Inferential Statistics Lecture Notes PDF
Document Details
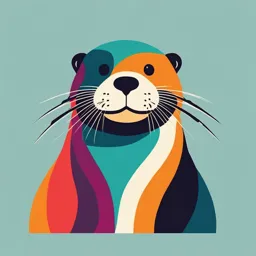
Uploaded by FairRiemann
Mansoura - Manchester
Prof. Eman Omar Khashaba
Tags
Summary
These lecture notes cover inferential statistics, focusing on hypothesis testing and the interpretation of p-values. The document details different types of statistical tests used in medical research.
Full Transcript
Mansoura – Manchester Programme for Medical Education Semester 9 “Inferential statistics” Learning outcomes At the end of this lecture, the student should be able to: ▪ Differentiate between normal and non -...
Mansoura – Manchester Programme for Medical Education Semester 9 “Inferential statistics” Learning outcomes At the end of this lecture, the student should be able to: ▪ Differentiate between normal and non -normally distributed data ▪ Recognize types of research errors. ▪ Select the type of test of significance according to type of data ▪ Recognize principles of test of significance ▪ Interpretation of test results & p value Introduction One of the important function of medical statistics is formulation & testing hypothesis. We often gather sample data to assess how much evidence there is against a specific hypothesis about the population. We use a process known as hypothesis testing (or significance testing) to quantify our belief against a particular hypothesis. Box (1): Hypothesis testing Quoted from Petrie and Sabin(2006) pg. 1 Prof. Eman Omar Khashaba Mansoura – Manchester Programme for Medical Education Semester 9 Steps for hypothesis testing 1. Define the null and alternative hypotheses. 2. Choose the level of significance (0.05 or less). 3. Pick and compute the test statistic (t or chi ect…..). 4. Compute the p-value 5. Check whether to reject the null hypothesis by comparing the p-value to the level of significance. 6. Draw conclusion from the test. The “null hypothesis” The “null hypothesis” is a concept. The test method assumes (hypothesizes) that there is no (null) difference between the groups. The result of the statistical test either supports or rejects that hypothesis. The null hypothesis is generally the opposite of what we are actually interested in finding out. If we are interested if there is a difference between two treatments then the null hypothesis would be that there is no difference and we would try to disprove this. Null hypothesis (Ho); that no differences exist between groups. Alternative hypothesis (H1); there is differences between groups. What is the null hypothesis in a study? (a) To find out whether use of a new surgical technique reduces rates of wound infection. (b) To find out whether adenoidectomy reduces absence from school in children with middle ear disease. Answers (a) Rates of wound infection are the same with the new surgical technique as with old surgical technique. pg. 2 Prof. Eman Omar Khashaba Mansoura – Manchester Programme for Medical Education Semester 9 (b) In children with middle ear disease, rates of absence from school are the same after adenoidectomy as in comparable children who have not had the operation. As a consequence of sampling errors, statistical significance tests sometimes yield erroneous outcomes. Specifically, two errors may occur in hypothesis tests: Alpha error occurs when the null hypothesis is erroneously rejected, and beta error occurs when the null hypothesis is wrongly retained. Types of error in research Type I (α error): the probability of error to reject the null hypothesis when it is actually true. i.e to state that there is difference when the difference occurs by chance (false positive results). Type II (β error): the probability of error to accept the null hypothesis when it is actually false i.e to state that there is no difference when such difference exists (false negative results). pg. 3 Prof. Eman Omar Khashaba Mansoura – Manchester Programme for Medical Education Semester 9 Probability value (p-value) The p-value is the probability of obtaining the effect observed in the study (or one stronger) if the null hypothesis of no effect is actually true. Or The p value gives the probability of any observed difference have happened by chance. It is the cutoff for rejecting null hypothesis is (p=0.05). Interpretation of results: If p value less than 0.05 If p value more than 0.05 Reject null hypothesis Accept null hypothesis Accept alternative hypothesis Reject alternative hypothesis Example. Comparison of effect of treatment of bronchopneumonia with amoxicillin or erythromycin Amoxicillin Erythromycin Total Improvement at 5 days 144 (60%) 160 (67%) 304 (63%) No improvement at 5 days 96 (40%) 80 (33%) 176 (37%) Total 240 (100%) 240 (100%) 480 (100%) The results were χ2 = 2.3; p = 0.13 Test of significance In order to test a statistical hypothesis, tests of significance are used. They are mathematical