Learning Bayesian Networks PDF
Document Details
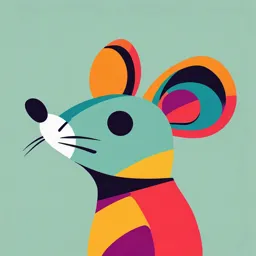
Uploaded by SuperiorSard5855
PPKE-ITK
Kristóf Karacs
Tags
Summary
This presentation details learning Bayesian networks, including probability estimation methods and structure evaluation. It covers concepts like maximum likelihood estimation (MLE), maximum a posteriori estimation (MAP), and likelihood calculation. The example uses a domain with three binary nodes and structures with nodes A, B, and C.
Full Transcript
Learning Bayesian Networks Artificial intelligence Kristóf Karacs PPKE-ITK 1 Learning Bayesian Networks n Sources for Bayesian Nets ¨ Human experts ¨ Data (measurement...
Learning Bayesian Networks Artificial intelligence Kristóf Karacs PPKE-ITK 1 Learning Bayesian Networks n Sources for Bayesian Nets ¨ Human experts ¨ Data (measurement) n What can be learned? ¨ Structure ¨ Probabilities n Typical case: structure from expert, probability from data 2 1 Learning probabilities n Given a data set D = {, …, } n m: # of nodes k: # of samples n Elements are assumed to be independent given M n Maximum likelihood estimate ¨ Find model M that maximizes P(D|M) 3 Estimation techniques n Bayesian prediction (optimal) ¨ Any probability connected to the observations is best estimated by concurrently considering all possible model (i.e. network structure) ¨ Not practical | = | | n Maximum a-posteriori estimation (MAP) ¨ Select the most likely structure | ℎ = argmax | = argmax = argmax | n Maximum likelihood estimation (MLE) ¨ In lack of information on a priori probabilities of structures, assume a uniform distribution, i.e. ∀ , : ≈ ℎ = argmax | = argmax | ≈ argmax | 4 2 Estimating probabilities V1 V2 V3 #( ) n ≈ #( ∧ ) n | ≈ #( ) #( ∧ ) n |¬ ≈ #( ) 5 Bayesian correction V1 V2 V3 #( ) n ≈ #( ∧ ) n | ≈ #( ) #( ∧ ) n |¬ ≈ #( ) 6 3 Measuring goodness of fit n Likelihood ¨ =∏ = ∏ ∏ = , n Log likelihood ¨ Easier to calculate and takes its maximum at the same point ¨ log = log ∏ ∏ = , = ∑ ∑ log = , 7 Learning the structure n For a fixed structure, maximum likelihood estimates for the CPT values are reasonable n For structures the best MLE model will have no conditional independence relationships (every node connected to every other) ¨ Undesirable, because of overfitting: good performance on training data, but low generalization capability ¨ Generalization arises from simplicity 8 4 Evaluation of structures n Two conflicting objectives ¨ good fit to data: maximize log likelihood ¨ low complexity: minimize total number of parameters n Maximize scoring metric by varying M (structure and parameters) given D log P(D|M) − α #(M) 9 Search in structure space n Brute-force method cannot be applied n Local search in structure space ¨ Starting with some initial structure ¨ Operators: add, delete, or reverse an arc ¨ Choosing the next state n Evaluation of candidates using maximum likelihood parameters n Hill climbing, simulated annealing n No directed cycles should occur in the structure 10 5 Initialization possibilities n No arcs n With a random ordering V1 … Vn ¨ variable Vi has all parents V1 … Vi-1 ¨ variable Vi has parents randomly chosen from V1 … Vn-1 n Best tree-network ¨ maximum-weight spanning tree based on pairwise mutual information between every pair of variables ¨ polynomial time algorithm 11 Bayesian net structure example n Domain with 3 binary nodes A n Measurement data ¨ {(0,1,1),(0,1,1),(1,0,0)} n Consider three structure candidates B C ¨ M1 {} ¨ M2 {A®B, A®C} ¨ M3 {B®A, C®A} n 1. Calculate parameter estimates for the CPTs n 2. Calculate P(D|Mi) for each structure with Bayesian correction 12 6