L13 RNA Sequencing PDF
Document Details
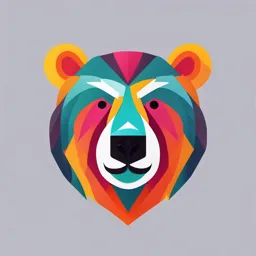
Uploaded by TimeHonoredLimerick2759
King's College London
2022
Natalie Prescott
Tags
Summary
This document from King's College London introduces RNA sequencing (RNA-seq). It covers learning outcomes, the rationale for sequencing RNA, and advantages over other methods for gene expression.
Full Transcript
Large-scale genomics: RNA sequencing Natalie Prescott 5BBG0205 Molecular Basis of Gene Expression Welcome to King’s College London Genetics Teaching Department @lifeatKings Learning outcomes After this lecture you should be able to: Introduce the theory and practice of RNA sequencing (RNA-...
Large-scale genomics: RNA sequencing Natalie Prescott 5BBG0205 Molecular Basis of Gene Expression Welcome to King’s College London Genetics Teaching Department @lifeatKings Learning outcomes After this lecture you should be able to: Introduce the theory and practice of RNA sequencing (RNA-seq) Explain the rationale for sequencing RNA Be aware of the challenges specific to RNA sequencing Discuss the advantages of RNA-seq over other methods for gene expression quantitation Provide an overview of RNA-seq analysis workflows Explain some general goals/applications of RNA-seq projects Welcome to King’s College London Genetics Teaching Department @lifeatKings Why measure gene expression? To tell us which genes are active (being expressed) To tell us how much (what level) they are being expressed To compare expression between tissue or cellular states e.g. normal and defective phenotype (forward genetics) Welcome to King’s College London Genetics Teaching Department @lifeatKings Terminology of gene expression Gene expression means: When the information from a gene is used in the synthesis of a functional gene product (For e.g. protein). To measure gene expression: We often measure the amount of transcription by quantifying the amount of RNA produced by a cell Welcome to King’s College London Genetics Teaching Department @lifeatKings Part 1 Coding and non-coding RNAs Welcome to King’s College London Welcome @lifeatkings to King’s College London Genetics Teaching Department @lifeatKings There is no such thing as ‘junk DNA’ Protein coding genes account for only 3% of the human genome The ENCODE project has shown that 80% of the human genome although ‘noncoding’ is ‘active’ and appears to be doing something! This includes at least 18,400 transcripts that do not result in a protein https://www.encodeproject.org/ Welcome to King’s College London Genetics Teaching Department @lifeatKings Some genes encode non-protein coding RNAs All eukaryotic genes are encoded by DNA For ‘non-coding RNAs’ the RNA is not translated so protein is NOT the ultimate destination It is thought that Human genes that are transcribed into non-protein-coding RNAs may equal or even exceed the number of protein- coding genes * We often shorten ‘non-protein-coding RNAs’ to ‘non-coding RNAs’ Welcome to King’s College London Genetics Teaching Department @lifeatKings Some genes encode non-protein coding RNAs All eukaryotic genes are encoded by DNA For ‘non-coding RNAs’ the RNA is not translated so protein is NOT the ultimate destination It is thought that Human genes that are transcribed into non-protein-coding RNAs may equal or even exceed the number of protein- coding genes * We often shorten ‘non-protein-coding RNAs’ to ‘non-coding RNAs’ Welcome to King’s College London Genetics Teaching Department @lifeatKings You already know some non-coding RNAs 1. tRNAs 2. rRNAs Welcome to King’s College London Genetics Teaching Department @lifeatKings Other classes on non-coding RNAs include… 3. short noncoding RNAs 4. long noncoding RNAs (lncRNA, say Micro RNAs (miRNA) “link RNAs”) Short interfering RNAs (siRNA) Small nuclear RNAs (snRNA) These regulate gene expression They interact with the genome (DNA), or mRNAs, or chromatin to regulate transcription of other genes including protein coding genes, e.g. by RNA interference Welcome to King’s College London Genetics Teaching Department @lifeatKings lncRNAs can help activate or repress transcription Scaffold for protein Scaffold/guide for transcription complexes factors Host genes for miRNA production Competing endogenous RNA for Decoy to inhibit transcription miRNAs Adapted from He et al. Oncotarget 2015 Welcome to King’s College London Genetics Teaching Department @lifeatKings