시스템생물학 PDF 중간범위 PDF
Document Details
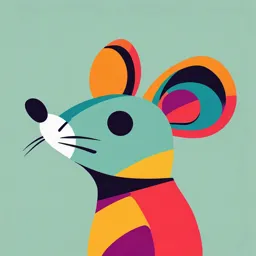
Uploaded by WellConnectedAquamarine
Konkuk University
Sung Ho Yoon
Tags
Summary
이 문서는 시스템생물학 개요 및 다양한 관련 주제를 다룹니다. 시스템생물학의 정의, 시스템, 시스템사고, 생물학적 모델링, 그리고 시스템생물학의 활용을 설명하고 있습니다. 또한, 생물학적 시스템의 다양한 측면을 다루는 모델링의 중요성을 강조합니다.
Full Transcript
같은 일을 반복하면서 다른 결과가 나오기를 기대하는 것은 미친 짓이다. The definition of insanity is doing something over and over again and expecting a different result. - Albert Einstein Ch. 1. Introduction Sung Ho Yoon Dept. Systems Biotechnology, Konkuk University Systems Biology in Drama vod...
같은 일을 반복하면서 다른 결과가 나오기를 기대하는 것은 미친 짓이다. The definition of insanity is doing something over and over again and expecting a different result. - Albert Einstein Ch. 1. Introduction Sung Ho Yoon Dept. Systems Biotechnology, Konkuk University Systems Biology in Drama vod 2 What is Systems Biology? https://www.youtube.com/watch?v=HCFoZDlV4FY vod 3 Systems Biology: A Short Overview https://www.youtube.com/watch?v=vWSsNi5uFVY vod 4 Cycle of Science • Science: systematic endeavor that builds and organizes knowledge in the form of testable explanations and predictions about the universe (Wikipedia) 귀납법 연역법 Prediction Experimentation 5 Paradigm Shift in Biological Research Genetics(유전학) Molecular Biology(분자생물학) Genomics(유전체학) Systems Biology(시스템생물학) Individual study on a few genes/proteins Integrated study on many genes/proteins (omics) Small scale research (individual lab) Large scale research (multidisciplinary team) Single genes All genes Gene Genome Transcript Transcriptome Protein Proteome Metabolite Metabolome 6 Meaning of X-omics What is shown Pervukhin, 2010 7 Genomes to Life 8 New Biology A New Biology for the 21st Century: Ensuring the United States Leads the Coming Biology Revolution (National Research Council, 2009) 9 Can a biologist fix a radio? Lazebnik Y, “Can a biologist fix a radio?” Cancer Cell 2:179 (2002) Biologist’s view of a radio Engineer’s view of a radio 10 The Delphic Boat • The oracle of Delphi asked: “If every plank in a boat is replaced over time, is it still the same boat?” • Biology is now asking: “If every molecule in a cell is replaced over time, is it still the same cell?” “If every cell in an organism is replaced over time, is it still the same organism?” The answer is basically ‘Yes !’ Thus, the interconnections of biological components of cells are taking center stage in biology. And we have the emergence of ‘Systems Biology’ 11 UNESCO World Heritage: Hwaseong fortress (세계문화유산 수원화성) 수원화성 남포루 (1950년대) 625 전쟁 때 대부분 파괴됨 수원화성 남포루 (현재) 화성 건설의 공사 보고서: 화성성역의궤(華城城役儀軌) What is “System”? • A system is any collection of interacting parts with emergent behavior. • Interactions of multiple elements can lead to behavior that is not immediately evident from the behavior of the single elements → Emergent properties 13 Systems Thinking • A discipline for seeing wholes rather than parts to understand the complex system vod https://www.youtube.com/watch ?v=FW6MXqzeg7M vod https://www.youtube.com/watch?v= AP7hMdnNrH4 14 Systemic Approach • Systemic: system-wide • Systematic : carefully planned processes • Reactions in an organism do not occur isolated! • Biological processes are the result of complex and dynamic interactions within and between cells, organs and entire organisms • Emergence is when the whole is greater than the sum of the parts (esp. when the system behavior is a nonlinear function of its variables ) The whole is more than the sum of its parts 15 Systems Biology – Backers & Attackers Volume 17 | Issue 19 | 27 Oct. 6, 2003, The Scientist 16 Systems Biology • Systems biology views biology as dynamic networks of interactions Predictive Network Biology • A systems view of disease postulates that disease arises from diseaseperturbed networks Non-Diseased Diseased 17 Systems Biology • Systems Biology – Study of the mechanisms underlying complex biological processes as integrated systems of many interacting components. (Leroy Hood) – System-level understanding of a biological processes and network (Kitano) – Study of the interactions between the components of biological systems, and how these interactions give rise to the function and behavior of that system (e.g. enzyme, metabolites in a metabolic pathway) (Wikipedia) – Study of an organism (or individual cells or organs) viewed as an integrated and interacting network of genes, proteins and biochemical reaction (Institute for Systems Biology) • Try to understand the “system” – Experimentally: massive data gathering and data mining (e.g. genome projects) – Conceptually: modeling and analyzing networks (i.e. interactions) of components 18 Why Systems Biology? • Biology has traditionally (and extremely successfully!) focused on what individual parts of a cell do. • But functionality of a system is determined crucially by the interactions of the parts. • Fundamental biological processes are dynamic. – Cell growth/division/differentiation – Metabolism – Signaling • Many diseases are caused by malfunctioning of the dynamic bionetworks. 19 Life is a Network ! 20 Life is a Networkn !! Life is an emergent property of the interconnections of biological components. Complexity in cellular networks of E. coli Metabolic network Protein network Regulatory network Genetic network 21 Systems Biology is Where Computer Science, Engineering and Biology Meet Components Methodology Kitano, 2002 22 Systems Biology !? • “Any sufficiently advanced technology is indistinguishable from magic” (Arthur C Clarke) • Is Systems Biology/Modeling: – an “Esoteric Knowledge”? – the way to understand biological systems? – a tool to solve practical problems? 