Bioinformatics: Data Analysis in Biology PDF
Document Details
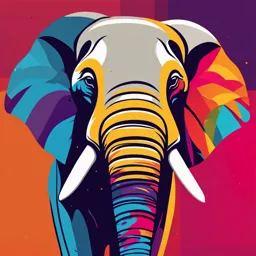
Uploaded by SprightlyHawkSEye
Tags
Summary
This document examines the field of bioinformatics, discussing the massive amounts of biological data generated by recent advancements in high-throughput -omics studies. It emphasizes the importance of combining domain knowledge with statistical and data analysis methods to extract meaningful insights, and highlights the need for innovative strategies to manage this volume of data.
Full Transcript
Originally copied from https://www.engagementaustralia.org.au/blog/blind-men-elephant § Big data in Biology – where is data coming from? § Data generation – end or means? Making sense of data § Four illustrative examples § Concerns A very broad and inclusive definition Ana...
Originally copied from https://www.engagementaustralia.org.au/blog/blind-men-elephant § Big data in Biology – where is data coming from? § Data generation – end or means? Making sense of data § Four illustrative examples § Concerns A very broad and inclusive definition Analyze biological data to extract patterns and generate hypothesis following are the underlying activities: (i) storage, search, and retrieval of data Creation of thematic databases (primary and/or derived data) (ii) integration of diverse types of data A very broad and inclusive definition Analyze biological data to extract patterns and generate hypothesis following are the underlying activities: (i) storage, search, and retrieval of data Creation of thematic databases (primary and/or derived data) (ii) integration of diverse types of data https://www.nature.com/ar https://www.freepik.com/free ticles/s41597-021-01080-w -photos-vectors/sound-audio Image data (static or Audio data video) Elephants have ‘names’ Plants cry when stressed Bird, insect chirps,... Data Text data Numeric data https://www.thieme-connect.de/products/ejournals/abstract/10.1055/s-0043-1774796 http://www.sthda.com/english/articles/24-ggpubr-publication-ready-plots/77-facilitating-exploratory-data-visualization-application-to-tcga-genomic-data/ a consequence of advances in several domains Algorithms Chemistry Computer hardware Cross-disciplinary studies Genetic engineering Instrumentation Microscopy Software Spectroscopy Language English DNA Alphabet 1 1 Letters of the alphabet 26 4 Grammar Exists Exists Meaning (or information) depends upon “Content” the sequence in which letters are used STRESSED ACT JUMBLE DESSERTS CAT BUJELM Order (or sequence) in which the letters A, C, G, and T appear in DNA Figure 16.22 in Campbell Biology, 10th edition 10,000 1,000 100 10 1 0.1 0.01 0.001 2003 2006 2010 2014 2018 2022 Accessed on 16 September 2024 https://www.genome.gov/about-genomics/fact-sheets/DNA-Sequencing-Costs-Data 10,000 1,000 100 10 1 § Moore’s law: compute power doubles once every two years 0.1 Describes long-term trend in hardware technology 0.01 § Why is Moore’s law referenced here? A technology that ‘keeps up’ with this law is deemed to be doing exceedingly well 0.001 2003 2006 2010 2014 2018 2022 Accessed on 16 September 2024 https://www.genome.gov/about-genomics/fact-sheets/DNA-Sequencing-Costs-Data 10,000 § Why did the trend breakaway in 2008 1,000 § Development of “Next Generation Sequencing” (NGS) technologies 100 10 1 0.1 0.01 0.001 2003 2006 2010 2014 2018 2022 Accessed on 16 September 2024 https://www.genome.gov/about-genomics/fact-sheets/DNA-Sequencing-Costs-Data Note: this is as of 2015 Data phase Astronomy Twitter (X) YouTube Genomics Acquisition 0.5-15 billion 500-900 25 zetta bytes 1 zetta bases (per year) tweets million hours 1-17 peta 2-40 exa Storage 1 exa bytes 1-2 exa bytes bytes bytes Metric prefixes kilo- mega- giga- tera- peta- exa- zetta- 10! 