OLS Asymptotics
Document Details
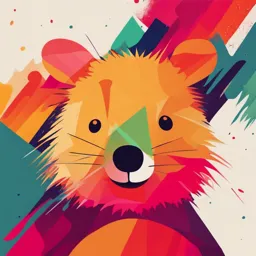
Uploaded by ClearerKoala
Ani Katchova
Tags
Summary
These lecture notes discuss the asymptotic properties of Ordinary Least Squares (OLS) estimators. The presentation covers consistency, comparing it to unbiasedness, and explaining asymptotic normality, with variances and standard errors in large samples as a key topic.
Full Transcript
OLS Asymptotics Ani Katchova © 2020 by Ani Katchova. All rights reserved. Outline • Consistency • Comparison of consistency versus unbiasedness • Asymptotic normality • Variances and standard errors in large samples 2 Consistency • An estimator ̂ ???????????? ???????????? is consistent if i...
OLS Asymptotics Ani Katchova © 2020 by Ani Katchova. All rights reserved. Outline • Consistency • Comparison of consistency versus unbiasedness • Asymptotic normality • Variances and standard errors in large samples 2 Consistency • An estimator ̂ ???????????? ???????????? is consistent if it converges in probability to the population parameter ???????????? ???????????? as the sample size ????????????increases. ???????????????????????????????????????????????? ̂ ???????????? ???????????? = ???????????? ???????????? , where ????????????= 0,1 ,… ,???????????? and ????????????→ ∞ Another way to express this is: • ???????????? ̂ ???????????? ???????????????????????? − ???????????? ???????????? < ???????????? →1 , where ????????????is any arbitrarily small value. • The probability P that the estimator ̂ ???????????? ???????????? obtained from a sample size ???????????? will be arbitrarily close to the population parameter ???????????? ???????????? goes to 1 as the sample size ????????????→ ∞. 3 Assumption 4: zero conditional mean (review) • Assumption 4: zero conditional mean • ???????????? ???????????? ????????????|???????????? ???????????????????????? = 0 • Expected value of error term given independent variables is zero. • Under assumptions 1 -4 (with the zero conditional mean assumption), the OLS estimator is unbiased. • ???????????? ̂ ???????????? ???????????? = ???????????? ???????????? • Expected value of the coefficient is the population parameter. With many samples, the average value of the coefficient will be the population parameter. 4 Assumption 4’: regressors are uncorrelated with error term • Assumption 4’: regressors are uncorrelated with the error term. • ???????????? ???????????? ???????????? =0and ???????????????????????????????????? ???????????? ???????????????????????? ,???????????? ???????????? = 0 • Assumption 4’ is weaker than assumption 4. • Under assumptions 1 -4’ (with the assumption that the regressors are uncorrelated with the error term), the OLS estimator is consistent. • ???????????????????????????????????????????????? ̂ ???????????? ???????????? = ???????????? ???????????? , where ????????????= 0,1 ,… ,???????????? • As the sample size ????????????increases, the sample coefficient ̂ ???????????? ???????????? converges in probability to the population parameter ???????????? ???????????? . 5 Consistency of the OLS estimator (proof) • Simple regression model: ????????????=???????????? 0 +???????????? 1 ???????????? 1 + ???????????? • The coefficient ̂ ???????????? 1 = ???????????????????????????????????? (???????????? 1 ,???????????? ) ???????????? ???????????????????????? (???????????? 1 ) = ???????????????????????????????????? (???????????? 1 ,???????????? 0 +???????????? 1 ???????????? 1 +???????????? ) ???????????? ???????????????????????? (???????????? 1 ) = = ???????????????????????????????????? (???????????? 1 ,???????????? 0 ) ???????????? ???????????????????????? (???????????? 1 ) + ???????????????????????????????????? (???????????? 1 ,???????????? 1 ???????????? 1 ) ???????????? ???????????????????????? (???????????? 1 ) + ???????????????????????????????????? (???????????? 1 ,???????????? ) ???????????? ???????????????????????? (???????????? 