PSYC4050 Course Overview PDF
Document Details
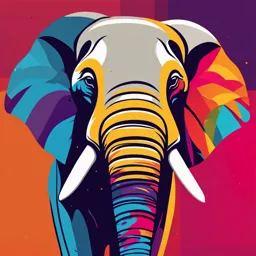
Uploaded by EvocativeDallas
UQ
Brendan Zietsch
Tags
Summary
This document is a course overview for a psychology statistics course, PSYC4050. It details the course's topics, including missing data, assumptions, exploratory and confirmatory factor analysis, logistic regression, mediation, and moderation analyses, as well as Bayesian inference. The overview also details the assessment structure, including assignments and a final exam.
Full Transcript
PSYC4050 COURSE OVERVIEW Lecturer: Brendan Zietsch Who am I? Why am I here? Undergrad in psychology (UQ) PhD in quantitative genetics (QIMR Berghofer) Postdoctoral research in evolutionary psychology and behavioural genetics (UQ) Now teaching you guys stats as w...
PSYC4050 COURSE OVERVIEW Lecturer: Brendan Zietsch Who am I? Why am I here? Undergrad in psychology (UQ) PhD in quantitative genetics (QIMR Berghofer) Postdoctoral research in evolutionary psychology and behavioural genetics (UQ) Now teaching you guys stats as well as doing research Science and stats Why do science? Describe phenomena in the world Predict outcomes of new events Explain why the world is the way it is How do we do it? Ideas (e.g. theories, hypotheses) Stats! Evidence (e.g. data) So why are we here? We need to understand stats to: Do research Evaluate research Understand the world Ideas (e.g. theories, hypotheses) Stats! Evidence (e.g. data) But I’ve already done stats! As a scientist, you need to either find new ways to answer old questions (why has no one answered in this way before?) find new questions (why has no one asked this question before?) Advanced statistical techniques can help open up a wider range of questions answer old questions better by reducing error, biases, and confounds As a clinician, you’ll need to consume science and therefore understand what the scientists are doing …including advanced statistical techniques A note on rules in statistics You may be used to learning rules for performing and interpreting statistical analyses As this stats class is the final one of your degree, meant to launch you into the world of real research, it’s time to level with you… There are no hard and fast rules In real research, statistical decisions are based on common sense and reasoning justifications specific to the situation at hand an ever-advancing methodological literature What is crucial is understanding the fundamentals of the methods so that you can make reasonable decisions and justify them appropriately Course Topics (available on ECP) Week Starting Lecture and Q&A Topic Tutorial Topic 19-Feb Course Overview No tutorial 26-Feb Missing Data: Assessment & Solutions Missing Data Analysis 4-Mar Assumptions Assumptions 11-Mar Exploratory Factor Analysis Exploratory Factor Analysis 18-Mar Confirmatory Factor Analysis & SEM Reading CFA & SEM Results 25-Mar Logistic Regression Logistic Regression 1-Apr Midsemester Break Midsemester Break 8-Apr Mediation Analysis with Bootstrapping Mediation Analysis with Bootstrapping 15-Apr Moderation Analysis with Regression Moderation Analysis with Regression 22-Apr No lecture (ANZAC Day) No tutorials (due to ANZAC Day) 29-Apr Moderation Analysis with ANOVA Moderation Analysis with ANOVA 6-May No lecture (due to Labour Day) No tutorials (due to Labour Day) 13-May Bayesian Inference Bayesian Inference 20-May No lecture No tutorials Course Content Emphasizes: conceptual understanding of analytic techniques statistical decision-making conducting analyses in SPSS (where appropriate) It doesn’t matter* if interpreting and understanding output you don’t know how critical evaluation of published results to do all these Formulae and computations are not the focus analyses yourself! Assumed familiarity with 3rd year statistics (i.e., PSYC3010) Review material posted on Blackboard *much… Course Structure One recorded lecture per week (all available beforehand) Covers theory, presents examples and applications When can I use this technique? What constraints does the technique impose on study design? What questions does the technique address? Weekly Workshop/Q&A session focussed on clarifying and deepening understanding of the week's lecture material In these sessions we will, in an interactive way, work through the logic of example exam questions. I will also answer any questions you may have about the lecture material, anything you feel you may not have fully understood, or are unsure about. Aim is to go beyond a surface level understanding of the lecture material. With statistics, students can often have good knowledge of the relevant terms and analyses while lacking the deeper understanding that is crucial for critically interpreting and planning analyses in the messy reality of research – and for correctly answering the quiz questions that are designed to test such understanding. By working through difficult quiz questions, why the wrong answers are wrong and the right answers are right, we will identify and correct misunderstandings and clarify tricky concepts. One 2-hour tutorial per week Practical details involved in conducting analysis in SPSS How do I interpret the results? What do they mean? How to critically evaluate published research Tips and consultation for assignments! Housekeeping: Tutorials Tutorial allocation is done via Class Sign-on You must sign up for a tutorial! Sign-on is how assignments are allocated to tutors You must attend the tutorial you signed up for Ad hoc swaps and changes: Seriously messy with limited computer lab space! If you absolutely cannot make your allocated time, contact me immediately. Tutorials: In Case of Emergency! Contact your tutor and whoever is taking the class you plan on attending instead Ensure all parties are aware Host tutor must agree to you attending their class—may not always be possible due to limited space Course Website on Blackboard Lectures Lecture recordings and slides will be available from the start of semester Tutorials Handouts available on Monday Notes with answers available after final tutorial for the week Assessment Overviews and data files Applications for extensions and re-marks (more later) Turnitin submission links Course Website on Blackboard Textbook and Readings No set textbook or readings for the course But feel free to Google more information, detail, different explanations of a topic etc, if that will help supplement your understanding of the lecture content Supplementary material provided for your own reference (e.g., for your thesis and other research projects) Additional Resources content area on Blackboard These materials are not assessable, nor will they appear in any assessment They are there for you to cast a critical eye over, and serve as points for discussion (e.g. between you and your supervisor) Textbook and Readings No set textbook or readings for the course But feel free to Google more information, detail, different explanations of a topic etc, if that will help supplement your understanding of the lecture content Supplementary material provided for your own reference (e.g., for your thesis and other research projects) Additional Resources content area on Blackboard These materials are not assessable, nor will they appear in any assessment They are there for you to cast a critical eye over, and serve as points for discussion (e.g. between you and your supervisor) Assessment Details Two assignments (each worth 30% of course grade) A1 – Missing data analysis, assumptions testing, and multiple regression A2 – Exploratory factor analysis, logistic regression, & mediation Submission Dates are on the Electronic Course Profile Online submission via Turnitin Final exam (40% of course grade) Centrally administered Multiple choice (we will go through example questions in the workshops) Open-book Further details to follow Assignment Extension Policy Submit your application before the due date! Electronic form available via the ECP – assessed by admin team (not me) An application does not guarantee approval! Illness