new-era-unconditional-convergence.pdf
Document Details
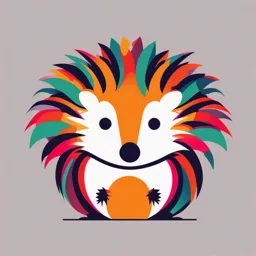
Uploaded by StraightforwardFeynman
UEH
2021
Tags
Full Transcript
The New Era of Unconditional Convergence Dev Patel, Justin Sandefur, and Arvind Subramanian Abstract The central fact that has motivated the empirics of economic growth—namely unconditional divergence—is no longer true and has not been so for decades. Across a range of data sources, poorer countri...
The New Era of Unconditional Convergence Dev Patel, Justin Sandefur, and Arvind Subramanian Abstract The central fact that has motivated the empirics of economic growth—namely unconditional divergence—is no longer true and has not been so for decades. Across a range of data sources, poorer countries have in fact been catching up with richer ones, albeit slowly, since the mid-1990s. This new era of convergence does not stem primarily from growth moderation in the rich world but rather from accelerating growth in the developing world, which has simultaneously become remarkably less volatile and more persistent. Debates about a “middle-income trap” also appear anachronistic: middle-income countries have exhibited higher growth rates than all others since the mid-1980s. Keywords: Unconditional convergence, economic growth, middle-income trap JEL: F43, N10, 047 Working Paper 566 February 2021 www.cgdev.org The New Era of Unconditional Convergence Dev Patel Harvard University [email protected] Justin Sandefur Center for Global Development [email protected] Arvind Subramanian Ashoka University [email protected] This paper extends an earlier piece which circulated under the title, “Everything you know about cross-country convergence is (now) wrong.” The ideas here benefited from discussions with and comments from Doug Irwin, Paul Johnson, Chris Papageorgiou, Dani Rodrik, and David Rosnick, as well as commentaries on our earlier piece from Brad DeLong, Paul Krugman, Noah Smith, and Dietrich Vollrath. Patel acknowledges support from a National Science Foundation Graduate Research Fellowship under grant DGE1745303. All errors are ours. Dev Patel, Justin Sandefur, and Arvind Subramanian, 2021. “The New Era of Unconditional Convergence.” CGD Working Paper 566. Washington, DC: Center for Global Development. https://www.cgdev.org/publication/new-era-unconditional- convergence The replication code and data for this paper are available here: https://www.cgdev.org/ sites/default/files/patel-sandefur-subramanian-replication-data.zip. More information on CGD’s research data and code disclosure policy can be found here: www.cgdev.org/page/ research-data-and-code-disclosure. Center for Global Development The Center for Global Development works to reduce global poverty 2055 L Street NW and improve lives through innovative economic research that drives Washington, DC 20036 better policy and practice by the world’s top decision makers. Use and dissemination of this Working Paper is encouraged; however, reproduced 202.416.4000 copies may not be used for commercial purposes. Further usage is (f) 202.416.4050 permitted under the terms of the Creative Commons License. www.cgdev.org The views expressed in CGD Working Papers are those of the authors and should not be attributed to the board of directors, funders of the Center for Global Development, or the authors’ respective organizations. 1 Introduction Since World War II, the economic fortunes of the poorest countries have been a major pre-occupation of economic research and policy-making, rendered more urgent by the fact that developing countries had been falling even further behind their richer counterparts—the phenomenon of unconditional divergence. For example, in 1950, per capita income in the United States was 17 times higher than that in India in purchasing-power parity terms (Penn World Tables 10.0). By 1990, that ratio had increased to a nearly 30-fold income differential. Rich and poor countries seemed to be growing in different worlds. Yet, by 2017, the ratio of U.S. to Indian per capita GDP had fallen to just 9:1. This paper documents that India was not alone: the central fact motivating cross-country growth analysis—namely unconditional divergence—has reversed course. The Solow (1956) growth model predicts that poor countries should grow faster than rich ones. Given access to the same technology, low-income countries with lower capital stocks should also have higher marginal product of capital and therefore grow relatively faster as they accumulate more capital. But the data told a different story. Starting with Barro’s (1991) seminal paper and reinforced in a number of important contributions since—including Pritchett (1997), Rodrik (2014) and a recent review paper (Johnson and Papageorgiou, 2020)—the literature found that over reasonably long time horizons, poorer countries do not, on average, grow faster than richer ones. Regressions of per capita GDP growth on the initial level of income per capita did not yield statistically significant evidence of convergence, and frequently the opposite. This null result spawned a great deal of research on