Basic Statistics in Testing PDF
Document Details
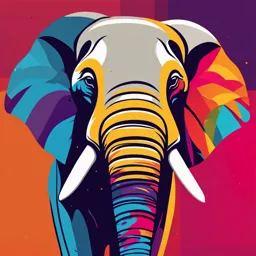
Uploaded by FantasticMermaid
Farhangian University
Tags
Summary
This document provides a general introduction to basic statistics, geared for language teaching and testing. It covers descriptive statistics and discusses the role of these statistics in daily life, with examples of their application, and the concept of tabulation of data. It aims to get students interested in statistics.
Full Transcript
4 Basic Statistics in Testing 4.1 Introduction In recent years more and more colleges are offering statistics courses at an elementary level. This Chapter is designed to serve as a general introduction to the statistics needed by students in the area of language teaching in...
4 Basic Statistics in Testing 4.1 Introduction In recent years more and more colleges are offering statistics courses at an elementary level. This Chapter is designed to serve as a general introduction to the statistics needed by students in the area of language teaching in general, and testing in particular. Students in this field must somehow demonstrate a knowledge of the language and methods of statistics. Accordingly, exercises and examples are chosen to interest such students. Most of us think of statistics as having something to do with charts or Tables of numbers. While this idea is not wrong, mathematicians and statisticians use the word statistics in a more general sense. Roughly speaking, the term statistics, as used by statisticians, involves collecting numerical information called data, analysing them, and making meaningful decisions on the basis of the outcome of the analyses. The role played by statistics in our daily activities is constantly increasing. As a matter of fact, a nineteenth century scientist, H.G. Wells, predicted that “statistical thinking will one day be as necessary for efficient citizenship as the ability to read and write.” The following examples on the uses of statistics indicate that a knowledge of statistics today is quickly becoming an improtant tool for any educated person. 1. The latest Central Bank statistics shows that the cost of living in Tokyo rose by 8% in one year. 2. Statistics shows that the use of drugs by pregnant women can be dangerous to the unborn child. 3. Statistics collected by insurance companies shows that today people on 37 the average are living longer than did their parents. 4. Statistics shows that if the air we breathe is excessively polluted, then undoubtedly some people will become ill. 5. Statistics shows that the world’s population is growing at a faster rate than the availability of food. 6. Statistics shows that any student, regardless of his high school background, will suceed in college if properly motivated. Very little formal mathematical knowledge is needed to collect and tabulate data. However, the interpretation of data in a meaningful way requires careful analysis. If not done by a statistician or mathematician who is trained to interpret data, statistics can be misused. The following example indicates how statistics can be misused. In the city of ‘x’ the occurrence of polio, thought to be nonexistent, increased by 100% from the year 1364 to 1365. Such a statistic would horrify any parent. However, upon careful analysis it was found that in 1364 there were 2 reported cases of polio out of a population of 1,000,000 and in 1365 there were four cases. 4.2 Choice of Actions Suggested by Statistical Studies The word statistics has different applications. Mathematicians have divided the field of statistics into two major areas called descriptive statistics and inferential statistics. The statistics used to summarize data are called descriptive statistics (Hatch and Farhady, 1982). In descriptive statistics we describe sample data. Each characteristic of the sample is called a statistic. From the statistic, i.e., the characteristic of a given sample, we can make inferences about the characteristic of a given population, called a parameter. This is possible through the utilization of the methods of inferential statistics. Inferential statistics is much more complicated than descriptive statistics. To understand what is involved, imagine that we are interested in the average oral language ability of students at a particular university. Since there are so many students attending the university, it would require an enormous amount 38 of work to interview each student and collect all the data. Furthermore, the procedure would undoubtedly be costly and take too much time. Possibly we can obtain the necessary information from a sample of sufficient size that would be appropriate for our needs. We could then use the data based upon this sample to make predictions about the entire student body, called the population. This is what statistical inference involves. 