or incident must be within 2 weeks of due date Ongoing issues that have affected you within 2 weeks of the due date Evidence is required to support application Medical certificate, police report, counsellor’s report, etc. Late submission penalty is 10% of the total mark deducted per late day or part thereof Saturdays, Sundays, and holidays all count as distinct days! Requests for Re-marks Step 1: Wait 1 week after the assignment has been returned before considering a re-mark Gives your tutor time to complete other marking for late assignments, etc. Step 2: Approach your tutor and ask for further feedback Opportunity for you and your tutor to discuss why you disagree with the mark you received, and why they gave the mark to begin with Step 3: If you still disagree with the mark, you are entitled to request a re- mark by a second, independent, marker Step 4: Submit a Request for Re-mark form within 30 days of assignment return date (form available on Blackboard) Note your second mark may be lower than the first (and often is) And the second mark is final! Tips and Tricks: How to do well in the course Show up to the workshops/tutorials Focus on understanding the content, rather than just surface knowledge If you’re not sure you understand, ask – and ask again Prepare for classes by reviewing lectures and tutorial handouts before class Revisit lectures, tutorial handouts, and notes after class Try not to fall behind—catch up on missed classes as soon as possible Tips and Tricks: How to do well in the course Don’t panic… Much of this stuff actually isn’t really tricky… Many analyses follow the same basic logic Many analyses are extensions of familiar analyses (like linear regression) Our focus is on the concepts and rationale behind different analyses The mathematical details are not the focus Opting for breadth of coverage over depth MISSING DATA Missing Data Definitions and Potential Problems 1 2 Male mean: 44.5 Female mean: 3 Total mean: 23.75 3 Missing data can be problematic Means ranged from 6 to 44.5 for males and 3 to 20 for females. This is an exaggerated example, but in real surveys, men generally report having around twice as many lifetime opposite-sex partners as women do, on average – even though in reality they must be equal… 4 5 6 Spurious effects due to selection bias Among American college students, being academically gifted is inversely related to being good at sport. Among people who have had a heart attack, smokers have better subsequent health than non-smokers. Among low birthweight infants, those whose mothers smoked during pregnancy are less likely to die than those whose mothers did not smoke. Spurious effects due to selection bias Think about selection bias in your own study E.g. if you are using the UQ student participation pool, your participants are highly selected on academic achievement (and thus probably IQ, contentiousness) age More broadly, most psychology studies use WEIRD samples from Western, Educated, Industrialised, Rich, Democratic societies What else? Anything specific to your particular study? 7 Selection bias vs missing data Conceptually similar potential problems, but Selection bias relates to who participates Missing data relates to what data participants do or don’t provide With missing data you can get an idea of what you’re missing and how problematic it is 8 9 Diagnosing Problems Caused by Missing Data Reduced Power, Biased Estimates, or Both? How problematic are missing data? Missing data can be benign If there is very little of it (e.g. 1.96 or < -1.96)? But this is of little utility Underpowered in small samples (where skewness might slightly matter) Will nearly always indicate skewness in large samples (where skewness is irrelevant) Assumption: Normally distributed residuals So this is not an important assumption, and can be usually be ignored Discussed in a very nice paper by Knief & Forstmeier (2021) Title: “Violating the normality assumption may be the lesser of two evils” If you’re concerned about thesis markers (or journal reviewers) being leery of your non- normal distributions, you can cite this paper to justify going ahead with the analysis 9 Assumption: No overly influential observations Although non-normal distributions aren’t in themselves problematic, they can make highly influential observations more likely …and these can be problematic Raise Type I error rate, bias regression estimates Outliers are values that are extreme, given the structure of the data Univariate outliers are simply observations far away from the mean, given the overall spread (variance) of the data E.g. observations >3 (or 3.29) standard deviations from the mean Multivariate outliers are observations whose value on one variable is far from what you would expect given its values on other variables E.g. a person weighing 55kg is not extreme, considered alone (univariate); nor is a person who is 1.85m tall. But the same person with both those measurements is extreme in multivariate space. Assumption: No overly influential observations Detecting multivariate outliers Mahalanobis distance A multivariate extension of univariate distance from mean Gives distance from centroid in multivariate space for all predictors (IVs) Check against cut offs based on Chi square distribution p 1 very influential, may be problematic 10 Assumption: No overly influential observations High leverage, low Very influential outlier influence outlier Assumption: No overly influential observations Detecting multivariate outliers Mahalanobis distance A multivariate extension of univariate distance from mean Gives distance from centroid in multivariate space for all predictors (IVs) Check against cut offs based on Chi square distribution p 1 very influential, may be problematic 11 Assumption: No overly influential observations Are the outliers real? Often multivariate outliers can be identified as obviously misentered data, or simply unrealistic – best to delete If they are real, again the course of action depends on the sample size Less problematic in a larger samples, because of the Central Limit Theorem – extreme values are expected if you collect enough data and won’t markedly affect the regression In a small sample, an extreme value is unlucky and could have a large impact on the regression – in this case, often best to regression with and without the outlier If the results are substantively different, this needs to be reported so the reader can judge results with appropriate caution Assumption: Homoskedasticity Equal variance of observations around the regression line In other words, variance of residuals is consistent across values of the predictor Y X 12 Assumption: Homoskedasticity Equal variance of observations around the regression line In other words, variance of residuals is consistent across values of the predictor Y residual Y Y Y predicted X X Homoskedasticity (roughly) Heteroskedasticity Heteroskedasticity Assumption: Homoskedasticity Is this one important?... Yes, excessive heteroskedasticity can bias estimates and lead to Type I error inflation – i.e. increased likelihood of spurious effects = bad There are various tests for heteroskedasticity E.g. Breusch–Pagan test White test – we’ll look at this one in the tutorial 13 Assumption: Homoskedasticity What causes heteroskedasticity? More likely in highly skewed data, with very long tails Transforming the dependent variable might help E.g. log transform Unmodelled variables, e.g. a moderator – different processes going on at different levels of the predictor Nonlinear effects Also helped by transforming variables What to do? Transform DV and see if it helps Think about what other variables might be at play Use heteroskedasticity-consistent standard errors (see tutorial) NB: doesn’t fix the underlying model-misspecification, so coefficients may still be biased Tell the reader so they can interpret the findings with appropriate caution Assumption: Independence of observations AKA independence of residuals or errors – effectively the same thing Is this one