expression of sample values that provide basis for testing statistical hypotheses. Statistical tests which enable us to decide whether to reject or accept hypotheses. pg. 4 Prof. Eman Omar Khashaba Mansoura – Manchester Programme for Medical Education Semester 9 It varies whether the data are parametric or non- parametric. Parametric data; Data which follow the normal distribution curve simply when the SD is less than one third of the mean i.e. the individuals in the group are homogenous. Non-parametric data; Data which do not follow the normal distribution curve simply when the SD is greater than one third of the mean i.e. there is great variation between the individuals in the group. Table (1): Types of significance tests and their uses in practice Significance test When to use Examples Parametric test t –test (student to compare two means. Mean of hemo- test) globin level between males and females. Paired t-test to compare two mean Comparing mean pre and post of cholesterol intervention. after giving lipid lowering agents. ANOVA To compare mean in Compare the mean of more than two groups. hemoglobin among Saudis, Yemeni and Egyptians. Repeated measure Compare three or more Compare baseline with 3m anova matched groups ,6m ,12 m after ttt) Pearson’s Correlation It indicates the degree Degree of correlation (r) to which two between serum calcium and quantitative variables bone mineral density. are related. Correlation coefficient (r) lies between: –1 to +1 (–1 < r < +1) Strength of correlation: pg. 5 Prof. Eman Omar Khashaba Mansoura – Manchester Programme for Medical Education Semester 9 – Weak positive correlation: 0 < r < 0.3 – Moderate positive correlation: 0.4 < r < 0.6 – Strong positive correlation: r > 0.7 Correlation is represented by: ‘Scatter diagram’ Linear regression Simple linear Predictors of hemoglobin regression: Only one level according to age and dependent variable and PCV (multilinear one independent regression). variable – Multiple linear regression: Only one dependent variable and more than one independent variable Multivariable logistic One dependent -Predictors of scaphoid regression qualitative variable and malunion among group of multiple independent patients factors “qualitative or -predictors of severe quantitative or mixed” occupational injuries such as age-occupation-drug abuse. Non parametric test Mann–Whitney to compare two Compare non normally corresponds to different quantitative distributed CRP in two unpaired t-test. variables with non- different groups (MI and normal distribution healthy group). pg. 6 Prof. Eman Omar Khashaba Mansoura – Manchester Programme for Medical Education Semester 9 Wilcoxon signed rank to compare two A researcher wishes to test”: corresponds to different quantitative explore whether the paired t-test variables with non- participants' pain levels normal distribution changed after they had before and after undergone the acupuncture intervention. Kruskal-Wallis test Compare three or more A researcher compares rate corresponds to One- unmatched or different their back pain on a scale of way ANOVA groups 1 to 10 in three different treatment groups. Friedman tests Compare three or more A research compares pain corresponds to matched groups level after treatment at 1 Repeated-measures week , 4 weeks and 6 weeks ANOVA for same group. Spearman’s rank Test correlation Testing relationship with correlation between 2 non- CRP and serum interleukins coefficient: normally distributed when both assume non corresponds to variables normal distribution. Pearson’s correlation coefficient. pg. 7 Prof. Eman Omar Khashaba Mansoura – Manchester Programme for Medical Education Semester 9 Tests of significance for qualitative data Chi-square test: it measures the association between qualitative variables. It uses absolute values. Fisher exact test: alternative to chi-square test when 50% of cell frequencies are less than 5. The “Mantel Haenszel test” is an extension of the χ2 test that is used to compare several two-way tables. Requirements of Chi-square test (χ2) The data must be in the form of frequencies The frequency data must have a precise numerical value and must be organized into categories or groups. The total number of observations must be greater than 20. The expected frequency in any one cell of the table must be greater than 5. If the number in any two cells or more is less than 5; Fisher exact test is used χ2 is used also for comparison between more than two groups Interpretation of test: Thankfully you do not need to know any mathematical calculation. Look for the p value to see how significant the result is. Remember: The smaller the p value, the smaller the chance that the “null hypothesis” is true. The higher the chance “the alternate hypothesis” is true When p 0.05 accept null hypothesis Best regards pg. 8 Prof. Eman Omar Khashaba