HS300 Lecture Confounding PDF
Document Details
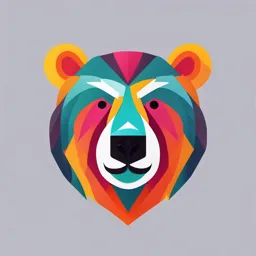
Uploaded by ReplaceableRainbow
Eastern Iowa Community Colleges
Tags
Summary
This document is a lecture on confounding in epidemiology. It discusses the concepts of confounding, bias, and random error in the context of public health studies.
Full Transcript
Confounding SAR HS300 Epidemiology How valid are the study findings? SAR HS300 Epidemiology Epidemiologists assess whether a study result is valid by assessing for 1. Random error 2. Bias 3. Confounding These three phenomena are alternate explanations for the observed association between...
Confounding SAR HS300 Epidemiology How valid are the study findings? SAR HS300 Epidemiology Epidemiologists assess whether a study result is valid by assessing for 1. Random error 2. Bias 3. Confounding These three phenomena are alternate explanations for the observed association between exposure and disease Threaten validity of the study SAR HS300 Epidemiology Systematic Error Key distinction from random error: this type of error is introduced by the investigative study process. 2 Types: 1. Selection Bias 2. Information Bias A more serious problem to study validity.. and now consider Confounding SAR HS300 Epidemiology Confounding A mixing of effects between the exposure, the outcome and a third extraneous variable known as a confounder. SAR HS300 Epidemiology Confounding A confounding variable is independently associated with both the risk factor (exposure) and the disease (outcome). Because of the dual association, the confounding variable creates a false association between the risk factor and disease or can exaggerate or hide a true association. SAR HS300 Epidemiology Criteria of Confounders To be a confounder, an extraneous factor must satisfy all 3 criteria: 1) Be a risk factor or marker for the disease. 2) Be associated with the exposure. 3) Not be an intermediate step in the causal path between exposure and disease. SAR HS300 Epidemiology C ? E D Confounder (C), Exposure (E), D (disease or outcome) SAR HS300 Epidemiology Coal Miners and Chronic Obstructive Pulmonary Disease (COPD) A study conducted in West Virginia reports that coal miners experience chronic obstructive pulmonary disease at 20 times the rate of non coal miners. Study was adjusted for age and income. SAR HS300 Epidemiology Coal Miners and Chronic Obstructive Pulmonary Disease (COPD) ?Confounder Coal Miners COPD SAR HS300 Epidemiology Coal Miners and Chronic Obstructive Pulmonary Disease (COPD) Reviewers consider factors other than mine exposure that may explain this very high reported difference between coal miners and non coal miners. Could smoking be a confounder? 3 questions: 1) Is smoking a risk factor for COPD? 2) Is smoking associated with coal mining? 3) Is smoking an intermediate step in pathway between mining and COPD? SAR HS300 Epidemiology Coal Miners and Chronic Obstructive Pulmonary Disease (COPD) Further investigation reveals that smoking among miners is 4 times the rate among non miners Smoking is independently associated with coal mining SAR HS300 Epidemiology Coal Miners and Chronic Obstructive Pulmonary Disease (COPD) Smoking Coal Miners COPD SAR HS300 Epidemiology Coal Miners and Chronic Obstructive Pulmonary Disease (COPD) Smoking is a confounder. Did smoking exaggerate ( a positive confounder) or weaken ( a negative confounder)the reported association between coal mining and COPD? SAR HS300 Epidemiology Variables Dependent Variable: an outcome (disease) we seek to explain or account for by the influence of the independent variable(s) Independent Variable: a risk factor or exposure SAR HS300 Epidemiology Confounding The distortion of an association between an exposure and an outcome because of the influence of a third variable that was not considered in the study design nor initial analysis. SAR HS300 Epidemiology The Ideal When you design a study, attempt to have exposed and unexposed groups comparable in every way – except the exposure under study…. SAR HS300 Epidemiology..with confounding, a risk factor apart from the exposure under study is distributed differently between the exposed and unexposed groups SAR HS300 Epidemiology Impact When uncontrolled the effects of a confounding variable cannot be distinguished from the study exposure SAR