important?... YES, very. Non-independence of observations overestimates the amount of independent evidence provided by the data points, If you don’t account for that then the precision of your estimates is exaggerated -> Type I error inflation – i.e. increased likelihood of spurious effects = bad 14 Assumption: Independence of observations What does it mean? Measurements for each data point are in no way influenced by or related to the measurements of other subjects Examples of non-independence Clustered data Multiple measurements of a variable from each individual Study participants are grouped in some way Family members Schools or classes By different experimenters Tested in sessions Serial data E.g. measures taken at time intervals Measures closer in time might be more likely to have similar errors than measure further apart Test with Durbin–Watson statistic Problem can also apply with spatially dependent data Assumption: Independence of observations What to do about non-independence? Clustered data Multi-level modelling – we’ll cover this later in the course Accounts for data structure Cruder solution – take mean of the clusters Will reduce the Type I error inflation because you’re no longer pretending you have more evidence than you really have However, you may now be using less evidence than you really have, which reduces power – not as severe a problem as Type I error inflation (i.e. it’s conservative) Serial data Other techniques, which we won’t cover in this course Autoregressive Integrated Moving Average Model – uses differences from one cell to next as input data 15 Assumption: No multicollinearity Multicollinearity is excessive correlation among predictors Some correlation between predictors is normal, and indeed if predictors were uncorrelated there would not be much use doing multiple regression But many high correlations can make regression weights for each predictor imprecise, unstable, and very hard to interpret Doesn’t affect the overall regression – e.g. R² is unaffected IV1 a Unique contributions b (a and c) small Overlapping DV contribution c IV2 (b) large Assumption: No multicollinearity Is this one important? Depends what you need your regression for If you’re only interested in the overall model, then it’s not a problem But if you’re interested in understanding the effects of the individual predictors, it can make the results useless or misleading How do we detect it? First step: just look at a correlation table of your predictors Any really high? (over.8 or.9) Can multicollinear variables be combined? (e.g. averaged to form one measure) Limitation – this is only checking bivariate relationships, whereas multicollinearity is a multivariate phenomenon Second step: look at formal indicators of multicollinearity 16 Assumption: No multicollinearity Formal indicators of multicollinearity (come in standard multiple regression output) Based on squared multiple correlations (R²) for each IV acting as a DV for the other IVs Yields two measures for each IV: Tolerance (1 – squared multiple correlation) What proportion of the variance in this IV cannot be explained by the other IVs? Multicollinearity problematic if Tolerance is less than 0.2 i.e. less than 20% of the variance in that IV is not explained by the other IVs Variance Inflation Factor (VIF) = inverse of Tolerance (1/tolerance) By what factor the standard error of the estimate of the beta has increased for that IV E.g. if 2 the SEE for that beta is 2 times what it would be if the IVs were uncorrelated > 5 problematic Assumption: No multicollinearity What to do if problems are detected in these formal tests? Go back and look if any variables can be combined (or deleted) Factor analyse the set of independent variables Centre or standardize independent variables Can reduce their intercorrelation of main effects with their interaction terms, if you have both in the model Collect more data Larger sample sizes increase precision of betas, counteracting the precision-decreasing effect of multicollinearity Don’t worry about it If you’re mainly interested in the overall model If you have a really large sample and are confident interpreting the betas of highly correlated predictors 17 Sum up and recap of our philosophy on assumptions testing Important to know about assumptions underlying statistical tests because only if these assumptions hold in our data are we sure that the outputs of the tests are strictly accurate However, real life data rarely conform precisely to these assumptions, so we are usually in a situation where we need to use informed judgement about how problematic various violations are Fortunately, regression-based analyses are generally robust to distributional assumptions, so we can be pretty relaxed about those Independence of observations is perhaps the most important one to be careful of The others may depend on various factors such as the aims of your analysis, the size of the sample, and the severity of the assumption violation Being knowledgeable about assumptions helps to navigate these decisions Above all, be transparent – don’t use researcher degrees of freedom to p-hack 18 EXPLORATORY FACTOR ANALYSIS Today’s Lecture Conceptual introduction to Exploratory Factor Analysis (EFA) Aims of the analysis Research questions EFA is applied to How Exploratory Factor Analysis works Preparing to conduct an EFA How many factors should I retain? Which method should I use to extract factors? 1 Conceptual Introduction to Exploratory Factor Analysis What are we doing when we do research? Trying to answer questions about psychological processes! Does intelligence predict life satisfaction? Is attention related to anxiety? We can’t directly observe the things we’re interested in researching! The Problem (Townsend & Ashby, 1983) 2 How psychologists have held onto their jobs… We come up with indirect ways of measuring things Questionnaires, experimental tasks, etc. Directly observable responses reflect unobservable psychological constructs We operationalize our theoretical constructs so when we run statistical tests on observable measures, we use them as a shorthand to talk about psychological constructs e.g. Differences in state anxiety scores described as “differences in anxiety” But we can only do this if our measures accurately reflect the psychological constructs we’re talking about! What Exploratory Factor Analysis Offers We often collect data on far more variables than we care to talk about (usually because measuring one thing with multiple variables increases reliability of the measurement) Simplification of a complex data set Organizes similar variables by assessing shared variance in responses Hypothetical constructs: not directly measured in study Meaning of constructs is based on content of variables and relevant theory Can help clarify what construct(s) variables are measuring Allows us to judge whether variables measure what we think they do 3 Example of Data Reduction Running Sums Lexical Decision 2+5+3–8+3=? HAND 7–2+4–7+5=? JUND Logical Sequences 5+4+5–9–2=? BOOK Speeded Algebra BIRD 3, 9, 10, 30, 31, 93, ? Scores on 6 BORT 2, 4, 6, 8, 10, 12, ? a7 3, 5, 2, 4, 1, 3, ? Different Tests 3 4 , solve for a. Linguistic Ability vs. Sentence comprehension Mathematical Ability? “He turns his head, surrendering the alabaster mask of his face to my fingers, which press Spelling Test everywhere because I am making memories.” – Bernadette Vaughn, Obbligato Ap_le Bartend_r Convenien_e Summarizing Data with Factors Factors directly summarize commonalities among different measures Unobserved “latent variable” Operationally defined in terms of how measures relate to one another Interpretation of a factor (i.e. what it means) depends on the variables the factor is derived from Not all measures contribute equally to a factor Factor loading refers to the correlation between an observed measure and the latent (unobserved) factor Exploratory Factor Analysis can help clarify questions about variables 4 Exploratory Factor Analysis: Example Research Questions Research Question #1 What