23 1.1 Biology in Time and Space • Biological systems such as organisms, cells, or biomolecules are highly organized in their structure and function. Hierarchical (or multi-scalar levels) of biological information 24 Length and Time Scales in Biology • Biological entities and their properties can be described at different levels of organization and different time scales. Figure 1.1 Length and time scales in biology 25 Separation of Time Scales • Gene expression consists of events in different time scales 1. The input signals usually activate transcription factors (TFs) on a sub-second time scale. 2. Binding of an active TF to its DNA biding site reaches equilibrium in seconds. 3. Transcription and translation takes minutes. 4. Accumulation of the protein takes many minutes to hours. Hence, 1 and 2 can be considered instantaneous when studying transcription networks. • Typical times scales in E. coli: 1. From input signal to TF activation: 1 msec 2. Binding active TF to its DNA site: 1 sec 3. Transcription + translation of the gene: 5 min 4. 50% change of protein concentration: 1 h 26 1.2 Models and Modeling • Model – Abstraction of the reality – Simplified (mathematical) representations of some real-world entity (e.g. biological processes in an organism), in equations or computer code – Summary of established knowledge about a system in a coherent mathematical formulation – Mimic some essential features of the study system while leaving out inessentials • Modeling – Process of creating and refining a model • Simulation – Manipulation of a model in such a way that it operates on time or space to predict how the system works 27 How-To Biochemical modeling Slide from Dr. Jürgen 28 Pahle How-To Metabolic Modeling 29 Typical Abstraction Steps in Mathematical Modeling Figure 1.2 (a) Biological system: E. coli produce thousands of different proteins. (b) Simplified mental model: Cells contain two enzymes of interest, X (red) and Y (blue). All other substances are disregarded for the sake of simplicity. (c) Model scheme: Interactions b/w X and Y are denoted in a wiring scheme - Each protein can be produced or degraded (black arrows). - X proteins increase the production of Y proteins. (d) Process model: All individual chemical processes are denoted as mathematical expressions with their rates a (occurrence per time). (e) Dynamic model: Deterministic rate equations for the protein concentrations x and y. (f) Quantitative results (Simulation): Predictions for the time-dependent concentrations by solving the model equations - Model refinement: If the predictions do not agree with experimental data, this indicates that the model is wrong or too much simplified. In both cases, the model has to be refined. Purpose and Adequateness of Models • Modeling is a subjective and selective procedure. • A model represents only specific aspects of reality but, if done properly, this is sufficient since the intention of modeling is to answer particular questions. • Limitation of modeling – Constrained by what we can measure, either to estimate parameters that are part of the model formulation or to validate model predictions – Accuracy of the model is highly dependent on the quality of the experiments used for its building 31 Why mathematical model of biological phenomena? • Impossible experiments become possible • Hypotheses can easily be tested • Other mathematical methods can be applied to an existing model (stability analysis, parameter estimation, etc.) • Models are repositories & means of communicating of biological knowledge or hypotheses • Modelling immediately exposes gaps in the current knowledge • Models can be used to predict and also to study and understand biological processes (exploratory/explanatory models) “I have come to believe that one's knowledge of any dynamical system is deficient unless one knows a valid way to numerically simulate that system on a computer.” D.T. Gillespie Inventor of stochastic simulation algorithm (SSA) 32 Advantages of Computational Modeling • Modeling drives conceptual clarification. – It requires verbal hypotheses to be made specific and conceptually rigorous. – The attempt to formulate current knowledge and open problems in mathematical terms often uncovers a lack of knowledge and requirements for clarification. • Modeling highlights gaps in knowledge or understanding. – During the process of model formulation, unspecified components or interactions have to be determined. – Computational models can be used to test whether proposed explanations of biological phenomena are feasible. • Modeling is cheap compared with experiments. • Models exert no harm on animals or plants – No ethical problems in experiments. No environmental pollution 33 • Modeling can assist experimentation. One may – test different scenarios that are not accessible by experiment. – follow time courses of compounds that cannot be measured in an experiment. – impose perturbations that are not feasible in the real system. – cause precise perturbations without directly changing other system components, which is usually impossible in real systems. • Model results can be presented in precise mathematical terms. – Graphical representation and visualization make it easier to understand the system. • Modeling generates well-founded and testable predictions. • Computational models serve as repositories of current knowledge, both established and hypothetical, about how systems might operate. – They provide researchers with quantitative descriptions of this knowledge and allow them to simulate the biological process, which serves as a 34 rigorous consistency test. 1.3 Basic Notions for Computational Models • A model can only describe certain aspects of the system – All other properties of the system (e.g., concentrations of other substances or the environment of a cell) are neglected or