering of scores around the mean in the distribution in the upper part of the figure (essay exam distribution) indicates 82 less dispersion, and the flatter curve of the distribution in the lower part of the figure (multiple-choice exam distribution) indicates more variety or dispersion. Each of the measures of dispersion discussed in this chapter—index of qualitative variation, range, interquartile range, variance, standard deviation, and coefficient of variation—tends to increase in value as distributions become flatter (as the scores become more dispersed). As with the three measures of central tendency, choosing which of the measures of dispersion to use depends on how the variables are measured. Indeed, the importance of the level of measurement of the variable to the appropriate selection of measures of central tendency and dispersion cannot be understated. For this reason, our discussion of central tendency and dispersion in this chapter has been organized according to whether you are working with a nominal, an ordinal, or an interval-ratio variable. So, for example, the mode and index of qualitative variation are most appropriate for nominal-level variables. The median, range, and interquartile range can be used with variables measured at either the ordinal or interval-ratio level; however, the mean, variance, standard deviation, and coefficient of variation are more appropriate for interval-ratio-level variables. Book Title: eTextbook: Statistics: A Tool for Social Research and Data Analysis, Fifth Canadian Edition 3.2. Nominal-Level Measures 3.2. Nominal-Level Measures Mode We begin our consideration of measures of central tendency with the mode. The mode of any distribution is the value that occurs most frequently. For example, in the set of scores 58, 82, 82, 90, 98, the mode is 82 because it occurs twice and the other scores occur only once. The mode is, relatively speaking, a rather simple statistic, most useful when you want a quick and easy indicator of central tendency or when you are working with nominal-level variables. In fact, the mode is the only measure of central tendency that can be used with nominal-level variables. Such variables do not, of course, have numerical “scores” per se, and the mode of a nominally measured variable is its largest category. For example, Table 3.1 reports the method of travel to work for a hypothetical sample of 100 workers. The mode 83 of this distribution, the single largest category, is “Automobile.” Table 3.1 Method of Travel to Work If a researcher wants to report only the most popular or common value of a distribution, or if the variable under consideration is nominal, then the mode is the appropriate measure of central tendency. However, keep in mind that the mode does have limitations. First, some distributions have no mode at all (see Table 2.5) or have so many modes that the statistic loses all meaning. Second, with ordinal and interval-ratio data, the modal score may not be central to the distribution as a whole. That is, most common does not necessarily mean “typical” in the sense of identifying the centre of the distribution. For example, consider the rather unusual (but not impossible) distribution of scores on an exam as illustrated in Table 3.2. The mode of the distribution is 93. Is this score very close to the majority of the scores? If the instructor summarized this distribution by reporting only the modal score, would they be conveying an accurate picture of the distribution as a whole? Table 3.2 A Distribution of Exam Scores Monkey Business Images/Shutterstock.com The mode is the most common value in a distribution or the largest category of a variable. For example, data for the method of travel to work show that the single largest category—the mode—is “Automobile.” Book Title: eTextbook: Statistics: A Tool for Social Research and Data Analysis, Fifth Canadian Edition 3.2. Nominal-Level Measures Index of Qualitative Variation Index of Qualitative Variation We begin our consideration of measures of dispersion with the index of qualitative variation (IQV). This statistic is the only measure of dispersion available for nominal-level variables, although it can be used with ordinal-level variables. The IQV is the ratio of the amount of variation actually observed in a distribution of scores to the maximum variation that could exist in that distribution. The index varies from 0.00 (no variation) to 1.00 (maximum variation). 84 To illustrate the logic of this statistic, let’s consider demographic changes in the Indigenous population in Canada, which has grown faster than the non- Indigenous population in part due to higher fertility rates. Table 3.3 presents data on the size of Indigenous and non-Indigenous populations for 1996, 2006, and 2016. (Note that the values in the table are percentages instead of frequencies, since this will greatly simplify computations.) If there were no diversity in the population (e.g., if everyone were Indigenous, or alternatively, if everyone were non-Indigenous), the IQV would be 0.00. At the other end of the scale, if the Canadian population was distributed equally across the two groups (i.e., if each group made up exactly 50% of the population), the IQV would achieve its maximum value (1.00). Table 3.3 Indigenous Identity in Canada, 1996, 2006, 2016 Source: Statistics Canada, 1996, 2006, and 2016 Census of Population By inspection, you can see that the Canadian population is becoming more diverse over time. Indigenous Peoples made up about 4.9% of the population in 2016 compared to 2.8% in 1996. Let’s see how the IQV substantiates these observations. The computational formula for the IQV is Formula 3.1 k(1002 − ∑ pct2) IQV = 1002(k − 1) where k = the number of variable response categories ∑ pct2 = the sum of the squared percentages of cases in the variable response categories To use this formula, the sum (∑) of the squared percentages (P ct2) must first be computed. (This means, of course, that our frequency distribution must include a column for the valid percentages.) So, we add a column for the 85 squared percentages (%2) to our frequency distribution, and we sum this column. This procedure is illustrated in Table 3.4. Table 3.4 Finding the Sum of the Squared Percentages 1996 2006 201 Identity % %2 % %2 % Indigenous 2.80 7.84 3.75 14.06 4.86 Non- 97.20 9,447.84 96.25 9,264.06 95.14 Indigenous Total = 100.00 100.00 100.00 ∑ P ct2 = 9,455.68 9,278.12 For each year, the square of 100 is 10,000, and the sum of the squared percentages (∑ P ct2) is the total of the second column. Substituting these values into Formula 3.1 for the year 1996, we have an IQV of 0.109: 2(10,000.00 − 9,455.68) 2(544.32) IQV = = = 0.109 10,000.00(1) 10,000.00(1) Because the values of k and 100 squared are the same for all three years, the IQV for the remaining years can be found by simply changing the values for ∑ P ct2. For 2006, 2(10,000.00 − 9,278.12) 2(721.88) IQV = = = 0.144 10,000.00(1) 10,000.00 and similarly, for 2016, 2(10,000.00 − 9,075.24) 2(924.76) IQV = = = 0.185 10,000.00(1) 10,000.00(1) Thus, the IQV, in a quantitative and precise way, substantiates our earlier impressions. Canada is growing more demographically diverse in terms of Indigenous Peoples. The IQV of 0.109 for the year 1996 means that the distribution of frequencies shows 10.9% of the maximum variation possible for the distribution. By 2016, the variation increased to 18.5% of the maximum variation possible. Canada grew increasingly diverse in Indigenous composition from 1996 to 2016. In summary, the IQV shows us that dispersion can be quantified even in nominal-level variables. The larger the IQV, the more dispersed the data are for that variable, and the smaller the IQV, the more similar the data. (For practice calculating and interpreting the IQV, see Problems 3.2 and 3.6.) 86 One Step at a Time Finding the Index of Qualitative Variation (IQV) 1: Ensure that your frequency distribution table includes a valid percentage column. 2: Add a squared percentage column, square the valid percentage values, and then enter them in this column. 