constructs are assessed by a set of variables? Making sense of satisfaction data Hypothetical hospital develops a patient satisfaction questionnaire 50 Questions, grab-bag of topics Contribution of EFA Can show that responses to the 50 questions “cluster” around specific issues These factors constitute different bases or “dimensions” of patient satisfaction (e.g., waiting time, interactions with staff, comfort, etc.) Can create composite measures of patient satisfaction 5 Research Question #2 What is the underlying structure of a construct? Is intelligence a uni-dimensional or multi-dimensional construct? Intelligence Intelligence Intelligence Type 1 Type 2 vs Test 1 Test 2 Test 3 Test 4 Test 5 Test 1 Test 2 Test 3 Test 4 Test 5 Contribution of EFA Administer multiple tests to extract underlying factor structure Can investigate relationships between different tests and different factors Research Question #3 Are constructs clearly distinct from one another? Do measures of self-esteem and self-worth tap the same construct? Two 5-item measures developed independently Constructs seem close, but we may need to measure both if they’re different Contribution of EFA Do the 5 self-esteem items and the 5 self-worth questions load onto a single factor, or do they load onto two distinct factors? Can examine the relationship between the factors—quantifying the overlap 6 How Exploratory Factor Analysis Works: A Conceptual Overview Variables, Variance, and EFA EFA is interested in relationships between different variables Are there patterns in the way our variables correlate with one another? Unique variance vs. shared variance (communality) Uniqueness: Proportion of variance that is not shared with other variables Communality: Proportion of variance that is shared with other variables Pattern of communalities determines the factor structure Reflects sub-groups of variables that correlate highly with each other, but have low correlations with other variables 7 From Shared Variance to Factors We assume patterns in our observed variables are driven by common causes (e.g. underlying psychological traits, processes) Goal of EFA is to arrive at a parsimonious factor structure Factors are clearly distinguishable but not too numerous! Two Step Process Extraction of Factors: capture as much shared variance as possible across all the extracted factors Rotation of Factors: Simplify the extracted factor structure What Happens During Extraction… Walking through a very simple scenario Suppose we administered tests of vocabulary and maths to people… Vocabulary Score Why talk about two things when we can potentially just talk about one? Is there a better way of representing our data? Maths Score 8 What Happens During Extraction… Walking through a very simple scenario Suppose we administered tests of vocabulary and maths to people… We now have a scatterplot in k-dimensional space Vocabulary Score Can we summarize the data in fewer than k dimensions? Pass k eigenvectors through data to capture shared variance Maths Score (eigenvector = factor) What Happens During Extraction… Walking through a very simple scenario Suppose we administered tests of vocabulary and maths to people… Once we remove variance along the first factor, there Vocabulary Score is only variance along one other dimension Maths Score 9 What Happens During Extraction… Walking through a very simple scenario Suppose we administered tests of vocabulary and maths to people… Once we remove variance along the first factor, there Vocabulary Score is only variance along one other dimension If we had more variables, there’d be more possible dimensions Maths Score What Happens During Extraction… Walking through a very simple scenario Suppose we administered tests of vocabulary and maths to people… Factor Loadings: What is the We started with vocabulary correlation between each f1= intelligence f1 score and maths score, observed variable and a given Vocabulary Score now we have two different factor? factors to describe the same data: Maths Vocabulary Intelligence, and Relative strength in f1 r = 0.5 r = 0.6 f2= relative strength in f2 vocabulary vs maths f2 r = 0.4 r = -0.3 vocabulary vs maths Maths Score 10 What Happens During Rotation… Walking through a very simple scenario Suppose we administered tests of vocabulary and maths to people… f1 Variance explained is the same as before, but the factor loadings are different Vocabulary Score (and sometimes more interpretable) f2 Maths Vocabulary f1 r=1 r=0 Maths f2 r=0 r=1 Maths Score (in this case the rotation is pointless, as it gives us back our measured variables) What Happens During Rotation… f2 = relative strength at verbal vs. non-verbal IQ (but can be useful with Vocab multiple variables) Information Comprehension f1 = general intelligence Maths Spatial rotation Matrix reasoning 11 What Happens During Rotation… f2 = Verbal intelligence (but can be useful with Vocab multiple variables) Information Comprehension Maths Spatial rotation Matrix reasoning f1 = Non-verbal intelligence But before rotation… How many factors to extract? Eigenvectors (factors) are extracted to account for all variance in the data But researchers rarely retain all factors Some factors just pick up on noise in the data Some factors explain very little variance Some factors may include only a single item So you need to establish… max=2 factors How many factors you are looking for (theory-driven) max=6 factors and / or How many substantive factors you have evidence for (data-driven) Various stopping rules are used to this end 12 Theory-driven extraction: A priori decision Based on previous knowledge of the constructs (i.e., the literature), you decide on the number of factors you want to extract from data Analysis is constrained to extract only this number of factors Pros Scientifically appropriate to make a priori decisions Constrained analysis might reduce severity of interpretation problems Cons If existing theory is underdeveloped, exploration is limited… Can’t address questions about the number of factors underlying constructs Data-driven extraction: based on eigenvalues Eigenvectors will be generated until all shared variance is explained or get to the k-th eigenvector (i.e. as many factors as variables) max=2 factors max=6 factors The amount of shared variance explained by an eigenvector is called its eigenvalue – scaled such that 1 is the amount explained by a single measured variable (incidentally, a factor’s eigenvalue is the sum of its squared factor loadings) 13 Data-driven stopping Rule #1: Kaiser’s Criterion If an eigenvalue > 1, a factor explains more variance than a single measured variable—achieves some degree of data reduction If an eigenvalue < 1, a factor explains less variance than a single measured variable—does not achieve data reduction Kaiser’s Criterion: retain every factor with eigenvalue > 1 Pros and Cons Permits “true exploration” of the data—no a priori commitments to factor structure Data-driven stopping Rule #2: Scree Test Scree plot: Shows eigenvalues for each extracted factor Discontinuity Principle: Retain factors associated with the steeply descending part of the plot Draw a straight line summarizing the descending part of the plot Draw a straight line summarizing the flat part of the plot Lines intersect at the point of inflection Retain number of factors to the left of the point of inflection (i.e. the non-scree factors) 14 Data-driven stopping Rule #3: Horn’s Parallel analysis Parallel analysis basically simulates a random dataset with the same number of observations and variables Components in the real dataset with eigenvalues less than the same component in the Parallel analysis are probably noise and are discarded Limitation: sensitive to sample size More factors in larger samples More in the tutorial… Data-driven stopping Rule #4: Velicer's Minimum Average Partial test Another method trying to distil the systematic variability in the data from the individual variability Do a factor analysis with only one factor, remove the variance of that