Quantitative Research Methods In Political Science Lecture 5 (10/03/2024) PDF
Document Details
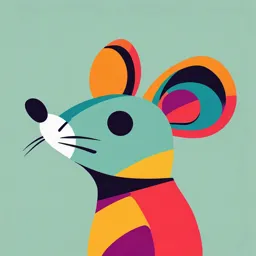
Uploaded by ConscientiousEvergreenForest1127
Toronto Metropolitan University
2024
Michael E. Campbell
Tags
Related
- Quantitative Research Methods in Political Science Lecture 3 PDF
- Quantitative Research Methods In Political Science Lecture 4 PDF
- Quantitative Research Methods in Political Science Lecture 6 PDF
- Quantitative Research Methods in Political Science Lecture 3 PDF
- Quantitative Research Methods In Political Science Lecture 8 PDF
- Quantitative Research Methods in Political Science Lecture 12 (12/05/2024) PDF
Summary
This lecture covers inferential statistics, specifically sampling and sampling distributions, in the context of quantitative research methods in political science. The instructor, Michael E. Campbell, outlines different sampling techniques, including convenience sampling, snowball sampling, and quota sampling.
Full Transcript
Quantitative Research Methods in Political Science Lecture 5: Inferential Statistics (Sampling and the Sampling Distribution) Course Instructor: Michael E. Campbell Course Number:...
Quantitative Research Methods in Political Science Lecture 5: Inferential Statistics (Sampling and the Sampling Distribution) Course Instructor: Michael E. Campbell Course Number: PSCI 2702 (A) Date: 10/03/2024 A Note on Symbols Depending on whether you are working with a sample or a population, the symbols we use to represent statistics differ… Descriptive vs. Inferential Statistics Descriptive Statistics Inferential Statistics Two Purposes for Descriptive Statistics: Allow us to generalize from samples 1. To summarize data or to describe the to population distribution of a single variable (univariate statistics) 2. To describe the relationship between two or more variables (bivariate or In other words… multivariate statistics) Include: Inferential statistics “involve using 1. Proportions, Percentages, Rates, information from a sample (a Ratios carefully chosen subset of the 2. Measures of Central Tendency population) to make inferences 3. Measures of Dispersion about a population” (Healey, 4. Measures of Association Donoghue, and Prus 2023, 19). When we sample, we’re taking a group of cases drawn from a larger population Example #1: if we wanted to know a Samples characteristic about the Canadian population, we would draw the sample from the 41 million plus Canadian population and Sampling Example #2: if we wanted to know a characteristic about Canadian University Students, we would draw the sample from the 1.16 million university students across Canada To survey every single person in these populations would be too expensive and time consuming Samples and Sampling Cont’d The problem is the populations we wish to study are almost always so large that we are unable to gather information from every case… Sampling and Samples Cont’d The solution is to use a sample –i.e., a carefully selected subset of the population Samples and Sampling Cont’d To get an accurate idea of what is occurring in population, the sample must reflect the population (i.e., it must be representative) This is true whether you’re working with people, cities, countries, corporations, etc… Example: You want to know the extent to which respect for politicians affects voter turnout in democratic countries… The population in this instance would be “democratic countries” and the sample would be drawn from these… Two overarching sampling techniques: 1. Non-probability Sampling 2. Probability Sampling Non-probability techniques are used when a researcher is not concerned with representing an entire population or if they lack the resources to select probability sample… Non- Probability Non-probability samples cannot be used to generalize to larger populations… Sampling There are three common types: 1. Convenience Sample 2. Snowball Sample 3. Quota Sample Convenience Samples Targets only individuals who possess characteristics that make them more accessible to researcher Example: researcher lives in Montreal, so they select only people who live in Montreal Problem: cannot generalize to people in Toronto, Calgary, etc… Snowball Sample Most often used for populations that are not easily accessible Examples: People in conflict zones Hard to reach populations (sex workers, drug cartels, etc.) Any population in which the subjects are “out of reach” “Snowball” is used because the sample increases in size as it moves away from source Researcher makes contact with a source, they provide another source, and so on… Until the sample builds and reaches necessary size for desired research Quota Sample The non-probability counterpart to stratified sampling Researcher decides on “strata” – e.g., a specific income bracket Attempt is made to collect sample that is representative of population in categories of interest Is non-random Representativeness However, when you use inferential statistics, the sample must accurately