10" 10# 10$% 10$& 10$' 10%$ PLoS Biol (2015) 13:e1002195 https://www.genome.gov/human-genome-project https://www.nature.com/articles/nature11119 Genome Then Now USD 2.7 billion USD 1,500 Human 1990-2003 for the first genome Half-a-day Several laboratories Single laboratory Tomato 2007-2012 for the first genome 150 variants in a year Notes available Genomics Proteomics Bioinformatics (2020) 18:5 There are >50 –omes and -omics Only genome is static All other –omes are spatiotemporally dynamic and condition-dependent “low” energy punctate formation “high” energy Notes available punctate: small dots or holes Signal Transduction and Targeted Therapy (2023) 8, Article number 311 Proteome Microbiome Epigenome Representative -omes Glycome Transcriptome Metabolome Lipidome Nat. Immunol. (2015) 16:902 § Data related to ‘biology’ are generated in very large amounts § Consequence of high-throughput –omics studies § Advances in several domains have contributed to this § Data generation has become affordable Drowning in a sea of data and starving for knowledge Sydney Brenner while accepting Nobel prize in Physiology or Medicine in 2002 Significant contributions to work on the genetic code and other areas of molecular biology Established the roundworm Caenorhabditis elegans as a model organism for the investigation of developmental biology https://www.nature.com/articles/d41586-021-02480-z Photographs were copied from the respective Wikipedia pages Biology must generate ideas as well as data Data must be a means to knowledge, not an end in themselves Sir Paul Nurse (Nobel prize in Physiology or Medicine, 2001) Made this observation in a 2021 World View article in Nature Discovered certain protein molecules that control the division of cells in the cell cycle https://www.nature.com/articles/d41586-021-02480-z Photographs were copied from the respective Wikipedia pages Big data by itself and abstract art... Rear view Front view An art installation @ the Bengaluru airport (Terminal 2) This artwork depicts the fundamental aspect of nature - how matter is interconnected and interrelated. The artist, Sri Saravanan Parasuraman, has used the seed germination process to represent this concept How do we make sense of data? What information is hidden? Huge amounts of data Rich in information?! https://medium.com/axioma-ai-journal/the-art-of-biological-data-science-d1444eae360e Biology Statistics Interpretation Data analysis Hypothesis testing Choice of metrics Context for data generation Pattern extraction Validity of inferences Develop algorithms Computer Science Storage + retrieval (query optimization, data transmission, archival, etc.) Domain knowledge Statistics Why, how, and what Data analysis Choice of metrics Pattern extraction Validity of inferences Develop algorithms Computer Science Storage + retrieval (query optimization, data transmission, archival, etc.) § Methods and hardware have evolved to deal with large and diverse data § A ‘traditional’ method might assume normal distribution of data. Develop new methods for data which does not conform to such assumptions Charmander Charmeleon Charizard Not to scale; https://bogleech.com/pokemon/p004 Achieve biological results leveraging domain knowledge of the problem Statistical and other types of data analysis algorithms to identify trends / patterns Technologies to support large-scale data storage, retrieval, and analysis Experiments for collecting data Genome Res. (2015) 25:1417 d g e w l e Achieve biological n o results k leveraging a in o m domain knowledge D of the problem ate) (i te r Statistical and other types d g e of data analysis ow le trends / patterns algorithms to kidentify n n m a i D o Technologies to support large-scale data storage, retrieval, and analysis d ge Experiments for collecting w e l data kno ain D om Genome Res. (2015) 25:1417 Observations + Knowledge Data science Question Statistics AI/ML Validate the Hypothesis hypothesis Perform Testable experiment prediction Design experiment § High-throughput –omics studies are generating large amounts of data § Making sense of data requires Domain knowledge Advanced statistical methods (includes AI and ML) Advances in computer science (storage, search, retrieval, transmission, etc.) Clinician’s observation § Normal pressure hydrocephalus (NPH) is a brain disorder Accumulation of cerebrospinal fluid in ventricles Ventricles: inter-connected cavities that form a network and used for “communication” Accumulation of fluid does not lead to higher pressure because ventricles expand to maintain normal pressure Such an expansion affects brain function The prefix hydro- denotes water Cephalus means “head” in Greek Clin. Neuroradiol. (2021) https://doi.org/10.1007/s00062-020-00993-0 Clinician’s query § 3D Magnetic resonance imaging (MRI) signs of NPH may precede clinical symptoms § Early detection helps in treatment, management, etc. § An experienced medical doctor can diagnose using 3D MRI images § Train an ML algorithm