factor from the correlations among variables, then calculate the average squared partial correlation between observed variables Do a two-factor analysis, remove these two factors’ variance from the correlations among variables, then calculate the average squared partial correlation between observed variables Repeat for k-1 factors, where k is the number of variables Extract number of factors that minimizes the average squared partial correlation between observed variables 15 Choosing a Stopping Rule If you have strong a priori ideas, specify the number of factors If you really are exploring, use a post-hoc method: Kaiser, Scree, Parallel analysis, Minimum Average Partial test Note that approaches might lead to different conclusions… Best approach is to try multiple approaches and check for consistency and clarity of interpretation Limitations of Extraction Extraction is a blunt instrument Each factor seeks to explain as much variance as possible But it doesn’t care about which variables contribute to each factor Especially so for “late” factors operating under more constraints Complex factor structure could emerge Factors could have variables with high loadings as well as variables with low loadings—makes it difficult to interpret some of these factors! Can make it difficult to cleanly identify meaningful subgroups of variables Solution: Try to simplify the structure with rotation 16 Rotation In the extraction phase, the first factor gobbles up all the variance it can, the next one gobbles up all of the remaining variance that it can, and so on This gives the factor structure a particular ‘shape’, which may not be ideal for interpretation Rotation realigns the factors (and factor loadings) in ways that can be more interpretable But rotation can only produce a clear factor structure if a clear factor structure exists in the data-set A More Realistic Example We want to measure disgust sensitivity So we give people a questionnaire asking how disgusted they are by 7 seven different things Not at all disgusting Extremely disgusting 17 A More Realistic Example A More Realistic Example Factor 1: substantial loadings from V1, V2, V3 – all other variables have negligible loadings Factor 2: substantial loadings from V4, V5, V3 – all other variables have negligible loadings Factor 3: substantial loadings from V6, V7, V1 – all other variables have negligible loadings 18 Next:Rotation Simplify the factor structure Maximize high loadings, minimize low loadings for each factor How? Eigenvectors (aka axes/factors) are rotated to better capture subsets of variables with high loadings Changes pattern of shared variance accounted for by factor Not every eigenvector needs to be rotated How Does That Work? Eigenvalue: Sum of squared loadings for a factor Increases in high loadings are matched by decreases in low loadings Across factors, the sum of eigenvalues therefore stays constant Where does rotation make the biggest difference? Variables that loaded onto multiple factors Less so for variables that loaded strongly onto a single factor Benefit is that this can “clean up” the factor structure 19 V2 Factor Rotation V2 V3 V3 V1 V1 V4 V4 V5 V5 V7 V7 V6 V6 Factor 1: substantial loadings from V1, V2, V3 V1 Stepping in dog poo – same as in extraction stage V2 Touching someone’s open wound V3 Seeing a cockroach on the floor Factor 2: substantial loadings from V4, V5 – changes in loadings: V3 now smaller; V4 and V5 now larger V4 Performing oral sex V5 A stranger rubbing your thigh on the bus Factor 3: substantial loadings from V6, V7 V6 A student cheating on a test – changes in loadings: V1 now smaller; V6 and V7 now larger V7 Someone cutting in line V2 Factor Rotation V2 V3 V3 V1 V1 V4 V4 V5 V5 V7 V7 V6 V6 V1 Stepping in dog poo Pathogen disgust V2 Touching someone’s open wound V3 Seeing a cockroach on the floor Sexual disgust V4 Performing oral sex V5 A stranger rubbing your thigh on the bus Moral disgust V6 A student cheating on a test V7 Someone cutting in line 20 For the paper reporting the real factor analysis and scale development, see Tybur, J. M., Lieberman, D., & Griskevicius, V. (2009). Microbes, mating, and morality: individual differences in three functional domains of disgust. Journal of Personality and Social Psychology, 97(1), 103. And for an application of it, see… Pathogen disgust positively associated with preference for physical attractiveness Moral disgust negatively associated with preference for physical attractiveness, positively associated with preference for intelligence Lee, A. J., Dubbs, S. L., Kelly, A. J., von Hippel, W., Brooks, R. C., & Zietsch, B. P. (2013). Human facial attributes, but not perceived intelligence, are used as cues of health and resource provision potential. Behavioral Ecology, 24(3), 779-787. 21 Next lecture… 22 EXPLORATORY FACTOR ANALYSIS II How to do it AKA: The details 1 Doing Exploratory Factor Analysis Remember that the broad aim of EFA is to simplify a data set Reduces the number of measured variables to a smaller number of unobservable latent variables (i.e. hypothetical constructs) Five Steps Step 1: Planning for the analysis Step 2: Decide on the number of factors to retain (if taking theory-driven approach) Step 3: Choose an extraction method Step 4: Choose a rotation method Step 5: Interpret the solution Step 1 Planning for the Analysis 2 Data Collection Which variables / items to assess? Depends on which constructs you want to differentiate How many variables / items are needed? Depends on the number of factors you think exist… Kline (1994) suggests a minimum of 3 variables per factor Tabachnick and Fidel (2007) suggest 5-6 variables per factor How many participants / cases are needed? Depends on the number of variables you have measured Child (1990) suggests 2 times the number of variables Bryman and Cramer (1997) suggest 5 times the number of variables Howitt and Cramer (2008) suggest no fewer than 50 participants Checking Assumptions Are the data measured on an interval scale? Need continuous measures with equal intervals between scale points EFA is possible with dichotomous variables, but becomes more complicated… Do scores on the measures vary enough? Need sufficient variability to explore correlations and relationships Do scores have linear correlations with each other? Technical point: Determines factorability of the data matrix Magnitudes ideally at least ± 0.30… …but if the correlations are too high, problems of invariance and parsimony Are scores (generally) normally distributed? No outliers: They can strongly distort correlations! Data need to be unskewed for the same reasons 3 Step 2 Decide on the number of factors to retain … if you’re taking a theory-driven approach Step 3 Choosing an Extraction Method 4 Reminder on Extraction Analyzes the pattern of correlations among variables Uses eigenvectors to extract factors (or components) that explain the pattern of shared variance (i.e., the correlation matrix) Computes factor loadings for each variable on each factor Several methods Principal Components Analysis Principal Axis Factoring Maximum Likelihood Methods differ in assumptions about variance in measured variables and specific computations involved Method 1: Principal Components Analysis Communalities are set to 1 for each measured variable Assumed that all variance is shared variance (i.e., no error or unique variance) Because all variance is treated as shared it analyzes both communalities and unique variance (other methods just deal with the former) Extracts components that are assumed to be uncorrelated Pros Finds the best mathematical solution Typically explains more variance than other methods Cons Measurement assumption is inappropriate for psychology… Factor loadings may be artificially high (since solution fitted to measurement error as well as shared variance) 5 Method 2: Principal Axis Factoring Communalities are estimated from empirical correlation matrix So communalities will be < 1 Analyzes only variance shared between measured variables Leaves out error and variance specific