simplified. – It is important – and, to some extent, an art – to construct models in such ways that the disregarded properties do not compromise the basic results of the model. • Models are never “right,” but can be appropriate and helpful. “All models are wrong but some are useful" Box, G.E.P. (1979) ”Robustness in the strategy of scientific model building” in Robustness in Statistics (R.L. Launer and G.N. Wilkinson, Eds.), Academic Press “The practical question is how wrong do they have to be to not be useful" Box, G.E.P. & Draper, N.R. (1987). Empirical ModelBuilding and Response Surfaces. Wiley. pp. 74 35 From cells to models Slide from Dr. Jürgen 36 Pahle • Model Statements – A model contains various kinds of statements and equations describing facts about the model elements. – In kinetic models, the dynamics is determined by a set of ordinary differential equations (ODEs) describing the substance balances. – Statements in other model types may have the form of equality or inequality constraints (e.g., in flux balance analysis), maximality postulates, stochastic processes, or probabilistic statements about quantities that vary in time or between cells. 37 Garbage in, Garbage out Image Credit – The Daily Omnivore 38 System state • State variables are a set of variables used to – summarize the properties of interest in the study system – predict how those properties will change over time – e.g. protein concentrations, expression of genes • A system is characterized by its state, a snapshot of the system at a given time. • Each modeling framework defines its own state of the system. – Kinetic rate equation model: a list of substance concentrations – Stochastic model: a probability distribution or a list of the current number of molecules of a species – Boolean model of gene regulation: a string of bits indicating for each gene whether it is expressed (“1”) or not expressed (“0”) 39 Variables, Parameters, and Constants • The quantities in a model can be classified as variables, parameters, and constants – y = a x2 + b + 3 – y = ekx – X = X0et • Constant – Quantity with a fixed value – e.g. the natural number e, Avogadro's number (number of molecules per mole) 40 • Parameter – Quantity with a given value – e.g. Km value of an enzyme in a reaction. • Variable – Quantity with a changeable value for which the model establishes relations – The state variables describe the system behavior completely. – State of the system is a set of values for all variables • Examples – the diameter d and volume V of a sphere obey the relation, V = πd3/6, where π and 6 are constants, V and d are variables, but only one of them is a state variable since the relation between them uniquely determines the other one. • Whether a quantity is a variable or a parameter depends on the model. 41 Model Classification (I) • Structural/Qualitative models – Components and their relations are described, e.g. using a graph representation • Kinetic/Quantitative models – Components and their interactions are assigned precise values, e.g. species concentrations or reaction fluxes. Study of how these values change over time. Slide from Dr. Jürgen 42 Pahle Model Classification (II) • By nature of future states – Deterministic model • the future states are determined by the current state • modeled as ordinary differential equation systems – Stochastic model • the future states are not precisely predetermined but a probability distribution • modeled as random process • By the nature of values – Discrete model: values are discrete – Continuous model: values are continuous 43 Model Assignment Is Not Unique • A biological process can be described with different (mathematical) models. • The choice of a mathematical model or an algorithm to describe a biological object depends on the problem, the purpose, and the intention of the investigator. • Modeling has to reflect essential properties of the system. • Different models may highlight different aspects of the same system. 44 1.4 Networks • Biological networks – – – – – Protein–protein interaction networks Protein–RNA interaction networks Metabolic networks Gene regulatory networks Signaling networks • Networks are best represented by graphs that consist of nodes and edges, which connect the nodes. Figure 1.3 Network with nodes (circles) and edges (lines between circles). Different node colors indicate different types of connected components (e.g., proteins, mRNAs, and metabolites). 45 1.6 Standards for Omics Data and Modeling • Common schemes (community standard data model) for data storage, data representation, and data transfer • X-omics data – Transcriptomics • Minimum Information About a Microarray Experiment (MIAME) – Proteomics • Proteomics experiment data repositories (PEDRo), • Human Proteome Organization consortium (HUPO) Proteomics Standards Initiative (PSI) • Biological models and pathways – XML (extensible markup language)-like language style • Systems Biology Markup Language (SBML) • CellML • Systems Biology Graphical Notation (SBGN) – Modeling & Simulation • Minimum Information Requested in the Annotation of Biochemical Models (MIRIAM) • Minimum Information About a Simulation Experiment (MIASE) 46 1.7. Model Organisms • If the basic biology is similar, it may make sense to study a simple organism rather than a complex one • Model organisms – – – – – Well-studied biology Fit for laboratory study Short life cycle Small, easy to maintain Rapid and reliable growth in a laboratory – Existence of excellent genetic tools – Genome-sequenced Figure 1.4 Model organisms (a) Escherichia coli (prokaryote) (b) The yeast Saccharomy ces cerevisiae (unicellular eukaryote (c) Th e nematode Caenorhabditis elegans (multi cellular organism) (d) The fruit fly Drosophi