3: Sum the squared percentages (∑ P ct2). 4: Count the number of valid variable response categories (k). 5: Enter the k and P ct2 values into the IQV formula, and compute the IQV. Book Title: eTextbook: Statistics: A Tool for Social Research and Data Analysis, Fifth Canadian Edition 3.3. Ordinal-Level Measures 3.3. Ordinal-Level Measures Median The median (Md) is a measure of central tendency that represents the exact centre of a distribution of scores. The median is the score of the case that is in the exact middle of a distribution: half the cases have scores higher and half the cases have scores lower than the case with the median score. If the median family income for a community is $75,000, then half the families earn more than $75,000 and half earn less. Before finding the median, we must place the cases in order from the highest to the lowest score—or from the lowest to the highest. Once this is done, we find the central or middle case. The median is the score associated with that case. When the number of cases (n) is odd, the value of the median is unambiguous because there is always a middle case. With an even number of cases, however, there are two middle cases; in this situation, the median is defined as the score exactly halfway between the scores of the two middle cases. To illustrate, assume that seven students are asked to indicate their level of support for the interuniversity athletic program at their university on a scale ranging from 10 (indicating great support) to 0 (no support). After arranging their responses from high to low, you can find the median by locating the case that divides the distribution into two equal halves. With a total of seven cases, the middle case is the fourth case, because there are three cases above and three cases below the fourth case. If the seven scores are 10, 10, 8, 7, 5, 4, and 2, then the median is 7, the score of the fourth case. To summarize: When n is odd, find the middle case by adding 1 to n and then dividing that sum by 2. With an n of 7, the median is the score associated with the [(7 + 1)/2] th , or fourth, case. If n is 21, the median is the score associated with the [(21 + 1)/2] th , or 11th, case. Now, if we make n an even number (8) by adding a student to the sample whose support for athletics is measured as a 1, we no longer have a single 87 middle case. The ordered distribution of scores is now 10, 10, 8, 7, 5, 4, 2, 1; any value between 7 and 5 technically satisfies the definition of a median (i.e., splits the distribution into two equal halves of four cases each). This ambiguity is resolved by defining the median as the average of the scores of the two middle cases. In the example above, the median is defined as (7 + 5)/2 , or 6. To summarize: To identify the two middle cases when n is an even number, divide n by 2 to find the first middle case and then increase that number by 1 to find the second middle case. In the example above with eight cases, the first middle case is the fourth case (n/2 = 4) and the second middle case is the [(n/2) + 1] th , or fifth, case. If n is 142, the first middle case is the 71st case and the second middle case is the 72nd case. Remember that the median is defined as the average of the scores associated with the two middle cases. 88 One Step at a Time Finding the Median 1: Array the scores in order from high score to low score. 2: Count the number of scores to see if n is odd or even. 3: The median is the score of the middle case. 4: To find the middle case, add 1 to n and divide by 2. 5: The value you calculated in step 4 is the number of the middle case. The median is the score of this case. For example, if n = 13 , the median is the score of the [(13 + 1)/2] th , or seventh, case. 3: The median is halfway between the scores of the two middle cases. 4: To find the first middle case, divide n by 2. 5: To find the second middle case, increase the value you computed in step 4 by 1. 6: Find the scores of the two middle cases. Add the scores together and divide by 2. The result is the median. For example, if n = 14 , the median is the score halfway between the scores of the seventh and eighth cases. 7: If the middle cases have the same score, that score is defined as the median. The procedures for finding the median are stated in general terms in the One Step at a Time box. It is important to emphasize that since the median requires that scores be ranked from high to low, it cannot be calculated for variables measured at the nominal level. Remember that the scores of nominal-level variables cannot be ordered or ranked: the scores are different from each other but do not form a mathematical scale of any sort. The median can be found for either ordinal or interval-ratio data, but it is generally more appropriate for ordinal-level data. Book Title: eTextbook: Statistics: A Tool for Social Research and Data Analysis, Fifth Canadian Edition 3.3. Ordinal-Level Measures Range and Interquartile Range Range and Interquartile Range The range (R), defined as the difference or interval between the highest score (H) and the lowest score (L) in a distribution, provides a quick and general notion of variability for variables measured at either the ordinal or the interval-ratio level. The mathematical formula for the range is Formula 3.2 R=H−L where R = the range H = the highest score L = the lowest score Unfortunately, since it is based on only the two most extreme scores in a distribution, R is misleading as a measure of dispersion if just one of these scores is either exceptionally high or exceptionally low. Such scores are often referred to as outliers. The interquartile range (Q) is a type of range that avoids this problem. The interquartile range is the distance from the third quartile (Q3) to the first quartile (Q1) of a distribution of scores. The mathematical formula for the interquartile range is Formula 3.3 Q = Q3 − Q1 where Q = the interquartile range Q3 = the value of the third quartile Q1 = the value of the first quartile 89 The first quartile, Q1 , is the point below which 25% of the cases fall and above which 75% of the cases fall. The third quartile, Q3 , is the point below which 75% of the cases fall and above which 25% of the cases fall. If line LH represents a distribution of scores, the first and third quartiles and the interquartile range are located as shown: Thus, Q is the range of the middle 50% of the cases in a distribution. Since it uses only the middle 50%, Q is not influenced by outliers. To illustrate the computation of the range and the interquartile range, let’s consider the following set of 11 scores: 5, 8, 10, 12, 15, 18, 21, 22, 24, 26, and 99. These scores are ordered from low to high, though placing them in order from high to low will produce identical results. Either way, an ordered distribution of scores makes the range easy to calculate and is necessary for finding the interquartile range. To find the range, R, for this set of scores, the lowest score (L) is subtracted from the highest score (H). Therefore, R=H−L = 99 − 5 = 94 To calculate Q, we must locate the first and third quartiles ( Q1 and Q3 ). To find the quartiles, first find the median for the ordered data (see the One Step at a Time: Finding the Median box). Next, divide the data so that there is an equal number of cases above and below the median. Finally, find the median of the lower and upper parts of the data. Q1 is equal to the median of the lower half of the data and Q3 to the median of the upper half. Q equals the difference between Q3 and Q1. A step-by-step guide for finding the interquartile range is provided in the One Step at a Time box. One Step at a Time Finding the Interquartile Range (Q) 1: Array the scores in order from low to high. For example, 2, 4, 6, 8, 10, 12, 14, 16, 18, 20, 22, 24. 2: Find the median of the data (see the One Step at a Time: Finding the Median box). Continuing with the example data in step 1, Md = (12 + 14)/2 = 13. 