Intro to Bioinformatics PDF
Document Details
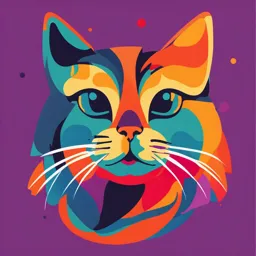
Uploaded by UndisputablePipa
Jashore University of Science and Technology
Tags
Summary
This document introduces bioinformatics as a field that combines biology, computer science, mathematics and engineering to analyze biological data. It details the goals, scope, and application of the discipline in diverse areas including genomics and disease analysis. It further outlines various techniques for sequence analysis and databases.
Full Transcript
Give detailed answer to this questions: 1. Bioinformatics is an interdisciplinary discipline- explain 2. Discuss about the goals and scope of Bioinformatics 3. What are the major areas of computational evolutionary biology and comparative genomics 4. Write about the application of bioinformatics...
Give detailed answer to this questions: 1. Bioinformatics is an interdisciplinary discipline- explain 2. Discuss about the goals and scope of Bioinformatics 3. What are the major areas of computational evolutionary biology and comparative genomics 4. Write about the application of bioinformatics in genetics of disease and analysis of mutation in cancer 5. Discuss about techniques and tools used in analysis of gene and protein expression 6. Define- a. Structural Bioinformatics b. Network and systems biology 7. What do you understand by biological databases? what are the main functions of biological databases 8. Write names of Human genome, Vertebrate and invertebrate databases 9. Write short note on: History of Bioinformatics 10. Write short note on: Genome annotation 11. Write short note on: Application of bioinformatics in current research Bioinformatics is an interdisciplinary field that develops methods and software tools for understanding biological data. As an interdisciplinary field of science, bioinformatics combines Computer Science, Biology, Mathematics, and Engineering to analyze and interpret biological data. Bioinformatics has been used for in silico analyses of biological queries using mathematical and statistical techniques. More broadly, bioinformatics is applied statistics and computing to biological science. Bioinformatics is both an umbrella term for the body of biological studies that use computer programming as part of their methodology, as well as a reference to specific analysis "pipelines" that are repeatedly used, particularly in the field of genomics. Common uses of bioinformatics include the identification of candidate genes and single nucleotide polymorphisms (SNPs). Often, such identification is made with the aim of better understanding the genetic basis of disease, unique adaptations, desirable properties (esp. in agricultural species), or differences between populations. In a less formal way, bioinformatics also tries to understand the organizational principles within nucleic acid and protein sequences, called proteomics. Introduction Bioinformatics has become an important part of many areas of biology. In experimental molecular biology, bioinformatics techniques such as image and signal processing allow extraction of useful results from large amounts of raw data. In the field of genetics and genomics, it aids in sequencing and annotating genomes and their observed mutations. It plays a role in the text mining of biological literature and the development of biological and gene ontologies to organize and query biological data. It also plays a role in the analysis of gene and protein expression and regulation. Bioinformatics tools aid in the comparison of genetic and genomic data and more generally in the understanding of evolutionary aspects of molecular biology. At a more integrative level, it helps analyze and catalogue the biological pathways and networks that are an important part of systems biology. In structural biology, it aids in the simulation and modeling of DNA,RNA, proteins as well as biomolecular interactions. History Historically, the term bioinformatics did not mean what it means today. Paulien Hogeweg and Ben Hesper coined it in 1970 to refer to the study of information processes in biotic systems. This definition placed bioinformatics as a field parallel to biophysics (the study of physical processes in biological systems) or biochemistry (the study of chemical processes in biological Sequences Sequences of genetic material are frequently used in bioinformatics and are easier to manage using computers than manually. Computers became essential in molecular biology when protein sequences became available after Frederick Sanger determined the sequence of insulin in the early 1950s. Comparing multiple sequences manually turned out to be impractical. A pioneer in the field was Margaret Oakley Dayhoff, who has been hailed by David Lipman, director of the National Center for Biotechnology Information, as the "mother and father of bioinformatics." Dayhoff compiled one of the first protein sequence databases, initially published as books and pioneered methods of sequence alignment and molecular evolution. Another early contributor to bioinformatics was Elvin A. Kabat, who pioneered biological sequence analysis in 1970 with his comprehensive volumes of antibody sequences released with Tai Te Wu between 1980 and 1991. Goals/scope To study how normal cellular activities are altered in different disease states, the biological data must be combined to form a comprehensive picture of these activities. Therefore, the field of bioinformatics has evolved such that the most pressing task now involves the analysis and interpretation of various types of data. This includes nucleotide and amino acid sequences, protein domains, and protein structures. The actual process of analyzing and interpreting data is referred to as computational biology. Important sub-disciplines within bioinformatics and computational biology include: Development and implementation of computer programs that enable efficient access to, use and management of, various types of information Development of new algorithms (mathematical formulas) and statistical measures that assess relationships among members of large data sets. For example, there are methods to locate a gene within a sequence, to predict protein structure and/or function, and to cluster protein sequences into families of related sequences. The primary goal of bioinformatics is to increase the understanding of biological processes. What sets it apart from other approaches, however, is its focus on developing and applying computationally intensive techniques to achieve this goal. Examples include: pattern recognition, data mining, machine learning algorithms, and visualization. Major research efforts in the field include sequence alignment, gene finding, genome assembly, drug design, drug discovery, protein structure alignment, protein structure prediction, prediction of gene expression and protein—protein interactions, genome-wide association studies, the modeling of evolution and cell division/mitosis. Bioinformatics now entails the creation and advancement of databases, algorithms, computational and statistical techniques, and theory to solve formal and practical problems arising from the management and analysis of biological data. Over the past few decades, rapid developments in genomic and other molecular research technologies and developments in information technologies have combined to produce a tremendous amount of information related to molecular biology. Bioinformatics is the name given to these mathematical and computing approaches used to glean understanding of biological processes. Common activities in bioinformatics include mapping and analyzing DNA and protein sequences, aligning DNA and protein sequences to compare them, and creating and viewing 3-D models of protein structures. Relation to other fields Bioinformatics is a science field that is similar to but distinct from biological computation, while it is often considered synonymous to computational biology. Biological computation uses bioengineering and biology to build biological computers, whereas bioinformatics uses computation to better understand biology. Bioinformatics and computational biology involve the analysis of biological data, particularly DNA, RNA, and protein sequences. The field of bioinformatics experienced explosive growth starting in the mid-1990s, driven largely by the Human Genome Project and by rapid advances in DNA sequencing technology. Analyzing biological data to produce meaningful information involves writing and running software programs that use algorithms from graph theory, artificial intelligence, soft computing, data mining, image processing, and computer simulation. The algorithms in turn depend on theoretical foundations such as discrete mathematics, control theory, system theory, information theory, and statistics. Sequence analysis Since the Phage φ-X174 was sequenced in the DNA sequences of thousands of organisms have been decoded and stored in databases. This sequence information is analyzed to determine genes that encode proteins, RNA genes, regulatory sequences, structural motifs, and repetitive sequences. A comparison of genes within a species or between different species can show similarities between protein functions, or relations between species (the use of molecular systematics to construct phylogenetic trees). With the growing amount of data, it long ago became impractical to analyze DNA sequences manually. Today, computer programs such as BLAST are used daily to search sequences from more than 260 000 organisms, containing over 190 billion nucleotides. These programs can compensate for mutations (exchanged, deleted or inserted bases) in the DNA sequence, to identify sequences that are related, but not identical. A variant of this sequence alignment is used in the sequencing process itself. DNA sequencing Before sequences can be analyzed they have to be obtained. DNA sequencing is still a non-trivial problem as the raw data may be noisy or afflicted by weak signals. Algorithms have been developed for base calling for the various experimental approaches to DNA sequencing. Sequence assembly Most DNA sequencing techniques produce short fragments of sequence that need to be assembled to obtain complete gene or genome sequences. The so-called shotgun sequencing technique (which was used, for example, by The Institute for Genomic Research (TIGR) to sequence the first bacterial genome, Haemophilus influenzae generates the sequences of many thousands of small DNA fragments (ranging from 35 to 900 nucleotides long, depending on the sequencing technology). The ends of these fragments overlap and, when aligned properly by a genome assembly program, can be used to reconstruct the complete genome. Shotgun sequencing yields sequence data quickly, but the task of assembling the fragments can be quite complicated for larger genomes. For a genome as large as the human genome, it may take many days of CPU time on large-memory, multiprocessor computers to assemble the fragments, and the resulting assembly usually contains numerous gaps that must be filled in later. Shotgun sequencing is the method of choice for virtually all genomes sequenced today, and genome assembly algorithms are a critical area of bioinformatics research. Genome annotation In the context of genomics, annotation is the process of marking the genes