HS300 Epidemiology Confounding reflects the fact that “epidemiologic studies are conducted among individuals with unevenly distributed characteristics.” SAR HS300 Epidemiology Confounding Example ………………. SAR HS300 Epidemiology Hypothetical study Investigators enrolled group of newly diagnosed diabetic adults (exposed group) & a group of adults without diabetes (unexposed group), followed for 10 years to determine the cumulative incidence of dementia. Risk of dementia among adults with diabetes Aschengrau A, Seage GR, Essentials of Epidemiology in Public Health, Jones and Bartlett Publ 2003 p.282 SAR HS300 Epidemiology Study Dementia Yes No Total Diabetes Yes 380 620 1,000 No 110 890 1,000 Total 490 1,510 2,000 SAR HS300 Epidemiology Measure of Association Relative Risk = a/a+b c/c+d Incidence rate in exposed Incidence rate in non exposed 380 Divided by 110 1000 1000 = 0.38/0.11 = 3.45 SAR HS300 Epidemiology Measure of association Risk of developing dementia was 3.5 times greater among adults with a diagnosis diabetes compared with adults without a diagnosis of diabetes Other risk factors?... …possible confounders.. SAR HS300 Epidemiology Possible confounders Age Subjects with diabetes were, on average, older than those without diabetes When confounding for age was controlled RR=2.0 Initial result RR = 3.5 was exaggerated by confounding by age SAR HS300 Epidemiology Possible confounders Age How to determine whether age is a confounder in this study? SAR HS300 Epidemiology Decision Tree to Determine if a Variable is a Confounder Aschengrau A, Seage GR, Essentials of Epidemiology in Public Health Evaluate Association between confounder and disease Association is present Association is absent Confounding NO CONFOUNDING POSSIBLE Evaluate Association between confounder and exposure Association is present Confounding PRESENT Association Absent NO Confounding SAR HS300 Epidemiology Step 1 Confounder and Disease Is there an independent association between age (possible confounder) and dementia (outcome)? SAR HS300 Epidemiology Assoc. Between Age & Dementia among exposed (with diabetes) Age Dementia Dementia Yes No Total 80 – 99 360 540 900 years 45 – 79 20 80 100 years Total 380 620 1000 RR = 2.0 SAR HS300 Epidemiology Assoc. Between Age & Dementia among participants without Diabetes Age Dementia Dementia Yes No Total 80 – 99 20 80 100 years 45 – 79 90 810 900 years Total 110 890 1000 RR = 2.0 SAR HS300 Epidemiology Step 1 Result: Confounder and Disease Yes! Age (potential confounder) is associated with Dementia (disease/outcome) SAR HS300 Epidemiology Step 2 Confounder and Exposure Is there an independent association between: age (confounder) & diabetes (exposure)? SAR HS300 Epidemiology Association Between Age and Diabetes (hypothetical) Age Diabetes Diabetes Yes No Total 80 – 99 900 100 1000 years 45 – 79 100 900 1000 years Total 1000 1000 2000 RR = 9.0 SAR HS300 Epidemiology Step 2 Result: Confounder and Exposure Yes! Age (potential confounder) is associated with Diabetes (exposure) SAR HS300 Epidemiology Confounding Age Subjects with diabetes were, on average, older than those without diabetes When confounding for age was controlled RR=2.0 Initial result RR = 3.5 was exaggerated by confounding by age SAR HS300 Epidemiology Magnitude of Confounding = Crude RR - Adjusted RR Adjusted RR = (3.5) - (2.0) (2.0) = 75% Large amount of confounding SAR HS300 Epidemiology Decision Tree to Determine if a Variable is a Confounder Aschengrau A, Seage GR, Essentials of Epidemiology in Public Health Evaluate Association between confounder and disease Association is present Association is absent Confounding NO CONFOUNDING POSSIBLE Evaluate Association between confounder and exposure Association is present Confounding PRESENT Association Absent NO Confounding SAR HS300 Epidemiology Of all studies, ecological studies are most susceptible to confounding More difficult to control for confounders at the aggregate level of data Example: fat consumption and breast Ca. (Age, body weight, hormone therapy) SAR HS300 Epidemiology Criteria of Confounders To be a confounder, an extraneous factor must satisfy the following criteria: 1) Be a risk factor or marker for the disease. 2) Be associated with the exposure. 