lncRNA functions are diverse and still being identified They have been shown to interact with and/or influence: Chromosome territories Chromatin remodelling Transcription Post transcription processing Translation Post translational modification Welcome to King’s College London Genetics Teaching Department @lifeatKings The eukaryotic transcriptome Welcome to King’s College London Genetics Teaching Department @lifeatKings The eukaryotic transcriptome The set of all the RNAs in a cell or population of cells (tissue) – including protein coding and non-coding Unlike the genome, the transcriptome varies between cells and depending on time, developmental stage and environment Represents all the genes that are being actively expressed at a given time in that cell or tissue Welcome to King’s College London Genetics Teaching Department @lifeatKings End of part 1 quiz 1. How much of the genome is active? a) 3%, b) 50%, c) 80% 2. Which is false? Noncoding RNAs: a) Are encoded in the genome by genes b) Are translated into proteins c) Regulate expression of other genes d) Account for at least half of the genes in the genome 3. What is the transcriptome? a) All the genes in a cell b) All the genes in the genome c) All the coding and non-coding RNAs in a cell d) All the mRNAs in a cell Welcome to King’s College London Genetics Teaching Department @lifeatKings Part 1 summary For many years, the non-coding genome was referred to as junk-DNA the ENCODE project has shown that most of this ‘junk’ DNA has a purpose Non-coding RNAs are functional transcripts that do not result in a protein The number of genes that encode non-coding RNAs may be equal to or even exceed the number of protein-encoding genes rRNA, tRNA, miRNA and lncRNA are all examples of non-coding RNAs The transcriptome is made up of everything that is transcribed at a particular time in a cell, and this will include coding RNA (i.e. protein coding) and non-coding RNAs (non-protein coding) Welcome to King’s College London Genetics Teaching Department @lifeatKings Part 2 High throughput sequencing using RNA as the starting material Welcome to King’s College London Welcome @lifeatkings to King’s College London Genetics Teaching Department @lifeatKings Previous methods for measuring gene expression qPCR Quantitative PCR Requires prior knowledge of the gene sequence Targeted Low throughput – one/few genes at a time Microarrays Hybridisation based Focus on protein coding (poly A) genes Requires sequence knowledge of “all the target genes” high throughput – thousands of genes at a time Welcome to King’s College London Genetics Teaching Department @lifeatKings Transcriptomics High throughput RNA The study of gene expression levels in sequencing each cell or tissue by quantifying the different levels of transcription of all RNAs No limits! Limited to the Allows Limited to the known sequencing of number of clones genes all transcripts made. Usually Limited to only represent the one probe top genes (highest expressed) Gene expression Northern blot microarray Dot blot of cDNA library Welcome to King’s College London Genetics Teaching Department @lifeatKings Review of Sanger sequencing 1. A sequence specific primer anneals to the template 1 to be sequenced 2. 2 Taq polymerase extends the primer using deoxynucleotide triphosphates or dNTPs (A, C, T 1 and G) 3. 3 The dNTPs mix also includes a small amount of 3 dideoxynucleotide triphosphates ddNTPs which 2 have been modified to terminate the chain when they are incorporated. They are each fluorescently labelled a different colour. 5 4. 4 After several rounds of extension all possible 4 lengths of chain are produced terminating with a different fluorophore representing the nucleotide at that position. 5. 5 These chains are separated by capillary electrophoresis and the identity of each terminal nucleotide determined by its fluorescence Welcome to King’s College London Genetics Teaching Department @lifeatKings Video – Illumina high throughput sequencing https://youtu.be/fCd6B5HRaZ8 Welcome to King’s College London Genetics Teaching Department @lifeatKings High throughput RNA sequencing High throughput! Unbiased survey of the entire transcriptome No need for prior knowledge of what genes/transcripts may or may not be present Quantitative the number of sequencing ‘reads’ derived from each RNA is directly related to the starting amount of that RNA in the sample Welcome to King’s College London Genetics Teaching Department @lifeatKings RNA-seq overview 3. Load onto flow cell 1. Sample of interest 2. Isolate all RNA 3. Library preparation Welcome to King’s College London Genetics Teaching Department @lifeatKings RNA-seq overview 3. Load onto flow cell 1. Sample of interest 2. Isolate all RNA 4.Sequencing 3. Library preparation 4. Clonal amplification Welcome to King’s College London Genetics Teaching Department @lifeatKings RNA RNA-seq library 5’ AAAAA preparation 1 Fragment (heat or sonication) 1. 