1 ) = ???????????? 1 ???????????????????????????????????? (???????????? 1 ,???????????? 1 ) ???????????? ???????????????????????? (???????????? 1 ) + ???????????????????????????????????? (???????????? 1 ,???????????? ) ???????????? ???????????????????????? (???????????? 1 ) = ???????????? 1 + ???????????????????????????????????? (???????????? 1 ,???????????? ) ???????????? ???????????????????????? (???????????? 1 ) • Using assumption 4’ that ???????????????????????????????????? ???????????? 1 ,???????????? =0, then • ???????????????????????????????????????????????? ̂ ???????????? 1 = ???????????? 1 • The OLS estimator is consistent under assumptions 1- 4 ’. 6 PropertyUndesirable propertyFormula Assump- tions Sample size Distribution unbiasedness biasedness ???????????? ̂ ???????????? ???????????? = ???????????? ???????????? 1-4 Small/any sample t, normal consistency inconsistency ????????????????????????????????????????????????̂ ???????????? ???????????? = ???????????? ???????????? 1-4’ Large sample asymptotically normal Consistency versus unbiasedness • The OLS estimator may be biased in small samples with assumptions 1- 4 ’. The stronger assumption 4 zero conditional mean is needed for the OLS estimator to be unbiased. • Unbiasedness is ideal and holds with any sample size. But if unbiasedness cannot be achieved in a small sample, then at least consistency can be achieved with a large sample. 7 Omitted variable bias (review) • The “true” population regression model is: ????????????=???????????? 0 +???????????? 1 ???????????? 1 +???????????? 2 ???????????? 2 +???????????? • We need to estimate: � ???????????? = ̂ ???????????? 0 + ̂ ???????????? 1 ???????????? 1 + ̂ ???????????? 2 ???????????? 2 • But instead we estimate a misspecified model: � ???????????? = � ???????????? 0 + � ???????????? 1 ???????????? 1 , where ???????????? 2 is the omitted variable from this model. • If ???????????? 1 and ???????????? 2 are correlated, there will be a relationship between them ???????????? 2 =???????????? 0 +???????????? 1 ???????????? 1 + ????????????= ???????????? 0 +???????????? 1 ???????????? 1 +???????????? 2 ???????????? 0 + ???????????? 1 ???????????? 1 +???????????? +???????????? = (???????????? 0 + ???????????? 2 ???????????? 0 )+ (???????????? 1 + ???????????? 2 ???????????? 1 )???????????? 1 + ???????????? 2 ???????????? + ???????????? The coefficient that will be estimated for ???????????? 1 when???????????? 2 is omitted will be biased. 8 Omitted variable bias (review) • An unbiased coefficient is when ???????????? ̂ ???????????? 1 = ???????????? 1 , but this coefficient is biased because ???????????? � ???????????? 1 = ???????????? 1 +???????????? 2 ???????????? 1 , where ???????????? 2 ???????????? 1 is the bias. • With an omitted variable, the coefficient will not be biased if • ???????????? 2 =0. Looking at ???????????? =???????????? 0 +???????????? 1 ???????????? 1 +???????????? 2 ???????????? 2 +????????????, this means that ???????????? 2 does not belong in the model ( ???????????? 2 is irrelevant). • ???????????? 1 =0. Looking at ???????????? 2 = ???????????? 0 +???????????? 1 ???????????? 1 + Omitted variable bias –asymptotic analog • A consistent coefficient is when ????????????????????????????????????????????????̂ ???????????? 1 = ???????????? 1 , but this coefficient is inconsistent because ????????????????????????????????????????????????̂ ???????????? 1 = ???????????? 1 +???????????? 2 ???????????? 1 , where ???????????? 2 ???????????? 1 is the bias. • With an omitted variable, the coefficient will be consistent if • ???????????? 2 =0. Looking at ???????????? =???????????? 0 +???????????? 1 ???????????? 1 +???????????? 2 ???????????? 2 +????????????, this means that ???????????? 2 does not belong in the model ( ???????????? 2 is irrelevant). • ???????????? 1 =0. Looking at ???????????? 2 = ???????????? 0 +???????????? 