and other biological features in a DNA sequence. This process needs to be automated because most genomes are too large to annotate by hand, not to mention the desire to annotate as many genomes as possible, as the rate of sequencing has ceased to pose a bottleneck. Annotation is made possible by the fact that genes have recognisable start and stop regions, although the exact sequence found in these regions can vary between genes. The first description of a comprehensive genome annotation system was published in 1995 by the team at The Institute for Genomic Research that performed the first complete sequencing and analysis of the genome of a free-living organism, the bacterium Haemophilus influenzae. Owen White designed and built a software system to identify the genes encoding all proteins, transfer RNAs, ribosomal RNAs (and other sites) and to make initial functional assignments. Most current genome annotation systems work similarly, but the programs available for analysis of genomic DNA, such as the GeneMark program trained and used to find protein-coding genes in Haemophilus influenzae, are constantly changing and improving. Following the goals that the Human Genome Project left to achieve after its closure in 2003, a new project developed by the National Human Genome Research Institute in the U.S appeared. The socalled ENCODE project is a collaborative data collection of the functional elements of the human genome that uses next-generation DNA-sequencing technologies and genomic tiling arrays, technologies able to automatically generate large amounts of data at a dramatically reduced per-base cost but with the same accuracy (base call error) and fidelity (assembly error). Computational evolutionary biology Evolutionary biology is the study of the origin and descent of species, as well as their change over time. Informatics has assisted evolutionary biologists by enabling researchers to: trace the evolution of a large number of organisms by measuring changes in their DNA, rather than through physical taxonomy or physiological observations alone, more recently, compare entire genomes, which permits the study of more complex evolutionary events, such as gene duplication, horizontal gene transfer, and the prediction of factors important in bacterial speciation, build complex computational population genetics models to predict the outcome of the system over time track and share information on an increasingly large number of species and organisms Future work endeavours to reconstruct the now more complex tree of life. The area of research within computer science that uses genetic algorithms is sometimes confused with computational evolutionary biology, but the two areas are not necessarily related. Comparative genomics The core of comparative genome analysis is the establishment of the correspondence between genes (orthology analysis) or other genomic features in different organisms. It is these intergenomic maps that make it possible to trace the evolutionary processes responsible for the divergence of two genomes. A multitude of evolutionary events acting at various organizational levels shape genome evolution. At the lowest level, point mutations affect individual nucleotides. At a higher level, large chromosomal segments undergo duplication, lateral transfer, inversion, transposition, deletion and insertion.þl] Ultimately, whole genomes are involved in processes of hybridization, polyploidization and endosymbiosis, often leading to rapid speciation. The complexity of genome evolution poses many exciting challenges to developers of mathematical models and algorithms, who have recourse to a spectrum of algorithmic, statistical and mathematical techniques, ranging from exact, heuristics, fixed parameter and approximation algorithms for problems based on parsimony models to Markov chain Monte Carlo algorithms for Bayesian analysis of problems based on probabilistic models. Many of these studies are based on the homology detection and protein families computation. Pan genomics Pan genomics is a concept introduced in 2005 by Tettelin and Medini which eventually took root in bioinformatics. Pan genome is the complete gene repertoire of a particular taxonomic group: although initially applied to closely related strains of a species, it can be applied to a larger context like genus, phylum etc. It is divided in two parts- The Core genome: Set of genes common to all the genomes under study (These are often housekeeping genes vital for survival) and The Dispensable/Flexible Genome: Set of genes not present in all but one or some genomes under study. A bioinformatics tool BPGA can be used to characterize the Pan Genome of bacterial species. Genetics of disease With the advent of next-generation sequencing we are obtaining enough sequence data to map the genes of complex diseases such as diabetes, infertility, breast cancer or Alzheimer's Disease. Genome-wide association studies are a useful approach to pinpoint the mutations responsible for such complex diseases.þ8] Through these studies, thousands of DNA variants have been identified that are associated with similar diseases and traits. Furthermore, the possibility for genes to be used at prognosis, diagnosis or treatment is one of the most essential applications. Many studies are discussing both the promising ways to choose the genes to be used and the problems and pitfalls of using genes to predict disease presence or prognosis. Analysis of mutations in cancer In cancer, the genomes of affected cells are rearranged in complex or even unpredictable ways. Massive sequencing efforts are used to identify previously unknown point mutations in a variety of genes in cancer. Bioinformaticians continue to produce specialized automated systems to manage the sheer volume of sequence data produced, and they create new algorithms and software to compare the sequencing results to the growing collection of human genome sequences and germline polymorphisms. New physical detection technologies are employed, such as oligonucleotide microarrays to identify chromosomal gains and losses (called comparative genomic hybridization), and single-nucleotide polymorphism arrays to detect known point mutations. These detection methods simultaneously measure several hundred thousand sites throughout the genome, and when used in high-throughput to measure thousands of samples, generate terabytes of data per experiment. Again the massive amounts and new types of data generate new opportunities for bioinformaticians. The data is often found to contain considerable variability, or noise, and thus Hidden Markov model and change-point analysis methods are being developed to infer real copy number changes. Two important principles can be used in the analysis of cancer genomes bioinformatically pertaining to the identification of mutations in the exome. First, cancer is a disease of accumulated somatic mutations in genes. Second cancer contains driver mutations which need to be distinguished from passengers. With the breakthroughs that this next-generation sequencing technology is providing to the field of Bioinformatics, cancer genomics could drastically change. These new methods and software allow bioinformaticians to sequence many cancer genomes quickly and affordably. This could create a more flexible process for classifying types of cancer by analysis of cancer driven mutations in the genome. Furthermore, tracking of patients while the disease progresses may be possible in the future with the sequence of cancer samples. Another type of data that requires novel informatics development is the analysis of lesions found to be recurrent among many tumors. Gene and protein expression Analysis of gene expression The expression of many genes can be determined by measuring mRNA levels with multiple techniques including microarrays, expressed cDNA sequence tag (EST) sequencing, serial analysis of gene expression (SAGE) tag sequencing, massively parallel signature sequencing (MPSS), RNA-Seq, also known as "Whole Transcriptome Shotgun Sequencing" (WTSS), or various applications of multiplexed in-situ hybridization. All of these techniques are extremely noise-prone and/or subject to bias in the biological measurement, and a major research area in computational biology involves developing statistical tools to separate signal from noise in high-throughput gene expression studies. Such studies are often used to determine the genes implicated in a disorder: one might compare microarray data from cancerous epithelial cells to data from non-cancerous cells to determine the transcripts that are up- regulated and down-regulated in a particular population of cancer cells. Analysis of protein expression Protein microarrays and high throughput (HT) mass spectrometry (MS) can provide a snapshot of the proteins present in a biological sample. Bioinformatics is very much involved in making sense of protein microarray and HT MS data; the former approach faces similar problems as with microarrays targeted at mRNA, the latter involves the problem of matching large amounts of mass data against predicted masses from protein sequence databases, and the complicated statistical analysis of samples where multiple, but incomplete peptides from each protein are detected. Cellular protein localization in a tissue context can be achieved through affinity proteomics displayed as spatial data based on immunohistochemistry and tissue microarrays. Analysis of regulation Regulation is the complex orchestration of events by which a signal, potentially an extracellular signal such as a hormone, eventually leads to an increase or decrease in the activity of one or more proteins. Bioinformatics techniques have been applied to explore various steps in this process. For example, gene expression can be regulated by nearby elements in the genome. Promoter analysis involves the identification and study of sequence motifs in the DNA surrounding the coding region of a gene. These motifs influence the extent to which that region is transcribed into mRNA. Enhancer elements far away from the promoter can also regulate gene expression, through three-dimensional looping interactions. These interactions can be determined by bioinformatic analysis of chromosome conformation capture experiments. Expression data can be used to infer gene regulation: one might compare microarray data from a wide variety of states of an organism to form hypotheses about the genes involved in each state. In a singlecell organism, one might compare stages of the cell cycle, along with various stress conditions (heat shock, starvation, etc.). One can then apply clustering algorithms to that expression data to determine which genes are co-expressed. For example, the upstream regions (promoters) of co-expressed genes can be searched for over-represented regulatory elements. Examples of clustering algorithms applied in gene clustering are k-means clustering, self-organizing maps (SOMs), hierarchical clustering, and consensus clustering methods. Analysis of cellular organization Several approaches have been developed to analyze the location of organelles, genes, proteins, and other components within cells. This is relevant as the location of these components affects the events within a cell and thus helps us to predict the behavior of biological systems. A gene