STA 773 Advanced Econometric Methods Lecture 3 PDF
Document Details
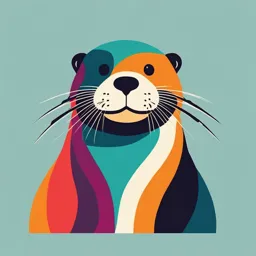
Uploaded by SharperMolybdenum5450
University of Ibadan
Olusanya E. Olubusoye and Ephraim Ogbonna
Tags
Summary
This document provides a lecture on maximum likelihood estimation (MLE), a powerful method in econometrics for estimating regression model parameters. It discusses the principle and properties of MLE, including consistency and asymptotic normality. This lecture is from the course "Advanced Econometric Methods" at the University of Ibadan.
Full Transcript
STA 773: Advanced Econometric Methods Olusanya E. Olubusoye1 and Ephraim Ogbonna2 1,2 Department of Statistics, Faculty of Science, University of Ibadan, Nigeria 1,2 Centre for Econometric and Applied Research (CEAR), Ibada...
STA 773: Advanced Econometric Methods Olusanya E. Olubusoye1 and Ephraim Ogbonna2 1,2 Department of Statistics, Faculty of Science, University of Ibadan, Nigeria 1,2 Centre for Econometric and Applied Research (CEAR), Ibadan, Nigeria ye October 2023 uso Course Outline 1. K-Variable Linear Equation (Johnston and DiNardo 2007) 2. Maximum Likelihood and Instrumental Variables lub 3. Univariate Time Series Modelling 4. Multiple Equation Models 5. Generalized Method of Moments 6. Panel Data.O 7. Discrete and Limited Dependent Variable Models 8. Bayesian Regression (Normal Linear Regression Models) (Koop 2003) aE References Johnston, J. and J.E. DiNardo (2007). Econometric Methods. McGraw-Hill economics series. McGraw-Hill. isbn: 9780071259644. url: https://books.google.com.ng/books?id=GB0InwEACAAJ. ny Koop, G. (2003). Bayesian econometrics. J. Wiley, Chichester. isbn: 9780470845677, 0470845678. usa Ol 1 2 MAXIMUM LIKELIHOOD 2 Maximum Likelihood 2.1 Introduction Maximum Likelihood Estimator (MLE) is a powerful and widely used method for the estimation of the parame- ters of regression models. It is widely used in econometrics, machine learning, and various scientific disciplines. The goal of MLE is to find model parameter values that maximize the likelihood function. Put differently, MLE seeks the parameter values that make the observed data most probable according to the model. It is foundational in statistical modeling and inference. 2.2 The Principle of Maximum Likelihood ye ′ Let y = y1 y2... yn be an n–vector of sample values, dependent on some k–vector of unknown parameters β ′ = β1 β2... βk. Let the joint density be written f (y; β) which indicates the dependence on β. uso Likelihood f unction = L (β; y) = f (y; β) Maximizing the likelihood function with respect to β amounts to finding a specific value, say β̂ , that maximizes the probability of obtaining the sample values that have actually been observed. Then β̂ is said to be the MLE of the unknown parameter vector β. lub In most applications it is simpler to maximize the log of the likelihood function. We denote the log-likelihood by l (β; y) = lnL (β; y) or l = lnL.O δl 1 δl Then δβ = L δβ and β̂ that maximises l will also maximizes L The derivative of L w.r.t β is known as the score, S (β; y) aE The MLE, β̂, is obtained by setting the score to zero, that is, by finding the value of β that solves δl S (β; y) = (42) δβ ny 2.3 Properties of MLEs The major properties of MLEs are large–sample, or asymptotic, ones. They hold under fairly general conditions. usa 1. Consistency P lim β̂ = β 2. Asymptotic