MED106 Research Methods in Medicine & Essential Medical Statistics PDF
Document Details
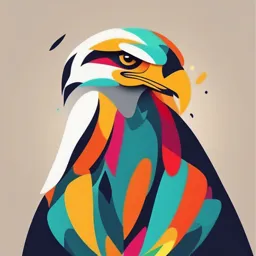
Uploaded by AppreciableDouglasFir
University of Nicosia Medical School
2024
Elena Critselis
Tags
Summary
This document provides lecture notes on information bias (measurement error) in research methodology. It covers different types of information bias, and includes examples and methods for minimizing such biases, along with testing understanding exercises. The notes are sourced from the University of Nicosia Medical School.
Full Transcript
MED106 Research Methods in Medicine & Essential Medical Statistics Systematic error in research II: Information bias (Measurement Error) Elena Critselis, MPH PhD Associate Professor in Epidemiology and Public Health Department of Primary Care and Population Health University of Nicosia Medical Schoo...
MED106 Research Methods in Medicine & Essential Medical Statistics Systematic error in research II: Information bias (Measurement Error) Elena Critselis, MPH PhD Associate Professor in Epidemiology and Public Health Department of Primary Care and Population Health University of Nicosia Medical School Learning Objectives Describe how information bias affects the validity of research studies. Differentiate between the concepts of validity and reliability. Describe the major types of validity and reliability. Systematic Error (Bias) There are two main types of bias in research: 1. Selection bias: Systematic error arising from mistakes conducted during the selection of the study sample. 2. Information bias: Systematic error arising from mistakes conducted during the measurement of key study variables (i.e. exposure and outcome). Different types of information bias Local Admissions (EU) Information bias Information bias arises from wrong and/or inaccurate assessment(s) of the exposure and/or the disease outcome variables. Such mistakes may arise: From the researchers’ part (unintentionally) From the participants’ part (unintentionally or intentionally). There is also instrument bias (fault of the measurement instruments used) which falls under the researcher’s part. Information bias arising from researcher actions: Assessor Bias Wrong/inaccurate diagnosis due to a clinical error. May occur when researchers are not “blinded” to exposure or outcome status of participants. Wrong/inaccurate measurements due to a faulty instrument/machine. Wrong/inaccurate measurements due to poor training of the assessor(s). Mistakes during recording and/or transferring of data from paper form to electronic form. Examples of information bias arising from researcher actions: Wrong/inaccurate diagnosis due to a clinical error: Vascular dementia being incorrectly diagnosed as Alzheimer’s Disease by a research doctor Type 2 diabetes cases missed (not diagnosed) due to only one fasting glucose measurement Wrong/inaccurate measurements due to a faulty instrument/machine: Measuring blood pressure using an inaccurate (imprecise) instrument Measuring levels of a blood biomarker using a wrongly- calibrated machine Wrong/inaccurate measurements due to poor training of assessor: A researcher measuring height inaccurately due to lack of training A researcher assessing cognitive function based on a questionnaire he/she is not trained to administer Examples of information bias arising from researcher actions (cont’d) Examples of mistakes during recording of data: Errors done when reading results from instrument/machine outputs (i.e. blood pressure, lipids, glucose measurements etc.) Errors done when noting down manual measurements (i.e. height, waist, etc.) Examples of mistakes during transferring data from paper form into electronic form: Errors done when transferring results from questionnaires to electronic datasets Errors done when transferring lab (biochemical) results from paper form to electronic datasets Errors done when transferring disease status data for participants (diseased/not diseased) from paper form to electronic form Test your understanding! When investigating the association between lifestyle factors (i.e. dietary intake, physical activity, sleep quality, etc) and depression among both students and faculty at the University of Nicosia, which of the following research methods scenarios is not likely to introduce information bias? A. Administering a physical activity questionnaire by an untrained researcher. B. Including as study participants only undergraduate students. C. Making errors when transferring responses from a dietary questionnaire in paper format to an electronic database. D. Measuring the weight of participants using a wrongly calibrated weighing scale. E. Judging whether participants are depressed based on self-report. Test your understanding! When investigating the association between lifestyle factors (i.e. dietary intake, physical activity, sleep quality, etc) and depression among both students and faculty at the University of Nicosia, which of the following research methods scenarios is not likely to introduce information bias? SOLUTION: A. Administering a physical activity questionnaire by an untrained researcher. B. Including as study participants only undergraduate students. C. Making errors when transferring responses from a dietary questionnaire in paper format to an electronic database. D. Measuring the weight of participants using a wrongly calibrated weighing scale. E. Judging whether participants are depressed based on self-report. Information bias arising from participant actions (misreporting) Wrong/inaccurate answers from participants due to: Misinterpretation of a question. A sensitive issue relating to the question. Poor recall (recall bias). Participants intentionally responding incorrectly. Overall, information bias arising from participant actions is called response bias (i.e. when people do not answer questions truthfully for some reason). Examples of information bias arising from participant actions Examples of wrong/inaccurate answers from participants due to a misinterpretation of a question: Participants requested to report frequency of intake of a food item per week, but instead they report per month. Participants