ontology category, cellular compartment, has been devised to capture subcellular localization in many biological databases. Microscopy and image analysis Microscopic pictures allow us to locate both organelles as well as molecules. It may also help us to distinguish between normal and abnormal cells, e.g. in cancer. Protein localization The localization of proteins helps us to evaluate the role of a protein. For instance, if a protein is found in the nucleus it may be involved in gene regulation or splicing. By contrast, if a protein is found in mitochondria, it may be involved in respiration or other metabolic processes. Protein localization is thus an important component of protein function prediction. There are well developed protein subcellular localization prediction resources available, including protein subcellualr location databases, and prediction Nuclear organization of chromatin Data from high-throughput chromosome conformation capture experiments, such as Hi-C (experiment) and ChlA-PET, can provide information on the spatial proximity of DNA loci. Analysis of these experiments can determine the three-dimensional structure and nuclear organization of chromatin. Bioinformatic challenges in this field include partitioning the genome into domains, such as Topologically Associating Domains (TADs), that are organised together in three-dimensional space. Structural Bioinformatics Protein structure prediction is another important application of bioinformatics. The amino acid sequence of a protein, the so-called primary structure, can be easily determined from the sequence on the gene that codes for it. In the vast majority of cases, this primary structure uniquely determines a structure in its native environment. (Of course, there are exceptions, such as the bovine spongiform encephalopathy — a.k.a. Mad Cow Disease — prion.) Knowledge of this structure is vital in understanding the function of the protein. Structural information is usually classified as one of secondary, tertiary and quaternary structure. A viable general solution to such predictions remains an open problem. Most efforts have so far been directed towards heuristics that work most of the time.[citation needed] One of the key ideas in bioinformatics is the notion of homology. In the genomic branch of bioinformatics, homology is used to predict the function of a gene: if the sequence of gene A, whose function is known, is homologous to the sequence of gene B, whose function is unknown, one could infer that B may share A's function. In the structural branch of bioinformatics, homology is used to determine which parts of a protein are important in structure formation and interaction with other proteins. In a technique called homology modeling, this information is used to predict the structure of a protein once the structure of a homologous protein is known. This currently remains the only way to predict protein structures reliably. One example of this is the similar protein homology between hemoglobin in humans and the hemoglobin in legumes (leghemoglobin). Both serve the same purpose of transporting oxygen in the organism. Though both of these proteins have completely different amino acid sequences, their protein structures are virtually identical, which reflects their near identical purposes. Other techniques for predicting protein structure include protein threading and de novo (from scratch) physics-based modeling. Network and systems biology Network analysis seeks to understand the relationships within biological networks such as metabolic or protein—protein interaction networks. Although biological networks can be constructed from a single type of molecule or entity (such as genes), network biology often attempts to integrate many different data types, such as proteins, small molecules, gene expression data, and others, which are all connected physically, functionally, or both. Systems biology involves the use of computer simulations of cellular subsystems (such as the networks of metabolites and enzymes that comprise metabolism, signal transduction pathways and gene regulatory networks) to both analyze and visualize the complex connections of these cellular processes. Artificial life or virtual evolution attempts to understand evolutionary processes via the computer simulation of simple (artificial) life forms. Molecular interaction networks Interactions between proteins are frequently visualized and analyzed using networks. This network is made up of protein—protein interactions from Treponema pallidum, the causative agent of syphilis and other diseases. Tens of thousands of three-dimensional protein structures have been determined by X-ray crystallography and protein nuclear magnetic resonance spectroscopy (protein NMR) and a central question in structural bioinformatics is whether it is practical to predict possible protein—protein interactions only based on these 3D shapes, without performing protein—protein interaction experiments. A variety of methods have been developed to tackle the protein—protein docking problem, though it seems that there is still much work to be done in this field. Other interactions encountered in the field include Protein-ligand (including drug) and protein— peptide. Molecular dynamic simulation of movement of atoms about rotatable bonds is the fundamental principle behind computational algorithms, termed docking algorithms, for studying molecular interactions. Sequence development We start with a very basic review of biology, necessary for any further work, but largely sufficient for getting started in computational biology. One can (and must) learn more "on the job" Biomolecules are sequences of monomers (DNA, RNA=nucleotide sequences, proteins=amino acid sequences). DNA is the molecule that contains the entire blueprint for an organism. It contains genes that encode the sequences for every protein in the organism, as well as non-coding regions that, among other things, contain regulatory mechanisms for when and in what order different genes get turned on, and may have other functions as well. Most genes code for proteins; some genes code for RNA molecules that play various roles in the cell. Both DNA and RNA are polymers of "nucleotides" which are bases of four kinds [adenine=A, cytosine=C, guanine=G, thymine-T (DNA only), uracil=U (RNA only)] attached to sugar-phosphate backbones. Apart from the one difference in bases, RNA and DNA are very similar except that DNA usually exists in double-stranded "base-paired" form and RNA is in single-stranded form. The backbone of DNA (or RNA) is not symmetrical: each monomer has a 5'-phosphate group at one end and a 3'-hydroxyl group at the other. Each strand is usually read from the 5' to the 3' end. The two strands go in opposite directions. The nucleic acids are base-paired A to T, G to C. A-T bonds are weaker (double-bonds), G-C bonds are stronger (triple-bonds).Proteins are the "building blocks" of life, responsible for a vast number of cellular processes.They regulate genes, catalyse various biochemical reactions, form machinery for synthesis of othermolecules (including other proteins) and are important parts of organelles and tissues. They arepolymers of amino acids (carboxylic acids with an amide group and a side chain). There are twentynaturally occurring amino acids, differing in their side chains.Proteins tend to "fold" into complex three-dimensional conformations; usually the fold is unique and misfolding is rare. The details of the fold are biochemically important. Usually a few active "domains" (for example, binding to DNA, interaction with other proteins) help the protein play important roles in gene regulation, catalysis, etc; these domains tend to be well conserved across species, while the rest of the protein sequence can mutate a lot. Much computational effort goes into studying protein structure and function, but we will not discuss this vast subject here. Genes that code for proteins are first "transcribed" to "messenger RNA" (mRNA) molecules, and then the RNA is "translated" to proteins. Each "codon" of three nucleotides corresponds to a unique amino acid. Since there are 4 nucleotides, there are 64 possible codons; three of these are "stop codons" (TAA, TAG, TGA) (sometimes called "nonsense codons") and don't code for amino acids, instead indicating a stop to transcription. The remaining 61 code for 20 amino acids. Several codons (up to six) thus can code for the same amino acid. The "start codon" is ATG, which codes for the amino acid methionine. What are the biological problems? There are of course a huge number of problems in biology that can benefit from a quantitative treatment, ranging from single molecule behaviour to population biology and ecology. From the title, we are already restricting ourselves to bioinformatics, but we will mainly focus on DNA sequence analysis, with only occasional mention of proteins. The following are a few issues of interest to biologists (and often of medical importance) that could benefit from analysis of DNA sequence: Cellular processes: how the cell carries out its normal tasks; how it responds to external events like heat shock and starvation; how it carries out complex cascades of events such as the process of cell division (mitosis). Development: How a complex organism (eg a worm, a fly, a human) develops from a single fertilised egg. As this embryonic cell divides, the daughter cells also slowly differentiate into functions. This happens as a result of "gradients" of various factors (some of them maternal) that change gene regulation in different parts of the embryo and ultimately cause different cells to develop in highly specialised ways. Evolution: How different species evolve, how new functionality develops. All cellular and developmental processes are controlled by genes that get turned on in response to some external condition (stress, starvation, embryonic gradients) or cyclically (cell cycle). Computational study of how these genes are regulated and how they function is very useful. This is done by analysing the gene sequence and regulatory DNA sequence of the organism itself, and by comparison of this sequence with already-annotated sequence from other organisms. Highly similar (homologous) genes exist among widely different organisms; such genes are called "orthologues". Many subsystems in widely different organisms are very similar and are regulated by orthologous proteins; some proteins exist largely unchanged from primitive archaebacteria all the way to humans. Moreover, many genes with heavy sequence identity often exist in the same organism, arising from ancestral "gene duplication" events; their function is often slightly differentiated, and in fact this is a major driving factor in evolution. There are now "high-throughput" microarray experiments that can essentially give the response of every gene in the genome; analysing, clustering and interpreting this data, and combining it with other computational tasks in gene regulation, is of great interest. Finally, the study of phylogeny (evolutionary history of organisms) and the classification (taxonomy) of organisms has been revolutionised by DNA sequencing. Aims and tasks of Bioinformatics The aims of bioinformatics are threefold. First, at its simplest bioinformatics organizes data in a way that allows researchers