to detect NPH using 3D MRI images Widespread deployment can, to a large extent, mitigate inadequate ‘supply’ of expert doctors Clin. Neuroradiol. (2021) https://doi.org/10.1007/s00062-020-00993-0 Domain knowledge serial sections from caudal to cranial increase of CSF volume in red to yellow decrease of GM volume in dark to light blue Colour bars indicate corresponding z-scores Clin. Neuroradiol. (2021) https://doi.org/10.1007/s00062-020-00993-0 Domain knowledge § Input data: 3D MRI images + ML expert Group 1: affected individuals (positive data) Group 2: not affected individuals (negative data) o Group 2 should match Group 1 in age, gender, etc. § An expert doctor Certifies that individuals are affected / not affected Identifies features of a 3D MRI image that are diagnostic Clin. Neuroradiol. (2021) https://doi.org/10.1007/s00062-020-00993-0 ML expert § Using positive and negative data, “train” an ML algorithm A well-trained algorithm can “classify” an unseen data (new image) Image is from an affected (or not affected) individual § Skill requirement Data acquisition and manipulation (programming) Train an algorithm based on ‘features’ identified by clinician Clin. Neuroradiol. (2021) https://doi.org/10.1007/s00062-020-00993-0 Societal benefit § Assessment: comparison with that of doctors Two senior doctors (26 and 19 years of experience) Two junior doctors (4 and 2 years of experience) § Performance of ML algorithm was as good as that of senior doctors! Clin. Neuroradiol. (2021) https://doi.org/10.1007/s00062-020-00993-0 Domain knowledge § Myoglobin – oxygen storage § Hemoglobin – oxygen transport HbA: adult hemoglobin HbF: fetal hemoglobin § Hemoglobin, a subunit – part of HbA and HbF § Hemoglobin, b subunit – part of HbA § Hemoglobin, g subunit – part of HbF Biologist’s query Protein biochemistry Structural biology § Myoglobin (Mb), Hb a subunit, Hb b subunit, Hb g subunit Physiology Evolution § Fold in the same way, have a heme group, bind oxygen § Functionally different Changes in amino acid sequence → functional differences? 1L2K.pdb Domain knowledge + ML expert Gather sequences of globins (indicated below) from various species Myoglobin Hemoglobin Hemoglobin Hemoglobin sequences a subunit b subunit g subunit sequences sequences sequences Statistics expert Sequence Comparison group(s) Inference group With Hb a, b, and g Storage versus Myoglobin With itself subunits transport Hemoglobin a With Hb b and g Hb subunit With itself subunit subunit composition Hemoglobin b Adult and fetal With itself With Hb g subunit subunit hemoglobin Hemoglobin g With itself subunit Hypothesis generation Protein biochemistry Structural biology Physiology § Which positions in the sequence are Evolution common to myoglobin, and a, b, and g subunits of hemoglobin § Why myoglobin is monomeric... a, b, and g subunits olimerize § Why oxygen saturation curves of HbA (ab)2 and HbF (ag)2 are different 1L2K.pdb Slide #12 in today’s lecture Genesis of the question AATCACAGTTCAACTTGATCCGTTCTAAGTTAG Output AAACATGAAATTCTTCATTGTCTTGGTTGCCGC TTTGGCTTTGGCTGCCCCCGCCATGGGCAAGAC CTTCACACGCTGCTCGTTGGCCCGTGAAATGTA CGCCTTGGGTGTACCCAAATCCGAATTGCCCCA ATGGACCTGTATTGCTGAACACGAATCCTCGTA Identify ‘genes’ Orphan protein MDDQLEMYKECITKQLEEVDMLSSIYC Function ‘Translate’ using ? SPGEMHIFDPGVISDFNEFLQNPTNEN VMMYLKAHLDYSIKLQCGRQNDKIEIR IELPHMYPLLENAIVIVHTPLLTKNKE codon table IYLKKELELYIESMDKTETYIYQVLSW Genesis of the question 𝑆→𝑃 All enzymes Biomedical literature Retrieve sequences database from a database Identification of characteristic pattern Pattern found in the orphan protein? Yes? Then, assign function Domain knowledge 𝑆→𝑃 All enzymes Biomedical literature database To read a few thousand research papers to gather information https://www.biorxiv.org/content/10.1101/2024.07.22.604620v1.full Facilitate hypothesis generation 𝑆→𝑃 Experienced researcher: ∼7 min/paper All enzymes Trained LLM: 0.6 min/paper Biomedical Human gets fatigued literature Time difference increases with no. of papers database Fatigue-induced errors creep in Train a LLM to read through the research papers ChatGPT is a type of LLM https://www.biorxiv.org/content/10.1101/2024.07.22.604620v1.full Genesis: an unmet need § Women undergo exploratory surgery for pelvic mass Pelvic mass: enlargements (growth) in the cervix, ovary, uterus Exploratory surgery: surgery for the purpose of