different aspects of cross-country growth. One strand, drawing inspiration from Lucas (1988), King et al. (1988), and Romer (1990), explored whether controlling for factors such as human capital, investment, financial sector development, foreign aid, trade, etc., could recover conditional convergence (Barro, 1991). Other lines of work focused on non-linearities in the growth process that create con- vergence clubs (Quah, 1993; Durlauf and Johnson, 1995) and on the cross-country dispersion in the level of income per capita (called σ-convergence). It is not our purpose to summarize this vast literature but just to note that the mo- tivation for much of the empirical growth literature was the result showing the absence of unconditional convergence. Instead, our purpose here is to document a set of key and related facts that demonstrate that, since about the mid-1990s, that null finding has been reversed: economic growth is now unambiguously characterized by unconditional convergence. The stellar performance of a selected group of developing countries since the 1960s and 1970s, especially in East Asia, has for long been the object of study and debate (Birdsall et al., 1 1993). But the fact that a large proportion of developing countries had started growing faster than advanced countries and at an accelerating pace began to be noticed about a decade ago (Subramanian, 2011; The Economist, September 22, 2011; Roy et al., 2016). Recent papers have examined this remarkable performance among developing countries through the lens of trade (Irwin, 2019) and structural transformation (Diao et al., 2019). Parallel work to ours by Kremer et al. (2020) documents a trend towards unconditional convergence since the 1960s, highlighting dramatic changes in policies and institutions over the same period. They find a decline in the growth-institution slope that results in a narrowing gap between conditional and unconditional convergence. Building on Patel et al. (2018), in this paper we focus on establishing three central facts about developing country growth performance to decompose this headline convergence result. First, since the mid-1990s, developing countries began to converge toward levels of income of advanced countries. This process accelerated and became strongest in the 2000s. In technical terms, the coefficient in the unconditional convergence regression flipped sign in the 1990s, becoming statistically significant since the 2000s. The speed of this convergence has remained quite modest. Whereas Barro and Sala-i- Martin (1992) suggested a rate of catch-up of about two percent every year within advanced economies, and Sachs and Warner (1995) find a similar speed of convergence across open economies, we estimate a much smaller magnitude across all countries since 2000. At this pace, the average developing country will close half the gap between its current and steady state income in only about 170 years. Recent cross-country convergence is not driven by advanced nations lowering their growth performance but rather by developing countries raising theirs. This is true even in the post- global financial crisis period. Essentially, the entire distribution of growth amongst rich countries has remained stable over time; in contrast, the entire distribution of poor country growth has shifted up. For low-income countries, therefore, improving average performance is associated with almost equal gains at the tails of the distribution. This also means that an increasing number of poor countries have avoided egregiously bad performance: 42% of low- income countries experienced a negative average growth rate during the 1980s as compared to 16% of that group in the 2000s and 2010s. Second, contrary to suggestions of a middle-income trap, we find in fact that cross- sectional growth in the period of convergence exhibits an inverted U-pattern. Whereas middle- and low-income countries have been growing faster than advanced economies since the 1990s, middle-income countries have been consistently growing faster than all groups, even low-income countries, since the 1980s. This strong middle-income performance—more trampoline than trap—may well have obscured the fact and speed of convergence. 2 Finally, the era of unconditional convergence is also associated with lower volatility and higher growth persistence within developing countries. This suggests that not only has average cross-sectional growth performance changed between rich and poor countries but so too has the underlying growth process within countries. This is particularly reassuring given Easterly et al.’s (1993) result intimating that developing country growth was highly unstable, reflected in low correlations of growth across time, and hence more vulnerable to external shocks and less amenable to domestic policy. Lower volatility and greater persistence is even more marked for middle-income countries. 