MBBS January 23: Introduction to Biostatistics Statistics in Practice (King's College London) PDF
Document Details
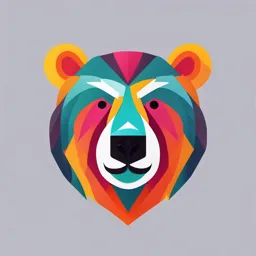
Uploaded by StableEpilogue
King's College London
2023
Dr Yanzhong Wang
Tags
Summary
This presentation introduces biostatistics, specifically focusing on the application of statistics in real research. It includes aims and learning objectives in the topic of biostatistics to outline the key areas of the subject, as well as statistical approaches to clinical problems. The information presented is intended for students of MBBS.
Full Transcript
MBBS January 23: Introduction to Biostatistics Statistics in Practice Dr Yanzhong Wang, Reader in Medical Statistics School of Life Course and Population Sciences Aims for today (learning objectives) ‘To understand the role of statistics in real research through senior s...
MBBS January 23: Introduction to Biostatistics Statistics in Practice Dr Yanzhong Wang, Reader in Medical Statistics School of Life Course and Population Sciences Aims for today (learning objectives) ‘To understand the role of statistics in real research through senior statisticians’ own collaborative research’ (Not to learn statistics as some methods very advanced but to see how it is used. Not for examining) Clinical Speciality Areas: Hepatology Neurology Multimorbidity Contents for talks Statistics in acute liver failure Statistics in stroke Statistics in multimorbidity Example 1: Acute liver failure Development and validation of a dynamic outcome prediction model for paracetamol-induced acute live failure Background Most widely used prediction tool internationally - the King’s College Criteria - is outdated. We aimed to develop a simple prognostic tool to reflect current outcomes and generate a dynamic updated estimation of risk of death. Methods Patients from intensive care units in the UK (London, Birmingham, and Edinburgh) and Denmark (Copenhagen). Development of prognostic models using Cox proportional hazards in a derivation dataset; tested in initial and recent external validation datasets. Model discrimination using area under receiver operating characteristic curve (AUROC) and calibration by root mean square error (RMSE). Results - KCH (King’s College Hospital, London) Results - external data Day1/Day2 predictive models Model performance – external validation sets Predicted survival curves Online app Our new online app will also be able to collect the data entered into it by liver specialists world-wide and use this to further validate and fine-tune the model to reflect what’s happening on the ground. https://alf01.hscr.kcl.ac.uk/FirstCup/ What’s new Previous models were static, our model is dynamic and updating, so the prognosis changes if a patient’s condition changes for worse or better. A practical high performance statistical model, for the first time, to make individual and updated survival predictions with over 90% precision. New online app to collect the data world-wide and to further validate and fine-tune the model Example 2: The South London Stroke Register Long-Term Trends in Incidence and Risk Factors for Ischaemic Stroke Subtypes The South London Stroke Register (南伦敦卒中注册中心) South London Stroke Register Inner city: high level of deprivation Source population of 357308 , made up of 56% White, 25% Black (7% Black Caribbean, 14% Black African, and 4% Black Other), 4% mixed (white and black) and 15% of other ethnic Group (UK census 2011). Southwark Lambeth 22 SLSR Methods & Case Ascertainment SLSR is a prospective study established in 1995. Conducts population-based case ascertainment of first-ever strokes in a defined population of inner London, over 6000 patients so