Chapter 3 Research Methodology PDF
Document Details
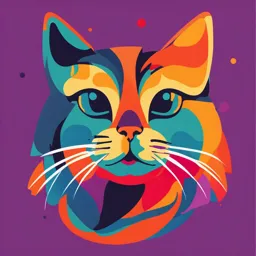
Uploaded by RewardingBandoneon1899
Tags
Summary
This document provides a comprehensive overview of research methodology, focusing on the key components of quantitative research. It explores topics such as research design, sampling techniques, and data analysis methods.
Full Transcript
CHAPTER 3 Research Methodology Parts of Research Methodology Research Research Research Research Design Sampling Participants Instruments Data Data Gathering Analysis Procedure Research Design defined as the ration...
CHAPTER 3 Research Methodology Parts of Research Methodology Research Research Research Research Design Sampling Participants Instruments Data Data Gathering Analysis Procedure Research Design defined as the rational and coherent overall strategy that the researcher uses to incorporate all the vital components of the research study. Hence, in order to find meaning in the overall process of doing your research study, a step-by-step process will be helpful to you. Data Analysis Research Design According to Fraenkel and Wallen (2007, p 15), the research designs in quantitative research are mostly pre-established. Hence having an appropriate research design in quantitative research, the researcher will have a clearer comprehension of what he is trying to analyze andData interpret. Analysis Quick Review Quantitative Research Designs have five general classifications: descriptive, correlational, ex post facto, quasi-experimental, and experimental. Data Analysis Research Sampling The population is the totality of all the objects, elements, persons, and characteristics under consideration. It is understood that this population possesses common characteristics about which the research aims to explore. There are two types of population: target Datapopulation Analysis and accessible population Research Sampling 1. Target Population - the actual population. For instance, “All Senior High School Students enrolled in Science, Technology, Engineering, and Mathematics (STEM) in the Division of Cagayan de Oro City.” Data Analysis Research Sampling 2. Accessible Population - the portion of the population in which the researcher has reasonable access. For instance, “All Senior High School enrolled, STEM strand at Marayon Science High School – X.” Data Analysis Research Sampling Sampling pertains to the systematic process of selecting the group to be analyzed in the research study. The goal is to get information from a group that represents the target population. Once a good sample is obtained, the generalizability and applicability of findings increases. Data Sample refers to the representative subset of the Analysis population. Sample Heuristics. This approach refers to the general rule or rule of Size the thumb for sample size. This is the earliest established approach for sample sizes for different research designs. Research Design Suggested Number of Subjects/Participants Descriptive Research 10% to 20% maybe required Comparative Research 15 subjects or groups Survey 800 Correlational 100 to 200 Ex post Facto 30+ Experimental 30 or more Sample Size Formulas. Are also being established for the computation of an acceptable sample size. You can use different formulas depending on what you know and do not know about the population. These tools are also available online. One formula for determining sample size that you can follow is the Data Cochran especially if you have large sample size. Analysis Sample Size Data Analysis Power Analysis Statistical Power is the probability of rejecting the null hypothesis. The ideal statistical power of a research study is 80%. With the statistical power, it will be used to identify the sufficient sample size for measuring the effect size of a certain treatment. Effect Size is the e level of difference between the Data experimental group and the control group. Analysis. With the effect size, you will have an idea of how small or large the difference is. Probability Sampling Simple Random Sampling - it is a way of choosing individuals in which all members of the accessible population are given an equal chance to be selected. There are various ways of obtaining samples through simple random sampling. These are fishbowl technique, roulette wheel, or use of the table of random numbers. Data Analysis Probability Sampling Stratified Random Sampling - also gives an equal chance to all members of the population to be chosen. However, the population is first divided into strata or groups before selecting the samples. The samples are chosen from these subgroups and not directly from the entire population. This procedure is best used when the variables Data of the study are also grouped into classes such as genderAnalysis and grade level. Probability Sampling Cluster Sampling - is usually applied in large-scale studies, geographical spread out of the population is a challenge, and gathering information will be very time-consuming. It