to access existing information and to submit new entries as they are produced, eg the Protein Data Bank for 3D macromolecular structures. While data- curation is an essential task, the information stored in these databases is essentially useless until analyzed. Thus the purpose of bioinformatics extends much further. The second aim is to develop tools and resources that aid in the analysis of data. For example, having sequenced a particular protein, it is of interest to compare it with previously characterized sequences. This needs more than just a simple text-based search and programs such as FASTA and PSI-BLAST must consider what comprises a biologically significant match. Development of such resources dictates expertise in computational theory as well as a thorough understanding of biology. The third aim is to use these tools to analyze the data and interpret the results in a biologically meaningful manner. Traditionally, biological studies examined individual systems in detail, and frequently compared those with a few that are related. In bioinformatics, we can now conduct global analyses of all the available data with the aim of uncovering common principles that apply across many systems and highlight novel features. Application of Bioinformatics in current research Currently almost every field of biological research has accepted this biological research weapon and following it, whether it is molecular biology or genetics, or even agriculture. There a complete new emerging field of genome informatics is there which is completely based on bioinformatics tools. Apart from these there are so many areas where bioinformatics is readily being accepted with primary role in prediction of structure similarity and functional similarity in novel drug molecule research also. They perform initially tasks such as Submitting DNA Sequences to the Databases This is one of important thing in biological research, where scientists sequence DNA, and RNA, but until it is not getting deposited to any public sequence database, that cannot be beneficial for scientific community. It became very essential to submit all the sequenced data to public sequence repositories. 13 Lecture notes in Bioinformatics Some of the important public repositories are DDBJ, EMBL, and Genebank. These sequence data can be submitted to repositories in two ways, either by email submission or by online submission through sequence submission tools. There are specific tools for every public sequence repository (Table 1). Table 1: Public sequence depositories SEQUENCE DATABASE DDBJ EMBL GENEBANK Table 1: Public sequence depositorieS Table 2: Human Genome Databases, Browsers and Variation Resources dbVar Database of Genomic Structural Variation ENCODE Project ENCyclopedia Of DNA Elements Ensembl Human Human genes generated automatically by the Ensembl gene builder Entrez Gene Searchable database of genes, defined by sequence and/or located in the NCBI Map Viewer Genome Putting sequences into a chromosome context Consortium GWAS Central centralized compilation of summary level findings from genetic association studies HapMap international lnvitational Database an integrated database of human genes and HapMap HapMap Project transcripts Human Genome A global analysis of human segmental duplications Segmental Duplication Database Human Structural Genome browser Variation Database 1000 Genomes A Deep Catalog of Human Genetic Variation UCSC Human Genome Genome browser Browser Gateway VEGA Human Manual annotation of finished genome sequence Some of other vertebrate databases are listed below in Table 2. Table 2: Human Genome Databases, Browsers and Variation Resources Table 2: Vertebrate databases and genome browsers Database Description ZFIN Zebrafish Information Network Xenbase A Xenopus web resource VEGA Vertebrate Genome Annotation containing manual annotation of vertebrate finished genome sequence UCSC Genome Bioinformatics Genome Browser Tetraodon Genome Browser RGD Rat Genome Database Rabbit Genome Resources Rabbit Genome Browser Pig Genome Resources Pig Genome Browser lizardbase A centralized and consolidated informatics resource for lizard research MGI Mouse Genome Informatics Fugu The Fugu genomics project Ensembl Genome databases for vertebrates and other eukaryotic species Bovmap mapping the Bovine genome AgBase A curated, open-source resource for functional analysis of agricultural plant and animal gene products BirdBase A Database of Avian Genes and Genomes ARKdb Species databases includes: Cat, Chicken, Cow, Deer, Horse, Pig, Salmon, Sheep, Tilapia, Turkey AnolisGenome A community resource site for Anolis genomics and genetic studies These are List of some invertebrate databases and genome browsers available currently (Table 3). Table 2a: Vertebrate databases and genome browsers 15 Lecture notes in Bioinformatics Table 3: List of some invertebrate databases and genome browsers Worm Base The biology and genome of C. elegans VectorBase Invertebrate vectors of human pathogens TAIR The Arabidopsis Information Resource Stella Base Nematostella vectensis Genomics Database SpBase Strongylocentrotus purpuratus Sea Urchin Genome Database SGD Saccharomyces Genome Database Porn Base A scientific resource for fission yeast IGGI International Glossina Genome Initiative HGD Hymenoptera Genome Database Gramene A resource for comparative grass genomics GOBASE The Organelle Genome Database GenProtEC E.Coli genome and proteome database FlyBase A database of the Drosophila genome Ensembl Genomes EcoGene The Database of Escherichia coli Sequence and Function dictyBase Central resource for Dictyostelid genomics Dendrome A Forest Tree Genome Database Daphnia Genome Database Genome browser ChlamydDB Tthe green alga Chlamydomonas reinhardtii and related species ANISEED Ascidian Network for lnSitu Expression and Embryological Data AspGDA spergillus Genome Database BeetleBase The model organism database for Tribolium castaneum Caenorhabditis Genome Genome browser Sequencing Projects The Cotton Genome Genome browser Database Cacao Genome Database Genome browser Candida Genome Database Genome browser Table 3: List of some invertebrate databases and genome browsers After submission every database provides an unique accession number to submitted sequence after verification and duplication checks. If it is an unique sequence, then accession number is given as a single letter followed by 5 digit number, but recently due to huge number of submission two letters followed by 6 digit of number for accession number is now proposed. Genomic Mapping and Mapping Databases Gene mapping is one of the technique to estimate accurate position of gene and corresponding distance between related genes of similar type. After complete evaluation we can reach to a conclusion of genome map for complete genome for that particular organism. Information Retrieval From Biological Database Developing biological database and its availability online was one of the primary concerns at initial stage of biological research, but now as we have many biological database and data is in form of text, table and pictures and many other formats. We should really know that how to retrieve exact data from a suitable database. Database may be of text retrieval, sequence retrieval or it may also include structural data retrieval importance. Sequence Alignment and Database Searching Alignment of sequence with compare to other relevant and similar sequence is very much needed in biological research to understand relation between two sequences and also to predict structure and function based on sequence similarity. For basic alignment of sequences use of BLAST is very common. Based on number of sequences involved in sequencing, we can classify these alignments into pairwise alignment or multiple sequence alignment. Predictive Methods Using DNA Sequences Gene-finding strategies can be Classified into three major categories. Content-based methods rely on the overall, bulk properties of a sequence in making determination. Characteristics considered here include how often particular codons are used, the periodicity of repeats, and the compositional complexity of the sequence. Because different organisms use synonymous codons with different frequency, such clues can provide insight into determining regions that are more likely to be exons. In site-based methods, the focus turns to the presence or absence of a specific sequence, pattern, or consensus. These methods are used to detect features such as donor and acceptor splice sites, binding sites for transcription factors, polyA tracts, and start and stop codons. comparative methods make determinations based on sequence homology. Here, translated sequences are subjected to database searches against protein sequences to determine whether a previously characterized coding region corresponds to a region in the query sequence. Although this is conceptually the most straightforward of the methods, it is restrictive because most newly discovered genes do not have gene products that match anything in the protein databases. Tools associated with these are Grail, Genscan, Fgenes, procrustes and many others developed with bioinformatics. Predictive Methods Using Protein Sequences o There are tools based on predictive methods using protein sequences, such as PSI-Pred, NRpred, PSEAPred. There are other methods also based on motif level, residue level, signal level, peptide level, domain level and profile based]. Sequences Assembly and Finishing Methods At present,the sequencing process is often talked of as consisting of two parts, namely, assembly and finishing, but in practice there is considerable overlap between the two. Assembly is the process of attempting to order and align the readings, and finishing is the task of checking and editing the assembled data. This includes performing new sequencing experiments to fill gaps or to cover segments where the data is poor and adjudicating between conflicting readings when editing. Phylogenetic Analysis o Phylogenetic analysis is also one of the important implementation of bioinformatics in biological research. Phylogenetic analysis is study of ancestral history of an organism. Here after sequence and structural similarities we try to relate organism's ancestral history to show how origin of organism was related to each other and "what was order of evolution". We actually do evolutionary history analysis by phylogenetic analysis. There are many tools available online as well as commercial packages also like PHYLIP. It uses tree generation methods with algorithms based on methods such as UPGMA, and neighbor joining. Comparative Genome Analysis o Comparative genome analysis is also being performed in various researches at many levels such as academics and professional researches. By comparing the finished reference sequence of the human genome with genomes of other organisms, researchers can identify regions of similarity and difference. This information can help scientists better understand the structure and function of human genes and thereby develop new strategies to combat human disease. Comparative genomics also provides a powerful tool for studying evolutionary changes among organisms, helping to identify genes that are conserved among species, as well as genes that give each organism its unique characteristics. Large-Scale