la melanogaster (multicellular organism in developmental biology) (e) the mouse Mu s musculus (mammals) 47 Recap • Functionality of a system is determined crucially by the interactions of the parts. • Systems Biology views an organism (or individual cells or organs) viewed as an integrated and interacting network of genes, proteins and biochemical reaction. • Biological processes can be modeled as various types and mathematical formalisms. • Model is abstraction of the reality. • Model is not the reality and not certain. • Emergent properties: Interactions of multiple elements can lead to behavior that is not immediately evident from the behavior of the single elements 1 Education is learning what you didn’t even know you didn’t know. - Daniel J. Boorstin Ch. 2. Modeling of Biochemical Systems Sung Ho Yoon Dept. Systems Biotechnology, Konkuk University The Surprisingly Deep Connection of Math and Biology vod https://www.youtube.com/watch?v=qZGsKVMPXos 3 Weather Forecast: Knowledge 4 Weather Forecast: Modeling Mathematical model of major physical interactions related to weather phenomena 5 Modifiied Slide from Dr. Jürgen Pahle Weather Forecast: Simulation 6 Modifiied Slide from Dr. Jürgen Pahle Weather forecast YTN TV (2016.08.21) https://www.youtube.com/watch?v=uAFeOlpaEYA 한 기상 전문가는 "과거 정부의 연구 용역 결과 예보 정확도는 수치예보 모 델 성능이 40%, 모델에 입력되는 기 상 관측 자료가 32%, 예보관 능력이 28%를 차지한다고 분석됐다"며 "아무 리 성능이 뛰어난 장비더라도 예보관 능력이 떨어지면 예보는 틀릴 수 밖에 없다"고 말했다. 연합뉴스 (2016.08.23) 7 Evaluation of a model: How reliable are weather forecasts? 기상청 "예보 정확도 92%"...믿어지십니까? (YTN, 2017.8.22) 강수 예보 정확도 92% → Accuracy 비예보 적중률: 46% → PPV vod https://www.youtube.com/watch?v=JkuuApbK3_s 8 Weather Reality (Truth) forecast No Rain Rain (D) It will not rain (negative, True Negative (TN) False Negative (FN) It will rain (positive, T) False Positive (FP) True Positive (TP) • Accuracy (정확도) (TP+TN)/(TP+FP+FN+TN) – Proportion of true results, either true positive or true negative – 정답을 맞추는 능력 • Positive Predictive Value (PPV) TP/(TP+FP) – Predictive value of a positive test (검사 적중률) – Probability of having the true positive given a positive prediction – 검사에서 양성인 사람 중에서 실제로 양성인 사람의 비율 (확률) 9 Examples of Biochemical Networks • Metabolic networks: transformation of mass of organic molecules • Signaling networks: transfer of information from signaling molecules (e.g. hormones) to targets in the cell • Protein-protein interaction networks: interactions (e.g. binding) between proteins. Often detailed mechanisms or functions are not known. • Gene regulation networks: high-level conceptual descriptions of interactions between genes (also called transcriptional network) Slide from Dr. Jürgen 10 Pahle Need for Mathematical Modelling • Chemical species are constantly transformed by many different reactions building a complex network • Therefore, it is difficult to intuitively figure out how the concentrations of the different species behave over time We need support by mathematical modelling and software tools to do that Slide from Dr. Jürgen 11 Pahle 2.1 Overview of Common Modeling Approaches for Biochemical Systems • Models can be used to predict and also to study and understand biological processes (exploratory/explanatory models) • What question is the model supposed to answer? Is it built to – explain a surprising observation? (explanatory) – relate separate observations with each other and with previous knowledge? (explanatory) – make predictions, for example, about the effect of specific perturbations? (exploratory) • Often, a major function of models is to make assumptions about the underlying process explicit and, hence, testable. 12 • A mathematical model of a biological system can describe very different aspects and, hence very different approaches are employed. • Three major types of computational/mathematical models – Network-based models – Rule-based models – Statistical models 13 Modeling of Biological Process is Iterative 14 Iterative Modelling Cycle Slide from Dr. Jürgen 15 Pahle Model creation / refinement Slide from Dr. Jürgen 16 Pahle Simulation, analysis, parameter scanning/sampling Slide from Dr. Jürgen 17 Pahle Optimization & Parameter fitting Slide from Dr. Jürgen 18 Pahle Procedure of Model Building 1) Define the question that the model shall help to answer. 2) Seek available information: • Read the literature. • Look at the available experimental data. • Talk to experts in the field. 3) Formulate a mental model. 4) Decide on the modeling concept (network-based or rule-based, deterministic or stochastic, etc.) 5) Formulate the first (simple) mathematical model. 6) Test the model performance in comparison to the available data. 7) Refine the model, estimate parameters. 8) Analyze the system (parameter sensitivity, static and temporal behaviors, etc.) 9) Make predictions for scenarios not used to construct the model. (Simulation) • Gene knockout or overexpression • Application of different stimuli or perturbations 10) Compare predictions and experimental results. 19 Interpretation of Simulation Results • If model predictions and the new experimental data are in agreement, the model can – cover correctly important aspects of the described system – be used to make further predictions. • If predictions and experimental tests differ, important aspects of the biological process have not been – understood correctly, – presented appropriately, – or completely ignored • The incorrect model can lead to find – missing links, – alternative explanations, – or better parameter sets to explain the observations 20 2.2 ODE Systems for Biochemical Networks • Systems of ordinary differential equations (ODE) – the most frequently used approach to model the static and dynamic behaviors of biochemical networks • Formulation of an ODE model for a dynamic biochemical reaction network 1) Identify basic building blocks (all compounds and all reactions). 