also involves grouping of the population according to subgroups or clusters. It is a method where multiple clusters of people from the chosen population will be created byData the researcher in order to have homogenous characteristics. Analysis Probability Sampling Systematic Sampling - e is as simple as selecting samples every nth (example every 2nd, 5th) of the chosen population until arriving at a desired total number of sample size. Therefore, the selection is based on a predetermined interval. Dividing the population size by the sample size, the interval will be obtained. Data Analysis Research Participants also called a human subject or an experiment, trial, or study participant or subject, is a person who voluntarily participates in human subject research after giving informed consent to be the subject of the research. Data Analysis Research Instruments These are basic tools researchers used to gather data for specific research problems. Common instruments are performance tests, questionnaires, interviews, and observation checklist. However, interviews and observation checklists can still be used in quantitative research once the information gathered is Data translated into numericalAnalysis data. Characteristics of a Good Instrument Concise - a good research instrument is concise in length yet can elicit the needed data. Sequential - questions or items must be arranged well. It is recommended to arrange it from simplest to the most complex. Valid and Reliable - the instrument should pass the tests of validity and reliability to get more appropriate and accurate information. Data Analysis Easily Tabulated - before crafting the instruments, the researcher makes sure that the variable and research questions are established. Ways in Developing an Instrument 1. Adopting an Instrument - already utilized instruments from previous related studies. 2. Modifying an Existing Instrument - when the available instruments do not yield the exact data that will answer the research problem. 3. Researcher Made Questionnaire - Data corresponds to the variable and scope of his current study. Analysis Common Scales Used 1. Likert Scale. This is the most common scale used in quantitative research. Respondents were asked to rate or rank statements according to the scale provided. Data Analysis Common Scales Used 2. Semantic Differential. In this scale, a series of bipolar adjectives will be rated by the respondents. This scale seems to be more advantageous since it is more flexible and easier to construct. Validity A research instrument is considered valid if it measures what it supposed to measure. For instance, when measuring oral communication proficiency level of students, speech performance using rubric, or rating scale is more valid than students are given multiple choice tests. Data Validity also has several types: face,Analysis content, construct, concurrent, and predictive validity. Types of Validity of Instrument 1. Face Validity. It is also known as “logical validity.” It calls for an initiative judgment of the instruments as it “appear.” Just by looking at the instrument, the researcher decides if it is valid. 2. Content Validity. An instrument that is judged with content validity meets the objectives of the study. It is done by checking the statements or questions if this Dataelicits the needed Analysis information. Types of Validity of Instrument 3. Construct Validity. It refers to the validity of instruments as it corresponds to the theoretical construct of the study. It is concerning if a specific measure relates to other measures. 4. Concurrent Validity. When the instrument can predict results like those similar tests already validated, it has concurrent validity. 5. Predictive Validity. When the instrument Data can produce results similar to those similar tests thatAnalysis will be employed in the future, it has predictive validity. This is particularly useful for the aptitude test. Reliability Reliability refers to the consistency of the measures or results of the instrument. Data Analysis Types of Reliability 1. Test-retest Reliability. It is achieved by giving the same test to the same group of respondents twice. The consistency of the two scores will be checked. 2. Equivalent Forms Reliability. It is established by administering two identical tests except for Datawordings to the same group of respondents. Analysis Types of Reliability 3. Internal Consistency Reliability. It determines how well the items measure the same construct. It is reasonable that when a respondent gets a high score in one item, he will also get one in similar items. There are three ways to measure the internal consistency; through the split-half coefficient, Cronbach’s alpha, and Kuder-Richardson formula. Data Analysis Data Gathering Procedure Data Gathering in quantitative research usually observes survey, observation, and experiment. There are three phases in data gathering of research that need to be presented in the research