asked how often they smoke, but instead respond how many cigarettes they smoke. Examples of wrong/inaccurate answers from participants due to a sensitive issue relating to the question: Participants giving inaccurate information regarding sensitive socioeconomic information (i.e. household income, housing status, food insecurity, etc). Participants giving inaccurate information on seemingly unhealthy behaviors (i.e. smoking, excessive alcohol use, substance abuse, etc.). Participants giving inaccurate information on illicit or illegal behaviors (i.e. illegal substance abuse, domestic violence, criminal activities etc.). Participants giving inaccurate information on disease status, particularly when associated with social stigma (i.e. mental health disorders, such as alcoholism, addictions, schizophrenia, etc). Examples of information bias arising from participant actions (cont’d) Examples of wrong/inaccurate answers from participants due to poor recall: Participants not remembering their past exposures (i.e. whether they were exposed to pesticides in the past). Participants forgetting to report diseases which have occurred in the past. Participants forgetting to report past medication use. Examples of intentional wrong/inaccurate answers from participants: Participants providing wrong/inaccurate answers due to careless behavior. Participants providing random or pattern answers (i.e. circling all ‘1s’). Types of information bias Misclassification bias: Error(s) in correctly classifying participants as having been exposed (vs not) and/or having the disease outcome (vs not having the disease). Usually due to measurement instrument inaccuracy or error. May occur in any type of study design. Recall Bias: As compared to healthy individuals, diseased participants may differentially recollect / report past exposure(s), particularly when the specific exposure(s) causes the disease. Differential recall may arise from over-reporting of past exposure(s) in the case group as well as under-reporting of past exposure(s) in the control group. Most often occurs in case-control studies. Interviewer Bias: A researcher’s preconceived knowledge and perceptions may influence the structure of questions and their manner of presentation, hence affecting the accuracy of study participants’ responses. The interviewer asks leading questions or has an inconsistent interview approach between cases and controls. May occur in any type of study design, particularly when researchers are not blinded to participants’ exposure or disease status. Types of information bias (cont’d) Hawthorne effect: Participants act differently when they know that they are being observed. Observer Bias: Researchers may have preconceived expectations of what they should find in an examination, particularly if they are not blinded to participants’ exposure status and/or medical history. Surveillance bias: The group with the known exposure or outcome may be followed more closely or for longer time periods than the comparison group. Usually due to researcher’s bias. Examples of misclassification bias Inaccuracies in methods of data acquisition may result in the misclassification of subjects with respect to exposure and/or disease status. May arise from either researchers’ or participants’ actions. Usually due to limited sensitivity and specificity of diagnostic tests, or inadequacy of information derived from medical records. Measurement error in the ascertainment of: Exposure Outcome/disease Covariates (e.g. confounders) Examples: Exposed incorrectly classified as non-exposed, or vice versa (e.g. misclassification of vaccination status due to lack of health record). Cases incorrectly classified as controls, or vice versa (e.g. misclassification with respect to the presence of breast cancer based on breast ultrasound vs mammography). Example of recall bias Cases remember exposure differently than controls (e.g. risk of malformation) Mothers of Children with Controls m alform ation Took tobacco, alcohol, drugs a b Did not take c d Mothers of children with malformations remember past exposures better than mothers with healthy children Overestimation of “a” overestimation of OR Example of interviewer bias Investigator asks cases and controls differently about exposure (e.g: soft cheese and listeriosis) Cases of listeriosis Controls Eats soft cheese a b Does not eat soft cheese c d Investigator may probe listeriosis cases about consumption of soft cheese (knows hypothesis) Overestimation of “a” overestimation of OR Methods for minimizing bias Be purposeful in the study design to minimize the chance for bias. Example: Use more than one control group. Define a priori specific mutually exclusive criteria for defining who has been exposed (versus not exposed) and/or who has the disease outcome (versus no disease) so that there is no overlap between categories/groups. Define categories within groups clearly (e.g. age group categories, highest educational level attained, etc). Set up strict guidelines for data collection. Train observers / interviewers to obtain data in the same fashion. It is preferable to use more than one observer or interviewer, but not so many that they cannot be trained in an identical manner. Optimize questionnaire(s) used through pilot study. Test your understanding! In an investigation into familial breast cancer, when participants forget to report other members of the family that have been diagnosed with the same disease, this may lead to which of the following biases? A. B. C. D. Information bias arising from participants’ actions Information bias arising from researchers’ actions Selection bias due to participants’ errors Selection bias due to researchers’ errors Test your understanding! In an investigation into familial breast cancer, when participants forget to report other members of the family that have been diagnosed with the same disease, this may lead to which of the following biases? SOLUTION: A. Information bias arising from participants’ actions B. Information bias arising from researchers’ actions C. Selection bias due to participants’ errors D. Selection bias due to researchers’ errors How information bias may affect research