to a variable Goal is to maximize the variance in the observed variables that is explained by the extracted factors Pros Measurement assumption is appropriate for psychology! Method 3: Maximum Likelihood Like Principal Axis Factoring, estimates communalities from empirical correlation matrix, analyzes only shared variance, and allows for the possibility of correlated factors Differs from Principal Axis Factoring in that the goal is to maximize the likelihood of reproducing the observed correlations between variables May result in a different solution than just “fitting” the variances Pros Measurement assumption is appropriate for psychology Provides a goodness of fit test (though seldom reported) comparing observed correlation matrix vs. the one produced by the factor solution 6 Choosing an Extraction Method Consideration: a priori ideas vs. pure exploration For a priori ideas, best to use PAF or ML over PCA Consideration: Measurement assumptions If observed variables are free of unique variance, can use PCA …but if you’re in showbiz (or psychology), working with people, children, or animals, use PAF or ML Consideration: Relationship between test constructs If there is reason to believe unobserved constructs are independent, use PCA If constructs could be correlated, use PAF or ML Consideration: So… am I using PAF or ML? Results are usually consistent across methods; follow conventions in subfield Step 4 Choosing a Rotation Method 7 What is Rotation? Disgust scale: Short Form V2 V1 Stepping in dog poo V1 V2 Touching someone’s open wound Assess all this stuff V3 and begin EFA… V3 Seeing a cockroach on the floor V4 A student cheating on a test Factor 1 V5 Someone cutting in line V4 V5 Factor 2 We get two clusters of variables, Coordinates in factor space but interpretation is ambiguous: reflect variable loadings on Each cluster of variables loads extracted factors onto both factors! What is Rotation? Disgust scale: Short Form V2 V1 Stepping in dog poo V1 Factor 1 V2 Touching someone’s open wound V3 V3 Seeing a cockroach on the floor V4 A student cheating on a test Factor 1 V5 Someone cutting in line V4 V5 Factor 2 Factor 2 What we want is a simple factor structure where variables load primarily onto one factor each 8 Methods of Rotation Two classes of methods with different assumptions about how factors might be correlated Orthogonal Rotation Assumes factors are uncorrelated Factors remain orthogonal after rotation Oblique Rotation Assumes factors can be correlated Factors may not be orthogonal after rotation Orthogonal Rotation Methods Independent factor axes V2 V1 Factor 1 Maintains original eigenvectors V3 Angle of rotation is the same for all axes Varimax Rotation Minimize complexity of factors V4 V5 Identifies cluster of variables that defines any one factor Quartimax Rotation Factor 2 Minimize complexity of variables Identifies variables defined by only one factor Equamax Rotation Attempts to minimize complexity of both factors and variables, but results are unstable… Don’t do this. 9 Oblique Rotation Methods V2 Correlated factor axes V1 V3 V2 Different angles of rotation for different V1 axes of the factor solution V3 Factor 1 Performed using two kinds of loadings V4 V5 Pattern Loadings: Indexes unique relation between a factor and a variable, partialling out effects of other factors (like a partial Factor 2 correlation) Structure Loadings: Indexes relation between a factor and a variable without accounting for other factors (like a bivariate correlation) Oblique Rotation Methods Correlated factor axes V2 Different angles of rotation for different V1 axes of the factor solution V3 Factor 1 Oblimin Rotation V4 V5 Minimizes sum of cross-products of pattern loadings to get variables to load on only a single factor Factor 2 Promax Rotation Raises orthogonal loadings to a power to The mathematics become more tedious reduce small loadings, then rotates axes to with oblique rotation, but the guiding accommodate this modified interim solution principles are the same as for orthogonal rotation 10 Which Rotation Method Should I Use? Ultimately depends on theory and measurement assumptions Are constructs of interest likely to be correlated or uncorrelated? Varimax is most common orthogonal method (we care more about having simple factors than simple variables in psychology) Oblimin is most common oblique method, but they all generate similar solutions... Pure exploration is acceptable Contrast solutions generated by orthogonal and oblique rotations Make a judgment about which method produces a simpler solution Goal of EFA is to produce a simple interpretable factor structure No obvious right and wrong here… Now Look at What You’ve Done: Navigating Output 11 What SPSS Provides as Output Initial Factor Solution Communalities Variance explained by each factor / component Information about extracted factors / components Factor loadings Information about rotated factor solution For orthogonal rotation: Factor loadings For oblique rotation: Pattern and Structure loadings Correlations between factors Only if oblique rotation was used What You Need to Do Identify patterns in the rotated factor solution Loadings are calculated for every variable on every factor Look for groups of variables that load strongly on one factor ( > 0.70), but weakly ( < 0.30) on other factors Once variables that load on a factor have been found, consider what it is they have in common—Interpret the content of the variables Identify the construct represented by each factor Based on content of variables and existing theory These are a subjective decisions—justify your interpretations! 12 Interpreting Loadings: Positive vs. Negative Loadings are often positive, but can be negative For the initial factor solution (unrotated), you need not worry about the signs of the factor loadings Can reflect artifacts of the (iterative) EFA algorithm Unrotated solutions are often not clearly interpretable Do consider the signs of the loadings for the rotated solution though If all signs are consistent, it is often easier to interpret the factor Loadings with opposite signs can be expected in the case of reversed items, common in questionnaire scales When Signs Differ + - + - + All these items load strongly on Extraversion, but the signs differ 13 Troubleshooting A variable loads strongly on multiple factors Antithetical to the data-reduction goal of EFA Could reflect a higher-order or more complex factor Could remove this variable to increase interpretability of general solution… …but need to consider the importance of the variable (and potential theoretical relevance of a higher-order factor) Troubleshooting A variable does not load strongly on any factor Variable does not have much to do with constructs of interest Drop the variable and re-run the analysis Unproblematic—this variable does not contribute to the factor structure Factor is uninterpretable, given its constituent variables Uninterpretable factors are not very useful to anybody… Easiest option is to drop these variables and re-run analysis 14 Heywood cases Situation when communality for a variable is estimated to be > 1 or an eigenvalue for a factor is estimated to be negative Named after Heywood (1931) – sometimes numerical values that solve the optimization problem posed by EFA are logically impossible values Communalities > 1 do not make sense (more than all the variance cannot be shared) Negative eigenvalues do not make sense (can’t explain less than none of the variance) Why does this happen? Optimization procedure “solves” the factor analysis problem by availing itself of logically impossible numbers—the math works, but nothing else does… Empirically, Heywood cases often arise when there are too few data points, given the number of factors extracted, or variables are highly correlated Dealing with Heywood cases Drop highly correlated variables Highly correlated variables can make it difficult to identify factor structure Collect more data Can assist in clarifying factor structure Maximum Likelihood methods are more