paper: before, during, and after procedures. Data Analysis Techniques in Collecting Data 1. Observation. It is gathering information about a certain condition by using senses. The researcher records the observation as seen and heard. This is done by direct observation or indirect observation using gadgets or apparatus. An observation checklist aids the researcher in recording the data gathered. Data Analysis Techniques in Collecting Data 2. Survey. Data gathering is done through interview or questionnaire. By means of questionnaire you use series of questions or statements that respondents will have to answer. Basically, respondents write or choose their answer from given choices. On the other hand, interview is when you ask respondents orally to tell you the responses. Since you are doing quantitative research, it is expected that Data responses have Analysis numerical value either it is nominal or ordinal in form. Techniques in Collecting Data 3. Experiment. When your study is an experimental design, it was already discussed in the previous lesson that it would use treatment or intervention. After the chosen subjects, participants, or respondents undergone the intervention, the effects of such treatment will be measured. Data Analysis Three Phases in Data Collection The data gathering procedure is presented in a paragraph format in your research paper. Basically, the contents are the steps you are going to follow: (1) before you will gather the data, (2) what to do during the actual gathering of data, and (3) the things to consider after data has been gathered. Data The following are the suggested steps butAnalysis not limited to it, are the procedures in gathering quantitative data. Before Prepare the research instruments Identify the authorities that will be involved and need to ask permission Determine the samples size and corresponding respondents; per group if applicable. Ask consent form (if respondents are 18 years old above) or parent's consent (if minor). Data Analysis Pilot test the research instrument if needed. During Clear the instructions provided to the respondents. Administer the research instrument or implement the research intervention, if applicable. Collect or gather or take note of the responses. Data Analysis After Summarize the data gathered, in a tabular form Analyze the summarize data corresponding to the research questions. Data Analysis Data Analysis Data Analysis is a process in which gathered information are summarized in such a manner that it will yield answers to the research questions. Statistical Treatment makes explicit the different statistical methods and formulas needed to analyze the research data. Data Analysis Data Analysis Descriptive Statistical Techniques provides a summary of the ordered or sequenced data from your research sample. Examples of these tools are frequency distribution, measure of central tendencies (mean, median, mode), and standard deviation. Data Analysis Data Analysis Inferential Statistics is used when the research study focuses on finding predictions; testing hypothesis; and finding interpretations, generalizations, and conclusions. Since this statistical method is more complex and has more advanced mathematical computations, you can use computer software to aid your analysis.Data Analysis Types of Statistical Analysis of Variable Univariate Analysis means analysis of one variable. Bivariate Analysis is analysis of two variables such as independent and dependent variables Multivariate Analysis involves analysis of the multiple Data relations between multiple variables. Analysis Parametric Tests vs Non - Parametric Tests Parametric Tests - are those that make assumptions about the parameters of the population distribution from which the sample is drawn. This is often the assumption that the population data are normally distributed. Non-parametric Tests - are “distribution-free” and, as Data such, can be used for non-normal variables. Analysis Parametric Tests vs Non - Parametric Tests Types of Statistical Techniques Test of Relationship Between Two Variables Pearson’s r (parametric) Phi Coefficient (non-parametric for nominal and dichotomous variables) Spearman’s Rho (non-parametric for ordinal variable) Types of Statistical Techniques Test of Difference Between Two Data Sets from One Group T-test for Dependent Samples (parametric) McNemar Change Test (non-parametric for nominal and dichotomous variables) Wilcoxon Signed-Rank Test (non-parametric for ordinal variable) Types of Statistical Techniques Test of Difference between Two Data Sets from Two Different Groups T-test for Independent Samples (parametric) Two-way Chi-square (non-parametric for nominal variable) Mann-Whitney U Test (non-parametric for ordinal variable) Types of Statistical Techniques Test More than Two Population Means Analysis of Variance or ANOVA (parametric) Test the Strength of Relation or Effect or Impact Regression (parametric) FINALLY! END OF CHAPTER 3 RESEARCH METHODOLOGY DISCUSSION