reflect the population In other words, it must be representative of the population A sample is considered representative if it reproduces the important characteristics of the population To achieve this, we use technique known as “Probability Sampling” Probability Sampling Often referred to as “Random Sampling” Selected using careful techniques that are far from random in execution A Bad Example: You want to know something about the Canadian population, so you wait outside your local grocery store and survey the first 1000 people who exit… Question: What is the problem with this? Probability Sampling Cont’d Answer: the sample would not be representative, nor random It would simply give us information on people who were shopping there on that day They would probably live nearby The town might have a certain economic situation (low-, middle-, or upper- class) Might be of certain ethnic background depending on city (e.g., St-Bruno QC vs. Okotoks AB) Probability Sampling Cont’d The goal of probability samples is to achieve representativeness A sample should reflect characteristics from population from which it is drawn Example: if you wanted to know something about Canadian Population, you have to take into account 22.00% are Francophone Traits of population should be reflected in the sample, regardless of size Sample should share same proportions of characteristics as population EPSEM You can never guarantee sample will be completely representative of population… To maximize chances of representativeness, There are refinements to this technique: follow EPSEM principle 1. Systematic Random Samples 2. Stratified (or Hierarchical) Random Samples EPSEM: “Equal Probability of SElection 3. Cluster Samples Method” When using EPSEM, you are ensuring every But in this course, we are primarily case in the population has equal probability concerned with Simple Random Samples of being selected Most basic EPSEM sampling techniques produce Simple Random Samples First, you need to list all elements or cases in a population Selection You also need a system for selecting cases that Process for guarantees that every case has an equal Simple chance of being selected Random Example: you need a sample of 1000 Sample Canadians You could pull their names out of a hat (this is EPSEM) Most commonly, samples are selected using tables of random numbers (which are random and have no pattern to them) A Table of Random Numbers Selection Process for a Random Sample Using Table of Random Numbers First, assign each case on the population list a unique ID number Let’s say you need a sample of 500 students Second, select cases for the sample when their ID number corresponds to Obtain a list of all students at the the number in the table university from the registrar's office Each student has a 6-digit ID number Example: You want to know the (from 000000 to 999999) percentage of students in a university who work during the semester Each time a randomly selected 6-digit number matches the ID of a student, Students at university is 20 000 (N = that student is selected from the sample… 20 000) Student #1 has ID number 501101 Student #2 has ID number 691791 Selection Process for a Random Sample Using Table of Random Numbers Cont’d Repeat the process until you have selected 500 students Part of this list would look like this… Selection Process for a Random Sample Using Table of Random Numbers Cont’d Works towards compilation of random sample, because numbers in table are random… Each number has same chance of being selected as others When you reach desire sample size, stop process Does not guarantee representativeness (known as sampling error) A strength of inferential statistics: “allow the researcher to estimate the probability of this type of error and interpret the results accordingly” (Healey, Donoghue, and Prus 2023, 155). Quick Note on Additional Sampling Techniques Systematic Random Stratified Random Cluster Samples Does notSamples use random table of Samples A series of two or more Used when researcher cannot numbers simple random samples get complete list of population operating within same Selects from list based on population But they can get complete list intervals (e.g., every tenth of groups, or “clusters” case down the list) For example, rather than taking sample of students For example, you want to take As long as there is no inherent from across schools… a sample from Kelowna BC ordering in your data, it should accurately represent population You take a sample from You select from clusters of But not as good as simple within each school streets, as opposed to whole random sample population Sampling Error Samples rarely match population of interest perfectly (there will be “mismatch” between sample and population)… This is referred to as “Sampling Error” Is made up of systematic and random error Examples: 1. Gathering representative sample of homeless populations (hard to get information on population) 2. Issues of Non-Response (certain types of people are less