2 Convergence The neoclassical growth model implies that poorer countries with smaller capital stocks per worker will grow faster than richer ones until their incomes per capita converge, condi- tional on preferences and technologies. Barro and Sala-i-Martin (1992) derive an empirical test of unconditional convergence assuming these conditions hold, which they argue is plau- sible for U.S. states and less so for countries. Growth of economy i between time t and time t + s is a function of initial income, y(i,t) , and a speed of convergence parameter, β. 1 − e−βs 1 yi,t+s ln =α− ln (yi,t ) + εi,t+s (1) s yi,t s The authors document a convergence rate of approximately 2 percent per annum across U.S. states but no unconditional convergence across countries, confirming earlier findings from Barro (1991). Only after conditioning on school enrollment and government consump- tion as a share of GDP are they able to produce conditional convergence around the world at a similar annual rate to that within the U.S. In what follows, we return to this basic specification in equation (1), testing for uncon- ditional convergence in cross-country data up to the most recent available data (generally t + s = 2019), and systematically varying the starting point, t, from 1960 to 2010. Fol- lowing Barro and Sala-i-Martin (1992), we estimate β using non-linear least squares with heteroskedasticity-robust standard errors. 2.1 Convergence and Its Timing Figure 1 plots the β parameter from the unconditional convergence regression in equation (1) relating average growth of real per capita GDP to its initial level along with 95 percent confidence intervals. The growth rates are calculated from every year (the starting point shown on the x-axis) to a fixed end-point which is the latest year for which data are available. 3 Johnson et al. (2013) show that growth rates vary widely across datasets. Accordingly, we plot the annual βs for three standard sources: the Penn World Tables (PWT) (Feenstra et al., 2015), the World Development Indicators (WDI) (World Bank, 2020), and the Maddison Project (Bolt and van Zanden, 2020).1 Since convergence is only meaningful beyond short horizons, the last starting point shown is 2010. A positive β denotes convergence and divergence otherwise. The specific point estimates vary across datasets, but the broadly consistent pattern across them all is that for start dates between 1960 and 1995, the β parameter is either negative (for the PWT and Maddison data) or positive and statistically insignificant (for the WDI data); for start dates after 1995, the βs turn positive in all data sets and also become statistically significant. This graph does not hold the sample of countries fixed, using all available observations for each period. Appendix Figure A.1 recreates the graph using a constant sample of countries since 1980, which is the earliest year that covers a substantial number of low-income countries across all data sets. The same convergence pattern emerges from that specification. In Figure 1, all the growth rates are with respect to a fixed terminal date (2018 in Mad- dison and 2019 in the PWT and WDI). What happens if we chose other end points? Figure 2 plots a heat map which shows the β parameter for the unconditional growth regression for every start date since 1960 (on the y-axis) and every terminal date (on the x-axis) using the PWT. Red denotes divergence and blue signifies convergence. Looking across specifications, we see that convergence is not driven by the selection of the latest available year as the terminal date. Rather, even looking at growth rates up to the global financial crisis, the pattern changes from red to blues starting with initial incomes measured in the 1990s. This same pattern holds in the other data sets (see Appendix Figures A.2 and A.3) and in a fixed sample of countries since 1980 (see Appendix Figure A.4). 2.2 The Speed of Convergence From the perspective of development, it is important not just that convergence occurs but that it happens at a pace fast enough for standards of living to improve quickly. Sala- i-Martin (1996) notes that the standard speed of convergence from the data is two percent. Barro (2015) based on a longer historical exercise concludes that this number is close to 1.7 1 In the WDI data, all growth rates are in constant local currency units converted to dollars at market exchange rates. In the Maddison and PWT data, the growth rates are adjusted for PPP changes across time based on Feenstra et al. (2015). The latest available years of data (and the end points of the convergence regressions shown in figure 1) are 2019 for the PWT, 2018 for Maddison, and 2019 for the WDI. We exclude small countries (with populations under 1 million in each year) and oil producers as defined by the International Monetary Fund. For years in the WDI prior to when the PPP series is available, we apply growth rates from constant national dollars retroactively. 