Genome Analysis Large scale genome analysis is complete genome sequencing, and this application has much advancement as next generation sequencing and bioinformatics tools like illumina have been developed to analyze them very quickly. These tools are generally termed as sequencer and playing a vital role in modern biological research. There are so many other application in pharmaceutical research also have been seen these days as it also deals with systems biology and pathways of metabolites and their relation to biological functioning similarity. Recent Advancement However bioinformatics is still in its nascent stage, but continuous improvement is making it more efficient. Mostly with inclusion of various computer language incorporation in this field and development of software packages for analysis of biological data is contributing to recent advancement in this field. Drug designing software packages like "Sanjeevni" developed by 1 1T Delhi, India maestro from Schrodinger also is contributing a lot to it. Indian agricultural statistical research institute (IASRI) also is making a huge contribution towards bioinformatics research by creating so many databases on agricultural and biological area. Most recent use of bioinformatics has been seen in novel drug molecule discovery and ligand analysis for protein targets in human physiological cycle to receive most possible cure for lethal diseases in short period]. There is plenty of docking software available, which are very efficient and proved their accuracy also Review and conclusion With inclusion of large number of tools and implementation of bioinformatics in various biological research areas, it is now showing its existence and importance simultaneously. Now a day every experiment in biological research is getting associated with bioinformatics. It has made research very simple and fast, but still validation for various techniques are still in process for its accuracy. We are able to get a lot of results in a minute, which was not possible by using wet lab techniques in biotechnology. Guide to NCBI Databases tools & Services/uses. The National Center for Biotechnology Information (NCBI), a division of the National Library of Medicine (NLM), has created a large number of databases that are freely available to researchers. These databases represent a vast store of information about genetics, genomics, proteomics, and medicine. All of these databases can be reached from the Entrez search page. This page also allows cross searching of all the NCBI databases, a feature called Entrez Global Query. There are two main functions of biological databases: 1. Make biological data available to scientists. As much as possible of a particular type of information should be available in one single place (book, site, database). Published data may be difficult to find or access, and collecting it from the literature is very time-consuming. And not all data is actually published in an article. 2. To make biological data available in computer-readable form. Since analysis of biological data almost always involves computers, having the data in computerreadable form (rather than printed on paper) is a necessary first step. One of the first biological sequence databases was probably the book "Atlas of Protein Sequences and Structures" by Margaret Dayhoff and colleagues, first published in 1965. It contained the protein sequences determined at the time, and new editions of the book were published well into the 1970s. Its data became the foundation for the PIR database. The computer became the storage medium of choice as soon as it was accessible to ordinary scientists. Databases were distributed on tape, and later on various kinds of disks. When universities and academic institutes were connected to the Internet or its precursors (national computer networks), it is easy to understand why it became the medium of choice. And it is even easier to see why the World Wide Web (WWW, based on the Internet protocol HTTP) since the beginning of the 1990s is the standard method of communication and access for nearly all biological databases. As biology has increasingly turned into a data-rich science, the need for storing and communicating large datasets has grown tremendously. The obvious examples are the nucleotide sequences, the protein sequences, and the 3D structural data produced by X-ray crystallography and macromolecular NMR. An new field of science dealing with issues, challenges and new possibilities created by these databases has emerged: bioinformatics. Other types of data that are or will soon be available in databases are metabolic pathways, gene expression data (microarrays) and other types of data relating to biological function and processes. One very important issue is the frequency and type of errors that the entries in a database have. Naturally, this depends strongly on the type of data, and whether the database is curated (added, deleted, or modified by a defined group of people) or not. For the sequence databases, the errors may be either in the sequence itself (misprint, wrong on entry, genuine experimental error...) or in the annotation (mistaken features, errors in references,...). In the 3D structure database (PDB), structures have been deposited which were later discovered to contain severe errors. The error handling policy differs considerably between databases. If one needs to use any particular database heavily, then the implications of its particular policy need to be considered. The present document will touch on only the largest and most frequently used databases. We will begin with an introduction to the Entrez search interface and will then proceed to the details of some of the individual NCBI databases. Entrez Entrez is the unified search interface for NCBI databases. This common interface allows easy linking between results in different databases. Entrez Search Tips The Boolean operators AND, OR, and NOT may be used and must be in all caps. To see exactly how Entrez has interpreted your query, see the "Search details" box on the right side of the screen. Use quotation marks to enclose a phrase. Use the asterisk for truncation (e.g., bacteri* will retrieve bacteria, bacterium, bacteriophage). Enter author names in the format Johnson AB with no punctuation. Entrez will recognize this as an author name and search only that field. When in doubt, or when the initials are not known, use Johnson[AUTHOR]. Clicking on "Advanced Search" will display a numbered list of searches for the current session. Previously run searches may be combined using the syntax #2 AND Field specific searching is also available under "Advanced Search." Alternately, one can use Entrez search field qualifiers (e.g., rbcL[GENE] to search only the gene name field). BLAST Like Entrez, BLAST (Basic Local Alignment Search Tool) is not a database itself, but a means of accessing the data in NCBI databases, particularly in the nucleotide and protein databases. BLAST allows researchers to directly search nucleotide or protein sequence data. For instance, a researcher can submit a sequence through BLAST to see if there are similar sequences already in the NCBI databases. In addition, BLAST can be used to align two sequences using a tool called bhseq. PubMed PubMed is the major bibliographic database from NCBI. It searches MEDLINE, a database from the NLM that covers "medicine, nursing, dentistry, veterinary medicine, the health care system, and the preclinical sciences, such as molecular biology." PubMed also allows access to articles that are out of scope for MEDLINE, but which appear in journals indexed by MEDLINE. All material included in PubMed Central (NLM's online journal archive) is indexed, as well as a few additional databases from NL-M. PubMed employs a system called Automatic Term Mapping to match search terms to the Medical Subject Headings (MeSH) vocabulary. To see how terms have been mapped, see the "Search details" box on the right side of the screen. This is an invaluable tool for troubleshooting a search. Clicking "Advanced Search" on the PubMed search page provides many options for customizing a search. Limits include human vs. animal subjects, male vs. female subjects, age of subjects, article type, and journal type. Advanced search will also display your search history. The PubMed help file provides guidance on structuring searches and managing search results. Online Mendelian Inheritance in Man (OMIM) OMIM is a database from Johns Hopkins University for human genetics containing short articles with references on genetic disorders. It is an excellent starting point for any question involving human genetics as it links out to bibliographic records in PubMed and to sequence records. Nucleotide Databases The nucleotide sequence data in NCBI is a composite of the data from GenBank, the European Molecular Biology Laboratory (EMBL) and the DNA Databank of Japan (DDBJ). NCBl's nucleotide data is divided into three sub-databases: 1. GenBank Expressed Sequence Tags (EST) — these are generally short sequences derived from mRNA isolated from a particular tissue at a particular stage of development. 2. GenBank Genome Survey Sequence (GSS) - these are sequences derived from whole- genome sequencing projects. 3. CoreNucleotide — all nucleotide sequences that are not ESTs or GSSs. Confusingly, the links on the Entrez search page to EST, GSS, and CoreNucleotide all go to the same Entrez Nucleotide search interface, so when a search is performed in any one of the sub-databases, results are returned from all three. Links are provided at the top of the results page so that results from a particular sub-database may be isolated. Among the nucleotide sequences, there are some that are uncurated, meaning that they are in the database just as they were submitted by researchers. Other records are referred to as reference sequences (or RefSeq) and are curated by NCBI. RefSeq records are identified by accession numbers beginning with two letters and an underscore (e.g., NM For more information about the nucleotide databases, see Chapter 1 of the NC-BI Handbook. For more information about the RefSeq project, see Chapter 18. Protein Database The protein database contains data from GenBank, EMBL, and DDBJ as well as sequences submitted to various other sources including SWISS-PROT. As with the nucleotide database, RefSeq records are identified by accession numbers beginning with two letters and an underscore. Genome Database The genome database provides views of entire genomes and chromosomes. Results are displayed via NCBl's Map Viewer, from which the user can zoom in on a region of interest. The Map Viewer is highly customizable, allowing users to control what types of maps are displayed and the level of resolution. Links to other NCBI databases are provided. For help using the Map Viewer, see Chapter 20 of the NCBI Handbook. Structure Database The structure database contains three-dimensional images of proteins from the protein database. It is searchable by keyword or by protein or nucleotide sequence. Protein images can be manipulated using the free CN3D tool. Help is available from the database help screen. Gene Database The gene database allows the user to search for individual genes from among the genomes represented in RefSeq, providing useful summary statements about the gene and links to other NCBI databases. As in the Genome database, results may be examined using the sequence viewer. Taxonomy The taxonomy database contains the names of all organisms that are represented by nucleotide or protein sequences in the NCBI databases. Records contain links to higher taxa, nomenclatural synonyms, and links to the various databases in which records for a given organism reside. DNA and Protein sequencing and analysis. Introduction: Before 1970's there was no direct method to determine the nucleotide sequence. In the mid of 1970's, two methods developed for the direct sequencing of DNA. These were the Sanger Coulson's chain termination method and Maxam Gilbert's chain termination method. For which they shared Nobel Prize in Chemistry (1980). DNA sequencing is the process of determining the precise order of nucleotides within a DNA molecule. It includes any method or technology that is used to determine the order of the four bases—adenine, guanine, cytosine, and thymine—in a strand of DNA. The advent of rapid DNA sequencing methods has greatly accelerated biological and medical research and discovery.