likely to respond to surveys) Selecting a Random Sample In SPSS SAMPLING DISTIRBUTION Sampling Distribution When working with samples, we typically know nothing about the population… If we knew this information, we wouldn’t need the sample Inferential statistics allow us to learn about population, using only information from sample The sample is only important insofar as it helps us learn about the population Sampling Distribution Cont’d To date, you learned three types of information necessary for characterizing a variable: 1. The shape of the distribution 2. Some measure of central tendency 3. Some measure of dispersion All of this can be ascertained for a variable by analyzing a sample But again, we do not know anything about the population! Sampling Distribution Cont’d To link information about sample to population, we use the Sampling Distribution Sampling Distribution: “a theoretical, probabilistic distribution of a statistic for all possible samples of a certain sample size (n)” (Healey, Donoghue, and Prus 2023, 156) Is based on the laws of probability, not empirical information In inferential statistics, there are always three distinct distributions involved… 1. The Sample Distribution: this exists in reality (i.e., it is empirical) and we know that Sampling the shape, central tendency, and dispersion of any variable can be known for the sample Distribution Cont’d 2. The Population Distribution: this also exists in reality (i.e., it is empirical), but information about it is unknown. 3. The Sampling Distribution: this does not exist in reality (i.e., it is non-empirical, or theoretical). But using the laws of probability, we know a great deal about its distribution. Sampling Distribution Cont’d Sampling distribution allows ability to estimate the probability of any sample outcome Like the Normal Curve, it doesn’t exist in reality (is theoretical) It can only be obtained hypothetically… Constructing Sampling Distribution Example #1 We want to know information about the age of a community of a small town (N=10 000) We select an EPSEM sample of 100 people and ask each their age… From this sample, we get a mean age of 27 This is only one sample of a nearly infinite amount that can be collected from a population of this size… Constructing Sampling Distribution Example #1 Cont’d We can collect an infinite number of samples where n=100 from a population of 10 000 The mean of 27 is one of millions of possible sample outcomes Let’s say you take a second sample (n=100) and find the mean is 30 Constructing Sampling Distribution Example #1 Cont’d Let’s say you collected an infinite number of samples and sample means… Each would be slightly different from the other because no two samples will be exactly the same But not every sample will be representative (EPSEM doesn’t guarantee this) Some means will be very high and others very low… We know this because of the Normal Curve Constructing Sampling Distribution Example #1 Cont’d Now, let’s say the true mean of the population is 30 years old The majority of sample means will fall near 30 Since you are sampling into infinity, and randomly, there will be an equal number of “misses” on each side And the sampling distribution will take on a symmetrical shape (i.e., unskewed) All of this information can be summarized in two thereoms… Theorem #1 – Repeated Random Samples “If repeated random samples of size n are drawn from a normal population with mean and standard deviation , then the sampling distribution of the sample means will be normal, with mean and standard deviation ” (Healey, Donoghue, and Prus 2023, 159). In other words, if we begin with a trait that is normally distributed across a population and we take repeated samples of the same size, then the sampling distribution of sample means will be normal in shape (which also tells us about the mean and std. dev.) Theorem #1 Cont’d If we know something is normally The formula for the Standard distributed in the population, the Error is: sampling distribution will also be normal Since it is normally distributed, the meanS 𝜎 E = of the sampling distribution will be the √ 𝑛 same as the population In this equation: is the population standard The standard deviation of the sampling deviation distribution (i.e., the standard error) is n is the sample size equal to the standard deviation of the population divided by the square root of the sample size (n) Therefore, we can estimate the mean and standard deviation of a population using sample statistics Theorem #2 – The Central Limit Theorem But theorem #1 requires that the distribution be normal in shape (what if we don’t know?) “If repeated samples of size n are drawn from any population with mean and standard deviation , then, as n becomes large, the sampling distribution of sample means will approach normality, with mean and standard deviation ” (Healey, Donoghue, and Prus 202, 159). In other words, this theorem tells us that if something (i.e., a trait) is not normally distributed in the