4 percent, although these magnitudes are based on models of conditional convergence. The magnitudes for unconditional convergence have been estimated in analysis of growth within countries (Barro and Sala-i-Martin, 1990) and growth across open economies (Sachs and Warner, 1995). Both indicate a magnitude of unconditional convergence of just over two percent for open economies. For example, Sachs and Warner (1995) estimate that between 1970 and 1989, among countries that were open throughout this period, rich countries grew at 2.3 percent every year compared with 4.5 percent for developing economies. One intuitive way of capturing the speed of convergence is the length of time required for poor countries to close half the gap between their steady state and current income levels. The β parameter implies a certain time-frame for this half-life given by equation 2. 1 − e−βs τ = − ln (2) / ln 1 − (2) s For example, the conditional convergence coefficient estimated in Sala-i-Martin (1996) im- plies that the typical developing country would eliminate half the gap between steady state per capita GDP and its current income in τ = 35 years. Our analysis, however, yields much smaller magnitudes for β, and therefore for the half- life τ. For the period 2000-2019, based on a sample of 124 countries in the PWT, the β parameter is.00425 with a standard error of.00156. This β yields, in turn, an estimate for a half-life catch-up of τ = 170 years. In this sense, convergence is occurring at a very modest pace. This magnitude of β-convergence has only managed to reduce the overall variance of per capita GDP across countries—known as σ-convergence—since the 2008 Global Financial Crisis. β-convergence can fail to produce σ-convergence if the initial distribution of income has a lower variance than the steady state. Formally, if we assume that shocks to growth are mean zero with a fixed variance and independent across time and space, β-convergence is a necessary but not sufficient condition for σ-convergence (see Young et al. (2008) for a proof). Empirically, we find no evidence of meaningful σ-convergence, even well into the era of unconditional β-convergence (see Appendix Figure A.5). 2.3 Decomposing Convergence Is convergence happening because rich countries have slowed down, because poor coun- tries have accelerated their growth, or some combination of the two? One possibility is that post-1990s convergence reflects worsening performance in the OECD rather than improving poor country performance (De Long, 2018). In Figure 3, 5 we plot the unconditional convergence relationship for a group of countries for two time periods, 1960-2000 and 2000-2019. We see clearly that convergence in the recent period is a consequence of both improving per capita GDP growth in poor countries and declining growth in rich countries. So, the latter is not the cause of convergence. In fact, it’s both.2 Points representing poorer countries have moved up in the post-2000 period (second panel) compared with the post-1960 period (first panel). At the same time, points representing rich countries have drifted down in the second panel compared to the first. But in general, the upward drift of poor countries is greater than the downward drift in growth of rich countries. Rich country growth was broadly stable between 1960 and the mid- 1990s and then declined in the mean by about half a percentage point. In contrast, for both low- and middle-income countries, the entire distribution of country growth rates shifts up since the early 1990s, having been broadly stationary previously. The 5th percentile moves up by close to.5 and 2 percentage points, respectively for low-and middle-income countries, and the 95th percentile by about 1.5 and 2 percentage points, respectively. The mean moves up by a little under 1 and 1.5 percentage points, respectively for low- and middle-income countries relative to the pre-convergence era. A striking feature of the period of convergence is that poor countries manage to avoid extreme adverse outcomes, reflected in almost the entire distribution clocking positive growth rates (Broadberry and Wallis, 2017). Whereas in the 1980s and 1990s (the era of divergence), 24 to 42 percent of low-income countries experienced negative growth, by the 2010s, only 16 percent of countries did so, as shown in Table 1. Unconditional convergence happens therefore not because rich countries’ performance declines but mostly due to the entire distribution of poor countries improving theirs. There were much fewer “valleys” in the experience of poor countries in the convergence era and sub- stantially more “hills” (Pritchett, 1997), resulting in substantially better average performance and in unconditional convergence. An additional dimension of interest related to unconditional convergence is regional. There is an extensive literature on the dynamic performance of Asia and the consistent under-performance of sub-Saharan Africa (Birdsall et al., 1993; Collier and Gunning, 1999). Here we want to relate the role of the different regions in the basic convergence result in Figure 1. We plot, in Figure A.7, for the same horizons and same starting and end-points, the β parameters by excluding countries in each of the three major regions: Asia, Africa, 2 Note that testing convergence for periods starting much later than the 1990s could raise concerns about conflating long-term patterns and cyclical swings. For comparison, the earlier growth literature