diagnosis § Pelvic mass is cancerous in around 15-20% of cases § Better prognosis if surgery is by an expert when pelvic mass is cancerous Surgery by experts: fewer experts / locations Surgery by general surgeons: relatively more numbers / locations https://edrn.nci.nih.gov/data-and-resources/publications/22543921-1991- differential-diagnosis-of-a-pelvic-mass-improved-algorithms-and-novel-biomarkers/ Domain knowledge § Develop a “test” to diagnose cancerous pelvic mass Sensitivity: no cancerous instance is missed out Specificity: only cancerous instances are diagnosed as cancerous § Approach: identify biomarkers using proteomics studies Choice of specific proteomics technique, number of samples available, quality of samples (mode of collection, number of freeze-thaw cycles, patient history, etc.) https://academic.oup.com/clinchem/article/56/2/327/5622538?login=true Statistics expert § A multi-variate analysis index was developed Serum levels of five proteins (biomarkers discovered by proteomics) Menopausal status of patient (text input: yes, no, transition) § Approved by the Food and Drug Administration (FDA) of the US of America FDA is responsible for protecting the public health by ensuring the safety, efficacy, and security of human and veterinary drugs, biological products, and medical devices https://edrn.nci.nih.gov/data-and-resources/biomarkers/ova1/ https://edrn.nci.nih.gov/data-and-resources/publications/20962299-1805-the-road-from-discovery-to-clinical-diagnostics- lessons-learned-from-the-first-fda-cleared-in-vitro-diagnostic-multivariate-index-assay-of-proteomic-biomarkers/ § Reproducibility of reported results: a very serious concern § Reproducibility Project: Cancer Biology examined this issue 193 experiments from 53 selected papers – insufficient details to attempt reproduction After 8 years and with $1.5 million spent, only 26% of the studies could be attempted experimentally. Of these 26% experiments, o at least 85% of the quantitative effects were smaller than reported o only 46% of binary effects were reproduced. Synthetic Biology (2023) 8: ysad014 Input is garbage § Data is not curated § Irrelevant data § Does not meet algorithm’s assumptions Best algorithm (?) §... Parameters optimal values (?) Compute power – not a limitation Garbage = senseless output GIGO Garbage in Garbage out https://www.westcoastinformatics.com/news/medical-datas-garbage-in-garbage-out-challenge-nhfc8-yhzjl AI / ML algorithm Big data Prediction A black box is used here metaphorically to depict an algorithm In common parlance, a black box is a complex system or device whose internal workings are hidden or not readily understood. WIREs Computational Statistics (2023) 15:e1617 AI / ML algorithm Big data Prediction § Why did the algorithm make this prediction? § What is the underlying biological pattern? WIREs Computational Statistics (2023) 15:e1617 Flexible tether Cow A wooden peg https://commons.wikimedia.org/wiki/File:Tethered_bull_Holstein_Mexico_p2.jpg Flexible tether (rich in Gly and Pro) NH2 COOH Anchoring domain Catalytic domain (transmembrane domain) § Input: a large number of protein sequences share the “tethered cow” architecture § Algorithm: detects a pattern (pattern is in the form of “numbers”) What has the algorithm learned? NH2 COOH § Use this learning to predict if a new protein has this architecture... How trustworthy is this prediction? § Explainable methods: derive post hoc understanding of what a model has learnt § Interpretable models: inherently provide an intelligible definition of their parameters and architecture WIREs Computational Statistics (2023) 15:e1617 § Gut feeling or intuition (day to day parlance) A strong belief about someone or something that cannot completely be explained and does not have to be decided by reasoning https://www.freepik.com/premium-vector/gut-brain-connection-microbiome-concept-enteric-nervous-system-human-body-small-large-intestine-signals-from-brain-digestive-tract-colon-bowel- cerebrum-flat-vector-illustration_18234860.htm#query=gut%20feeling&position=47&from_view=keyword&track=ais_hybrid&uuid=c5f23aa0-7217-4261-bf61-b78ab9ad3da9 § There are several errors in biological databases § Reasons are manifold § Users have to be cautious while deciding to invest resources based on database information https://academic.oup.com/bib/article/23/6/bbac416/6764545 ✓ ✓ ✓ ✓ ✓ ✓ ✓