3: Divide the ordered data into two equal parts at the median. Continuing this example, the lower half = 2, 4, 6, 8, 10, 12 and the upper half = 14, 16, 18, 20, 22, 24. 4: Find the median of the lower half of the data. (This value is equal to Q1.) Continuing this example, Md of 2, 4, 6, 8, 10, 12 = (6 + 8)/2 = 7. 5: Find the median of the upper half of the data. (This value is equal to Q3.) Continuing this example, Md of 14, 16, 18, 20, 22, 24 = (18 + 20)/2 = 19. 6: Subtract Q1 from Q3. (This value is equal to Q.) Finishing the example, Q = 19 − 7 = 12. Continuing with the example above, we first divide the ordered data into two equal parts at the median (Md = 18). The scores in the lower half are 5, 8, 10, 12, and 15, and the scores in the upper half are 21, 22, 24, 26, and 99. We then find the median of the lower half of the data, or Q1 , which equals 10, and the median of the upper half of the data, or Q3 , which equals 24. We subtract Q1 from Q3 to find the interquartile range. Therefore, Q = Q3 − Q1 = 24 − 10 = 14 90 Clearly, the interquartile range (Q = 14) is preferred to the range (R = 94) as a measure of dispersion for this example, because it eliminates the influence of outliers and provides more accurate information about the amount of diversity within the distribution. As another example, consider the following set of four scores: 2, 3, 8, and 9. R for this data set is 9 − 2 , or 7. To calculate Q, the data set is divided into two equal parts at the median ( Md = 5.5 or [3 + 8]/2 ), so scores 2 and 3 make up the lower half of the data set and scores 8 and 9 the upper half. The median of the lower half, Q1 , is 2.5, or (2 + 3)/2 , and the median of the upper half, Q3 , is 8.5, or (8 + 9)/2. Hence, Q equals 8.5 − 2.5 , or 6. In conclusion, the range and interquartile range provide a measure of dispersion based on just two scores from a set of data. These statistics require that scores be ranked from low to high—or from high to low—so that they can be calculated for variables measured at either the ordinal or the interval-ratio level. Since almost any sizable distribution will contain some atypically high and low scores, the interquartile range is a more useful measure of dispersion than the range because it is not affected by extreme scores, or outliers. In the next section, we will look at a graphical device used for displaying range and interquartile range information for ordinal and interval-ratio variables. (The median, R, and Q may be found for any ordinal or interval-ratio variable in the problems at the end of this chapter.) Book Title: eTextbook: Statistics: A Tool for Social Research and Data Analysis, Fifth Canadian Edition Chapter 3. Measures of Central Tendency and Dispersion 3.4. Visualizing Dispersion: Boxplots 3.4. Visualizing Dispersion: Boxplots Together with the median, the range and interquartile range can be represented in a graph known as the boxplot, giving us a helpful way to visualize both central tendency and variability. It has the advantage of 91 conveniently displaying the centre, spread, and overall range of scores in a distribution and can be used with variables measured at either the ordinal or the interval-ratio level. The boxplot is based on information from a five-number summary, consisting of the lowest score, first quartile, second quartile (the median), third quartile, and highest score in a distribution. To construct a boxplot requires a few steps. First, we calculate the first, second (the median), and third quartiles. Second, we draw a box between the first and third quartiles. (The height of the box is arbitrary but should be reasonably proportional to the rest of the graph.) Third, we draw a vertical line dividing the box at the median value. Fourth, we draw a horizontal line (whisker) from the lowest score, indicated by a small vertical line, to the box. We do the same for the highest score to the box. To illustrate, Table 3.5 presents data from the OECD (Organisation for Economic Co-operation and Development) on total (public and private) health care spending in 26 countries. These countries spent at least $3,000 USD per person on health care in 2019. Countries are ranked from highest to lowest in 92 the table. To facilitate international comparisons, each country’s currency was converted to US dollars using the 2019 purchasing power parity, or PPP, exchange rate. The PPP exchange rate is a ratio (see Chapter 2) of the cost of an identical set of goods and services in two countries. Because the PPP exchange rate considers a wide range of products and services within countries and is therefore less vulnerable to market fluctuations, it is preferred over conventional exchange rates when doing cross-country comparisons. Further, as with the OECD health data, it is common to express PPP in US dollars, or the ratio of the prices paid for the same group of goods and services in the US and another country in its own currency. Table 3.5 Total Health Care Expenditure per Person, 2019 Rank Country Expenditure $ (US PPP dollars) 26 (highest) United States 11,072 25 Switzerland 7,732 24 Norway 6,647 23 Germany 6,646 22 Austria 5,851 21 Sweden 5,782 20 Netherlands 5,765 19 Denmark 5,568 18 Luxembourg 5,558 17 Belgium 5,428 16 Canada 5,418 15 France 5,376 14 Ireland 5,276 13 Australia 5,187 12 Japan 4,823 11 Iceland 4,811 10 United Kingdom 4,653 9 Finland 4,578 8 Malta 4,262 7 New Zealand 4,204 6 Italy 3,649 5 Spain 3,616 4 Czech Republic 3,428 Rank Country Expenditure $ (US PPP dollars) 3 Korea 3,384 2 Portugal 3,379 1 (lowest) Slovenia 3,224 Source: OECD, https://data.oecd.org/healthres/health-spending.htm. Since there is an even number of scores, the median (Q2) is the score located halfway between the two middle cases, or between cases 13 (Australia) and 14 (Ireland). Given that the 2019 per capita health expenditures in Australia and Ireland were $5,187 and $5,276, respectively, the median is $5,187 + $5,276 $10,463 = = $5,231.50 2 2 Next, we find the first quartile (Q1) and the third quartile (Q3). When we divide the ordered scores into two equal parts at the median (Q2) , there is an odd number of scores (13) below the median, and an odd number of scores (13) above the median. The median of each of these individual sets of scores is the score of the , or seventh, case in each set. The seventh case below 13 + 1 2 Q2 is New Zealand, with a 2019 per capita health expenditure of $4,204, so Q1 = $4,204. The seventh case above Q2 is Netherlands, with an expenditure of $5,765, so Q3 = $5,765. (We can easily calculate the interquartile range with this information: Q = Q3 − Q1 = $5,765 − $4,204 = $1,561.) We still need the lowest (L) and highest (H) scores to construct our boxplot. It is of course easiest to recognize these scores in an ordered list. Of the 26 countries whose 2019 per capita health care spending was at least $3,000, the United States spent the most ($11,072) and Slovenia spent the least ($3,224). Thus, the highest and lowest scores in our boxplot, represented by the small vertical lines, are $11,072 and $3,224, respectively. (We can now also easily calculate the range: R = H − L = $11,072 − $3,224 = $7,848.) With the values of the five-number summary at hand (L, Q1 , Q2 , Q3 , and H), we are ready to construct our boxplot. We draw a box between the first and third quartiles (i.e., between $4,204 and $5,765), and a vertical line dividing the box at the median value (i.e., at $5,231.50). Then we draw a horizontal line (whisker) from the lowest score (i.e., $3,224), indicated by a small vertical line, to the box, and a line from the largest score (i.e., $11,072), also indicated by a small vertical line, to the box. Figure 3.2 displays a boxplot of the data provided in Table 3.5. Figure 3.2 Boxplot: Total Health Care Expenditure per Person, 2019. Based on data from Table 3.5. L: Low score ($3,224); Q1 : First quartile ($4,204); Q2 : Second quartile or median ($5,231.50); Q3 : Third quartile ($5,765); H: High score ($11,072); Q: Interquartile range ($1,561); R: Range ($7,848). At a quick glance, the boxplot in Figure 3.2 shows