focused on the 1960âĂŞ85 period in the PWT. 6 and Latin America. Like all specifications in this paper, these regressions are not population weighted, and thus each country counts equally.3 The persistent drag of Africa is evident from Figure A.7. The convergence coefficient in the sample without SSA is about 0.75 percentage points greater than that in the entire sample. In the other direction, the impact of Asia is also evident: the convergence coefficient is significantly lower in the sample without Asian countries than that in the entire sample, even in the era of convergence. The mild surprise is Latin America not exercising a greater drag in the era of convergence: the β parameters in the sample without Asia and without Latin America are not strikingly different. One possibility is that because Latin American countries are on average richer than African countries, or even those in Asia, their slower growth is not inconsistent with convergence. 3 The Missing “Middle-Income Trap” Based on the experience of selected Latin American countries, Gill and Kharas (2015) articulated the idea of a “middle-income trap.” 4 They applied this to describe economies that were being “squeezed between the low-wage poor country competitors that dominate in mature industries and the rich-country innovators that dominate in industries undergoing rapid technological change.” It is possible that the success of this idea owed to the background belief in divergence. If developing countries on average are not catching up, it is plausible that the richer among them are not able to transition out of middle-income status. In fact, we observe precisely the opposite: Looking at the relationship between initial income and growth non-parameterically, as shown in the right panel of Figure 3 for the post- 2000 period, growth rates follow an inverted U-shape across the income distribution. The poorest countries are growing faster than the rich countries, but the countries in the middle 3 The non-linear least squares estimation from Figure 1 becomes quite sensitive to the starting value in these smaller samples, and thus we instead estimate ordinary linear least squares of the specification: 1 yi,t+s s ln yi,t = α + λ ln (yi,t ) + εi,t+s , where β is calculated afterward by solving β = − ln (λs + 1) /s. 4 There are many ways of defining middle income, and all of them have deficiencies. The World Bank’s definition is somewhat arbitrary, based on a metric (GNI computed by the Atlas method) that is not completely clear and also only available since the late 1980s. In order to make a definition meaningful for temporal comparisons, we do the following. At the starting point of the sample in 1960, we define high- income and low-income as the top and bottom quartiles of the GDP per capita distribution and the rest as middle-income. In years since 1960, we calculate the relative position of these original cut-offs to the United States and apply those thresholds dynamically. This too is arbitrary, but we check that our results are robust to changes in these thresholds. The initial classification passes some important smell tests: for example, India and China are defined as low-income. And, the quartile-based cut-offs, yield the following relative thresholds: low-income countries are those with per capita GDP below 7.85 percent of the United States level; and middle-income countries are those with per capita GDP greater than this threshold and less than 33.55 percent of US per capita GDP. 7 are growing even faster. Instead of a trap, we seem to have a trampoline.5 This is corroborated in Figure 4a, which shows that growth for middle-income countries has been consistently higher than for poor and rich countries, at least since the mid-1990s. We test this more formally for every year and for the three datasets, by adding a middle-income dummy to the unconditional growth equation as shown below. The sign and magnitude of the coefficient (γ) is a test for the middle-income trap. 1 − e−βs 1 yi,t+s ln =α− ln (yi,t ) + γ 1{y ≤ yi,t ≤ y} + εi,t+s (3) s yi,t s The sample and time frame for this regression are the same as those in Figure 1. The results (see Figure A.8 in the appendix) broadly confirm what is illustrated in Figure 4a. For much of the post-war period, there seems to be no advantage to having middle-income status. But that has changed since about 1985-1990: the middle-income indicator starts becoming positive and statistically significant. In the era of unconditional convergence, middle-income countries seem to grow about 21 to 34 of a percentage point faster than the typical country after controlling for the initial level of income. 