3) Not be an intermediate step in the causal path between exposure and disease. SAR HS300 Epidemiology Example Study in city of Los Angeles reports air pollution is associated with bronchitis SAR HS300 Epidemiology Possible confounders ??? …Urban crowding..high population density.. SAR HS300 Epidemiology Example 2 Study reports modest alcohol consumption lowers risk of heart disease Study reports that alcohol increases HDL, which decrease risk of heart disease Is HDL a confounder? How does alcohol exert its effect on heart disease? SAR HS300 Epidemiology Intermediate step in causal pathway Intermediate step: examine biological pathway by which the exposure is thought to impact the disease Mod. Alcohol consumption reduces risk of CVD by increasing the HDL level Mod alcohol----→HDL-----→risk CVD HDL is an intermediate step -> NOT a confounder SAR HS300 Epidemiology Steps in Assessing Confounding Confounding is a quantitative issue 1) Is confounding present? 2) What is the magnitude ? 3) What is the direction? SAR HS300 Epidemiology Assessing Confounding Direction of confounding: Exaggerate true association = POSITIVE Hide a true association = NEGATIVE SAR HS300 Epidemiology “Appreciable” Difference. Arbitrary Commonly > 10% - 20% difference is considered appreciable SAR HS300 Epidemiology Confounding Of the categories of systematic error: selection bias, information bias and confounding bias, ……….. …..only confounding may be controlled for in the data analysis!!! SAR HS300 Epidemiology Methods to Control Confounding Prevention strategies Data Analysis strategies SAR HS300 Epidemiology Methods to Control for Confounding in Design and Analysis Design Stage Analysis Stage ❑Randomization ❑Standardization ❑Restriction ❑Stratified analysis ❑Matching ❑Multivariate analysis SAR HS300 Epidemiology Methods to Control Confounding Prevention Strategies Prevention strategies--attempt to control confounding through the study design itself. Three types of prevention strategies: 1. Randomization 2. Restriction 3. Matching SAR HS300 Epidemiology Identifying potential confounders in study design phase Literature review To ascertain all known risk factors for the disease under study SAR HS300 Epidemiology Example Potential confounders in study of chemical contamination of drinking water and risk of breast cancer. What would you like to determine from the literature review? Known risk factors for breast cancer:… family history of breast ca, race, age at first delivery, hx of radiation rx… SAR HS300 Epidemiology Randomization Attempts to ensure equal distributions of the confounding variable in each exposure category …………….i.e. in each arm of an experimental design SAR HS300 Epidemiology Study of Treatment for Atherosclerosis Clopidogrel group Aspirin Group Characteristic (n=9599) (n=9586) Male (%) 72 72 White (%) 95 95 Current cigarette smoker (%) 29 30 Patients with a history of: Hypertension (%) 52 51 Stable angina (%) 22 22 High cholesterol levels (%) 41 41 SAR HS300 Epidemiology Maternal-Infant HIV Transmission Trail Placebo Zidovudine Group Characteristic group (n=239) (n=238) Median Age at entry (years) 25 25 White (%) 68 32 Gestational age at entry Median (weeks) 26 27 14-26 weeks (%) 52 50 >26 weeks (%) 48 50 Mean CD4 count at entry 560 438 SAR HS300 Epidemiology Randomization Advantages: Convenient, inexpensive; permits straightforward data analysis. Controls for known and unknown confounders Disadvantages: Need large sample sizes. Can only be used in experimental designs i.e. clinical or community trials (not in observational studies) SAR HS300 Epidemiology Restriction Study admission criteria are limited Entrance into the study is confined to individuals who fall within a specified category of the confounder e.g. age, gender, race For example, restricting participants to a narrow age category can eliminate age as a confounder. SAR HS300 Epidemiology Restriction Advantages: 1) Effective, simple, convenient, inexpensive 2) Provides complete control of known confounder Disadvantages 1) Unlike randomization, cannot control for unknown confounders. 2) Difficult to recruit enough subjects. 