us that the length of the box is considerably shorter than the length of the whiskers. In other words, Q (i.e., 93 $1,561) is much less than R (i.e., $7,848). Recall that R is significantly affected by outliers, or unusually high or low scores. A useful feature of the interquartile range, Q, is that it can help us detect low or high outliers. An outlier is commonly defined as a score more than 1.5Q above Q3 or below Q1. Hence, the lower outlier boundary is Q1 − 1.5Q = $4,204 − 1.5($1,561) = $1,962.50 and the upper outlier boundary is Q3 + 1.5Q = $5, 765 + 1.5($1, 561) = $8, 106.50 So, any score less than $1,862.50 is a low outlier, and any score more than $8,106.50 is a high outlier. Using this definition and referring to Table 3.5, the United States ($11,072) is the only outlier in this data set. The boxplot can also provide useful information on how scores are distributed. Notice that the median, Q2 , represented by the vertical line within the box, is closer to the right side of the box, Q3 , than it is to the left side of the box, Q1. This tells us that the 25% of cases located between Q2 and Q3 are both less spread out and more concentrated in the higher score range than are the 25% of cases located between Q1 and Q2. As you can see, the boxplot is a highly informative and useful graph for ordinal-level and interval-ratio-level variables. Book Title: eTextbook: Statistics: A Tool for Social Research and Data Analysis, Fifth Canadian Edition 3.5. Interval-Ratio-Level Measures 3.5. Interval-Ratio-Level Measures Mean The mean ( X , read as “ex-bar”), or arithmetic average, is by far the most ¯ commonly used measure of central tendency. It reports the average score of a distribution, and its calculation is straightforward: to compute the mean, add 94 the scores and then divide by the number of scores (n). To illustrate: A family- planning clinic administered a 20-item test of general knowledge about contraception to 10 clients. The number of correct responses (the scores of the 10 clients) was 2, 10, 15, 11, 9, 16, 18, 10, 11, and 7. To find the mean of this distribution, add the scores (total = 109) and divide by the number of scores (10). The result (10.90) is the average number of correct responses on the test. The mathematical formula for the mean is Formula 3.4 ∑ Xi ¯ X= n where ¯ X = the sample mean ∑ Xi = the sum of the scores n = the number of cases in the sample Let us take a moment to consider this formula. As noted in Formula 3.1, the symbol ∑ stands for “the summation of” and tells us to add the quantities immediately following it; in this case Xi. The symbol Xi (read this as “X sub i”) refers to any single score—or the ith score. If we wish to refer to a particular score in the distribution, the specific number of the score replaces the subscript. So, X1 refers to the first score, X2 to the second, X26 to the 26th, and so forth. Together, the operation of summing (∑) all the scores (ith) is symbolized as ∑ Xi. In plain language, this combination of symbols directs us to sum the scores, beginning with the first score and ending with the last score in the distribution. Formula 3.4 states in symbols what we can say in words (to calculate the mean, add the scores and divide by the number of scores), but in a very succinct and precise way. One Step at a Time Finding the Mean 1: Add up the scores (Xi). 2: Divide the quantity you found in step 1 (∑ Xi) by n (for a sample) or by N (for a population). It is important to point out that Formula 3.4, strictly speaking, is for the mean of a sample. The mean of a population is calculated using the same steps, but it is symbolized by the Greek letter mu, μ (pronounced “mew”), as shown in Formula 3.5. 95 Formula 3.5 ∑ Xi μ= N where μ = the mean ∑ Xi = the summation of the scores N = the number of cases in the population. Also note that to differentiate between population measures, technically called population parameters, and sample measures, called sample statistics, it is common practice to use Greek letters for parameters and Roman letters for statistics. So, for example, the Greek letter μ represents the population mean and the Roman letter X represents the sample mean. It is also customary to ¯ symbolize “number of cases” with the uppercase letter N in formulas for population parameters (e.g., Formula 3.5) and the lowercase letter n in formulas for sample statistics (e.g., Formula 3.4). These practices are followed throughout this textbook. Book Title: eTextbook: Statistics: A Tool for Social Research and Data Analysis, Fifth Canadian Edition 3.5. Interval-Ratio-Level Measures Some Characteristics of the Mean Some Characteristics of the Mean The mean is the most commonly used measure of central tendency, and we will consider its mathematical and statistical characteristics in some detail. First, the mean is always the centre of any distribution of scores in the sense that it is the point around which all of the scores cancel out. Symbolically, ¯ ∑(Xi − X ) = 0 Or, if we take each score in a distribution, subtract the mean from it, and add all of the differences, the resultant sum will always be zero. To illustrate, consider the following sample of scores: 10, 20, 30, 40, and 50. The mean of these five scores is 150/5, or 30, and the sum of the differences is presented in Table 3.6. 96 Table 3.6 A Demonstration Showing That All Scores on One Side of the Mean Are Equally Weighted to All Scores on the Other Side of the Mean The total of the negative differences (−30) is exactly equal to the total of the positive differences (+30) , and this will always be the case. This algebraic relationship between the scores and the mean indicates that the mean is a good descriptive measure of the centrality of scores. You may think of the mean as a fulcrum on a seesaw that exactly balances all of the scores. A second characteristic of the mean is called the least-squares principle, a characteristic that is expressed in this statement : 2 ¯ ∑ (Xi − X ) = minimum This indicates that the mean is the point in a distribution around which the variation of the scores (as indicated by the squared differences) is minimized. If the differences between the scores and the mean are squared and then added, the resultant sum will be less than the sum of the squared differences between the scores and any other point in the distribution. To illustrate this principle, consider the distribution of the five sample scores mentioned above: 10, 20, 30, 40, and 50. The differences between the scores and the mean have already been found. As illustrated in Table 3.7, if we square and sum these differences, we get a total of 1,000. If we perform that same mathematical operation with any number other than the mean—say the value 31—the resultant sum is greater than 1,000. Table 3.7 illustrates this point by showing that the sum of the squared differences around 31 is 1,005, a value greater than 1,000. Table 3.7 A Demonstration That the Mean Is the Point of Minimized Variation In a sense, the least-squares principle merely underlines the fact that the mean is closer to all of the scores than the other measures of central tendency. However, this characteristic of the mean is also the foundation of some of the most important techniques in statistics, including the variance and standard deviation. The final important characteristic of the mean is that every score in the distribution affects it. The mode (which is only the most common score) and 97 the median (which deals only with the score of the middle case or cases) are not affected in this way. This quality is both an advantage and a disadvantage. On the one hand, the mean uses all of the available information—every score in the distribution affects the mean. On the other hand, when a distribution has a few very high or very low scores (as noted in Section 3.3, these extreme scores are often referred to as outliers), the mean may become a very misleading measure of centrality. To illustrate, consider again the sample of five scores mentioned above: 10, 20, 30, 40, and 50. Both