findings Local Admissions (EU) How can information bias affect study results? Fundamental principle of research: If you want to investigate any association between two factors, first make sure that you measure these two factors accurately! Information bias can be introduced in the assessment of both the main exposure and the main outcome, thus the association between them will definitely be distorted. Information bias arising from participant actions is more common as compared to information bias arising from researcher actions. How can information bias affects study results? Information bias affects mainly studies that rely on self- reports (i.e. questionnairebased data collection). For assessing exposures (i.e. diet, physical activity, smoking, educational attainment, etc.), the most valid and reliable instruments have to be used. Exposure may be double – checked and confirmed usually via: Concomitant use of similar measurement tools (e.g. 24 hour dietary recall with 3 day food diary) Biomarkers Medical or other health records For assessing outcomes, particularly when diseases status is based on self-report, the presence of the disease outcome is double –checked and confirmed usually via: Information from participants’ personal GP Medical or other health records Pharmaceutical (or other) treatment administered. How can information bias affects study results? If a study relies solely on self-reports, then it should be assumed that information bias (measurement error) is operating to some extent. The presence of information bias always compromises the validity of the study results and in such a case, findings must be interpreted with caution! Example (false positive finding) A study investigated the association between physical activity and Alzheimer’s Disease (AD). The study was retrospective in design, meaning that existing AD patients and matched controls, were asked to report their past exposure. Assessment of physical activity was by participant self-reports. AD patients tended to report less physical activity than controls (likely due to poor recall). As a result, an association appeared in the study sample (low physical activity linked to AD), which in fact does not exist in reality (i.e. in the source population). This error was a result of information bias in the exposure of interest! Example (false negative finding) A study investigated the association between waist circumference and blood pressure. Blood pressure was assessed by an accurate sphygmomanometer. Waist circumference was assessed by non-trained staff and there was a particular difficulty assessing large waists. As a result, the anticipated association between waist circumference and blood pressure (higher waist – higher blood pressure) which exists in the source population, did not appear in the study sample. This error was a result of information bias in the exposure of interest! How bias affects study results? Note 1: False positive and false negative findings can arise also in situations where information bias affects the outcome of interest. If both exposure and outcome are affected by information bias, then the study findings are expected to be particularly biased (erroneous). Note 2: All types of biases (including selection bias), as well as random error, can give rise to false positive and false negative findings. Validity and reliability Any assessment tool used in research should ideally have a high validity and reliability. Validity: The extent to which an assessment tool (e.g. questionnaire, instrument, etc.) measures accurately what it is intended to measure. Criterion validity is the most common type used in medical research. In such a case, the results from the assessment tool of interest are compared with those of an established (“gold standard”) assessment tool. Reliability: The overall consistency of a measure, as regards producing the same results when administered under the same conditions in the same group of people. It is also known as reproducibility or repeatability, and includes the following types: Intra-observer reliability: Describes the agreement between results when the assessment tool is used by the same researcher (observer) on two or more occasions (under the same conditions and in the same test population). Inter-observer reliability: The degree of agreement between the results when two or more researchers (observers) administer the assessment tool on the same people under the same conditions. Validity If a determination is made that the findings of a study were not due to any bias / error, then the study is considered internally valid. In other words, the conclusions reached are likely to be correct for the circumstances of that particular study. This does not necessarily mean that the findings can be generalized to other circumstances (also known as external validity). When reading a study, one should be aware of potential biases that might have affected the conclusions and be able to predict what effect a given bias would be expected to have on the effect estimate(s) reported. Example of the effects of external validity The Physicians' Health Study concluded that aspirin use reduced the risk of myocardial infarction in adult male physicians in the United States. The study was carefully done and the study was internally valid, BUT it was not clear that the results could be extrapolated to women, or even to non-physicians (whose risk of myocardial infarction is generally lower than that of the population overall). Evaluating associations Note: DO NOT compromise internal validity with the goal of generalization in mind! An invalid result cannot be generalized. Thus, internal validity should never be compromised in an attempt to achieve generalizability. Optional further reading Petrie A. & Sabin C. Medical Statistics at a Glance, 3rd Edition, Chapter 3 [ISBN : 9781-4051-8051-1] Kirkwood B. & Sterne J. Essential Medical Statistics, Chapter 36 [ISBN: 978-0-86542871-3] Buring EJ. Epidemiology in Medicine, Chapter 11 [ISBN : 978-0316356367] http://www.bmj.com/about-bmj/resources- readers/publications/epidemiologyuninitiated/4-measurement-error-and- bias For additional information: [email protected]