4 Within-Country Growth Patterns in the Convergence Era While unconditional convergence and the lack of a middle income trap are both important findings, they relate to the cross-sectional pattern of growth. We present two other facts about within-country growth behavior that shed light on the growth processes underpinning the convergence result. In Figure 4b, we plot for the same periods and time horizons as in Figure 1, the distribu- tion of the within-country volatility of growth, measured by the standard deviation shown in equation 4.6 s Pt+s r=t+1 (∆ln(yir ) − ∆ln(yi )) σi = (4) s−1 We plot the average of this within-country volatility measure for each of the high-, middle-, and low-income groups. We find that the distribution of within-country growth volatility is low and broadly stable 5 Another focus of the literature on middle-income trap has been the propensity for growth slowdowns, i.e., decelerations rather than low growth levels (Eichengreen et al., 2013). Pritchett and Summers (2014) argue this phenomenon may reflect simple regression to the mean. 6 As we proceed from 1960 to the latest period, the number of years over which the standard deviation is calculated declines successively by one year. 8 for rich countries. More volatility is almost a defining characteristic of poor countries. Inter- estingly, this volatility declines considerably for both low- and middle-income countries. For middle-income countries, the decline begins around the 1980s, but for low-income countries, this decline in volatility coincides with the era of convergence. Next, in Figure 4c, we plot the evolution of within-country growth persistence.7 Persis- tence for country i is measured by the ρ coefficient as shown in equation 5. ρ = corr (∆ ln(yi,r ), ∆ ln(yi,r−1 )) for t < r ≤ t + s (5) We plot it for the same horizons as in Figure 1 and for all three income groups using the PWT. This figure illustrates some new and interesting patterns. High-income countries experienced more persistent growth only until the 1970s. Thereafter, it is growth in middle-income coun- tries that has been more persistent. Most striking is that in the era of convergence, growth in both low- and high-income country growth has become less persistent with magnitudes that are similar to each other and well below that of middle-income countries. These persistence results confirm the main insight of Easterly et al. (1993) and more recently Pritchett and Summers (2014), though with a slight twist. Easterly et al. (1993) established that growth rates are highly unstable over time, with a correlation across decades of.1 to.3. They contrasted that with the persistence of other country characteristics (such as education and investment) to argue that shocks rather than policy fundamentals had a key role in explaining the variance in cross-country growth.8 Our findings confirm the generally low persistence of growth over time, but the relative performance across income groups looks quite different. Persistence is no longer a direct function of income as it was in the 1960s. Instead, it has declined to very low levels for both high- and low-income countries. Middle-income countries now show the most persistent growth rates, in direct contradiction to the narrative of a middle-income trap. The lack of a middle-income trap has another subtle impact on convergence and its mag- nitude. In Figure 1, we saw that even in the era of convergence the β was modest, suggesting slow convergence. But might this be a consequence of the “middle income trampoline” it- self. It is possible that even though poor countries are growing faster than rich ones, the β parameter is dampened because middle-income countries are growing fastest.9 7 Figure A.6 presents the same figures for a fixed sample of countries since 1980. 8 Related work in Easterly (2001) shows that during the 1980s and 1990s, low-income countries experienced lower growth despite improvements in standard “policy” variables. 9 Krugman (2018) offers a possible explanation for this middle income trampoline: “One thing is clear: at any given time, not all countries have that mysterious ‘it’ that lets them make effective use of the backlog of advanced technology developed since the Industrial Revolution. Over time, however, the set of countries that have It seems to be widening. Once a country acquires It, growth can be 9 One way of understanding this is to adopt a slightly different perspective on convergence. Suppose we asked the question: how has the average poor country fared compared not to the entire sample of countries but to the growth of advanced countries per se? This amounts to a simple comparison of the average growth rates of rich and poor countries. In the period of convergence, this difference rises to 1.5 percentage points in the 2000s and.65 in the 2010s, as shown in Table 1. In fact, throughout this era, middle-income countries grow faster than low-income countries (see Figure 4a). 