population, we can still construct a normal curve if we increase the size of our samples (i.e., if we increase the size of n) The Central Limit Theorem removes the constraint of normality in the population (so long as the sample size is sufficiently large) Demonstrating the Central Limit Theorem Sufficient sample size for normality is 100 (a conservative estimate) But this varies based on: The distribution of the population The size of the population Demonstrati ng The 1. As the sample size increases, the sampling distribution approaches normality Central Limit 2. Even if the population is normally distribution the sampling distribution will become taller Theorem and narrower because the standard error Cont’d decreases 3. The more asymmetrical (i.e., not normally distributed) the shape of the underlying population, the larger the sample needs to be for normality to occur (i.e., n = 100) Constructing a Sampling Distribution Example #2 Let’s say you have a population of 4 people (N = 4) You want to know how much money each has Let’s say you know how much each person in the population has Person A has $2 Person B has $4 Person C has $6 Person D has $8 The population mean is () is $5 The population standard deviation () is Constructing a Sampling Distribution Example #2 Cont’d Noting this, if we take a sample of 2 (n = 2) from our population of 4 (N = 4) We can produce a total of 16 samples with a sample size of 2 and a population of 16 (but we must “sample with replacement”) This will give us 16 different sample means In our first random sample we get the same person with $2 twice This gives us our first sample mean of $2 Constructing a Sampling Distribution Example #2 Cont’d Eventually we will get all possible sample means (see right) We see: Mean of $5 occurs four times Mean of $4 and $6 occur three times each Mean of $3 and $7 occur twice each Mean of $2 and $8 occur only once each Constructing a Sampling Distribution Example #2 Cont’d Distribution of Population Example $9.00 $8.00 $7.00 $6.00 $5.00 $4.00 $3.00 $2.00 $1.00 $- 1 2 3 4 We can visualize the sample And you can see the distribution of distribution in histogram form (see data are symmetrical, even if the above) underlying shape the population is not (see above) Constructing a Sampling Distribution Example #2 Cont’d The mean of the sampling distribution should have the same mean as population The standard deviation of the sampling distribution (i.e., standard error) is equal to the standard deviation of the population divided by the square root of n (i.e., ) This is demonstrated in Table 5.3 The smaller the Standard Error, the more representative the sample is of the population This makes sense, because the Central Limit Theorem stipulates that the larger a sample, the more normally it will be distributed Standard Larger samples more accurately reflect the Error population, because the larger the sample the closer it is to the population size The ‘law of large numbers’ dictates that the larger the sample, the closer its mean will approach that of the sample The standard error quantifies the expected deviation of the sample mean from the population Linking the Population, the Sampling Distribution, and the Population Review Inferential statistics (link sample to pop.) Shape, central tendency, and dispersion of sampling distribution dictated by theorems which tell us: Select a random sample (using 1. so long as the sample is sufficiently EPSEM) large (i.e., n must be greater than 100), we know that the sampling distribution will be normal in shape Gather info. on sample traits 2. the sampling distribution will have the same mean as the population You do not need information on 3. the standard deviation of the sampling distribution (i.e., the pop. (can use concept of Standard Error) of the sampling sampling distribution) distribution is equal to the population standard deviation () divided by the square root of the sample size (n) Linking the Population, the Sampling Distribution, and the Population Review Cont’d Therefore, “the theorems tell us the statistical characteristics of this distribution (shape, central tendency, and dispersion), and this information But the sampling distribution is allows us to link the sample to the population” theoretical – we won’t know its mean (Healey, Donoghue, and Prus 2023, 168). We can’t realistically compute the means The sampling distribution is normal when n is for every possible sample (they are large; therefor… infinite)… 68.26% of sample means will fall within 1 standard errors of the mean (which is same as pop.) But given the theorems that underpin it, 95.44% of the sample means will fall within 2 we will usually only need to take one standard errors means from the mean sample to learn about the population 99.72% of sample means will fall within 3 standard errors from the mean a very small percentage (0.0026%) of sample This is the topic that we will cover for the means will fall beyond 3 standard errors from next several weeks the mean