1 ???????????? 1 +????????????, this means that ???????????? 2 and ???????????? 1 are not correlated. Note that ̂ ???????????? 1 = ???????????????????????????????????? (???????????? 2,???????????? 1) ???????????????????????????????????? (???????????? 1) • In other words, if the omitted variable ???????????? 2 is irrelevant ???????????? 2 =0or uncorrelated ???????????? 1 = 0, the coefficient will be consistent. 10 Asymptotic normality • Under assumptions 1-6 (Gauss Markov assumptions and normality), the coefficients have normal sampling distribution. • ̂ ???????????? ???????????? ~ ???????????????????????????????????????????????????????????????????????? (???????????? ???????????? ,???????????????????????????????????? ̂ ???????????? ???????????? ) • Under assumptions 1 -5 (Gauss Markov assumptions), the coefficients have asymptotically normal sampling distribution. • ̂ ???????????? ???????????? ???????????????????????????????????????????????????????????????????????? ???????????? ???????????? , ???????????????????????????????????? ̂ ???????????? ???????????? • In other words, even without normality of errors, asymptotic normality is achieved in large samples. 11 Asymptotic normality • The normality assumption 6 does not always hold in practice. • In small samples, the normality assumption 6 is needed for the t-tests and F-tests to be valid. • In large samples, without the normality assumption 6: • The OLS estimators are normal. • The t- tests and F -tests are valid. • Note that assumptions 1- 5 are still needed. 12 OLS properties • OLS properties that hold for any sample • Expected values and unbiasedness under assumptions 1-4 (linearity, random sample, no perfect collinearity, zero conditional mean) • Variance formulas under assumptions 1- 5 (linearity, random sample, no perfect collinearity, zero conditional mean, homoscedasticity) • Gauss- Markov theorem (BLUE) under assumptions 1- 5 • Exact sampling distributions (t-test and F-test) under assumptions 1- 6 (Gauss- Markov assumptions + normality) • OLS properties that hold in large samples (asymptotics) • Consistency under assumptions 1- 4’ (regressors uncorrelated with error term) • Asymptotic normality/tests under assumptions 1- 5 13 Variances of OLS estimators -asymptotics Recall, the variance of the OLS estimator: ???????????? ???????????????????????? ̂ ???????????? ???????????? = � ???????????? 2 ???????????????????????? ???????????? ???????????? (1 − ???????????? ????????????2 ) = � ???????????? 2 ???????????? ∗???????????????????????????????????? ???????????? ???????????? (1 − ???????????? ????????????2 ) • ???????????????????????? ???????????? ???????????? =∑ ???????????? =1 As the sample size increases, ???????????????????????????????????? ̂ ???????????? ???????????? changes by 1/????????????and ???????????????????????? ̂ ???????????? ???????????? changes by 1/????????????. 14 Standard errors and sample size example • Regression model: • ???????????? As sample size ???????????? increases, standard errors change at a rate of 1/???????????? • With larger sample size, standard errors are lower, leading to more significance of the coefficients. 15 Standard errors and sample size example Model with full sampleModel with half the sample VARIABLES wage wage educ 0.599*** 0.732*** (0.0513) (0.0875) tenure 0.169*** 0.209*** (0.0216) (0.0359) exper 0.0223* 0.0477** (0.0121) (0.0194) Constant -2.873*** -4.815*** (0.729) (1.237) Observations 526 262 R-squared 0.306 0.329 For the coefficient on experience, se1=0.0121, se2=0.0194, n1=526, n2=262. The ratios se1/se2=0.62 and ??????????????????/?????????????????? =0.71 are almost the same. With the full sample (double the sample size), standard errors are 62% lower. 16 Review questions • Define consistency. • Compare consistency and unbiasedness. • Define asymptotic normality. • At what rate does the variance change when the sample size changes? 17