vulnerable Switch to Principal Axis Factoring—rests on similar assumptions, but is less susceptible to returning Heywood cases 15 Some Key Points to Remember EFA can only analyze variables submitted to the analysis! Factors are “discoverable” only if you have data on the relevant variables Factor structure can change with the addition/removal of variables, as this changes the underlying correlational structure of the data Default settings may not provide enough iterations for EFA to converge on a good solution SPSS uses 25 as a default, but something more like 250+ is required in practice Take notes as you go If you do multiple analyses (e.g., different extraction/rotation methods, including/excluding certain variables), make sure you keep track of why you did them Easy to forget the decision-making chain given time lags in research… Step 5 Reporting Results of an EFA 16 What to Report List of variables (or items) submitted to the analysis Choice of extraction and rotation methods Including some justification for these choices Number of factors extracted, and the rule used to determine this e.g., a priori reasons, scree plot, Kaiser’s criterion… Proportion of variance accounted for by each factor What to Report Factor labels and summary of variables for each factor Factor loadings for each factor (rotated and unrotated) List range of loadings in text, but provide a full matrix in a Table Pattern loadings are most important for oblique rotations Correlations between factors (if relevant) 17 What to Report Factor labels and summary of variables for each factor Factor loadings for each factor (rotated and unrotated) List range of loadings in text, but provide a full matrix in a Table Pattern loadings are most important for oblique rotations Correlations between factors (if relevant) Decision Process vs. End Results? Lots of decision points in doing an EFA Polarization among researchers: Report everything or report only end result Err on the side of reporting more detail about process when… EFA result is central to your research question You are using an established measure/set of variables You are investigating theoretically motivated, a priori ideas Basically, there are no hard and fast rules here… Report what you want, but expect to have to justify your decisions, and be prepared to be asked to make changes! 18 Wrapping Up Exploratory Factor Analysis is all about choices Make sure you are making informed, defensible, decisions about extraction methods, stopping rules, and rotation methods There is an art to interpreting EFA solutions What patterns are presented in the factor loadings? What do the factors mean? Are there problems or issues with the rotated solution? Can fall back to purely exploratory analysis If factor structure is difficult to interpret, you can add or remove variables, and tinker But you must be transparent about this if you opt for pure exploration! Other Applications and a Look Ahead Factor analysis is not just used to uncover latent structure of data Can also use factor loadings to develop composite measures Pool information across multiple variables Factor analysis provides in-principle control of measurement error “Purer” measure of construct of interest Next up: Confirmatory Factor Analysis and Structural Equation Modeling Extends principles of EFA 19 CONFIRMATORY FACTOR ANALYSIS & STRUCTURAL EQUATION MODELING Structural Equation Modelling A very broad and powerful class of methods that fits networks of constructs to data Most of the analyses taught in PSYC4050 could be done through SEM Logistic regression (and any regression) Moderation and mediation ANOVA Multilevel modelling Confirmatory Factor Analysis (we will use SEM for this) 1 SEM - Observed and latent variables Observed (or measured, or manifest, or indicator) variables are the data, and are represented by boxes in SEM figures Latent variables (or factors) are hypothesised constructs, and are represented by circles in SEM figures The hypothesised causal relations between observed and/or latent variables are represented by arrows SEM components SEM contains two main components: Measurement model – i.e. the relations between latent variables (factors) and their indicators (measured variables) -> Confirmatory Factor Analysis Structural model – i.e. the hypothesised causal relations between latent variables (factors) -> path analysis 2 Structural model Measurement model Measurement model Purpose of Confirmatory Factor Analysis Identify latent psychological constructs (i.e. factors) that account for correlations among sub-sets of observed variables Determine how strongly each variable is associated with factors Test hypotheses about the factor structure underlying a set of observed variables 3 Confirmatory vs Exploratory Factor Analysis Exploratory Factor Analysis What is the structure of a data set? How many factors (e.g. psychological constructs) do our DVs tap into? More data-driven approach Confirmatory Factor Analysis Test specific hypotheses about the factor structure underlying a data set: Factor loadings, number of factors, associations between factors Theory-driven approach Exploratory Factor Analysis is Data-Driven Exploratory Factor Analysis begins Factor 1 Factor 2 with a bunch of observed variables Energetic.83.07 (i.e. things that we have measured) Outgoing.76.10 Sociable.88.02 Warm.12.83 We then determine factor loadings Trusting.21.76 for each variable on each factor, Sympathetic.08.91 uncovering correlational structure of Extraversion data Agreeableness 4 Exploratory Factor Analysis is Data-Driven Latent Variables (aka Factors) Factor 1 Factor 2 Extraversion Agreeableness Energetic.83.07 (Factor 1) (Factor 2) Outgoing.76.10 Sociable.88.02 Warm.12.83 Factor Loadings Trusting.21.76 Sympathetic.08.91 Energetic Outgoing Sociable Warm Trusting Symp. Observed variables Extraversion Agreeableness e1 e2 e3 e4 e5 e6 Error Terms (unique variance) Exploratory Factor Analysis is Data-Driven Factor 1 Factor 2 Extraversion Agreeableness Energetic.83.07 (Factor 1) (Factor 2) Outgoing.76.10 Sociable.88.02 Warm.12.83 Trusting.21.76 Sympathetic.08.91 Energetic Outgoing Sociable Warm Trusting Symp. Extraversion Agreeableness All factor loadings are accounted for—even if they are weak, and theoretically uninteresting 5 Summary: EFA is Data-Driven EFA provides a complete characterization of correlational structure Based purely on structure that is already present in the data All factor loadings are computed and represented in the model Even if they are very low and theoretically uninteresting… …and we sometimes have a sense of how our variables will load onto factors Including these terms lacks parsimony What if we imposed constraints on how variables load onto factors? Can we provide a good account of data if we ignore small loadings? Confirmatory Factor Analysis is Theory-Driven Extraversion Agreeableness Energetic Confirmatory Factor (Factor 1) (Factor 2) Outgoing Analysis begins with a bunch of Sociable dependent variables Warm (i.e., things that we ??????????????????????????????? Trusting have measured) Sympathetic Energetic Outgoing Sociable Warm Trusting Symp. We then consider how these variables might load onto e1 e2 e3 e4 e5 e6 hypothetical factors—which variables “go together”? 