normality Ol a β̂ ∼ N β, I −1 (β) That is, the asymptotic distribution of β̂ is normal with M ean =β V ariance = I −1 (β) I (β) is the information matrix " ′ # δ2 l δl δl I (β) = E = −E (43) δβ δβ δβδβ ′ STA 773: Advanced Econometric Methods by Olubusoye & Ogbonna 10 2 MAXIMUM LIKELIHOOD It is usually much easier to evaluate the second expression. When β is a k–vector, δl/δβ denotes a column vector of k partial derivatives, that is, δl /δβ1 δl /δβ2 δl =.. δβ . δl/ δβk ye Each element in this score (or gradient) vector is itself a function of β, and so may be differentiated partially w.r.t. each element in β. uso δ [δl/δβ] h δ2 l 2 δ2 l i = δβ 2 δβδ1 δβl... δβ1 δβk δβ1 1 2 Where the second-order derivates have been written as a row vector. Proceeding in this way yields a square, symmetric matrix of second–order derivative known as the Hessian matrix. δ2 l δ2 l 2 ... δβδ1 δβl lub δβ12 δβ1 δβ2 k δ2 l δ2 l 2... δβδ2 δβl δ2 l δβ2 δβ1 δβ22 k =..... δβδβ ′ ....... 2 2 2 δ l δ l δ l δβk δβ1 δβk δβ2... δβ 2 k.O 3. Asymptotic efficiency The asymptotic variance of β̂ is σ 2 n for single parameter β. √ This is same as n β̂ − β −→ N 0, σ 2. √ aE When β is a vector of parameters and β̂ is the MLE n β̂ − β −→ N (0, V ) for some positive definite matrix V. 4. Invariance If β̂ is the MLE of β and g (β) is a continuous function of β, then g β̂ is the MLE of g (β). 5. The score was zero mean and variance I (β) ny 2.4 ML Estimation of the Linear Model usa The linear regression model is y = Xβ + u with u ∼ N 0, σ 2 I) The likelihood function for a sample of n independent, identically and normally distributed disturbances is −n/2 −u′ u/2σ2 L = 2πσ 2 e (44) The multivariate density for y conditional on X is then Ol δu f (y |X ) = f (u) δy where δu/δy is the absolute value of the determinant formed from the n × n matrix of partial derivatives of the elements of u with respect to the elements of y. Here this matrix is simply the identity matrix. The log–likelihood function is ln L = ln f (y |X ) = ln f (u) n n 1 ′ = − ln 2π − ln σ 2 − uu 2 2 2σ 2 n n 1 ′ = − ln 2π − ln σ 2 − ln (y − Xβ) (y − Xβ) (45) 2 2 2σ 2 (46) STA 773: Advanced Econometric Methods by Olubusoye & Ogbonna 11 2 MAXIMUM LIKELIHOOD The vector of unknown parameters, θ, has k + 1 elements, namely = θ′ = β ′ , σ2 taking partial derivatives gives δl 1 = − (−X ′ y + X ′ Xβ) δβ σ2 δl n n ′ = − 2 + 4 (y − Xβ) (y − Xβ) δσ 2 2σ 2σ (47) ye Setting these partial derivatives to zero gives the MLEs as ′ ′ −1 ′ 2 β̂M LE = (X X) X yσ̂M = y − X β̂ y − X β̂ n (48) uso LE Note: β̂M LE = β̂OLS 2 e′ e σ̂M LE ) = n The variance estimator differs from least squares value by the divisor of n instead on n–k. We know from least–square theory that lub As a general rule, MLEs do not make corrections for degrees of freedom. E (e′ e/(n − k)) = σ 2.O k 2 2 2 2 Thus E σ̂M LE = σ (n − k) n = 1 − n σ < σ So that σ̂ 2 is biased for σ 2 The second–order derivatives are aE δ2 l X ′X X ′X 2 δ l = with − E = δβδβ ′ σ2 δβδβ ′ σ2 δ2 l X ′u δ2 l = with − E =0 δβδσ 2 σ 4 δβδσ 2 ! ny δ2 l X ′X δ2 l n 2 = 4 with − E 2 = δ(σ 2 ) 2σ δ(σ )2 2σ 4 Since E (u′ u) = nσ 2 usa The information matrix 1 is ′ β (X X) 0 I (θ) = I = σ2 n σ2 0 2σ 4 Andits inverse is ′ −1 2 β (X X) σ 0 Ol −1 I = σ2 0 n 2σ 4 The zero off-diagonal terms indicate that β̂ and σ̂ 2 are distributed independently of one another. STA 773: Advanced Econometric Methods by Olubusoye & Ogbonna 12