Lecture 9 Study Design Issues PDF
Document Details
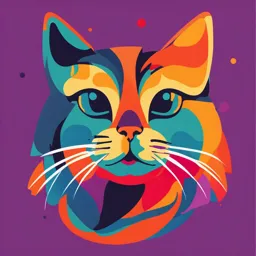
Uploaded by IntriguingTiger
Bay Atlantic University
Büşra YILMAZ
Tags
Summary
This lecture discusses research methods in dentistry, focusing on study design issues. It covers types of studies, including experimental and observational studies, and the importance of sample size and effect size estimations. It also explores ethical considerations, biases, and confounding factors in research.
Full Transcript
RESEARCH METHODS IN DENTISTRY Study Design Issues Assoc. Prof. Büşra YILMAZ School of Dental Medicine Department of Oral and Maxillofacial Radiology [email protected] Types of Study Research on people involves two approaches: experiments or observation without intervention. Studies may d...
RESEARCH METHODS IN DENTISTRY Study Design Issues Assoc. Prof. Büşra YILMAZ School of Dental Medicine Department of Oral and Maxillofacial Radiology [email protected] Types of Study Research on people involves two approaches: experiments or observation without intervention. Studies may describe disease prevalence or population characteristics or seek connections between diseases and factors like risk or treatment. Experimental Studies Observational Studies Types of Study Experimental Studies: These involve conducting experiments or interventions with a group and comparing them to another group that does not receive the intervention. Observational Studies: These focus on observing without intervening. They describe the prevalence of a disease or characteristics of a population and identify associations between diseases and other factors like risk factors or treatments. Purpose of Clinical Trials and Observational Studies Clinical Trials: Primarily examine the effectiveness of treatments and preventive measures. Observational Studies: Primarily used to identify risk factors and causes of diseases. Ethical Considerations Clinical Trials: Governed by ethical considerations; it's unethical to intentionally harm individuals for research purposes. Randomization in Trials: Ethically acceptable when uncertainty exists about the effectiveness of different treatments. Informed Consent: Essential in all studies involving human subjects. Participants must be fully informed about the study, its objectives, and potential outcomes. Obtaining patient consent is a legal requirement, especially in clinical trials. The distinction between experimental and observational studies The distinction between experimental and observational studies lies in intervention—experimental studies involve active interventions, while observational studies observe without intervening. Clinical trials and observational studies serve different purposes: clinical trials focus on testing treatments' effectiveness, while observational studies aim to identify risk factors and causes of diseases. Ethical considerations, such as obtaining informed consent and avoiding harm to participants, are crucial in all human studies. The distinction between experimental and observational studies Selecting the Sample Observational Studies' Sample Selection: Involves random sampling from a larger group to form the sample. Clinical Trials' Sample Selection: Participants meeting specific criteria are invited and allocated to different trial treatments using randomization. Selecting the Sample Random Sampling: Requires a representative sampling frame from the population of interest. Involves giving each individual in the sampling frame an equal chance of being included. Random Allocation in Clinical Trials: Defines a target population based on inclusion and exclusion criteria. Upon meeting criteria, participants are randomized to trial treatments. Simple randomization ensures each individual has an equal chance of being assigned to a treatment, aiding in fair distribution of characteristics that might impact outcomes across treatments. Sample Size Importance of Sample Size: Crucial for study design; too small a sample might miss important differences, while too large a sample wastes resources and delays decisionmaking. Estimating Sample Size for Comparisons: Statistical techniques help determine the appropriate sample size to detect clinically important effects. This estimation involves specifying: • The expected size of the effect or difference being investigated. • Significance level: The threshold for determining if a result is statistically significant. • Power: The probability of detecting a true effect when it exists. Sample Size Expected Size of Effect Crucial in determining sample size. Purpose: Helps avoid excessively large or small studies. Example: For a new oral cancer treatment expected to reduce fiveyear mortality by 10%, a study involving several hundred people would be needed to detect this effect accurately. Effect Measurement: Expressed as relative risk, risk difference, difference between means, or other relevant statistics for comparisons. Basis for Effect Size: Often derived from prior knowledge or, in its absence, determined as the minimum clinically significant effect. Significance Level It's the probability, obtained as a p-value, indicating the likelihood of an observed effect being due to chance. Determines the threshold for accepting or rejecting the presence of an effect. Common Level: Often set at 5%, indicating a 5% chance of concluding an effect when there's none. Stringent Levels: Researchers might choose more stringent levels, like 1%, to minimize the chance of this error and ensure greater confidence in the observed effect. Power in Studies Understanding power in studies is crucial—it gauges the likelihood of detecting a specified difference if it genuinely exists. Typically set around 80% or 90%, power helps avoid the mistake of concluding no difference when there might be one. Estimating sample size involves guesswork, especially in determining effect size. Smaller effects demand larger studies, making it harder to distinguish real differences from random variations. Power in Studies For instance, a trial on sedative gases showcased how a smaller expected effect requires a larger sample size for detection, emphasizing the balance between study size and resource utilization. Importantly, when results are 'not statistically significant,' it doesn't confirm equivalent effects between treatments; there's a possibility of missing a real and crucial effect due to a study's insufficient power, highlighting the significance of adequate sample sizes in research. Cross-Sectional Studies Quick, simple, and cost-effective, these studies capture information from a sample