2) Set boundary of the system. 3) Assign kinetic laws to all reactions. 4) Determine the values of the kinetic parameters. 5) Find out whether the system has a steady state or not. 6) Simulate the time course for a given set of parameter values and initial conditions. 7) Analyze the effect of perturbations (e.g knockouts / knockdowns / overexpression of enzymes catalyzing the individual reactions or of knockdowns or overexpression) 21 4.1 Reaction Kinetics and Thermodynamics • Chemical reactions – A process that leads to the transformation of one set of chemical substances to another – Spontaneous ↔ Non-spontaneous (needs energy input) – All chemical reactions in a cell are accompanied by changes in energy • Gibbs free energy (G) – Energy available to work (kJ/mol) – A state function that relates enthalpy (H), entropy (S), and temperature (T): G= H – T S – G0 = Gf0products – Gf0reactants – Measure of spontaneous process • G 0 → non-spontaneous reaction (endergonic: require energy) • G = 0 → equilibrium reaction • G 0 → spontaneous reaction (exergonic: release free energy) 22 Activation Energy • Energy required to bring all molecules in a chemical reaction into the reactive state • Catalysis is required to lower activation energy barrier 23 Reaction Rate 𝑣= 𝐶ℎ𝑎𝑛𝑔𝑒 𝑖𝑛 𝑎𝑚𝑜𝑢𝑛𝑡 𝑜𝑓 𝑟𝑒𝑎𝑐𝑡𝑎𝑛𝑡/𝑝𝑟𝑜𝑑𝑢𝑐𝑡 𝑇𝑖𝑚𝑒 𝑡𝑎𝑘𝑒𝑛 𝑓𝑜𝑟 𝑡ℎ𝑒 𝑐ℎ𝑎𝑔𝑒 𝑣 S1 + S 2 → P 𝑣 S1 + 3S2 → 2P = ∆𝐶𝑜𝑛𝑐. ∆𝑡𝑖𝑚𝑒 = 𝑑𝐶𝑜𝑛𝑐. 𝑑𝑡 𝑑[S1] = −𝑣 𝑑𝑡 𝑑[S2] = −𝑣 𝑑𝑡 𝑑[P] =𝑣 𝑑𝑡 𝑑[S1] = −𝑣 𝑑𝑡 𝑑[S2] = -3v 𝑑𝑡 𝑑[P] = 2v 𝑑𝑡 24 Elementary Reaction https://www.youtube.com/watch?v=0g1YwEbwifw vod 25 Rate Raw: Mass Action Reaction rate (v) ∝ Probability of a collision of the reactants ∝ Conc. reactants to the power of their molecularity (stoichiometry) 2S1 + S2 → P v = k [S1]2[S2] 26 Rate Raw: Michaelis-Menten Kinetics What assumptions? Figure 4.4 27 How-To Model Enzyme Catalysis Fast (pre-) equilibrium approximation [Michaelis and Menten] Kd Kd = k−1 k1 k1ES = k-1ES (Quasi-) Steady-state approximation [Briggs and Haldane] S(0) >> E(0) 28 Steady-State Approximation in Michaelis-Menten Enzyme Kinetics 29 http://www.copasi.org http://copasi.org/ 30 Introduction to COPASI vod https://www.youtube.com/watch?time_continue=8&v=4pH16ema-Lg 31 COPASI Model Creation Noncompetitive inhibition 2nd order (irr) Noncompetitive inhibition (rev) Henri-Michaelis-Menten (irr) https://www.youtube.com/watch?v=Q3IumEn9204 vod 32 Basic Plotting with COPASI vod https://www.youtube.com/watch?time_continue=101&v=qiYLMTsa-_Y 33 Exercises • Task 1: Create “branced.cps” from the copasi lecture video • Task 2: Upload & Simulate the model – Using cps files in COPASI example directory – e.g. C:\Program Files\copasi.org\COPASI 4.24.197\share\copasi\examples • Task 3: Simulate Michaelis-Menten Kinetics – Reaction • v1: E + S = ES , k1 = 0.01 ml/mmol/s, k2 = 0.01 /s • v2: ES -> E + P, k1 = 1 /s – Initial concentration • E, S : 1 mmol/ml • ES, P : 0 mmol/ml 34 Example: Metabolic Model Figure 2.1 Network and dynamics of a metabolic model with different constraints of the variables. Rate laws for all reactions are mass action with rate constant (i.e., ki = 1 (i = 1, ..., 4)). Initial conditions are (a, e) S0(0) = A3(0) = 1 mM and S1(0) = S2(0) = S3(0) = S4(0) = A2(0) = 0 mM (c) S0(0) = 1, A2(0) = A3(0) = 0.5, S1(0) = S2(0) = S3(0) = S4(0) = 0. Constant concentrations are (a) S0, S3, S4 (c) S0, S3, S4, A2, A3 (e) none v1 = k1*S0 v2 = k2*S1*A3 v3 = k3*S2 v4 = k4*S2*A3 35 Recap • Models can be used to predict and also to study and understand biological processes (exploratory/explanatory models). • Three major types of computational models are – Network-based models – Rule-based models – Statistical models • Modeling of biological process is iterative. • Both the qualitative and quantitative behavior of biological systems depend on the structure of the network and on the kinetics of its individual reactions or processes. 1 To ask the right question is harder than to answer it. - Georg Cantor Ch. 3. Structural Modeling and Analysis of Biochemical Networks I (Modeling of Metabolic Network) Sung Ho Yoon Dept. Systems Biotechnology, Konkuk University Computer-Simulation of Metabolic Network vod https://www.youtube.com/watch?v=dchHehGDfkc 3 Contents • • • • • • • • Metabolism (metabolic network) Metabolic flux Mass balance at steady state Reaction stoichiometry matrix (S) Linear algebra Null space Extreme pathways Constraint-based model 4 Metabolism (I) • Metabolism – Totality of all the chemical reactions that operate in a living organism – Produces mass, energy, and redox requirements for all cellular functions • Catabolism (catabolic reactions) – Degradative, energy-generating processes – Breakdown and produce energy • Anabolism (anabolic reactions) – Biosynthetic, energy-consuming processes – Use energy and build up cell components (e.g. proteins, nucleic acids, amino acids) • ATP Biomolecules Mechanical works .... Nutrients ADP Catabolic reaction (exergonic) Anabolic reaction (endergonic) ATP CO2, NH3, H2O Simple products, precursors – Universal carrier of metabolic energy – Linking catabolic and anabolic pathways 5 Metabolism (II) 6 Metabolism (III) - Central Carbon Metabolism Emmerling et al., 2002 7 Metabolic Network • Metabolite – Intermediates and products of metabolism – An organic substance (e.g. glucose, oxygen) – Usually small molecules • Biochemical reaction – Process in which two or more molecules (reactants) interact, usually with the help of an enzyme, and produce a product – Most of the reactions are catalyzed by enzymes (proteins) • Metabolic pathway (one of biochemical pathways) – Sequence of biochemical reaction steps connecting a specified