the mean and the median of this distribution are 30(X = 150/5 = 30; Md = score of third case = 30). What happens if ¯ we change the last score from 50 to 500? This change does not affect the median at all; it remains at 30 because the median is based only on the score of the middle case. The mean, in contrast, is very much affected because it takes all scores into account. The mean becomes 600/5, or 120. Clearly, the one extreme score in the data set disproportionately affects the mean. For a distribution that has a few scores much higher or lower than the other scores, the mean may present a very misleading picture of the typical or central score. The general principle to remember is that, relative to the median, the mean is always pulled in the direction of extreme scores. The mean and the median have the same value when and only when a distribution is symmetrical. When a distribution has some extremely high scores (this is called a positive skew), the mean always has a greater numerical value than the median. If the distribution has some very low scores (a negative skew), the mean is lower in value than the median. So, a quick comparison of the median and the mean always tells you if a distribution is skewed and the direction of the skew. If the mean is less than the median, the distribution has a negative skew. If the mean is greater than the median, the distribution has a positive skew. Figures 3.3, 3.4, and 3.5 depict three different histograms that demonstrate these relationships. As you can see, symmetry and skew are very important characteristics of distribution shape. More will be said about distribution shape in later chapters. Figure 3.3 A Positively Skewed Distribution Figure 3.4 A Negatively Skewed Distribution Figure 3.5 An Unskewed, Symmetrical Distribution So, which measure is most appropriate for each distribution? If the distribution is highly skewed (as depicted in Figures 3.3 and 3.4), the mean no longer 98 describes the typical or central score. Hence, the median should be used. If the distribution is unskewed, as shown in Figure 3.5, either measure can be used. As a final note regarding the mean, since its computation requires addition and division, it should only be used with variables measured at the interval-ratio level. Book Title: eTextbook: Statistics: A Tool for Social Research and Data Analysis, Fifth Canadian Edition 3.5. Interval-Ratio-Level Measures Variance and Standard Deviation Variance and Standard Deviation In Section 3.3, we explored the range and interquartile range as measures of dispersion. A basic limitation of both statistics, however, is that they are based on only two scores. They do not use all the scores in the distribution, and in this sense, they do not capitalize on all the available information. Also, neither statistic provides any information on how far the scores are from one another or from some central point such as the mean. How can we design a measure of dispersion that corrects these faults? We can begin with some specifications: a good measure of dispersion should do the following: 1. Use all the scores in the distribution. The statistic should use all the information available. 99 2. Describe the average or typical deviation of the scores. The statistic should give us an idea of how far the scores are from one another or from the centre of the distribution. 3. Increase in value as the distribution of scores becomes more diverse. This is a very handy feature because it permits us to tell at a glance which distribution is more variable: the higher the numerical value of the statistic, the greater the dispersion. One way to develop a statistic to meet these criteria is to start with the distance between each score and the mean. The distances between the scores and the mean are technically called deviations, and this quantity increases in value as the scores increase in their variety or heterogeneity. If the scores are more clustered around the mean (remember the graph for essay-style exam scores [Section A] in Figure 3.1), the deviations are small. If the scores are more spread out or more varied (like the scores for multiple-choice exams [Section B] in Figure 3.1), the deviations are greater in value. How can we use the deviations of the scores around the mean to develop a useful statistic? One course of action is to use the sum of the deviations as the basis for a statistic, but as we saw earlier in this section (see Table 3.6), the sum of the deviations is always zero. Still, the sum of the deviations is a logical basis for a statistic that measures the amount of variety in a set of scores, and statisticians have developed two ways around the fact that the positive deviations always equal the negative deviations. Both solutions eliminate the negative signs. The first does so by using the absolute values or by ignoring signs when summing the deviations. This is the basis for a statistic called the mean deviation, a measure of dispersion that is rarely used and will not be mentioned further. The second solution squares each of the deviations. This makes all values positive because a negative number multiplied by a negative number becomes positive. For example, Table 3.8 lists the sample of five scores from Table 3.6, along with the deviations and the squared deviations. The sum of the squared deviations (400 + 100 + 0 + 100 + 400) equals 1,000. Thus, a statistic 100 based on the sum of the squared deviations has many of the properties we want in a good measure of dispersion. Table 3.8 Computing the Standard Deviation Before we finish designing our measure of dispersion, we must deal with another problem. The sum of the squared deviations increases with the number of cases: the larger the number of cases, the greater the value of the measure. This makes it very difficult to compare the relative variability of distributions with different numbers of cases. We can solve this problem by dividing the sum of the squared deviations by the number of cases and thus standardizing for distributions of different sizes. These procedures yield a statistic known as the variance, which is symbolized as s2 for a sample and σ2 for a population. The variance is used primarily in inferential statistics, although it is a central concept in the design of some measures of association. For the purposes of describing the dispersion of a distribution, a closely related statistic called the standard deviation (symbolized as s for a sample and σ for a population) is typically used, and this statistic is our focus for the remainder of this section. Let’s first look at the formulas for the sample variance and the standard deviation: Formula 3.6 2 ¯ ∑ (Xi − X ) 2 s = n Formula 3.7 2 ¯ ∑ (Xi − X ) s= √ n where Xi = the score ¯ X = the sample mean n = the number of cases in the sample To compute the standard deviation, it is advisable to construct a table like Table 3.8 to organize computations. The five scores are listed in the left-hand column, the deviations are in the middle column, and the squared deviations are in the right-hand column. The sum of the last column in Table 3.8 is the sum of the squared deviations and can be substituted into the numerator of the formula: 2 ¯ ∑ (Xi − X ) s= √ n 1, 000 =√ 5 = √200 = 14.14 101 To finish solving the formula, divide the sum of the squared deviations by n and take the square root of the result. To find the variance, square the standard deviation. For this problem, the variance is s2 = 14.142 = 200. It is important to point out that some electronic calculators and statistical software packages (including SPSS) use a slightly different formula, with n−1 instead of n in the denominator to calculate the sample variance and standard deviation. This n − 1 is called Bessel’s correction, and it corrects for the underestimation of the population variance (s2) and standard deviation (s) that would occur if only n were used. Instead, we choose to correct for this bias at later points in the textbook—for example, in Chapter 6 when calculating confidence intervals for