at one point in time. However, they lack the longitudinal aspect of cohort studies and retrospective data collection of case-control studies. Their limitation lies in providing a static view, making it challenging to infer changes over time. Associations between risk factors and disease should be interpreted cautiously as the study's snapshot might favor those with long-term conditions, potentially skewing findings. Cross-Sectional Studies For instance, in a study on gingivitis, the longer someone has the condition, the more likely they are to be included, potentially highlighting different risk factors for long-term versus short-term cases and possibly leading to an unrepresentative sample of the entire gingivitis population. Comparison of Cohort and Case-Control Studies Cohort Studies: Less prone to bias compared to case-control studies due to starting before disease onset, avoiding recall bias. However, they can take many years as they follow individuals over time until enough develop the disease of interest. Less commonly used for rare diseases due to the need for prohibitively large sample sizes. Case-Control Studies: Prone to selection bias in choosing control groups, impacting study validity. Objective in All Studies: Ensure similarity between groups with and without the disease, except for exposure, to minimize bias and confounding factors. More on Obversational Studies Bias in Studies Definition: Bias influences study estimates, causing them to either overestimate or underestimate the true population value. It can stem from study design or execution and is challenging to rectify compared to confounding. Bias in Studies Sources of Bias Subject-Related Bias: Differences in chosen subjects among comparison groups affect study outcomes. Measurement Errors: Influence of belonging to specific comparison groups distorts conclusions. For instance, underreported alcohol intake among individuals with high consumption might weaken associations in alcohol- periodontitis studies. Biases from Sample Selection or Recruitment Selection or Allocation Bias: Participants are chosen in ways that don't represent the population of interest, impacting study validity. Response Bias: Participants who respond to studies differ from non-responders, potentially skewing results. Biases in Exposure or Disease Measurement From Patients: • Recall Bias: Patients with diseases might recall experiences differently than those without, particularly in retrospective studies. • Withdrawal Bias: Patients withdrawing from studies differ from those who don't, potentially causing biases. • Follow-Up Bias: Differences in follow-up due to exposure can lead to biases. From Researchers: • Assessment Bias: Awareness of treatment allocation may influence how results are rated. • Interviewer Bias: Interviewer knowledge of a subject's disease status might impact interview conduct. Comparison of Study Types Clinical Trials vs. Observational Studies: Clinical trials are less prone to biases due to randomization, blinding, and intention-to-treat analysis. Observational studies, like case-control studies, are more susceptible due to selection and recall biases, while cohort studies are less prone because they start before disease onset, drawing participants from the same population. Confounding in Studies Definition: Confounders are variables associated independently with both the outcome and the studied exposure, leading to misrepresented or masked relationships in studies. Common Confounding Factors: Age, gender, and time. Confounding in Studies Addressing Confounding Design Stage: Clinical Trials: Randomization aims to create treatment arms similar in characteristics, minimizing confounding effects. Observational Studies: Methods like matching during sample selection reduce known confounders. Analysis Stage: Clinical Trials: Usually require simpler analyses due to randomization, focusing on treatment effects. Observational Studies: Complex analyses, like multiple regression, are used to account for multiple influencing factors. Interpreting Results: Observational Studies: More prone to bias and confounding, requiring assessment to determine if effect sizes might be influenced by these factors. Larger effect sizes suggest stronger associations less impacted by biases. More on Clinical Trials Clinical Trial Phases: Dental journals mostly feature phase III trials that assess treatment effectiveness in substantial patient numbers. Some phase II trials focus on preliminary safety and efficacy assessments but lack conclusive outcomes. More on Clinical Trials Parallel Group vs. Cross-Over Trials: Parallel Group: Compares treatments between different patient groups. Cross-Over: Every patient receives both treatments, either simultaneously (split mouth design) or sequentially. It eliminates bias or confounding as each patient serves as their control. However, contamination between treatments needs consideration. Randomization of treatment sides or order minimizes biases. Cross-over trials aren't suitable for diseases that are cured since both treatments are experienced. Advantages of Cross-Over Trials: As each patient experiences both treatments, identical treatment groups minimize needed patient numbers compared to parallel trials. More on Clinical Trials Strength of Evidence for Causality from Different Study Types Clinical Trials: Provide the strongest evidence for causality. They establish clear time sequences, employing randomization and blinding to minimize biases and confounding. Cohort Studies: Offer strong evidence for causality as well. They demonstrate a clear time sequence as exposure precedes disease development during the study period. While less affected by bias than case-control studies, both can be subject to confounding. Case-Control Studies: Less robust for establishing causality compared to cohort studies. Sometimes challenging to determine if exposure occurred before the outcome. Prone to confounding. Cross-Sectional Studies: Limited in providing evidence for causality as they cannot determine time sequence or reversibility. General Rule: Clinical trials offer the strongest evidence, followed by cohort studies, while casecontrol studies provide weaker evidence for causality. Cross-sectional studies typically provide limited insights into causality. However, various aspects of study design and execution contribute to overall interpretation beyond this general guideline. References • Allan Hackshaw, Elizabeth Paul, Elizabeth Davenport. Evidence-Based Dentistry, An Introduction. 1st Edition. Wiley-Blackwell. June 2021. ISBN: 978-1-4051-2496-6