4.3 Tabulation of Data Before quantitative data can be understood and interpreted, it is usually necessary to summarize them. Table 4.1 shows a class record for a reading test administered at the beginning of the school year. The scores appear in the order they are recorded in the teacher’s class roll book. However, the scores do not mean very much in this form, and we can tell only with some difficulty whether, for example, the first-listed student, with a score of 90 points out of a possible 128, is superior or just average in reading readiness, compared with his classmates. Table 4.1 A Class Record for a Reading Test Student Score Student Score Student Score Student Score 1. 90 11. 59 21. 75 31. 81 2. 66 12. 95 22. 75 32. 71 3. 106 13. 78 23. 51 33. 68 4. 84 14. 70 24. 109 34. 112 5. 105 15. 47 25. 89 35. 62 6. 83 16. 95 26. 58 36. 91 7. 104 17. 100 27. 59 37. 93 8. 82 18. 69 28. 72 38. 84 9. 97 19. 44 29. 74 10. 97 20. 80 30. 75 39 4.3.1 Rank Order Ordinarily, the first step is to arrange the scores in the order of size, usually from highest to lowest. In a small class, this is often all that is necessary. Table 4.2 shows 38 scores arranged in the order of size from 112 to 44. This Table also shows the rank order of the pupils (1st, 2nd,... , 38th) and the scores tabulated without fruther grouping. It is now easy to see that a score of 90 gives a student a rank of 13 in a class of 38, or about one-third of the way from the top. Similarly, it is easy to interpret each of the other scores in terms of rank. But ties are likely to occur, especially in classes of 20 or more pupils. Notice, for example, that two pupils received a score of 97. Since it is not correct to say that one ranks higher than the other, we must assign them the same rank. Since there are six pupils who rank higher (1,2,3,4,5,6), the next two ranks, 7 and 8, are averaged, giving 7.5. In like manner the average of ranks 9 and 10 is 9.5, and so on for the other pupils with tied scores. There are three pupils with scores of 75, and there are 21 pupils who rank above this score; the average of the next three ranks (22, 23, and 24) is 23, which is the rank assigned to each of the scores of 75. In addition to the time and trouble required to determine these ranks, the list is long and inadequate for making comparisons with other classes that are much larger or much smaller; ranking 19th in a class of 38 pupils is poorer than ranking 19th in an equally capable class of 70 pupils. In order to alleviate these problems, frequency distributions are constructed. 4.3.2 The Frequency Distribution The list of scores can be made shorter by arranging the scores in a frequency distribution, sometimes simply called a distribution. The third and fourth columns of Table 4.2 show the simplest form of a distribution. The various scores are arranged in the order of size, here from 112 to 44, and to the right of each score is recorded the number of times it occurs. Each entry to the right of a score is called a frequency, abbreviated as f, and the total of the frequencies is represented by N. 40 Table 4.2 Rank Ordered Reading Scores from Table 4.1 Score Rank Score Frequency (f) 112 1 112 1 109 2 109 1 106 3 106 1 105 4 105 1 104 5 104 1 100 6 100 1 97 7.5 1 97 2 97 7.5 95 2 Sum=19 95 9.5 93 95 9-5 j 91 1 93 11 90 1 91 12 89 1 90 13 84 2 89 14 83 1 84 15.5 '1 82 1 84 15.5 J1 81 1 83 17 frequencies 82 18 80 81 19 78 1 80 20 75 3 78 21 74 1 75 23 72 1 75 23 71 1 75 23 70 1 74 25 69 1 Sum= 19 72 26 68 71 27 66 1 70 28 62 1 69 29 59 2 68 30 58 1 66 31 51 1 62 32 47 1 59 33.5 ] 44 1 59 33.5 J 58 35 N= 19+19=38 51 36 47 37 44 38 41 4.3.3 Determining Percentiles It is often important to determine the position of a score in a distribution. For example, imagine that the scores are numerical grades of students in a class and we wish to report how a given student is doing. The report might simply say that “Reza got the third highest mark in the class.” or “Amir got next to check the lowest mark.” What is learnt from this report is that the number of students on one side of each grade is known. However, the information given above does not enable one to determine how successful Reza or Amir was. One might assume that there have been three students or a hundred. In order to determine how well Reza or Amir did in their group, the total number of students must be known. Therefore, the status of a score should not simply be announced by the number of scores above or below it. The number of scores in the entire distribution (group) would also have to be made known. We shall now consider how to locate the position of a score in a distribution by assigning it the value called a percentile rank. But before we proceed any further, two other concepts should be clarified. They are: a) relative frequency b) cumulative frequency 4.3.3.1 Relative Frequency Relative frequency refers to the frequency of each score divided by the total number of scores. Suppose in an English class the number of students is 60 (N=60). The scores (out of a hundred) reported by the instructor are as presented in Table 4.3. Since we are dealing with students, we cannot say that 0.08 of the students passed the test or received a particular score. Therefore, the relative frequencies are usually multiplied by 100 and the result is called percentage. Then, it is said that 8 percent of the subjects passed the test or received a particular score. 