set of input and output metabolites by enzymes enzyme1 enzyme2 enzyme3 – Characteristics • • • • Complex Execute particular functions Flexible and redundant Conserved, but can adjust A B C D metabolite • Metabolic network: network of interacting metabolites through biochemical8 reactions Metabolic Network: Collection of Metabolic Pathways 9 Metabolic map representation of Escherichia coli metabolic genotype • Gray: Alternative carbon source metabolism • Light gray: The core metabolic pathways • Orange: Amino acid biosynthesis • Green: Vitamin and cofactor metabolism • Yellow: Nucleotide synthesis • Blue: Cell wall synthesis • Purple: Fatty acid synthesis 10 Size of reconstructed metabolic networks Model ID Organism Metabolites Reactions Genes 990 1250 844 iYO844 Bacillus subtilis 168 iML1515 Escherichia coli K-12 MG1655 1877 2712 1516 iMM904 Saccharomyces cerevisiae S288C 1226 1577 905 iMM1415 Mus musculus 2775 3726 1375 Recon3D Homo sapiens 5835 10600 2248 Data from BIGG database 11 Uses of Metabolic Network Model 12 McCloskey 2013 Metabolic Flux (I) • Flow of metabolites through the metabolic pathways • Rate of turnover of molecules through a metabolic pathway • Rate at which input metabolites are processed to form output metabolites (mmol/g DCW/h) • Fundamental determinant of cell physiology • Most critical parameter of a metabolic pathway A v1 E1 B v2 E2 C v3 v5 v4 E F A, B, C : metabolite E1, E2, ... : enzyme v1, v2, ... : flux D v6 G v7 H 13 Metabolic Flux (II) Input fluxes Volume of pool of water = metabolite concentration Output fluxes Slide credit: Jeremy Zucker 14 Metabolic Flux (III) Picture credit: Lue Huang 15 Flux estimation: Why don’t we just measure the fluxes? • There is currently no practical way to measure internal reaction rates in vivo, in a living cell in quantitative manner • Enzyme kinetics studies the reaction rates of individual enzymes in vitro, in a test tube, isolated from the rest of metabolism. These rates are in general not the same as in a living cell. • Microarrays and proteomics can give us estimates of concentration of mRNA or protein. However, these do not directly correlate with the reaction rates. These can be used to obtain qualitative estimates of pathway activity, but exact reaction rates v cannot be inferred. 16 Dynamic mass balance dx Vsyn Vdeg bi dt Concentration vector Stoichiometry Matrix dx S v dt Metabolic flux vector S: stoichiometric matrix (m n dimension) x: vector of metabolite concentrations (m 1) v: vector of reaction rates or fluxes through the metabolic reactions (n 1) bi: vector of external fluxes through system boundary (n 1) 17 Table 3.1 Different reaction networks, their stoichiometric matrices, and the respective system of ODEs 18 Problem with the Dynamic Mass Balance Reactions – Constant influx/outflux of the system boundary – Simple decay of A to B: mass action kinetics – Other reactions catalyzed by enzyme: MichaelisMenten kinetics b1, b2, b3 = constant v1 = k1*[A] v2 = Vmax2*[A]/(Km2 + [A]) v3 = Vmax3*[C]/(Km3 + [C]) v4 = Vmax4*[C]/(Km4 + [C]) dA/dt = - k1*[A] – Vmax2*[A]/(Km2 + [A]) + Vmax3*[C]/(Km3 + [C]) + b1 dB/dt = k1*[A] + Vmax4*[C]/(Km4 + [C]) – b2 dC/dt = Vmax2*[A]/(Km2 + [A]) - Vmax3*[C]/(Km3 + [C]) – Vmax4*[C]/(Km4 + [C]) – b3 • Vi is actually a function of concentration as well as several kinetic parameters. • Too many kinetic parameters! • The dynamic mass balance is hard to be solved as a matrix form. 19 Homeostasis of Metabolic Pathways • Organisms maintain homeostasis by keeping the concentrations of most metabolites at steady state • In steady state, the rate of synthesis of a metabolite equals the rate of breakdown of this metabolite • Even though the flux of metabolite flow (v) may be high, the concentration of S will remain almost constant by the preceding reaction). E.g. [glc]blood 5mM • The failure of homeostatic mechanisms leads to human disease (think diabetes: v1 for glc entry in blood ≠ v2 glc uptake into cells) • Pathways are at steady state unless perturbed • After perturbation, a NEW steady state will be established very quickly 20 Steady State Approximation of Metabolic Pathways • Steady state approximation – Concentration of internal metabolites (intermediates) remains the same in a duration of the reaction – No intermediates is allowed to accumulate. – An Intermediate in the reaction mechanism is consumed as quickly as it is generated – The rate of production of an intermediate is equal to the rate of its consumption • An intermediate is a species that is neither one of the reactants, nor one of the products. It transiently exists during the course of the reaction 𝑑[Int ] =0 𝑑𝑡 21 Quasi steady state (or pseudo steady state) • Metabolism usually involves fast reactions and high turnover of substances when compared to regulatory events. • The concentrations of metabolites tend to be very low, in the order of miromolar, or about 60,000 molecules per E. coli cell. Yet the metabolic fluxes are about 100,000 molecules per sec per cell. Thus, the average response time of a metabolic concentration is about 1 second. These transients are too fast for essentially all practical purposes. • Metabolites that are in higher concentrations, such as ATP, can have time constants that are on the order of minutes. Nevertheless, compared to the progression of an infection or bioprocessing this time is very short and metabolism is very fast and can be effectively considered to be in a steady state. Metabolic time scales and the quasi-steady state Fell, 1997 22 • Metabolic fluxes are in quasi steady-state relative to growth environment. • Therefore, analysis of metabolic networks is often based on the assumption that, on longer time scales, metabolite concentrations and reaction rates are constant. • The steady state approximation is generally valid because of fast equilibration of metabolite concentrations (seconds) with respect to the time scale of genetic regulation (minutes) 23 Mass Balance at Steady State (I) Input = Output (no accumulation) A v1 E1 B v2 E2 C v3 v5 v4 E F D v6 G v7 H At steady-states, v1 = v2 = v3 v4 = v5 v3 = v4 + v6 v1 = v5 + v7 At steady-states, v1 = v2 v2 = v3 + v5 + v7 v3 = v4 v4 + v5 = v6 v1 = v6 + v7 Image from Christopher Henry 24 Mass Balance at Steady State (II) dx S v dt 0 S v Steady state assumption 0 0 0 Now, we can solve the problem using linear algebra! 