1 Fragment RNA 2 NNNNNN Random primer plus linker 1 RT 2. 2 Make cDNA and add oligo tag 5’ NNNNNN 3. 3 Remove RNA (RNAse H) 3 e As RN 4. 4 Tag the other end by template extension NNNNNNX Random primer (3’ 4 blocked) plus linker 2 Linker 2 Linker 1 NNNNNNX Welcome to King’s College London Genetics Teaching Department @lifeatKings Clonal amplification The library is applied to the flow cell Welcome to King’s College London Genetics Teaching Department @lifeatKings Clonal amplification 1 The template cDNAs attach to the flow cell surface via linker 1 A copy is made via DNA polymerase using oligos on flow cell as a primer Welcome to King’s College London Genetics Teaching Department @lifeatKings Clonal amplification 2 Additional oligos on the flow cell are complementary to linker 2 Welcome to King’s College London Genetics Teaching Department @lifeatKings Clonal amplification 2 Additional oligos on the flow cell are complementary to linker 2 These cause the new strand to form a bridge Another copy is made via DNA polymerase using linker 2 complementary oligo as primer Welcome to King’s College London Genetics Teaching Department @lifeatKings Clonal amplification 3 Additional oligos on the flow cell are complementary to linker 2 These cause the new strand to form a bridge Another copy is made via DNA polymerase using linker 2 complementary oligos as a primer Welcome to King’s College London Genetics Teaching Department @lifeatKings Meanwhile, on other regions of the flow cell, different cDNAs originating from cDNA3 different genes are being amplified in the cDNA2 cDNA4 same way cDNA5 cDNA1 Welcome to King’s College London Genetics Teaching Department @lifeatKings Sequencing by synthesis 1. 1 A primer anneals to the linker at the end of the cDNA fragment attached to the flowcell 1 3 2. 2 Taq polymerase extends the primer using deoxynucleotide triphosphates or dNTPs (A, C, T and G) that have been modified to terminate the 2 chain when they are incorporated. They are each fluorescently labelled a different colour. 3. 3 When the correct dNTP is incorporated an image is taken. dNTPs Welcome to King’s College London Genetics Teaching Department @lifeatKings Sequencing by synthesis 1. 1 A primer anneals to the linker at the end of the cDNA fragment attached to the flowcell 3 1 2. 2 Taq polymerase extends the primer using deoxynucleotide triphosphates or dNTPs (A, C, T and G) that have been modified to terminate the chain when they are incorporated. They are each 2 fluorescently labelled a different colour. 4 5 3. 3 When the correct dNTP is incorporated an image is taken. 4. 4 Unlike Sanger sequencing this modification can be reversed so the next dNTP can then be added 5 The process continues until the whole chain is 5. sequenced Welcome to King’s College London Genetics Teaching Department @lifeatKings RNA-seq vs microarrays RNAseq data is highly reproducible (technical replicates are almost identical) Identifies around 40% more transcripts than microarray RNAseq is better at quantifying very low and very high expressed 2008 Genome Research genes doi: 10.1101/gr.079558.108 Welcome to King’s College London Genetics Teaching Department @lifeatKings Advantages of RNA-seq Can detect alternative splicing and novel exons Allows detection and quantification of novel RNAs Highly sensitive with large dynamic range Sequence read-out can detect mutations in the transcript Welcome to King’s College London Genetics Teaching Department @lifeatKings The challenges of working with transcriptomes Stability G G U G A U Abundance e As RN RNAs from highly expressed genes RNA samples are fragile - (e.g. rRNA) may obliterate/wipe purity, quantity, quality issues out the signal from low expressed genes Size RNA molecules come in a wide ABUNDANCE OF HUMAN range of sizes RNAS ribosomal RNA other noncoding RNA protein coding mRNA 5% 4% 91% Welcome to King’s