[l ] Knowledge of DNA sequences has become indispensable for basic biological research, and in numerous applied fields such as medical diagnosis, biotechnology, forensic biology, virology and biological systematics. The rapid speed of sequencing attained with modern DNA sequencing technology has been instrumental in the sequencing of complete DNA sequences, or genomes of numerous types and species of life, including the human genome and other complete DNA sequences of many animal, plant, and microbial species. DNA sequencing may be used to determine the sequence of individual genes, larger genetic regions (i.e. clusters of genes or operons), full chromosomes or entire genomes, of any organism. DNA sequencing is also the most efficient way to sequence RNA or proteins (via their open reading frames). In fact, DNA sequencing has become a key technology in many areas of biology and other sciences such as medicine, forensics, or anthropology The first DNA sequences were obtained in the early 1970s by academic researchers using laborious methods based on two-dimensional chromatography. Following the development of fluorescencebased sequencing methods with a DNA sequencer A sequencing has become easier and orders of magnitude faster. Previous Questions 1. Bioinformatics is an Interdisciplinary Discipline - Explain Bioinformatics is inherently interdisciplinary because it combines principles and methodologies from multiple fields to analyze and interpret complex biological data. The primary disciplines that converge in bioinformatics include: Biology: Provides the foundational understanding of living systems, including genomics, proteomics, and cellular processes. Computer Science: Develops algorithms, software tools, and databases to handle and analyze vast biological datasets. Mathematics and Statistics: Enables the development of models, algorithms, and statistical measures to identify patterns and relationships in data. Engineering: Contributes to system designs and computational frameworks that process biological information efficiently. Bioinformatics addresses diverse challenges, such as sequence alignment, genome annotation, and modeling molecular interactions. It enables large-scale biological analysis that was previously impractical, paving the way for innovations in fields like medicine, agriculture, and environmental science. 2. Goals and Scope of Bioinformatics Goals of Bioinformatics: 1. Data Organization: o Create and maintain databases for biological data, such as DNA, RNA, and protein sequences. o Example: The Protein Data Bank for 3D macromolecular structures. 2. Tool Development: o Design computational tools for efficient access, management, and analysis of biological data. o Examples: BLAST for sequence alignment, tools for structure prediction. 3. Data Analysis: o Derive meaningful insights by analyzing large datasets. o Applications: Gene function prediction, drug discovery, and evolutionary analysis. Scope of Bioinformatics: Genomics and Proteomics: Genome sequencing, annotation, and protein structure prediction. Systems Biology: Modeling interactions in metabolic pathways and gene regulation networks. Comparative Genomics: Identifying conserved and divergent genes among species. Medical Applications: Understanding genetic diseases and personalized medicine through genomic data. Agriculture: Crop improvement using genetic data to enhance traits like disease resistance. 3. Major Areas of Computational Evolutionary Biology and Comparative Genomics Computational Evolutionary Biology: This field uses computational tools to study the evolution and ancestry of organisms. Major areas include: Phylogenetics: Reconstructing evolutionary trees to understand species divergence. Population Genetics Models: Simulating and predicting genetic variations in populations over time. Genome Evolution Studies: Understanding gene duplications, horizontal gene transfers, and chromosomal rearrangements. Comparative Genomics: This involves comparing genomes across species to identify similarities and differences. Key areas include: Orthology Analysis: Establishing correspondence between genes across species to trace evolutionary processes. Mutation Studies: Analyzing point mutations, gene duplications, and structural variations like transpositions and inversions. Pan-genomics: Dividing genomes into core (common to all species) and dispensable (specific to some species) components to study diversity within a group. 4. Application of Bioinformatics in Genetics of Disease and Analysis of Mutation in Cancer Genetics of Disease: Bioinformatics plays a pivotal role in identifying and understanding the genetic basis of diseases: Genome-Wide Association Studies (GWAS): Identify DNA variants linked to diseases like diabetes and Alzheimer’s. Diagnostic and Prognostic Tools: Use genetic markers to predict disease susceptibility and progression. Personalized Medicine: Tailoring treatments based on an individual’s genomic data. Analysis of Mutations in Cancer: Bioinformatics is essential for decoding the complex genomic rearrangements in cancer cells: Mutation Identification: Advanced sequencing techniques detect somatic mutations in cancer genomes. Driver vs. Passenger Mutations: Distinguish mutations contributing to cancer (drivers) from incidental ones (passengers). Data Integration: Use tools like Hidden Markov Models to process vast amounts of noisy data from high-throughput sequencing. Tracking Disease Progression: Monitor mutations over time to guide treatment decisions. 5. Techniques and Tools Used in Analysis of Gene and Protein Expression Gene Expression Analysis: Techniques: o Microarrays: Measure mRNA levels across thousands of genes. o RNA-Seq (Whole Transcriptome Sequencing): High-throughput sequencing to analyze gene expression comprehensively. Tools: o Cluster Analysis: Groups genes based on co-expression (e.g., k-means clustering). o Promoter Analysis: Identifies regulatory motifs influencing gene expression. Protein Expression Analysis: Techniques: o Protein Microarrays: Assess protein abundance and interactions. o Mass Spectrometry (MS): Identify and quantify proteins in complex mixtures. Tools: o BLAST and PSI-BLAST: Identify similar protein sequences. o Proteomics Databases: Repositories like UniProt and Protein Data Bank. Regulatory Analysis: Promoter and enhancer analysis using tools like MEME to study gene expression regulation. Chromosome conformation capture (e.g., Hi-C) reveals 3D genome organization. By combining these tools and techniques, researchers can interpret large datasets, revealing insights into cellular mechanisms and their regulatory networks. 6. Define: a. Structural Bioinformatics Structural bioinformatics is a branch of bioinformatics that focuses on the analysis and prediction of the three-dimensional structure of biological macromolecules like proteins, DNA, and RNA. Its goals include: Understanding the relationship between structure and function of biomolecules. Predicting protein folding and molecular interactions using techniques like homology modeling, molecular docking, and protein threading. Studying molecular interactions such as protein-protein and protein-ligand binding to aid in drug discovery. b. Network and Systems Biology Network and systems biology involves the study of complex biological networks, such as protein- protein interaction networks, metabolic pathways, and gene regulatory networks. Its main goals include: Understanding how biological entities (genes, proteins, metabolites) interact to carry out cellular processes. Modeling and simulating these interactions to predict cellular behavior under different conditions. Integrating diverse data types (e.g., genomic, transcriptomic, proteomic) to form a comprehensive understanding of biological systems. 7. What Do You Understand by Biological Databases? What Are Their Main Functions? Biological Databases: Biological databases are repositories designed to store, organize, and retrieve biological data such as DNA sequences, protein structures, or genome annotations. These databases are essential for managing the vast amount of information generated in modern biology. Main Functions: 1. Data Storage: Provide a centralized location to store biological data in an organized and easily accessible manner. 2. Data Sharing: Enable the dissemination of biological data to the scientific community. 3. Search and Retrieval: Allow users to efficiently search for specific information using advanced query systems. 4. Integration: Link various datasets (e.g., genes, proteins, pathways) to provide a holistic view of biological phenomena. 5. Annotation and Curation: Ensure the quality and accuracy of stored data through expert review and updates. 8. Names of Human Genome, Vertebrate, and Invertebrate Databases Human Genome Databases: dbVar ENCODE Project Ensembl Human UCSC Human Genome Browser 1000 Genomes Project HapMap Project Vertebrate Databases: ZFIN (Zebrafish Information Network) MGI (Mouse Genome Informatics) Ensembl Vertebrate Databases AgBase (Agricultural species) ARKdb (Cat, Cow, Horse, and other vertebrates) Invertebrate Databases: WormBase (C. elegans) VectorBase (Human pathogen vectors) FlyBase (Drosophila genome) SGD (Saccharomyces Genome Database) EcoGene (E. coli sequence and function) 9. Short Note on: History of Bioinformatics The term "bioinformatics" was coined in the 1970s by Paulien Hogeweg and Ben Hesper to describe the study of information processes in biological systems. Initially, the field focused on sequence analysis as advancements in molecular biology made protein and DNA sequences available. Margaret Dayhoff pioneered the creation of protein sequence databases, which later evolved into comprehensive computational tools. The Human Genome Project in the 1990s gave bioinformatics a massive boost by requiring computational methods to manage and analyze the vast genomic data. Since then, bioinformatics has grown into a core discipline, integrating genomics, proteomics, and systems biology. 