sample means. Nevertheless, calculators and statistical software packages that use formulas with n − 1 will produce results that are at least slightly different from results produced using Formulas 3.6 and 3.7. The size of the difference will decrease as the sample size increases, but the problems and examples in this chapter use small samples, so the difference between using n and n − 1 in the denominator can be considerable. Some calculators offer the choice of using n − 1 or n in the denominator. If you use n, the values calculated for the sample standard deviation will match the values in this textbook. As a final point, like the mean, the variance and standard deviation for populations are calculated using exactly the same method as for samples, but population and sample measures are distinguished from each other using 102 different symbols. The variance and standard deviation formulas for populations are shown in Formulas 3.8 and 3.9, respectively. Formula 3.8 2 ∑ (Xi − μ)2 σ = N Formula 3.9 2 ∑ (Xi − μ) σ=√ N where Xi = the score μ = the population mean N = the number of cases in the population Applying Statistics 3.1. The Mean and Standard Deviation At a local preschool, 10 children were observed for one hour, and the number of aggressive acts committed by each was recorded in the following list. What are the mean and standard deviation of this distribution? Number of Aggressive Acts 2 ¯ ¯ Xi Xi − X (Xi − X ) 1 1 − 4 = −3 9 3 3 − 4 = −1 1 5 5−4=1 1 2 2 − 4 = −2 4 7 7−4=3 9 11 11 − 4 = 7 49 ¯ ∑ Xi 40 X= = = 4.0 n 10 2 ¯ ∑ (Xi − X ) 118 s= √ =√ = √11.8 = 3.43 n 10 During the study observation period, the children committed on average 4.0 aggressive acts, with a standard deviation of 3.44. Book Title: eTextbook: Statistics: A Tool for Social Research and Data Analysis, Fifth Canadian Edition 3.5. Interval-Ratio-Level Measures Interpreting the Standard Deviation Interpreting the Standard Deviation It is very possible that the meaning of the standard deviation (i.e., why we calculate it) is not completely obvious to you at this point. You might be asking, “Once I’ve gone to the trouble of calculating the standard deviation, what do I have?” The meaning of this measure of dispersion can be expressed in three ways. The first and most important involves the normal curve, and we will defer this interpretation until the next chapter. A second way of thinking about the standard deviation is as an index of variability that increases in value as the distribution becomes more variable. In other words, the standard deviation is higher for more diverse distributions and lower for less diverse distributions. The lowest value the standard deviation can have is zero, which occurs for distributions with no dispersion (i.e., if every single case in the distribution had exactly the same score). Thus, zero is the lowest value possible for the standard deviation, but there is no upper limit. A third way to get a feel for the meaning of the standard deviation is by comparing one distribution with another. You might do this when comparing one group against another or the same variable at two different times or places. For example, data collected over several decades by Environment and Climate Change Canada show that Calgary, Alberta, and Gander, Newfoundland and Labrador, have identical mean daily temperatures for the month of January (−7.1 degrees Celsius, °C) yet experience very different levels of variation in those temperatures: Calgary Gander ¯ ¯ X = −7.1 X = −7.1 s = 4.5 s = 1.8 Source: Environment and Climate Change Canada. https://climate.weather.gc.ca/climate_normals/index_e.html. 103 Gordon Wheaton/Shutterstock.com; Jeff Whyte/Shutterstock.com One way of understanding the standard deviation is to compare one distribution with another, by comparing the same variable at two different times or places, for example, the daily temperatures in January in Calgary, Alberta, and Gander, Newfoundland and Labrador. 104 One Step at a Time Finding the Standard Deviation (s) and the Variance (s2) of a Sample To Begin 1: Construct a computing table like Table 3.8, with columns for the scores (Xi) , the deviations (Xi − X ) , and the deviations squared ¯ [(Xi − X ) ]. 2 ¯ 2: List the scores (Xi) in the left-hand column. Add up the scores and divide by n to find the mean. As a rule, state the mean to two places of accuracy or two digits to the right of the decimal point. To Find the Values Needed to Solve Formula 3.7 1: Find the deviations (Xi − X ) by subtracting the mean from each ¯ score, one at a time. List the deviations in the second column. Generally speaking, you should state the deviations at the same level of accuracy (two places to the right of the decimal point) as the mean. 2: Add up the deviations. The sum must equal zero (within rounding error). If the sum of the deviations does not equal zero, you have made a computational error and need to repeat step 1, perhaps at a higher level of accuracy. 3: Square each deviation and list the result in the third column. 4: Add up the squared deviations listed in the third column. To Solve Formula 3.7 1: Transfer the sum of the squared deviations column to the numerator in Formula 3.7. 2: Divide the sum of the squared deviations (the numerator of the formula) by n. 3: Take the square root of the quantity you computed in the previous step. This is the standard deviation. To Find the Variance (s2) 1: Square the value of the standard deviation (s). Book Title: eTextbook: Statistics: A Tool for Social Research and Data Analysis, Fifth Canadian Edition 3.5. Interval-Ratio-Level Measures Coefficient of Variation Coefficient of Variation The standard deviation is considered an “absolute” measure because it describes dispersion in the units (e.g., years, kilograms) of the variable. The absolute nature of the standard deviation, however, makes it difficult to compare variables with different scales. On the other hand, a “relative” version of the standard deviation, called the coefficient of variation (CV), lets you directly compare the amount of dispersion in two distributions regardless of their scale. For example, you can use the CV to compare the income distribution of two countries with different currencies. The CV is simply the ratio of the standard deviation to its mean, or the standard deviation divided by the mean Formula 3.10 s CV = ¯ X where s = sample standard deviation ¯ X = sample mean Although the CV shows the relative size of the standard deviation, it is interpreted in the same way as the standard deviation: the higher the CV value, the greater the dispersion in the distribution. Likewise, it does not have an upper limit. The CV can be expressed as a percentage to ease interpretation. So, for example, if we examine the distribution of years of education for Canadian adults and find a mean of 15 years and a standard deviation of 5 years, then the CV is s 5 CV = (100%) = (100%) = 0.3333(100%) = 33.33% ¯ X 15 The standard deviation is 33.3% of the size of its mean. In conclusion, the standard deviation is the most important measure of dispersion because of its central role in many more advanced statistical applications. Since the standard deviation (and the coefficient of variation) is based on the mean, it should be used with variables measured at the interval- ratio level. Also, like the mean, the standard deviation uses all the scores in the 105 distribution and thus is disproportionately influenced by outliers and extreme 106 scores. When a distribution has outliers or extreme scores (a highly skewed distribution), the interquartile range should be used as the measure of dispersion. As noted in Section 3.3, the interquartile range uses only the middle 50% of the data in the distribution and is thus resistant to extreme scores. Finally, the coefficient of variation, as a standardized or scaleless measure of dispersion, is preferred to the standard deviation when comparing variables with different scales. (The mean and standard deviation and coefficient of variation may be found for any interval-ratio