42 Table 4.3 Relative Frequency Relative Frequency Percentage Score Frequency Compute Result 80 5 S’s 54-60 0.08 0.08X 100=8 70 15 154-60 0.25 0.25X 100=25 60 20 204-60 0.34 0.34X 100=34 50 15 154-60 0.25 0.25X 100=25 40 5 54-60 0.08 0.08X 100=8 4.3.3.2 Cumulative Frequency Cumulative frequency indicates the standing of any particular score in a group of scores. Cumulative frequency shows how many scores fall below the given score or point in a distribution. This is also used for the computation of percentile scores. Table 4.4 shows the scores of 125 studendts on a 40-item English placement test. Table 4.4 English Placement Test Scores Test Cumulative Frequency Percentile Score Frequency (0 (F) 38 1 125 (100) ^1 = 100 v ’ 125 37 1 124 36 3 123 35 5 120 34 9 115 33 8 106 32 17 98 31 23 81 (W0) -^-=64.8 or 65% 30 24 58 29 18 34 28 10 16 27 3 6 26 1 3 25 0 2 24 2 2 N=125 43 As shown in this Table, in the third column the sums are obtained when we add the frequency of successive intervals in the previous work. The column is constructed from the bottom up. There are 2 scores in the bottom interval. And there is zero score in the next to the bottom interval. Therefore to compute the cumulative frequency 2 is added to zero and so on. In the Table, simple, or absolute frequency is symbolized by the lower case letter f and cumulative frequency by the capital letter F. In order to compute the percentile rank of any level or point, the corresponding F should be divided by the total number of scores or students (i.e., N). The result is then multiplied by 100. For example, in the above Table the frequency for the students who scored 31 is 23. The corresponding cumulative frequency is 81. The Total number of scores or students (N) is 125. Therefore, the percentile rank (P) for this level is: P= 100 ^- = 100-^-=64.8 or 65% (rounded off) Thus percentile rank means that 65% of the students who took the test scored at or lower than the level in question. An improtant point to be remembered is that if two students in two different classes but at the same level both got the same score, their standing (percentile rank) may differ in their respective groups. The following example will clarify the point. Example Ali and Reza are twins, but are in different classes. Recently they both got 80 on a math test. The grades of the other students in their classes were: Ali’s class: 64, 67, 73, 73, 74, 77, 77, 78, 78, 79, 80, 80, 80, 82, 91, 94, 100 Reza’s class: 43, 65, 68, 73, 75, 76, 76, 77, 79, 80, 80, 80, 80, 85, 86, 87, 88, 90, 92, 96 Ali’s percentile rank: fyy x 100=76% [F(80)]=13 Reza’s percentile rank: X 100=65% [F(80)]=13 The above example reveals that the percentile rank of an individual score is often more helpful than the particular score itself. Although both Ali and 44 Reza had grades of 80, Ali’s percentile rank is considerably higher. If we assume that the levels of competition are equivalent in both classes, this may indicate that Ali’s performance is superior to Reza’s performance when compared to the rest of their respective classes. To conclude, information regarding the tabulation of data is summarized in Table 4.5 for quick reference. Table 4.5 Tabulation of Sample Data Frequency Relative Cumulative Score Percentage Percentile (0 Frequency Frequency (F) 80 5 0.08 8 60 100 70 15 0.25 25 55 92 60 20 0.34 34 40 67 50 15 0.25 25 20 33 40 5 0.08 8 5 8 4.4 Graphic Representation of Data There can be little doubt that the graphic representation of data is a valuable supplement to summarizing the data and statistical analysis. A graph or a chart tends to attract the reader’s attention. The average casual reader is likely to give scant attention to the ordinary printed matter in a research report and to be unimpressed by the mass of tabular data often piled up at the end. However, his eye is likely to be arrested by any picture or chart that may happen to be included, and this may lead him to read the entire discussion. A graph is often an effective method of clarifying a point. One small graph will often present a topic more clearly than a combination of paragraphs will. It is sometimes said that the pictures speak for themselves. In reality, statistics often stand speechless and silent, Tables are sometimes tongue-tied, and only the graph cries aloud its message. Ordinary numerical data are quite abstract; they convey their meaning vaguely and with effort to the average mind. The picture or graph is a more concrete representation of the data. 45 Spear’s description of the function of graphs is a fitting conclusion to this introducation: In the present day, when visual education in all aspects has become not only an aid to, but also a vital basis of learning, our attention is called more than ever before to the almost limitless possibilities in this field. The eye absorbs written statistics, but only slowly does the brain receive the message hidden behind written words and numbers. The correct graph, however, reveals that message briefly and simply. Its purposes, which follow, are clear from its context: 1. better comprehension of data than is possible with textual matter alone, 2. more penetrating analysis of subject than is possible in written text, 3. a check of accuracy. This triple purpose of the graph can be carried out through careful planning and familiarity with the functions of all types of graphs and media. The following six steps are fundamental to the development of a graphic presentation that will describe statistical data with clarity: 1. Determine the significant message in the data. 2. Be familiar with all types of charts and make the correct selection. 