6 Confirmatory Factor Analysis is Theory-Driven Factor 1 Factor 2 Extraversion Agreeableness Energetic.83 0 (Factor 1) (Factor 2) Outgoing.76 0 Sociable.88 0 Warm 0.83 Trusting 0.76 Sympathetic 0.91 Energetic Outgoing Sociable Warm Trusting Symp. We have made assumptions about the We have made assumptions about the number of latent variables (aka factors) way our dependent variables load (and e1 e2 e3 e4 e5 e6 needed to describe the correlational do not load) onto these latent variables structure of the data (i.e., factors) Confirmatory Factor Analysis is Theory-Driven Factor 1 Factor 2 Extraversion Agreeableness Energetic.83.85 0 (Factor 1) (Factor 2) Outgoing.76.78 0 Sociable.88.87 0 Warm 0.83.84 Trusting 0.76.78 Sympathetic 0.91.90 Energetic Outgoing Sociable Warm Trusting Symp. Note factor loadings “not present” in the figure are actually included in the model—however, We then consider how these variables might load onto they are forced take on a value of zero (i.e. no association), consistent with assumptions about hypothetical factors—which variables “go together”? underlying factor structure 7 Interim Summary: CFA is Theory-Driven CFA provides a characterization of correlational structure Constrained by assumptions about how DVs load onto factors Only some factor loadings take on non-zero values in the model Some factor loadings are constrained to equal zero Determined by a priori theoretical considerations The model is more parsimonious than EFA, and also theoretically principled! So how does this work, and why is this good? How Confirmatory Factor Analysis Works How well does the hypothesized factor structure account for data? Energetic Outgoing Sociable Warm Trusting Symp. Energetic Var(E) Outgoing Cov(E,O) Var(O) Sociable Cov(E,So) Cov(O,So) Var(So) Warm Cov(E,W) Cov(O,W) Cov(So,W) Var(W) Trusting Cov(E,T) Cov(O,T) Cov(So,T) Cov(W,T) Var(T) Symp. Cov(E,Sy) Cov(O,Sy) Cov(So,Sy) Cov(W,Sy) Cov(T,Sy) Var(Sy) Some of these covariances are going to be low… If our theory is good, we can predict which ones they are. 8 How Confirmatory Factor Analysis Works How well does the hypothesized factor structure account for data? Variables loading onto different factors are Extraversion Agreeableness (Factor 1) (Factor 2) uncorrelated If we predict non-zero covariances among some variables… Energetic Outgoing Sociable Warm Trusting Symp. …and covariances of zero for others, can we capture the data? How Confirmatory Factor Analysis Works How well does the hypothesized factor structure account for data? E O So W T Sy Ex Ag E V(E) O C(E,O) V(O) E O So W T Sy So C(E,So) C(O,So) V(So) W C(E,W) C(O,W) C(So,W) V(W) T C(E,T) C(O,T) C(So,T) C(W,T) V(T) How accurately can the Sy C(E,Sy) C(O,Sy) C(So,Sy) C(W,Sy) C(T,Sy) V(Sy) model recover the data? 9 How Confirmatory Factor Analysis Works How well does the hypothesized factor structure account for data? E O So W T Sy Ex Ag E V(E) O C(E,O) V(O) E O So W T Sy So C(E,So) C(O,So) V(So) W C(E,W) 0 C(O,W) 0 C(So,W) 0 V(W) T C(E,T) 0 C(O,T) 0 C(So,T) 0 C(W,T) V(T) How accurately can the Sy C(E,Sy) 0 C(O,Sy) 0 C(So,Sy) 0 C(W,Sy) C(T,Sy) V(Sy) model recover the data? Is our description of the data compromised by the simplifying assumptions made by our model? Estimating Model Parameters Ex Ag Pattern of factor loadings generates predictions about the values of items in the predicted variance-covariance matrix E O So W T Sy E O So W T Sy Adjust factor loadings to maximize E ? similarity between values in the O ? ? predicted variance-covariance matrix So ? ? ? and the values in the empirical one W ? 0 ? 0 ? 0 ? Hypothesized factor structure T ? 0 ? 0 ? 0 ? ? constrains values that can be predicted by the model Sy ? 0 ? 0 ? 0 ? ? ? 10 What are we left with? E O So W T Sy E V(E) Can evaluate quality of model O C(E,O) V(O) EMPIRICAL DATA predictions by computing the So C(E,So) C(O,So) V(So) discrepancy between Empirical and W C(E,W) C(O,W) C(So,W) V(W) Predicted data—via χ2 test T C(E,T) C(O,T) C(So,T) C(W,T) V(T) Sy C(E,Sy) C(O,Sy) C(So,Sy) C(W,Sy) C(T,Sy) V(Sy) E O So W T Sy E V’(E) If our model is good—predicted O C’(E,O) V’(O) MODEL PREDICTIONS data will be very similar to the So C’(E,So) C’(O,So) V’(So) empirical data! W 0 0 0 V’(W) T 0 0 0 C’(W,T) V’(T) Sy 0 0 0 C’(W,Sy) C’(T,Sy) V’(Sy) What does that tell us? Consistency of the theory with the data Does hypothesized factor structure ignore anything important (poor fit)? Does hypothesized factor structure closely capture the data (good fit)? Note that quality must be evaluated alongside parsimony—it is not exclusively determined by fit to data! Separates the wheat from the chaff (the essential from the inessential) What factors/loadings are needed to describe the structure of the data? Which aspects of the data can we ignore (without much loss of explanatory power)? 11 Choosing Between EFA and CFA Use EFA to explore a set of variables Newly developed variables to assess a construct Not sure about constructs underlying the variables Use CFA to test a priori hypotheses derived from: Existing theory Previous research using the same variables What About Both? Could use both techniques in a single paper EFA in study one to identify underlying factor structure CFA in study two to test the hypothesized factor structure from study one Can even use both techniques in one study Split the data file—50% of cases in each half Use EFA on one to identify factor structure, use CFA on the other to test …Note that this requires very large Ns You cannot use both methods on the same data though… would be like Hypothesising After the Results are Known (HARKing) 12 Doing Confirmatory Factor Analysis Just Follow These Steps! Step 1: Preliminaries (preparing for the analysis) Step 2: Evaluate model fit Step 3: Evaluate parameter estimates (i.e. pattern of factor loadings and correlations) Step 4: Evaluate alternative models 13 Preliminaries: Checking Assumptions Essentially the same as for Exploratory Factor Analysis Data should be measured on an interval scale Scores should cover a wide range on variable scales Variables should have linear correlations with each other Scores should be (generally) normally distributed Preliminaries: Specify the Model Assumptions are theory-driven How many constructs underlie the observed variables? How are observed variables expected to load onto factors (latent variables)? Are the factors correlated with one another? The CFA model you are testing encapsulates hypotheses about what the most important aspects of the data are What is essential for explaining the data? What can be ignored while explaining the data? 14 Preliminaries: Model Parameters Which parameters are fixed, which parameters are free? Fixed parameters take on predetermined values This model assumes some loadings are fixed to 0 Extrav. Agreeable Also assumes that factors are uncorrelated Ener. Outg. Soc. Wa. Trus. Sym. Parameters fixed to 0 are typically omitted from figures Preliminaries: Model Parameters Which parameters are fixed, which parameters are free? Fixed parameters take on predetermined values Free parameters are estimated from the data Algorithm searches for set of Extrav. Agreeable factor loadings that maximizes correspondence between data and model Ener. Outg. Soc. Wa. Trus. Sym. 15 Note on Free Parameters Free parameters add greater flexibility Allows the model to account for more patterns of data This can be good and bad Good: Can provide a better account of a wider range of data Bad: A model that can account for everything often doesn’t provide much insight into the data (it’s too complex to make sense of) Note on Free Parameters If all parameters are allowed to be free in CFA… Model provides a perfect account of data every time Can’t formally test hypotheses about the model (instead of just exploring as in EFA) I.e. the model is unfalsifiable So to test a hypothesis, at least one parameter must be constrained (e.g. fixed, or equated to another parameter) 16 Preliminaries: Sample Size Considerations Ensure statistical stability of the model Small samples mean model parameters cannot be estimated precisely -> model instability, low power to detect small effects Aim for 10+ participants per estimated parameter Shared and unique variance for DVs, factor loadings, factor correlations, etc. 5 participants per parameter may be OK (reduced statistical power) < 5 participants per parameter makes for poor parameter estimates Evaluating Model Fit: Is the Model Good? Model fit is the degree of similarity between the estimated variance- covariance matrix and the observed variance-covariance matrix If the two are very similar, the model provides a good fit to data Fit is evaluated in several different ways Against a null hypothesis (via χ2 test) In terms of absolute fit In terms of relative fit—often against a baseline “all zero correlations” model