variable in the problems at the end of this chapter.) Applying Statistics 3.2. Describing Dispersion The percentage of people in the labour force who work part-time in five western Canadian cities and five eastern Canadian cities is compared using data from the 2016 Census. Parttime workers are defined in the census as people who work mainly part-time weeks (29 hours or less per week) on the basis of all jobs held during the year 2015. Columns for the computation of the standard deviation have already been added to the tables below. Which group of cities tends to have a higher percentage of part-time workers? Which group varies the most in terms of this variable? Computations for both the mean and the standard deviation are shown below. Percentage of Labour Force with Part-Time Jobs, 2015, Eastern Cities Percentage of Labour Force with Part-Time Jobs, 2015, Western Cities With such small groups, you can tell by simply inspecting the scores that the western cities have higher percentages of part-time workers in their labour forces. This impression is confirmed by both the median, which is 22.10 for the eastern cities (Quebec City is the middle case) and 23.70 for the western cities (Winnipeg is the middle case), and the mean (21.78 for the eastern cities and 23.62 for the western cities). For both groups, the mean is similar to the median, indicating an unskewed distribution of scores. Also, the five western cities are much more variable and diverse than the eastern cities. The range for the western cities is 6.30 (R = 27.30 − 21.00 = 6.30) , much higher than the range for the eastern cities of 3.30 (R = 23.10 − 19.80 = 3.30). Similarly, the standard deviation for the western cities (2.36) is about twice the value of the standard deviation for the eastern cities (1.10). In summary, the five western cities average higher rates of part-time work and are also more variable than the five eastern cities. Source: Statistics Canada, 2016 Census of Population. Book Title: eTextbook: Statistics: A Tool for Social Research and Data Analysis, Fifth Canadian Edition Chapter 3. Measures of Central Tendency and Dispersion 3.6. Measures of Central Tendency and Dispersion for Grouped Data 3.6. Measures of Central Tendency and Dispersion for Grouped Data The techniques for ordinal and interval-ratio-level variables presented so far are used for ungrouped or raw data. These techniques can be readily extended to grouped, aggregate data in a frequency distribution. In this section, we will compute the median, mean, and standard deviation for sample data grouped in a frequency distribution. Table 3.9 shows a frequency distribution of exam scores for a sample of 25 students. To locate the median more easily, cumulative frequencies have been added to the table. Because the number of cases is odd, the median in this distribution is the score associated with the 13th case, or (25 + 1)/2. By adding frequencies, starting with the lowest score, we see that the 13th case is associated with the score 67. 107 Table 3.9 Computing the Median for Aggregate Data in a Frequency Distribution We can also readily obtain the mean for aggregate data using a slightly modified version of the formula used to find the mean for ungrouped data (see Formula 3.4). The formula for aggregate data is Formula 3.11 ∑(fXi) ¯ X= n where ¯ X = the mean ∑(fXi) = the summation of each score multiplied by its frequency n = the number of cases in the sample Table 3.10 demonstrates the calculation of the mean for the distribution of exam scores described in the previous table. Table 3.10 Computing the Mean for Aggregate Data in a Frequency Distribution The first (Xi) and second (f) columns in the distribution show each score and its frequency, respectively. The third column (fXi) displays the product of multiplying these numbers together. For example, the first score, 58, is multiplied by its frequency, 2, which equals 116. The mean of the frequency distribution (X ) is the sum of each score multiplied by its frequency divided ¯ by the number of cases in the sample (n). ∑(fXi) 1, 758 ¯ X= = = 70.32 n 25 Thus, these 25 students have an average exam score of 70.32. The formula for the standard deviation (s) for aggregate data in a frequency distribution is Formula 3.12 2 ¯ ∑ f(X i − X ) √ s= n where f = the number of cases with a score Xi = the score ¯ X = the mean n = the number of cases Table 3.11 demonstrates the calculation of s. 108 Table 3.11 Computing the Standard Deviation for Aggregate Data in a Frequency Distribution The first (Xi) and second (f) columns in the distribution show each score and its frequency, respectively. The third column (Xi − X ) contains the ¯ deviations, and the fourth column [(Xi − X ) ] contains the squared 2 ¯ deviations. The fifth column [f(Xi − X ) ] displays the product of 2 ¯ multiplying the frequencies and the squared deviations together. Formula 3.12 is solved by taking the square root of the sum of these products, of ∑ f(Xi − X ) , divided by n: 2 ¯ 2 ¯ ∑ f(X i − X ) 3, 483.38 √ s= =√ = √139.34 = 11.80 n 25 Thus, the average deviation of these 25 exam scores from the mean, 70.32, is 11.80. Book Title: eTextbook: Statistics: A Tool for Social Research and Data Analysis, Fifth Canadian Edition Chapter 3. Measures of Central Tendency and Dispersion 3.7. Choosing a Measure of Central Tendency and a Measure of Dispersion 3.7. Choosing a Measure of Central Tendency and a Measure of Dispersion Throughout this chapter, we have emphasized that the selection of a measure of central tendency or dispersion should, in general, be based on the level of measurement, meaning whether you are working with a nominal, an ordinal, or an interval-ratio variable. Table 3.12 summarizes the relationship between the levels of measurement and the measures of central tendency and dispersion. The most appropriate measure of central tendency and dispersion for each level of measurement is in italics. Table 3.12 The Relation between Level of Measurement and Measures of Central Tendency and Dispersion Level of Measure(s) of Measure(s) of Dispersion Measurement Central Tendency Nominal Mode Index of qualitative variation Ordinal Mode, median Index of qualitative variation, range, interquartile range Interval-ratio Mode, median, Index of qualitative mean variation, range, interquartile range, variance, standard deviation We have to keep in mind, however, that each measure of central tendency and measure of dispersion is defined in a unique way. For example, even though 109 they have a common purpose, the three measures of central tendency are quite different from one another and have the same value only under certain, specific conditions (i.e., for symmetrical distributions). So, while your choice of an appropriate measure of central tendency or dispersion generally depends on the way you measure the variable, you must also consider how the variable is distributed. The mean and standard deviation are the most appropriate measures for a variable measured at the interval-ratio level; however, the median and interquartile range should be used if an interval-ratio variable has outliers or extreme scores (i.e., is highly skewed). Because of this, it is therefore also common to report more than one measure of central tendency or dispersion whenever a variable’s level of measurement permits this. 110 111 Reading Statistics 3. Measures of Central Tendency and Dispersion As is the case with frequency distributions, measures of central tendency and dispersion are not always presented in the professional research literature. These reports focus on the relationships between variables, not on describing them one by one. Univariate descriptive statistics are usually just the first step in the data analysis, not the ultimate concern, and probably will not be included in the final publication. On the other hand, some statistics (e.g., the mean and standard deviation) serve a dual function. They not only are valuable descriptive statistics but also form the basis for many analytical techniques. Thus, they