3. Meet the audience on its own level; know and use all appropriate visual aids. 4. Give detailed and intelligible instructions to the drafting room. 5. Know the equipment and skills of the drafting room. 6. Recognize effective results. 4.4.1 Drawing a Frequency Distribution The ordinary frequency distribution may not give a very clear picture of the data. There are three common methods of representing a distribution of scores graphically: the bar graph, the histogram, and the frequency polygon. 4.4.1.1 The Bar Graph The bar graph is commonly used to graphically describe data. In bar graphs 46 vertical bars are used. The height of each bar represents the number of members; that is, the frequency of that class. To construct such graphs the following steps are followed. 1. First draw two axes (a horizontal line and a vertical one). 2. Enter the scores of the students on the horizontal axis and the frequency of each score on the vertical one. 3. Draw the bars around these frequency points. Example The following data are the results of a group of students on a place ment test. 10, 12, 12, 12, 13, 13, 13, 13, 14, 14, 16, 16 The data are represented in Figure 4.1 as a simple frequency distribution in the form of a bar graph Scores (x) f 16 2 14 2 13 4 12 3 10 1 4 3 10 H 12 13 14 15 16 Scores Figure 4.1 A Bar Graph Representing the Frequency of Scores 47 4.4.1.2 The Histogram The histogram is a series of columns, each having as its base one class interval and as its height the number of cases, or frequency, in that class. Figure 4.2 represents a histogram showing the distribution of scores obtained from administering an English vocabulary test to 48 students. The data are as follows: Class interval f 28 - 32 1 33 - 37 2 38 - 42 4 43 - 47 5 48 - 52 6 53 - 57 7 58 - 62 8 63 - 67 9 68 - 72 4 73 - 77 1 78 - 82 1 Since the greatest frequency is 9, in the 59.5 - 64.5 class, it is not necessary to extend the vertical or frequency scale at the left above 9. And since the scores range from the 29.5 - 54.5 class to the 74.5 - 79.5 class, it is necessary to represent the horizontal scale only through that distance. For clarity, however, it is customary to extend the scale one class interval above and below that range. In order to avoid having the Figure be too flat or too steep, it is recommended to arrange the scales so that the width of the histogram itself is about one and two-thirds times its height-that is, the ratio of height to width should be approximately 3:5. 48 Figure 4.2 A Histogram, Representing the Scores on an English Vocabulary Test 4.4.1.3 The Frequency Polygon The process of constructing a frequency polygon is very much like that of constructing the histogram. In the histogram, the top of each column is indicated by a horizontal line, the length of one class interval, placed at the Percentages assigned Figure 4.3 A Frequency Polygon Representing the Scores on an English Vocabulary Test 49 proper height to represent the frequency in that class. But in the polygon a point is located above the midpoint of each class interval and at the proper height to represent the frequency in that class. These points are then joined by straight lines. As the frequency is zero at the classes above and below those in the distribution, the polygon is completed by connecting the points that represent the highest and the lowest classes with the base line at the midpoints of the class intervals next above and below. Figure 4.3 shows a polygon for the same data represented by a histogram in Figure 4.2. 4.5 Descriptive Statistics The previous section dealt with how the properties of a collection of scores can be depicted graphically or in a tabular form. Frequently a graph or a Table of data tells us more than we want or need to know, but the message it conveys may be time-consuming to communicate. Usually, for description just two or three properties of a set of scores are singled out. These properties (e.g., the typical “size” of scores and their spread) may be describable by indexes known as summary statistics. In the following two sections, summary statistics which describes the typical “size” of a set of scores and their spread will be discussed. 4.5.1 Measrues of Central Tendency As a student, you may wish to know not only how you performed on an examination, but also how well, in general, the other students performed. When we teach, of course, our students may want the same information. The measures of central tendency-the mode, the median, and the mean all present this type of information. 4.5.1.1 The Mode The most easily obtained measure of central tendency is the mode. The mode is the score that occurs most frequently in a set of scores. Not every set of scores has a single mode by a strict interpretation of this definition. 