College London Genetics Teaching Department @lifeatKings End of part 2 quiz 1. Select all that apply. RNA-seq: a) Is highly sensitive b) Requires prior knowledge of gene sequences c) Can identify more transcripts than microarrays d) Can detect novel non-coding RNAs 2. Specifically, the library preparation step in RNA-seq involves…? a) Extraction and purification of whole RNA from a tissue b) The conversion of multiple RNAs into a cDNA library of similarly sized fragments with adapters c) Sequencing by synthesis of clonally simplified cDNAs 3. Why remove ribosomal RNA before RNA-seq? a) They are not very interesting b) They are too small and need to be captured separately c) They are abundant and would consume too many sequencing reads Welcome to King’s College London Genetics Teaching Department @lifeatKings Part 2 summary RNA-sequencing is a form of next generation sequencing (NGS) that uses whole RNA from a cell or tissue as it’s starting material It provides a quantitative unbiased survey of the entire transcriptome The three main steps in RNA-seq after RNA isolation are library preparation, clonal amplification and then sequencing. RNA-seq has several advantages of microarrays including: the ability to detect novel transcripts, it is quantitative over a much larger dynamic range, and it can provide sequence level information. Welcome to King’s College London Genetics Teaching Department @lifeatKings Part 3 Analysis of RNA-seq data Welcome to King’s College London Welcome @lifeatkings to King’s College London Genetics Teaching Department @lifeatKings NGS RNA-seq data output OUTPUT Post sequencing LIBRARY input sequencing Data library Short sequence reads 50 - 150 bp long Up to 500 million reads Format = fastq Prepared cDNA library with adaptors loaded onto a lane of Illumina HiSeq a flow cell Welcome to King’s College London Genetics Teaching Department @lifeatKings.FASTQ files Large text files with millions of lines corresponding to short sequence reads from NGS One file per sample FastQ files make it easy to manipulate and parse nucleotide sequences using simple text-processing tools and scripting languages like R and Python. Welcome to King’s College London Genetics Teaching Department @lifeatKings RNA-seq analysis ‘pipeline’ Downstream (post sequencing) bioinformatic analysis Varies between institutes – although gradually becoming more standardised Computationally intensive - requires knowledge of general-purpose computer programming language such as Perl or Read Transcript Gene Quantification alignment assembly identification Raw Bowtie/TopH Cuffdiff sequence Cufflinks at alignment Cufflinks (A:B data (cuffmerge) (genome) comparison) (.fastq files) Reference Gene Inputs genome annotation CummRbund (.fa file) (.gtf file) Welcome to King’s College London Genetics Teaching Department Visualization @lifeatKings RNA-seq analysis ‘pipeline’ Downstream (post sequencing) bioinformatic analysis Varies between institutes – although gradually becoming more standardised Computationally intensive - requires knowledge of general-purpose computer programming language such as Perl or Read Transcript Gene Quantification alignment assembly identification Raw sequence Bowtie/TopHat Cufflinks Cuffdiff data alignment Cufflinks (cuffmerge) (A:B comparison) (.fastq files) (genome) Reference Gene Inputs genome annotation CummRbund (.fa file) (.gtf file) Welcome to King’s College London Genetics Teaching Department Visualization @lifeatKings Read alignment Putting the puzzle back together Take short sequence reads and based on sequence similarity, find the best match for it in the reference human genome Welcome to King’s College London Genetics Teaching Department @lifeatKings Read alignment genome pre RNA transcript reads Welcome to King’s College London Genetics Teaching Department @lifeatKings Read alignment genome pre RNA transcript reads Welcome to King’s College London Genetics Teaching Department @lifeatKings The level of expression is calculated from the number of aligned sequence reads for the gene’s mRNA Read count = 6 Kidney Gene X Read count = 18 Heart Transcript Quantification The number of reads that align to a gene will be directly proportional to the number of RNA molecules that were present in the original sample Higher read count = higher expression Welcome to King’s College London Genetics Teaching Department @lifeatKings Read count has