25 Flux Distribution at Steady State 26 Reaction Stoichiometry (S) Representation of a biochemical reaction as a reaction equation • Gene: glk • Enzyme: Glucokinase • Reaction: 1 Glucose + 1 ATP “reactions” or “substrates” are consumed 1 Glucose-6-Phosphate + 1 ADP “products” are produced Stoichiometry: the quantitative relationships of the reactants and products in a reaction Stoichiometric matrix: matrix representation of stoichiometry of biochemical reactions Glucokinase Glucose ATP -1 -1 G-6-P ADP +1 +1 27 Translating Biochemistry into Stoichiometric Matrix Slide from Bernhard Ø Palsson 28 How-To Make S Matrix Metabolites Reactions S 29 From Genomes to the S Matrix Columns encode reactions Rows encode metabolites The same reaction can be included as multiple roles (paralogs) Gene A Gene B Gene C Gene D Gene E Gene E’ Enzyme A Enzyme B/C Enyzme D Enzyme E Enzyme E’ R1 R2 R3 R4 R5 R6 R7 R8 R9 R10 A B C D E F G H I -1 0 0 -2 0 0 1 0 1 0 -1 0 -1 0 0 0 1 0 Same rxn 30 Stoichiometric Matrix (S) • Can be derived from annotated genomes given knowledge of enzyme stoichiometries • A mathematically compact description of metabolic maps • Has characteristic connectivity and graph properties • The stoichiometric matrix is ‘sparse’, i.e. few non-zero elements • It has well defined associated fundamental sub-spaces • These subspaces are keys to understanding pool and pathway formation, and thus model reduction and conceptual simplification 31 Constraint-Based Model (I) Find a steady-state flux distribution through all biochemical reactions by restricting the possible flux values that can take Biological constraints • Mass balance: metabolite production and consumption rates are equal - Quasi steady state : Sv = 0 • Thermodynamic: irreversibility of reactions, vi ≥ 0 (for all i reactions) • Reaction (or enzyme) capacity : αi ≤ vi ≤ βi Directly measurable fluxes • Growth rate • Availability of nutrients: composition of media • Product formation, oxygen uptake, CO2 emission, etc. • Balancing of energy metabolites (e.g. maintenance of ATP) • Precursor demand from a chemical analysis of cell mass composition 32 Example 1 (I) Solution space & Extreme pathways Problem Given the reaction network, define • biologically feasible region in x-z domain. • sets of possible flux patterns u A x Condition • irreversible reactions: x 0, y 0, z 0, u 0, v 0, w 0 • u (or substrate uptake rate) = 1 (mol/g DCW/h) Wiechert “Modeling and simulation: tools for metabolic engineering” 2002 B v y z C w 33 Example 1 (II) Step 1 : Set up flux-balance for each metabolite u For A: u - x - y = 0 A x B v z y For B: x - v - z = 0 C For C: y + z - w = 0 w 34 Example 1 (III) Step 2 : Impose constrains to the equations • u=1 • Irreversible reactions: x, y, z, v, w 0 For A: u-x-y = 0, y = u-x = 1-x u y 0 1-x 0, x 1 A x B v For B: x-v-z = 0, v = x-z y z v 0 x-z 0, z x C For C: y+z-w = 0, w = y+z, w = (1-x) + z w w 0 1-x+z 0, z x-1 x0 z0 35 Example 1 (IV) Solution z0 zx y<0 1 x<0 z x1 zx-1 0 0 z<0 1 x x0 Solution space of feasible fluxes Phenotypic definition from genotype ! 36 Example 1 (V) Extreme points of the feasible flux space Possible flux patterns For A: u-x-y = 0, 1-x-y = 0 z For B: x-v-z = 0 Mode 1 x B For C: y+z-w = 0 A x=0 y=1 z=0 u=1 v=0 w=1 Feasible fluxes x B v A z Mode 3 y w x u Mode 2 x B C w y C z v u x=1 y=0 z=1 u=1 v=0 w=1 u v A z y C w x=1 y=0 z=0 u=1 v=1 w=0 37 Constraint-Based Model (II) http://2014.igem.org/Team:Valencia_UPV/Modeling/fba 38 Constraint-Based Model (III) Mass balance S·v = 0 Subspace of Rn Unconstrained solution space Thermodynamic vi 0 Convex cone Capacity vi vmax Bounded convex cone Feasible (or allowable) solution space The set of all points that satisfy all the constraints 39 Online Course of Linear Algebra • Prof. Gilbert Strang at MIT • https://ocw.mit.edu/courses/mathematics/18-06-linear-algebraspring-2010/ 40 Linear Algebra(I): Vectors https://www.youtube.com/watch?v=fNk_zzaMoSs vod 41 Linear Algebra(II): Linear combinations, span, and basis vectors https://www.youtube.com/watch?v=k7RM-ot2NWY vod 42 Linear Algebra(III): Linear transformations and matrices https://www.youtube.com/watch?v=kYB8IZa5AuE vod 43 Linear Algebra(IV): Rank, orthogonality, and column span in a 3x3 matrix Rank • number of dimensions in the column space • number of dimensions in the output https://www.youtube.com/watch?v=RkEVuZ0x5mI vod 44 Linear Algebra(V): Inverse matrices, column space and null space https://www.youtube.com/watch?v=uQhTuRlWMxw vod 45 Null Space of Stoichiometric Matrix (S) dx S v dt 0 S v Steady state assumption Null space (kernel) of a matrix • A set of vectors that lands on the origin • The space of all vectors that become null (they land on the zero vector) 0 0 0 Null space matrix of S = Nul(S) = kernel of S = kernel matrix (K) of S S K 0 Null space (kernel) matrix K Sets of possible flux patterns 46 Null Space of Stoichiometric Matrix (S) • Contains all the solutions to Sv=0 • These are the steady state solutions to the dynamic mass balances • The null space contains all the steady state flux distributions • The dimension of the null space is the number of columns in the