50 Notes 1. When all of the scores in a group occur with the same frequency, it is customary to say that the group of scores has “no mode”. Thus there is no mode in the group of scores of (1,1,4,4,7,7). 2. When two adjacent scores have the same frequency and this common frequency is greater than that for any other score, the mode is the average of the two adjacent scores. Thus the mode of the group of scores of (0,1,1,2,2,2,3,3,3,4) is 2.5. 4.5.1.2 The Median The median (Md) is the score at the 50th percentile in a group of scores. It is the score that divides the ranked scores into halves, such that half of the scores are larger than the median, and the other half of the scores are smaller. Notes 1. If the data include an odd number of united scores, e.g., 11,13,18,19,20, the median is the middle score when they are ranked, Md = 18 2. If the data include an even number of united scores, e.g. 4,9,13,14, the median is the point halfway between the central values when the scores are ranked: Md = (9+ 13)/2 = 11 4.5.1.3 The Mean The mean of a distribution is commonly understood as the arithmetic average. The term grade point average, familiar to students, is a mean value. It is computed by dividing the sum of all the scores by the number of scores. It is represented through the following formula: Y - 2X N where: X = mean S = sum of X = any individual score in a distribution N = number of scores 51 Example x X,= 6 X2= 5 X3=4 X4= 3 X5= 2 x6=i XX= 21 N = 6 The mean has some interesting properties. If we subtract X from the score Xp the resulting difference is a deviation score (D)-it can be either negative or positive. If we were to find the deviation score for each of the N scores in the set, the sum of all “N” deviation scores would be exactly zero. This property is illustrated in Table 4.6. Table 4.6 Properties of the Mean Scores: (0,1,1,3,5,), N = 5, X = 2 Score Mean Deviation Score (D) 0 2 -2 1 2 -1 1 2 -1 3 2 1 5 2 3 SD = 0 Another property of the mean concerns the N deviation scores. The sum of the squared deviations of scores from their arithmetic mean is less than the sum of the squared deviations around any point other than X. For example, the sum of squared deviations of 0,1,1,3,5, around the mean is: 52 (0 - 2)2 = 4 (1 - 2)2 = 1 (1 - 2)2 = 1 (3 - 2)2 = 1 (5 - 2)2 = 9 2D2 = 16 The sum of the squared deviations of 0,1,1,3,5, around 1 is greater than 16, which is 21 in this case. (0 - l)2 = 1 (1 - l)2 = 0 (1 - l)2 = 0 (3 - l)2 = 4 (5 - l)2 = 16 2D2 = 21 4.5.2 Measures of Variability Although the mode , median, and mean are very useful in analyzing a set of data, there are some disadvantages in using them alone. These measures only locate the center of the distribution. In certain situations the location of the center may not be adequate to provide a logical picture of the data. We need some method of analyzing variation, that is, the difference among the terms of a distribution. In this section we will discuss some of the most commonly used methods for computing variation. These methods include range, variance and standard deviation. 4.5.2.1 Range The range of a set of numbers is the difference between the largest number in the distribution and the smallest number. Let us consider Hossein, who is interested in determining the best time to test vocabulary. During one week, he studied vocabulary and took the tests in the mornings, and during the second week, he studied vocabulary and took the tests at nights: The number of words he learned each day according to the tests are as follows: 53 Number of Words Learned Tests in the Mornings 15, 26, 30, 39, 45 Tests at Nights 29, 30, 31, 32, 33 In each case, the average number of words he learned was 31. But -when does he learn better? When he studied in the mornings, the number of words he learned varied from 15 to 45. So the range for the mornings is 45- 15 = 30. When he studied at night, the number of words he learned ranged from 29 to 33 words. So the range for the nights is 33 — 29 = 4. It can be understood that his vocabulary learning at nights was more equally distributed than that in the mornings. The range is by far the simplest measure of variation to calculate since only two numbers are needed. However, it is not commonly used because it does not tell us anything about how the other terms vary. Furthermore, if there is one extreme value in a distribution, the dispersion or the range will appear very large. If we remove the extreme term, the dispersion may become quite small. Because of this shortcoming, other measures of variation such as variance and standard deviation are used. 4.5.2.2 Variance and Standard Deviation To calculate the variance of a set of numbers, we first calculate the mean of the numbers. We then subtract the mean from each number, i.e., (X - X), and square the result. Finally, we compute the average of these squares. The result is called the variance of the numbers. If we now take the square root of the variance, we get the standard deviation of the numbers. The variance of a set of N numbers is the average of the squares of the differences of the numbers from the mean. If X represents the mean, then: Variance = S