may be reported in the latter role if not in the former. When included in research reports, measures of central tendency and dispersion are most often presented in some summary form such as a table. A fragment of such a summary table might look like this: Variable ¯ X s n Age 33.2 1.3 1,078 Number of children 1.5 0.7 1,078 Years married/common-law 7.8 1.5 1,052 Income 75,786 1,600 987 These tables succinctly describe the overall characteristics of the sample, and, if you inspect the table carefully, you will have a good sense of the sample on the traits relevant to the project. Note that the number of cases varies from variable to variable in the preceding table. This is normal in social science research and is caused by missing data or incomplete information for some of the cases. Means, Standard Deviations, and High and Low Scores for Selected Variables: United States versus Canada United States Canada (n = 2,205) (n = 2,253) Variable ¯ ¯ X s X s Low High Warmth −0.06 0.92 0.06 1.06 −4.40 1.20 Emotional −0.11 0.95 0.12 1.03 −4.38 1.39 support Positive −0.11 0.88 0.07 1.06 −3.47 1.43 control Caregiving −0.13 1.05 0.10 0.95 −3.52 2.40 Harsh 0.29 0.69 −0.30 1.16 −0.88 3.17 discipline Masculine 33.03 1.16 28.54 6.58 0 66 norm adherence Statistics in the Professional Literature In this instalment of Reading Statistics, we look at a recent study that examines and compares the relationship between adherence to traditional masculine norms (independent variable) and father involvement (dependent variable) in two countries–Canada and the United States. The authors hypothesize that embracing traditional masculinity is negatively related to father involvement and that this relationship is stronger in the United States than in Canada. Cross-country comparative analysis such as this study provides a valuable research methodology because it allows researchers to investigate the influence of sociocultural and political factors on a given phenomenon such as fathering behaviour. The study uses multiple measures of fathering behaviour and involvement, including participating in caregiving, positive control through monitoring children’s behaviours and actions, harsh disciplinary practices, and warm behaviour and emotional support provided to children. Adherence to traditional masculine norms was measured using a multidimensional scale based on a series of questions about different domains of masculinity, including quest for status and success above all else, limited emotionality, risktaking behaviour, and the desire to control people and social situations. The descriptive statistics (means, standard deviations, and high and low scores) for these variables are reproduced in the table above. As is usually the case, the authors report these statistics as background information and to help identify differences and important patterns. The actual hypotheses in the study are tested with more advanced statistics, some of which are reviewed in Chapter 14. Overall, these basic statistics suggest that fathering behaviours and actions are different in the two countries. Canadian fathers tend to be warmer and are more likely to provide emotional, caregiving, and positive-control support than their US counterparts. They are also less likely to use harsh discipline and to adhere to masculine norms. These differences in fathering behaviour and gender norms point to the importance of societal, cultural, and political forces in shaping parental and family life within each country. Want to find out more about this study? See the following source. Source: Shafer, K., Petts, R., and Scheibling, C. (2021). Variation in masculinities and fathering behaviors: A cross-national comparison of the United States and Canada. Sex Roles, 84, 439–453. Book Title: eTextbook: Statistics: A Tool for Social Research and Data Analysis, Fifth Canadian Edition Chapter 3. Measures of Central Tendency and Dispersion 3.8. Interpreting Statistics: The Central Tendency and Dispersion of Income in Canada 3.8. Interpreting Statistics: The Central Tendency and Dispersion of Income in Canada A sizable volume of statistical material has been introduced in this chapter, so we will conclude by focusing on meaning and interpretation. What can you say after you have calculated, for example, means and standard deviations? Remember that statistics are tools to help us analyze information and answer questions. They never speak for themselves, and they always have to be understood in the context of some research question or test of a hypothesis. This section provides an example of interpretation by posing and answering some questions about the changing distribution of income in Canada: “Is average income rising or falling?” “Does the average Canadian receive more or less income today than in the past?” “Is the distribution of income becoming more unequal (i.e., are the rich getting richer)?” We can answer these questions by looking at changes in measures of central tendency and dispersion. Changes in the mean and median tell us, respectively, about changes in the average income for all Canadians (mean income) and income for the average Canadian (median income). The coefficient of variation (CV) is used to measure dispersion (i.e., the level of inequality) in the distribution of income. The higher the CV value, the greater the dispersion or inequality in the income distribution. SEVENNINE_79/Shutterstock.com We can interpret and extract meaning from statistics as we seek to answer questions and draw conclusions about things such income distribution in Canada. Before considering the data, we should keep in mind that virtually any distribution of income is positively skewed (see Figure 3.3). That is, in any group, locality, province, or nation, the incomes of most people are grouped around the mean or median, but some people—the wealthiest members of the group—have incomes far higher than the average. Because the mean uses all the scores, it is pulled in the direction of the extreme scores relative to the median. In a positively skewed distribution, the mean is greater than the median. Likewise, the CV, which is based on the mean and standard deviation, may be influenced by exceedingly high or low incomes. Also note that income is measured at four points in time (1986, 1996, 2006, and 2016), and only for Canadians working full-time to make the results more comparable from one year to the next. Income is also expressed in 2016 dollars to eliminate changes caused by inflation over the years. Without this adjustment, recent incomes would appear to be much higher than older 112 incomes, not because of increased purchasing power and well-being, but rather because of the changing value of the dollar. Turning to the results, Figure 3.6 shows that the median income for the average full-time worker was about $40,000 in 1986. By 2016, the median income for the average Canadian worker was over $50,000, after recovering from a period of decline between 1996 and 2006. By contrast, mean income increased significantly between 1996 and 2006, suggesting that workers with the highest incomes became more affluent relative to the bulk of the working population over this period. Figure 3.6 Mean, Median, and Coefficient of Variation (CV) for Income of Full-Time Workers, 1986–2016, Canada (in 2016 dollars). Source: Statistics Canada, 1986, 1996, 2006, and 2016 Census of Population, Public Use Microdata Files. A widening gap between the mean and median incomes reflects a growing positive skew in the distribution of income, so we would expect to see that incomes grew more dispersed. Figure 3.6 shows that the coefficient of variation increased considerably between 1996 and 2006, suggesting greater income inequality among Canadian workers. Between 2006 and 2016, mean and median income grew in tandem, and the coefficient of variation decreased marginally. Overall, the findings provide some evidence that people with modest incomes continue to have modest incomes and, consistent with ancient folk wisdom, the rich are getting richer. 113