matrix minus the number of independent rows (the rank of the matrix) Rank theorem dim Nul(S) n rank(S) 47 Reduced row echelon form by Gauss-Jordan Elimination vod https://www.youtube.com/watch?v=0fTSBIBD7Cs vod https://www.youtube.com/watch?v=UhhfKXBnnEo 48 How to find the null space and the nullity of a matrix: Example https://www.youtube.com/watch?v=EpcCNOe-oyE vod 49 Example1: Null space (Finding the basis vectors for the null space) Ax = 0 Reduced row echelon form (using Gauss-Jordan elimination) • Fixed variables: variables in pivot columns (x1, x3) • Free variables: variables in the columns between the pivot columns (x2, x4, x5) 50 • The matrix A has two pivot columns (1 and 3) and three free variables (2,4,5). • All the variables can be expressed in terms of the free variables (parametric form) • The basis vectors u, v, w span the 3-dimensional null space – Au = Av = Aw = 0 – u, v, w is an independent set of vectors. 51 Example 2. Null Space (Example 3.2 from text) • Find a set of v satisfying Sv = 0 for the following reaction scheme v1 S1 v2 S2 v3 v6 S3 v4 S v5 Find the null space (or kernel) of S Nul(S) = (b1, b2, b3) = -1 1 1 -1 0 1 -1 0 1 0 0 1 0 1 0 1 0 0 • The rank theorem holds: dim Nul(S) = n – rank(S) 3 = 6 -3 • The entries for v2 and v3 are always equal in any steady state, the fluxes through reactions 2 and 3 must be equal. 52 Flux Cone • Subspace of feasible steady states within the space spanned by all positive-valued vectors for rates of irreversible reactions, vi, i = 1, ..., r. • Null space with all positive numbers • Convex polyhedron in the flux space Figure 3.1 Flux cone for (a) dim(Nul(S)) = 4, (b) N2, (c) N3 in Table 3.1 53 Extreme Pathways (EPs) • Minimal set of possible flux patterns, allowing to describe all feasible steady state flux distributions • Minimal set of reactions that cannot be further decomposed • Convex basis for the null space • Extreme lays of a convex polyhedron in the flux space v ≥ 0 for internal fluxes: reversible reaction is decomposed into forward and backward reactions 54 Example 1: Extreme pathways Sv = 0 Reduced row echelon form b2 is not feasible (not acceptable)! Find basis for the Null space 1 0 0 -1 0 0 0 1 0 -1 0 1 0 0 1 -1 0 1 0 0 0 0 1 -1 55 Basis transformation b1 b2 p1 p2 56 Example 2: Extreme pathways Step 1: Write stoichiometric matrix of the network Stoichiometric Matrix u A x B v z Fluxes y Balance Equations : C A:u-x-y=0 w B:x-v-z=0 C:y+z-w=0 x y z u v w -1 -1 0 1 0 0 1 0 -1 0 -1 0 0 1 1 0 Metabolite A Matrix Notation B C S= 0 -1 57 Step 2: Solve Sv = 0 for finding “Null space of S” Nul(S) = (b1, b2, b3) = 1 1 0 1 1 0 -1 0 1 0 0 1 1 0 0 1 0 0 0 1 1 1 1 1 0 1 0 0 1 0 0 0 1 1 0 1 p1 = b1+b3 P = p2 = b2 p3 = b3 = 58 Internal Flux vs External Flux Internal fluxes v : Internal Flux Exchange fluxes b : Exchange Flux A v1 b1 B b2 S= Metabolite Intracellular Internal fluxes • take place with in the cell (within the system boundary) • are hard to measure, but often we know their maximum value • are irreversible Exchange fluxes (or boundary fluxes) • • • • flow across the cellular boundary often can be measurable experimentally connect the inside metabolites of the cell to the outside metabolites are reversible: positive if mass is flowing out of the cell, and negative if mass is flowing into the cell (exit only: bi ≥ 0, enter only: bi ≤ 0) 59 Example 3: Extreme pathways Problem Given the reaction network, define sets of possible flux patterns A B C E D System Boundary 60 Step 1: Define fluxes, system boundary, and constrains Constraints b2 A v1 B v2 b1 v6 C v4 E b4 v5 v3 • For all Internal fluxes to be non-negative vi ≥ 0 (i = 1,2,...,7) v7 • For external fluxes D System Boundary enter only: bj ≤ 0 (j = 1,2,3,4) v : Internal Flux b3 exit only: bj ≥ 0 b : Exchange Flux Note that elementary reactions for boundary fluxes are: b1 A b2 C b3 D b4 E 61 Step 2: Write stoichiometric matrix of the network Stoichiometric Matrix Fluxes Balance Equations : v1 v2 v3 v4 v5 v6 v7 b1 b2 b3 b4 Metabolite A : -v1-b1 = 0 A -1 0 0 0 0 0 -1 0 0 0 B 1 -1 -1 1 0 0 0 0 0 0 0 C 0 1 0 0 -1 -1 0 0 -1 0 0 D : v3+v5-v4-v7-b3=0 D 0 0 1 -1 1 0 -1 0 0 -1 0 E : v6+v7-b4=0 E 0 0 0 0 1 1 0 0 B : v1+ v4-v2-v3=0 C : v2-v5-v6-b2=0 Matrix Notation S= 0 0 0 Note that elementary reactions for boundary fluxes are: b1 A b2 C b3 D b4 E 62 -1 Step 3: Solve Sv = 0 for finding “Null space of S” b2 v2 v6 P1 v1 b1 v3 P4 P2 P3 P5 P6 b4 v4 v5 v7 b3 b4 63 Elementary Flux Mode (EFM) (Optional) • A minimal sets of reactions that allow steady state dynamics • A minimal set of reactions that cannot be further decomposed • All steady state fluxes are linear combinations of elementary flux modes • A flux mode M comprising v is called reversible if the set M' comprising -v is also a flux mode • An elementary flux mode can be interpreted as a minimal set of enzymes that could operate at steady state (with all irreversible reactions used in the appropriate direction) • Number of EFMs ≥ number of basis vectors of the null space 64 https://mathbio.colorado.edu/index.php/MBW:The_(Right) _Null_Space_of_the_Stoichiometric_Matrix 65 Relation b/w EFM and EP (Optional) • Extreme pathways is a subset of elementary flux modes. • A flux mode is an extreme pathway if 1) all reactions are nonnegative, that is, v = virr; 2) it belongs to the edges of the flux cone, which also means that it represents a basis vector of K. • To achieve the first, reversible reactions are broken down into their forward and backward components and exchange fluxes have to be defined in the appropriate direction. • The extreme pathways are systemically independent. 66 Figure 3.2 Schematic representation of elementary flux modes for the reaction network (a) v2 is reversible, (b) v2 is irreversible. 67