10. Short Note on: Genome Annotation Genome annotation is the process of identifying and marking functional elements within a genome, such as genes, regulatory sequences, and other features. It involves two key steps: Structural Annotation: Identifying gene locations, coding regions, and intron/exon boundaries. Functional Annotation: Assigning roles to genes or sequences based on homology, experimental data, or computational predictions. Automated tools like GeneMark and Ensembl are widely used for annotation due to the scale of modern genomic data. Genome annotation is vital for understanding gene function, regulatory mechanisms, and evolutionary relationships. 11. Short Note on: Application of Bioinformatics in Current Research Bioinformatics plays a crucial role in various domains of current research: Genomics: Assists in genome sequencing, annotation, and comparative analysis. Drug Discovery: Identifies drug targets and simulates molecular interactions for new therapies. Personalized Medicine: Uses genomic data to develop individualized treatment plans. Agriculture: Enhances crop traits by studying genetic variations and regulatory pathways. Evolutionary Studies: Tracks evolutionary changes using genomic and proteomic data. Bioinformatics tools like BLAST, genome browsers, and molecular docking software streamline these applications, making it indispensable in modern biological research. Summary from gpt: Summary of Key Topics This comprehensive text outlines the vast scope of bioinformatics, emphasizing its role in sequence analysis, structural biology, and systems biology. Sequence Analysis: DNA Sequencing and Assembly: o Sequences are analyzed to determine genes, regulatory elements, and structural motifs. o Techniques like shotgun sequencing assemble short DNA fragments to reconstruct entire genomes. Genome Annotation: o Automates the identification of genes and functional elements in genomes using start and stop regions. o Tools like GeneMark enhance annotation accuracy. Comparative Genomics: o Analyzes genome similarities and differences to trace evolutionary processes (e.g., mutations, duplications). o Techniques identify conserved and flexible genomic components, aiding species- specific studies. Pan Genomics: o Divides genomes into core (common) and dispensable/flexible genes to study gene diversity within taxa. Disease Genetics and Cancer Mutations: Genetics of Disease: o Next-generation sequencing links genetic variants to complex diseases like diabetes and cancer. o Genome-Wide Association Studies (GWAS) identify disease-related mutations for diagnosis and prognosis. Cancer Genomics: o Sequencing uncovers mutations (driver vs. passenger) and structural variations in cancer cells. o Computational tools manage large datasets and refine predictions using Hidden Markov Models and other algorithms. Gene and Protein Expression: Gene Expression Analysis: o Techniques like microarrays and RNA-Seq measure mRNA levels to identify upregulated or downregulated genes. Protein Expression Analysis: o Tools like protein microarrays and mass spectrometry analyze protein abundance and interactions. Regulatory Analysis: o Studies promoter regions and enhancer interactions to understand gene expression control. Structural Bioinformatics: Protein Structure Prediction: o Homology modeling, protein threading, and de novo modeling predict protein structures and functions. o Studies identify critical regions in proteins for interactions and stability. Protein-Protein and Protein-Ligand Interactions: o Docking algorithms simulate molecular interactions, crucial for drug design and systems biology. Systems Biology and Network Analysis: Network Analysis: o Integrates diverse biological data (e.g., genes, proteins) to map interactions in metabolic or regulatory networks. Systems Biology: o Uses simulations to model cellular processes, aiding in understanding pathways like metabolism and gene regulation. Molecular Interaction Networks: o Examines physical and functional relationships between proteins, leveraging data from X-ray crystallography and NMR spectroscopy. This text highlights bioinformatics as a multidisciplinary field that accelerates research across genomics, proteomics, disease studies, and computational biology through advanced tools and techniques. Relation to Other Fields - Summary Bioinformatics intersects with various disciplines but remains distinct in its focus and application: Key Relationships: 1. Bioinformatics vs. Biological Computation: o Biological Computation: Combines bioengineering and biology to create biological computers. o Bioinformatics: Uses computational tools to analyze biological data like DNA, RNA, and protein sequences. 2. Bioinformatics and Computational Biology: While often used interchangeably, bioinformatics focuses on data management and o tool development, whereas computational biology emphasizes modeling and understanding biological systems. 3. Growth Factors: o The field saw significant growth in the mid-1990s due to advancements in DNA sequencing technologies and projects like the Human Genome Project. 4. Methods and Algorithms: o Bioinformatics relies on software that incorporates: Graph Theory and Artificial Intelligence: For pattern recognition and network analysis. Data Mining and Image Processing: To extract insights from complex datasets. Computer Simulation: To model biological processes. o These methods are rooted in foundational disciplines such as discrete mathematics, system theory, information theory, and statistics. Bioinformatics acts as a bridge, integrating computation with biological research to derive meaningful insights from large-scale biological data. Sequence Development - Summary The section provides an overview of the basic biological concepts and challenges associated with sequence analysis and its applications in bioinformatics. Key Concepts: 1. Biomolecules: o DNA and RNA are nucleotide sequences; proteins are amino acid sequences. o DNA is the blueprint for an organism, containing coding (genes) and non-coding regions that regulate gene expression. o RNA is usually single-stranded, while DNA is double-stranded with complementary base-pairing (A-T, G-C). 2. Proteins: o Composed of 20 amino acids, proteins fold into unique three-dimensional structures critical for their function. o Proteins regulate genes, catalyze reactions, and are essential for cellular processes. 3. Central Dogma: o DNA is transcribed into mRNA, which is translated into proteins. o Codons (3-nucleotide sequences) specify amino acids; start codon (ATG) codes for methionine, and stop codons signal the end of translation. Applications in Bioinformatics: 1. Biological Problems Addressed by DNA Sequence Analysis: o Cellular Processes: Understanding tasks like mitosis and responses to external conditions. o Development: Studying gene regulation during organismal growth and cell differentiation. o Evolution: Tracing species evolution and the development of new functionalities. 2. Gene Regulation and Function: o Analysis of DNA and regulatory sequences aids in understanding gene activation. o Comparative studies of homologous genes (orthologues) across organisms highlight evolutionary conservation. 3. Gene Duplication: o Gene duplications lead to functional diversification and are a driving force in evolution. 4. High-Throughput Experiments: o Microarrays and similar tools analyze gene expression patterns, enabling clustering and interpretation of vast data. 5. Phylogenetics and Taxonomy: o DNA sequencing has revolutionized the study of evolutionary relationships and organism classification. This foundational understanding bridges biology and computational techniques, allowing bioinformatics to address diverse biological challenges effectively. Aims and Tasks of Bioinformatics - Summary Bioinformatics has three primary aims: 1. Data Organization: o Store, curate, and provide access to biological data, such as 3D macromolecular structures in the Protein Data Bank. o Ensure accessibility for researchers to retrieve and contribute new entries. 2. Tool Development: o Create computational tools (e.g., FASTA, PSI-BLAST) to analyze and compare biological data. o These tools require expertise in both computational theory and biological understanding. 3. Data Analysis and Interpretation: o Use developed tools to extract biologically meaningful insights from data. o Enable large-scale analyses across diverse datasets to uncover common principles and identify novel features. By achieving these aims, bioinformatics supports advanced biological research and facilitates discoveries through comprehensive data integration and analysis. NCBI Databases Overview 1. Purpose of Biological Databases: o Make biological data accessible to researchers in a centralized, searchable, and computer-readable form. o Examples include nucleotide sequences, protein structures, and gene expression data. 2. Key Tools and Databases: o Entrez: A unified search interface for accessing various NCBI databases with features like Boolean operators, truncation, and advanced search capabilities. o BLAST (Basic Local Alignment Search Tool): Aligns nucleotide or protein sequences to find similarities and infer functional or evolutionary relationships. o PubMed: Bibliographic database for literature in medicine, biology, and related fields, featuring MEDLINE and PubMed Central records. o OMIM (Online Mendelian Inheritance in Man): Focuses on human genetics and genetic disorders, linking to PubMed and sequence data. 3. Major Databases and Their Functions: o Nucleotide Databases: Include GenBank, EMBL, and DDBJ; divided into sub-databases like EST (Expressed Sequence Tags), GSS (Genome Survey Sequence), and CoreNucleotide. o Protein Database: Contains protein sequences from GenBank, SWISS-PROT, and more, with curated RefSeq records for reliability. o Genome Database: Displays entire genomes and chromosomes, with tools like the Map Viewer for customized visualizations. o Structure Database: Stores 3D protein images, searchable by keywords or sequences, with interactive tools like CN3D. o Gene Database: Provides information on individual genes with links to related data in RefSeq and genome viewers. o Taxonomy Database: Catalogs all organisms represented in NCBI databases, linking to higher taxa and associated datasets. 4. Development of Databases: o Evolved from printed formats (e.g., Atlas of Protein Sequences by Margaret Dayhoff) to digital formats, leveraging the internet and WWW for easy accessibility. o As biology has become data-rich, bioinformatics emerged to manage, analyze, and interpret these vast datasets. 5. Error Management and Curation: o Errors in sequence or annotation are common and vary across curated and uncurated databases. o Users must consider database policies regarding error handling and curation for effective use. NCBI tools and databases provide critical resources for genetics, genomics, proteomics, and more, facilitating research with advanced search and visualization capabilities. The provided content focuses on various aspects of bioinformatics techniques and applications related to genomics, sequencing, and computational analysis. Here's a summarized version: Key Topics in Bioinformatics 1. Genomic Mapping and Databases: o Gene mapping determines the position and distance between genes in an organism's genome. o Genome maps enable a comprehensive understanding of an organism’s genome. 