3) Limits generalizability of study findings SAR HS300 Epidemiology Matching Pair Matching Matches subjects in the study groups according to the value of the suspected or known confounding variable to ensure equal distributions e.g. by 5 or 10 year age strata SAR HS300 Epidemiology Example Cohort study on exercise and risk of colon cancer 1. Lit review: potential confounders: age, gender, obesity 2. Exposed subject (exerciser) enrolls, 55 yo male, normal BMI 3. Unexposed subject (non exerciser), 55 yo, male, normal BMI SAR HS300 Epidemiology Matching (cont’d) Advantages: 1) Fewer subjects are required than in unmatched studies of the same hypothesis. 2) Useful in small case control studies 3) When confounder is a complex nominal variable – occupation, neighborhood (associated with complex web of environmental variables) SAR HS300 Epidemiology Matching Disadvantages: 1) Costly because extensive searching and recordkeeping are required to find matches. SAR HS300 Epidemiology Matching Disadvantages 2) When one matches subjects on a potential confounder that particular exposure variable can no longer be evaluated with respect to its contribution to risk. (Not possible to study the relationship between the matching factor and outcome in case control) SAR HS300 Epidemiology Analysis Strategies to Control Confounding 1. Standardization 2. Stratification 3. Multivariate techniques- SAR HS300 Epidemiology Standardization Stratification used commonly for demographic variables e.g. age, race, gender SAR HS300 Epidemiology Analysis Strategies to Control Confounding 1. Stratification—the result of separating a sample into several subsamples according to specified criteria such as age groups, SES … analyses performed within each stratum. SAR HS300 Epidemiology Example Case control study of DDE (metabolite by- product of pesticide, DDT) exposure and breast cancer (hypothetical) Crude OR (without age stratification): 1.9 SAR HS300 Epidemiology Crude Data from a Case-Control Study of DDE Exposure and Breast Cancer DDE Level Cases Controls High 500 600 Low 1500 3400 Total 2000 4000 Odds Ratio = 1.9 SAR HS300 Epidemiology Age-Stratified Data from Case-Control Study of DDE Exposure and Breast Cancer Age less than 50 years Age 50 years and older DDE Levels Cases Controls Cases Controls High 50 300 450 300 Low 450 2700 1050 700 Total 500 3000 1500 1000 Stratum-specific odds ratio = 1.0 Stratum-specific odds ratio = 1.0 SAR HS300 Epidemiology Interpretation of Age Stratified Data from Study of DDE Exposure and Breast Cancer 1. Note that total numbers in cells in 2 stratified tables add up to total number in the crude data table 2. When stratified, no association between breast cancer and DDE among women in either stratum: < 50 and women 50 years and older. OR = 1.0 for each age category SAR HS300 Epidemiology Interpretation of Age Stratified Data from Study of DDE Exposure and Breast Cancer The appreciable difference between the crude odds ration (O.R=1.9) and stratum specific odds ratios (O.R=1.0) indicates that.. …confounding by age is present SAR HS300 Epidemiology Advantages of Stratification 1. Performing analyses within strata is a direct and logical strategy. 2. The computational procedure is straightforward. 3. Allows epidemiologists to view the raw data SAR HS300 Epidemiology Disadvantages of Stratification 1. Small numbers of observations in some strata. (example – next slide) 2. Difficulty in interpretation when several confounding factors must be evaluated. SAR HS300 Epidemiology Cannot control for many variables simultaneously Control for four variables simultaneously: Gender (2 categories – male, female) Age (5 categories 70) Race/ethnicity (3 categories: white, black, hispanic) Smoking: (3 categories: current, past and never smoker) How many strata (cells)? SAR HS300 Epidemiology How many strata (cells)? 2 X 5 X 3 X3 = 90 Even with large study, the number of subjects in some cells would be very small or zero, making analysis less valuable SAR HS300 Epidemiology Analysis Strategies to Control Confounding 3. Multivariate techniques- When need to control for many confounding variables simultaneously SAR HS300 Epidemiology Multivariate techniques … construct mathematical models that describe simultaneously the influence of exposure and other factors that may be confounding the effect. SAR HS300 Epidemiology Multivariate techniques Multivariate analysis should be performed only after conducting a stratified analysis Stratified Analyses -> Multivariate analysis Any variable that changes the crude measure of association by an appreciable amount in the stratified analysis should be retained in the multivariate analysis SAR HS300 Epidemiology Multivariate Techniques Advantages: 1. Allow for simultaneous control of several exposure variables in a single analysis. Disadvantages: 1. Potential for misuse. SAR HS300 Epidemiology Residual Confounding Confounding that remains even after many confounding variables have been controlled 1. Confounder for which data was not collected. 