its limitations when quantifying gene expression via RNA-seq In previous example we were comparing the expression of the same gene in two different tissues What if you wanted to compare the expression of two different genes: Gene X Read count = 16 Gene Y Read count = 16 Welcome to King’s College London Genetics Teaching Department @lifeatKings RPKM – the units of gene expression in RNA-seq Measures gene expression normalized (corrected) by: The total number of reads obtained for the sample The length of gene Reads Per Kilobase of transcript per Million mapped reads RPKM[gene X] = no. of sequence reads[gene X, sample Y] gene length * millions of total aligned reads[all genes, sample Y] Welcome to King’s College London Genetics Teaching Department @lifeatKings Example: Differential gene expression analysis using RNA-seq The genes affected by changes in expression may provide clues to the underlying biology Compare one tissue to another (e.g. tumour & normal) Calculate RPKM per gene in both tissues Compare the fold change difference (FC) Welcome to King’s College London Genetics Teaching Department @lifeatKings Example: Using RNA-seq to identify non-coding mutations in rare disease Patients with rare muscle disorder Genome (DNA) sequencing could not identify any coding mutations They performed RNA-seq of muscle biopsies in 50 undiagnosed patients Compared to muscle RNA-seq data from healthy subjects Cummings et al., Sci Transl Med 2017 Welcome to King’s College London Genetics Teaching Department @lifeatKings Identified a cryptic splice site mutation within an intron that creates a new exon in COL1A6 C>T Diagram of part of COL6A1 gene Sequencing reads showed an apparantly novel exon in four patients Sequencing reads from normal control Leads to insertion of 24 amino acids into the protein which disrupt gene function The inclusion of the novel exonwas found to be due to a C>T mutation creating a strong donor splice site deep within an intron of COL6A1 Sequencing reads from a patient Mutations affecting the coding region of COL1A6 were already known to cause other muscle disorders Welcome to King’s College London Genetics Teaching Department @lifeatKings End of part 3 quiz 1. Select all answers that apply. Read alignment in RNA-seq: a) Uses FASTQ files generated from NGS data b) Can only be performed if the sequence reads are derived from known genes c) Locates short sequence reads in the genome based on sequence similarity d) Is an important stage in the post sequencing analysis pipeline 2. RPKM is often better than ‘read count’ for quantifying gene expression in RNA-seq because (select one correct answer): a) It is easier to calculate b) It allows you to compare expression in different genes that are different sizes c) It tells you a lot about the quality of the sample 3. Which of these technologies below do not involve RNA: a) RNA-seq b) RT-PCR c) ChIP-seq d) Transcriptomics e) Gene expression microarrays Welcome to King’s College London Genetics Teaching Department @lifeatKings Summary part 3 The main challenges of RNA-seq analysis relate to computational burden of read mapping and alignment. Analysis pipeline uses the short sequence reads output from the NGS machine in a text-based FASTQ format As part of the analysis pipeline the short sequence reads are mapped back to the reference genome using alignment algorithms via a suite of programs Sequence read count can be used to accurately quantify gene expression, but when comparing between different genes, gene size must be accounted for by calculating RPKM RNA-seq has a wide range of applications including differential gene expression and genetic diagnosis. Welcome to King’s College London Genetics Teaching Department @lifeatKings Thank you! Welcome to Genetics Teaching King’s College London Welcome Department @lifeatkings to King’s College London Genetics Teaching Department @lifeatKings Why we calculate LOG fold change in differential gene expression Example 1 Normal sample(gene A) = 50 reads Tumour sample(gene A) = 100 reads i.e. gene expression has increased in tumour Fold Change = 100/50 = 2 log2FC = 1 Calculate Example 2 log2 fold Normal sample(gene A) = 100 reads change to Tumour sample(gene A) = 50 reads make up and down scales i.e. gene expression decreased in tumour equal Fold Change is 50/100 = 0.5 log2FC = -1 Welcome to King’s College London Genetics Teaching Department @lifeatKings