2. Information Retrieval from Biological Databases: o Databases store text, sequences, and structural data, allowing researchers to retrieve precise information for biological studies. 3. Sequence Alignment and Database Searching: o Essential for comparing DNA or protein sequences to predict their structure and function. o Tools like BLAST support pairwise and multiple sequence alignments. 4. Predictive Methods Using DNA and Protein Sequences: o DNA-based methods: Content-based: Analyze sequence properties like codon usage and repeats. Site-based: Detect motifs like splice sites and transcription factor binding sites. Comparative: Use sequence homology for identifying coding regions. o Protein-based methods: Tools like PSI-Pred and others analyze motifs, domains, and peptide-level data. 5. Sequence Assembly and Finishing: o Assembly: Align and order DNA sequence fragments. o Finishing: Verify and refine sequences, resolving conflicts and filling gaps. 6. Phylogenetic Analysis: o Studies evolutionary history through sequence and structural similarities. o Tools like PHYLIP use algorithms like UPGMA and neighbor-joining to construct phylogenetic trees. 7. Comparative Genome Analysis: o Compares genomes to identify conserved and unique genes, aiding in understanding gene function and evolutionary changes. 8. Large-Scale Genome Analysis: o Enabled by advanced sequencing technologies like Illumina, it involves rapid genome sequencing and analysis. o Applications extend to systems biology, pharmaceutical research, and metabolic pathway studies. Bioinformatics integrates advanced computational tools with biological research, revolutionizing genome studies, evolutionary analysis, and biomedical advancements. Some more summary/ques Below is an overview of the answers to the bioinformatics questions you've posed: 1. Bioinformatics Overview What is bioinformatics, and how is it used in biological studies? Explain the interdisciplinary nature of bioinformatics with examples. 1. Bioinformatics Overview Bioinformatics is the interdisciplinary field that combines biology, computer science, and information technology to analyze biological data, such as genetic sequences. It plays a key role in biological studies by enabling the storage, analysis, and interpretation of large biological datasets (like genomes). Bioinformatics is essential in genomics, molecular biology, and systems biology. It aids in tasks such as gene identification, sequence alignment, and drug development. 2. History of Bioinformatics Who were the pioneers in bioinformatics, and what were their contributions? How did the Human Genome Project influence the growth of bioinformatics? 2. History of Bioinformatics Key pioneers in bioinformatics include: Margaret Dayhoff: One of the first to apply computational methods to protein sequences. Paulien Hogeweg: Co-coined the term "bioinformatics" in the 1970s. The Human Genome Project (HGP), completed in 2003, accelerated the growth of bioinformatics by generating massive amounts of genetic data, pushing the need for computational tools to analyze and interpret this data. 3. Goals and Tasks of Bioinformatics What are the three primary aims of bioinformatics, and how are they achieved? How does bioinformatics differ from traditional biological research methods? 3. Goals and Tasks of Bioinformatics The primary aims of bioinformatics are: 1. Data Management: Storing and organizing large biological datasets (like genomic sequences). 2. Data Analysis: Analyzing biological data to identify patterns or anomalies. 3. Data Interpretation: Understanding the biological significance of data (e.g., function of genes). Bioinformatics differs from traditional biological research by leveraging computational tools and algorithms for high-throughput data analysis, as opposed to manual experiments. 4. Sequencing and Sequence Analysis What are the steps involved in DNA sequencing and sequence assembly? How is shotgun sequencing performed, and what are its challenges in genome assembly? 4. Sequencing and Sequence Analysis DNA sequencing involves the steps of DNA extraction, amplification, sequencing, and data analysis (alignment and assembly). Shotgun sequencing is performed by randomly breaking DNA into small fragments and sequencing them, followed by assembling the sequences into the full genome. Challenges include dealing with repeated regions and errors in sequencing data. 5. Comparative Genomics Define orthology analysis and its role in comparative genomics. How does comparative genomics help in understanding evolutionary changes? 5. Comparative Genomics Orthology analysis involves comparing genes in different species to determine their evolutionary relationships. Comparative genomics provides insights into evolutionary changes, gene conservation, and adaptations across species. 6. Gene and Protein Analysis What tools and algorithms are used to predict protein structure and function? Discuss the importance of protein localization in functional analysis. 6. Gene and Protein Analysis Tools like BLAST, Pfam, and InterPro are used to predict protein structure and function. Protein localization is crucial for understanding a protein's role in the cell, as its location can determine its function. 7. Applications of Bioinformatics in Current Research How is bioinformatics applied in novel drug discovery? Explain how bioinformatics contributes to agricultural research. 7. Applications of Bioinformatics in Current Research Bioinformatics is applied in drug discovery by identifying drug targets, screening potential drug compounds, and analyzing clinical trial data. In agriculture, bioinformatics helps in improving crops by analyzing genetic data to identify traits like disease resistance or drought tolerance. 8. Techniques in Mutation and Cancer Genomics What are the challenges in analyzing mutations in cancer genomes? Explain the difference between driver mutations and passenger mutations. 8. Techniques in Mutation and Cancer Genomics Challenges in analyzing mutations in cancer genomes include the complexity and heterogeneity of tumors. Driver mutations are the genetic alterations that directly contribute to cancer, while passenger mutations do not influence cancer progression. 9. Computational Evolutionary Biology What are the major computational models used in evolutionary biology? How do bioinformatics tools assist in reconstructing the tree of life? 9. Computational Evolutionary Biology Computational models such as molecular clock models, coalescent theory, and phylogenetic trees are used to study evolutionary relationships. Bioinformatics tools help reconstruct the tree of life by analyzing genetic variations and comparing sequences from different species. 10. Network and Systems Biology What is the role of network analysis in studying protein-protein interactions? How does systems biology integrate data from different biological levels? 10. Network and Systems Biology Network analysis is used to study protein-protein interactions (PPIs), which are crucial for cellular processes. Systems biology integrates data from various biological levels (e.g., genomics, transcriptomics, proteomics) to provide a holistic understanding of cellular functions. 11. Genome Annotation What are the key components of structural and functional genome annotation? Discuss the significance of ENCODE and its contributions to genome annotation. 11. Genome Annotation Genome annotation involves identifying genes and their regulatory regions, as well as predicting their functions. The ENCODE project has contributed significantly to functional genome annotation by providing comprehensive data on human genome function, such as identifying regulatory elements. 12. Bioinformatics Databases What are the differences between curated and uncurated biological databases? Name and describe the primary public sequence repositories. 12. Bioinformatics Databases Curated databases are manually reviewed and updated for accuracy (e.g., GenBank), while uncurated databases are automatically populated (e.g., Ensembl). Major public sequence repositories include: GenBank: A comprehensive nucleotide sequence database. EMBL-EBI: Hosts many biological databases and tools for analysis. DDBJ: A collaborative project with GenBank and EMBL. 13. Gene Regulation and Expression How is promoter analysis used to study gene expression? Explain the clustering algorithms commonly applied in gene expression studies. 13. Gene Regulation and Expression Promoter analysis is used to study gene expression by identifying regulatory regions upstream of genes. Clustering algorithms like k-means or hierarchical clustering are applied in gene expression studies to group genes with similar expression patterns. 14. Structural Bioinformatics What is homology modeling, and how is it used in protein structure prediction? Discuss the techniques used for predicting protein structures de novo. 14. Structural Bioinformatics Homology modeling predicts protein structures based on known structures of similar proteins. Techniques for de novo prediction include methods like ab initio modeling, which predicts protein structures without using homologous templates. 15. Large-Scale Genome Analysis What advancements in sequencing technologies have improved large-scale genome analysis? How does bioinformatics assist in managing and analyzing large genomic datasets? 15. Large-Scale Genome Analysis Advancements in next-generation sequencing (NGS) technologies have significantly improved large- scale genome analysis by providing faster and cheaper sequencing. Bioinformatics tools manage and analyze vast amounts of genomic data, helping researchers identify key genetic variants. 16. Techniques in Phylogenetics Describe the role of tools like PHYLIP in phylogenetic analysis. How does molecular phylogeny differ from traditional taxonomy? 16. Techniques in Phylogenetics PHYLIP is a widely used software suite for phylogenetic analysis. Molecular phylogeny uses genetic data to reconstruct evolutionary relationships, while traditional taxonomy relies on morphological traits. 17. Applications of Bioinformatics in Medicine How does bioinformatics support personalized medicine? Discuss the role of genome-wide association studies (GWAS) in identifying disease-related genes. 17. Applications of Bioinformatics in Medicine Bioinformatics supports personalized medicine by analyzing an individual's genetic data to predict disease risk and tailor treatments. Genome-wide association studies (GWAS) help identify genes associated with diseases by comparing the genomes of affected and unaffected individuals. 18. Emerging Fields in Bioinformatics What is pan-genomics, and how is it classified into core and dispensable genomes? How has next-generation sequencing transformed cancer genomics research? 18. Emerging Fields in Bioinformatics Pan-genomics studies the complete genetic diversity within a species, including both the core genome (common to all individuals) and the dispensable genome (unique to some individuals). Next- generation sequencing has revolutionized cancer genomics by enabling detailed analysis of tumor genomes, identifying mutations, and guiding treatment strategies. These concepts illustrate the broad and interdisciplinary nature of bioinformatics and its critical role in advancing biological research across various domains. Let me know if you'd like further details on any specific topic!