5 Caveats and Discussion It is important to emphasize that our analysis is all at the level of countries. These country-level patterns do not map neatly onto outcomes for individuals. The new era of convergence coincides with a remarkable decline in global earnings inequality (Hammar and Waldenstr om, 2015), and corresponds roughly to the rising rump in Lakner and Milanovic’s (2015) famous “elephant graph.” As they show—and the more recent work of Alvaredo et al. (2018) puts in even starker relief—the key factor slowing the decline in global inequality is the elephant’s trunk: the explosion of top incomes not revealed in aggregate GDP figures. But at the country-level at least, we should update, perhaps even shed, three of the deeply entrenched ideas about cross-country growth: unconditional divergence, middle- income traps, and volatile and unstable growth within poor countries over time. Since the mid-1990s, it’s not “just” China, India, or a select group of Asian countries that have done well; developing countries on average outpaced the developed world. And in this era of unconditional convergence, middle-income countries—far from being stuck in a trap— experienced reduced volatility and more persistent growth. In other words, the facts have changed considerably. That convergence happened over the last 25 years is, of course, no guarantee that it will continue. Until we know what caused it (exogenous factors related to cheap finance, Chinese growth, and/or country-specific attributes), and until we know the impact of future developments (such as deglobalization, climate change, and rise of labor-saving technology), rapid, precisely because best practice is so far ahead of where the country starts. And because the frontier keeps moving out, countries that get It keep growing faster. Japan’s postwar growth was vastly faster than that of the countries catching up to Britain in the late 19th century; Korea’s growth from the mid-60s even faster than Japan’s had been; ChinaâĂŹs growth faster still. “The It theory also, I’d argue, explains the U-shaped relationship Patel et. al. (2018) find between GDP per capita and growth, in which middle-income countries grow faster than either poor or rich countries. Countries that are still very poor are countries that haven’t got It; countries that are already rich are already at the technological frontier, limiting the space for rapid growth. In between are countries that acquired It not too long ago, which has vaulted them into middle-income status, but are able to grow very fast by moving toward the frontier.” 10 unconditional convergence cannot be taken for granted. That said, we must finally acknowledge that what has happened since the mid-1990s is historically remarkable. Developing countries as a group have broken from a pattern of development going back nearly 500 years. Between the 1400s and the industrial revolu- tion, there was a reversal of fortune (Acemoglu et al., 2002) and subsequent divergence, involving today’s poor countries that were once rich falling behind industrial nations. Since the industrial revolution, reversal gave way to divergence in growth rates between rich and poor nations (Pritchett, 1997). Both those historical trends have been arrested since the mid-1990s, making the unconditional convergence finding truly noteworthy. 11 References Acemoglu, Daron, Simon Johnson, and James A. Robinson, “Reversal of Fortune: Geography and Instiuttions in the Making of the Modern World Income Distribution,” The Quarterly Journal of Economics, 2002, 117 (4), 1231–1294. Alvaredo, Facundo, Lucas Chancel, Thomas Piketty, Emmanuel Saez, and Gabriel Zucman, World Inequality Report 2018, Harvard University Press, 2018. Barro, Robert J., “Economic Growth in a Cross Section of Countries,” Quarterly Journal of Economics, 1991, 106 (2), 407–443. , “Convergence and Modernisation,” Economic Journal, 2015, 125 (585), 911–942. and Xavier Sala-i-Martin, “Economic Growth and Convergence across The United States,” National Bureau of Economic Research Working Paper, 1990, (3419). and , “Convergence,” Journal of Political Economy, 1992, 100 (2), 223–251. Birdsall, Nancy M., Jose Edgardo L. Campos, Chang-Shik Kim, W. Max Corden, Lawrence MacDonald, Howard Pack, John Page, Richard Sabor, and Joseph E. Stiglitz, The East Asian Miracle: Economic Growth and Public Policy, Washington, D.C.: World Bank Group, 1993. Bolt, Jutta and Jan Luiten van Zanden, “Maddison Style Estimates of the Evolution of the World Economy,” Maddison Project Database, version 2020, 2020. Broadberry, Stephen and John Joseph Wallis, “Growing, Shrinking, and Long Run Economic Performance: Historical Perspectives on Economic Development,” NBER Work- ing Paper, 2017, (23343). Collier, Paul and Jan Willem Gunning, “Why Has Africa Grown Slowly?,” The Journal of Economic Perspectives, 1999, 13 (3), 3–22. Diao, Xinshen, Margaret McMillan, and Dani Rodrik, “The Recent Growth Boom in Developing Economies: A Structural-Change Perspective,” in “The Palgrave Handbook of Development Economics.” 2019. Durlauf, Steven and Paul Johnson, “Multiple Regimes and Cross-Country Growth Be- havior,” Journal of Applied Econometrics, 1995, 10 (4), 365–384. Easterly, William, “The Lost Decades: Explaining Developing Countries’ Stagnation in Spite of Policy Reform 1980-1998,” Journal of Economic Growth, 2001, 6. , Michael Kremer, Lant Pritchett, and Lawrence H. Summers, “Good Policy or Good Luck? Country Growth Performance and Temporary Shocks,” Journal of Monetary Economics, 1993, 32 (3), 459–483. 