far. Uses multiple overlapping reporting system. Completeness of case ascertainment was estimated at ≈80% (capture- recapture). Aetiological classification of ischaemic stroke by TOAST criteria into: - Large artery atherosclerosis (LAA) - Cardio-embolism (CE) - Small vessel occlusion (SVO) - Other determined (OTH) - Undetermined aetiologies (UND) Statistical Methods 𝑺𝑳𝑺𝑹 𝒅𝒂𝒕𝒂 (𝟐𝟎𝟎𝟎−𝟐𝟎𝟏𝟓) 𝑰𝒏𝒄𝒊𝒅𝒆𝒏𝒄𝒆 = × 100,000 𝑶𝑵𝑺 𝒄𝒆𝒏𝒔𝒖𝒔 𝒆𝒔𝒕𝒊𝒎𝒂𝒕𝒆𝒔 (𝟏𝟗𝟗𝟏, 𝟐𝟎𝟎𝟏, 𝟐𝟎𝟏𝟏) Direct age-standardisation to mid-year population of England and Wales (2011 census). Trends analysed by Poisson regression (2012-15 vs 2000-03), Cochrane- Armitage test, and loess regression. Calculated for each TOAST subtype and further stratified by age ( 20) Statistical themes of multimorbidity research Theme 1: Prevalence and determinants of multimorbidity in an urban, multi-ethnic area over 15-years and the effect of applying resolved/remission codes on prevalence estimates. Theme 2: Identifying longitudinal clusters of multimorbidity in an urban setting by cluster analyses. Theme 3: Inequalities in developing multimorbidity over time: multimorbidity trajectories by multistate Markov chain models. Distribution of patient long-term condition count by age group ( n = 816 901) Long-term condition prevalence among all patients (n = 816 901) The ten most common dyads and triads in the multimorbid sample, comparing the 2005-2010 vs. 2016-2020 cohort. Graphical depiction of chronic diseases, age, gender, deprivation and ethnicity using Multiple Correspondence Analysis Example of a patient’s trajectory as recorded in the dataset Representation of the five state Markov model Representation of the first order Markov chain to model probabilities of acquiring three conditions Transition matrix Selected probabilities from the transition matrices of each multi-state model, for people who enter the study at age 40−59 Multimorbidity research output 1. Bisquera, A., Turner, E. B., Ledwaba-Chapman, L., Dunbar-Rees, R., Hafezparast, N., Gulliford, M., Durbaba, S., Soley Bori, M., Fox-Rushby, J., Dodhia, H., Ashworth, M., & Wang, Y. (2022). Inequalities in developing multimorbidity over time: a population-based cohort study from an urban, multi-ethnic borough in the United Kingdom. The Lancet Regional Health - Europe, 12,. https://doi.org/10.1016/j.lanepe.2021.100247 2. Soley Bori, M., Bisquera, A., Ashworth, M., Wang, Y., Durbaba, S., Dodhia, H., & Fox- Rushby, J. (2021). Identifying multimorbidity clusters with the highest primary care use: 15 years of evidence from a multi-ethnic metropolitan population. British Journal of General Practice. https://doi.org/10.3399/BJGP.2021.0325 3. Ledwaba-Chapman, L., Bisquera, A., Gulliford, M., Dodhia, H., Durbaba, S., Ashworth, M., & Wang, Y. (2021). Applying resolved and remission codes reduced prevalence of multimorbidity in an urban multi-ethnic population. Journal of Clinical Epidemiology, 140, 135-148. https://doi.org/10.1016/j.jclinepi.2021.09.005 4. Bisquera, A., Gulliford, M., Dodhia, H., Ledwaba-Chapman, L., Durbaba, S., Soley Bori, M., Fox-Rushby, J., Ashworth, M., & Wang, Y. (2021). Identifying longitudinal clusters of multimorbidity in an urban setting: a population-based cross-sectional study. The Lancet Regional Health - Europe, 3,. https://doi.org/10.1016/j.lanepe.2021.100047 5. Soley Bori, M., Ashworth, M., Bisquera, A., Dodhia, H., Lynch, R., Wang, Y., & Fox- Rushby, J. (2021). Impact of multimorbidity on health care costs and utilisation: a systematic review of the UK literature. British Journal General Practice, 71(702), e39- e46. https://doi.org/10.3399/bjgp20X713897 Daily Mail: One in five people in south London live with multiple long-term conditions Any questions?