2. Differences in risk within a category of the confounder. (e.g. too broad an age group) SAR HS300 Epidemiology To Evaluate Study Validity Assess for the presence of: 1. Random error 2. Bias 3. Confounding Alternate explanations for the observed association between an exposure and disease SAR HS300 Epidemiology Random Error “A random error leads to a false association between exposure and disease that arises from “chance”, a uncontrollable force that seems to have no assignable cause”. Unlike bias and confounding, random errors are unsystematic, because they arise from an unpredictable, non-discernable process SAR HS300 Epidemiology Random Error Poor Precision. Sampling Error Variability in Measurement Reduce random error by increasing the sample size (& number of measurements) SAR HS300 Epidemiology Systematic Error Systematic error is introduced by the investigative study process, as distinct from random error May be due to: Bias (by the investigator) - Selection Bias - Information Bias SAR HS300 Epidemiology Systematic Error Systematic error is …..a more serious problem to study validity than random error. SAR HS300 Epidemiology Selection Bias Relation between exposure and disease is different for those who participate in or complete the study and those, who theoretically would be eligible for the study but, who do not participate. Or dropped out. Examples: self selection – who responds to health surveys (? smokers, obese). Drop outs Remember: drop outs only refer to prospective studies (cohort and experimental) SAR HS300 Epidemiology Selection Bias 1. Control selection bias 2. Self selection bias 3. Differential surveillance 4. Selective survival/Loss to follow up SAR HS300 Epidemiology Information/Observation Bias Information bias may be introduced as a result of measurement error in assessment of both exposure and disease. SAR HS300 Epidemiology Types of Information Bias - Recall bias: better recall among cases than among controls. Example: Family recall bias. Interviewer/abstractor bias--occurs when interviewers probe more thoroughly for an exposure in a case than in a control. Prevarication (lying) bias--occurs when participants have ulterior motives for answering a question and thus may underestimate or exaggerate an exposure. SAR HS300 Epidemiology Publication Bias Occurs because of the influence of study results on the chance of publication. Studies with positive results are more likely to be published than studies with negative results. SAR HS300 Epidemiology VERY IMPORTANT Selection and Information bias ❑ Cannot be corrected in the analysis phase of the study Must be avoided in the design and conduct of the study Confounding may be controlled for in the analysis HS 300 Epidemiology Question #1 A study of the relationship between contact lens use and the risk of eye ulcers. The crude relative risk is 3.0 and the age-adjusted relative risk is 1.5. Is age a confounder in this study? Why? Justify your answer. SAR HS300 Epidemiology Question #2 A case control study of the relationship between cigarette smoking and pancreatic cancer. In this study, coffee drinking is associated with smoking and is a risk factor for pancreatic cancer among both smokers and non-smokers. Is coffee drinking a potential confounder in this study? Why? Justify your answer. SAR HS300 Epidemiology Question #3 A study of the relationship between exercise and heart attacks that is conducted among men who do not smoke. Is gender a confounder in this study? Why? Justify your answer. SAR HS300 Epidemiology Question #4 A cohort study of the risk of liver cirrhosis among female alcoholics. Incidence rates of cirrhosis among alcoholics are compared to those among non –alcoholic women. Non-alcoholics are individually matched to alcoholics on month and year of birth. Is age a confounder in this study? Why? Justify your answer. SAR HS300 Epidemiology SAR HS300 Epidemiology