12 Eichengreen, Barry, Donghyun Park, and Kwanho Shin, “Growth slowdowns redux: New evidence on the middle-income trap,” National Bureau of Economic Research Working Paper, 2013, (18673). Feenstra, Robert C., Robert Inklaar, and Marcel P. Timmer, “The Next Generation of the Penn World Table,” American Economic Review, 2015, 105 (10), 3150–3182. Gill, Indermit S. and Homi Kharas, “The Middle-Income Trap Turns Ten,” The World Bank Policy Research Working Paper, 2015. Hammar, Olle and Daniel Waldenstr om, “Global Earnings Inequality, 1970—2018,” The World Bank Policy Research Working Paper, 2015. Irwin, Douglas, “Does Trade Reform Promote Economic Growth? A Review of Recent Evidence,” Working Paper, 2019. Johnson, Paul and Chris Papageorgiou, “What Remains of Cross-Country Conver- gence?,” Journal of Economic Literature, 2020. Johnson, Simon, William Larson, Chris Papageorgiou, and Arvind Subramanian, “Is Newer Better? Penn World Table Revisions and Their Impact on Growth Estimates,” Journal of Monetary Economics, 2013, 60 (2), 255–274. King, Robert G., Charles I. Plosser, and Sergio T. Rebelo, “Production, Growth and Business Cycles: I. The Basic Neoclassical Model,” Journal of Monetary Economics, 1988, 21 (2-3), 195–232. Kremer, Michael, Jack Willis, and Yang You, “Converging to Convergence,” Working Paper, 2020. Krugman, Paul, “Notes on Global Convergence (Wonkish and Off-Point),” The New York Times, October 2018. Lakner, Christoph and Branko Milanovic, “Global Income Distribution: From the Fall of the Berlin Wall to the Great Recession,” World Bank Economic Review, 2015, 30 (2), 203–232. Long, Bradford De, “How Confident Are We That Middle-Income Convergence to the Global Productivity Frontier is Now the Rule? Not At All,” 2018. Lucas, Robert E., “On the Mechanics of Economic Development,” Journal of Monetary Economics, 1988, 22, 3–42. Patel, Dev, Justin Sandefur, and Arvind Subramanian, “Evertyhing You Know About Cross-Country Convergence Is (Now) Wrong,” October 2018. Vox EU. Pritchett, Lant, “Divergence, Big Time,” Journal of Economic Perspectives, 1997, 11 (3), 3–17. 13 and Lawrence H Summers, “Asiaphoria meets regression to the mean,” National Bureau of Economic Research Working Paper, 2014, (20573). Quah, Danny, “Empirical Cross-Section Dynamics in Economic Growth,” European Eco- nomic Review, 1993, 37 (2-3), 426–434. Rodrik, Dani, “The Past, Present, and Future of Economic Growth,” Challenge, 2014, 57 (3), 5–39. Romer, Paul M., “Endogenous Technological Change,” Journal of Political Economy, 1990, 98 (5). Roy, Sutirtha, Martin Kessler, and Arvind Subramanian, “Glimpsing the End of Economic History? Unconditional Convergence and the Missing Middle Income Trap,” Center for Global Development Working Paper 438, 2016. Sachs, Jeffrey D and Andrew M. Warner, “Economic Convergence and Economic Policies,” National Bureau of Economic Research Working Paper, 1995, (5039). Sala-i-Martin, Xavier, “The Classical Approach to Convergence Analysis,” Economic Journal, 1996, 106 (437), 1019–1036. Solow, Robert M., “A Contribution to the Theory of Economic Growth,” Quarterly Jour- nal of Economics, 1956, 70 (1), 65–94. Subramanian, Arvind, Eclipse: living in the Shadow of China’s Economic Dominance, Washington, D.C.: Peterson Institute for International Economics, 2011. The Economist, “A Game of Catch-Up: Special Report,” The Economist, September 22, 2011. World Bank, The, World Development Indicators, Washington, D.C.: World Bank, 2020. Young, Andrew T., Matthew J. Higgins, and Daniel Levy, “Sigma Convergence versus Beta Convergence: Evidence from U.S. County-Level Data,” Journal of Money, Credit and Banking, 2008. 14 Figure 1: Unconditional convergence from various starting dates to today.01 Maddison PWT.0075 WDI.005 β.0025 0 -.0025 -.005 60 65 70 75 80 85 90 95 00 05 10 19 19 19 19 19 19 19 19 20 20 20 Initial Year Note: The figure plots the β parameter of unconditional convergence for three separate data series: Mad- dison, the Penn World Tables 10.0, and the World Development Indicators. Each coefficient comes from a non-linear least squares regression of real per capita GDP PPP growth rate to the latest available year on the initial per capita income: 2018for Maddison and 2019 for the PWT and WDI. The plotted y 1−e−βs parameter is β from the specification: 1s ln i,t+s yi,t = α − s ln (yi,t ) + εi,t+s , described further in section 2. The starting year spans 1960 to 2010. The sample excludes oil exporters and countries with populations under 1 million. 15 Figure 2: Unconditional convergence: alternative starting and ending dates >.0035 [.0025,.0035] 2010 [.0015,.0025] [.0005,.0015] [-.0005,.0005] 2000 [-.0015, -.0005] [-.0025, -.0015] [-.0035, -.0025] Start Year 1990 [-.0045, -.0035] [-.0055, -.0045].0035 [.0025,.0035] 2010 [.0015,.0025] [.0005,.0015] [-.0005,.0005] 2000 [-.0015, -.0005] [-.0025, -.0015] [-.0035, -.0025] Start Year 1990 [-.0045, -.0035] [-.0055, -.0045].0035 [.0025,.0035] 2010 [.0015,.0025] [.0005,.0015] [-.0005,.0005] 2000 [-.0015, -.0005] [-.0025, -.0015] [-.0035, -.0025] Start Year 1990 [-.0045, -.0035] [-.0055, -.0045].0035 [.0025,.0035] [.0015,.0025] 2010 [.0005,.0015] [-.0005,.0005] [-.0015, -.0005] [-.0025, -.0015] [-.0035, -.0025] Start Year 2000 [-.0045, -.0035] [-.0055, -.0045]