Formulas PDF
Document Details
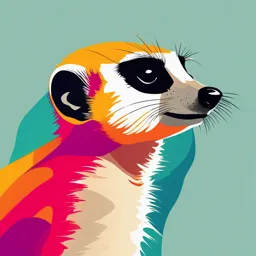
Uploaded by TroubleFreeAutomatism8196
Tags
Summary
This document discusses various financial formulas, statistical concepts, and financial data concepts, including different factor models like CAPM, 3FF, 5FF, 4FF, etc., and how to obtain stock market data from databases like CRSP. The document also covers moments in statistics, such as mean and variance.
Full Transcript
03/05/2024 - Meting 2 Batistics : -Descriptie statistics Hypothesis Testing - Regression - Databases : -...
03/05/2024 - Meting 2 Batistics : -Descriptie statistics Hypothesis Testing - Regression - Databases : - VBA : > - mention Chat GPT used VPN from Stolian Quiz 1 pasic : and article with mimber -go to get one see we find articles ↳ scolar Table 1. etc google - or B-on (if google scholar doesn't work) Check Email for : REFINITIV (LSEG) Relevant Patabase A - Sabi > - information on public + private comparis ↑ lefti CRSP stock market data (prices returns, marked op) - + E , ul U. Compustat > accounting data (invest longterm cebt) - - S. , Metrics Option > options - - - 1/B/E/S - analysts - S12 - mutal funds - TASS + hedge funds - WRDS Debt Loans Excel-SD) - Deals > Equity M &A - , , , panes wing/sets/iables/utput : Meeting - 3 MRP Zamethr Sier 3 S · Value. CMA MOM -> On KF.. Website below Factor Models - 3FF = CAPM + SMB + HML - 5FF = 3FF + CMA + RMW - 4FF = 3FF + MOM - PS = 3FF + MuM + LIQ · Data Text : to coluers Home Str + H-Replace > , by - · Factor Value of SMB in October 2005 Fama/French 3 Relevant Zelange : 1 , 2 3 , Factor models ▪ Capital Asset Pricing Model (CAPM) Ri – rf = a + b MRP + e where: – Ri is the return of a firm i – rf is the risk-free rate – MRP is the market risk premium which is the difference between the market return (RM) and risk-free (rf) MRP = RM - rf Data Science for Finance 3 Factor models ▪ 3 Fama-French factor model (3FF) Ri – rf = a + b1 MRP + b2 SMB + b3 HML + e where: – SMB: Small minus Big (mkt cap) or SIZE Small stocks minus large stocks – HML: High minus Low (book to market ratio) or VALUE Cheap stocks minus expensive stocks See a definition of these factors in Kenneth French website Data Science for Finance 4 Factor models ▪ 5 Fama-French factor model (5FF) Ri – rf = a + b1 MRP + b2 SMB + b3 HML + b4 RMW + b5 CMA + e where: – SMB: Small minus Big (mkt cap) – HML: High minus Low (book to market ratio) – RMW: Robust minus Weak (operating profitability) Most profitable stocks minus least profitable stocks – CMA: Conservative minus Aggressive (investment) Invest conservatively stocks minus Invest aggressively stocks Data Science for Finance 5 Factor models ▪ Carhart factor model (Carhart or 4FF) Ri – rf = a + b1 MRP + b2 SMB + b3 HML + b4 MOM + e where: – SMB: Small minus Big (mkt cap) – HML: High minus Low (book to market ratio) – MOM: Winners minus Losers (past performance in stock returns) or MOMENTUM Highest performer stocks minus least performer stocks Data Science for Finance 6 Factor models ▪ Another 5 factor model…an extension of PS (not 5FF) Ri – rf = a + b1 MRP + b2 SMB + b3 HML + b4 MOM + b5 LIQ + e where: – LIQ: Iliquid minus Liquid (liquidity shocks) Iliquid stocks minus liquid stocks From Pastor & Stambaugh (existent in WRDS) Data Science for Finance 7 Factor models Summary ▪ CAPM ▪ 3FF = CAPM + SMB + HML ▪ 5FF = 3FF + CMA + RMW ▪ 4FF = 3FF + MOM ▪ PS = 3FF + MOM + LIQ ▪ This is just a mnemonic and you can never write like this Data Science for Finance 8 Kenneth French website Data ▪ Kenneth French website (how to get market and factors data?) ▪ See details for description ▪ VW vs. EW ▪ Annually, monthly, weekly, daily Data Science for Finance 9 CRSP ▪ CRSP (how to get stock market data?) – How to get prices? – How to get holding returns? – How to get number of shares outstanding? – How to get CRSP VW and EW? – See description of stock exchange variable – Watch out for empty cells in the time-series Data Science for Finance 10 CRSP Example: CRSP Daily Stock File ▪ From CRSP. You have access to different pricing data for the US Equity Markets. Virtually all US traded stocks since 1963 are in CRSP. ▪ Stock prices are within the “Stock / Security Files” menu on the left. ▪ The simplest query you can submit is on ‘Daily Stock File’, which includes daily prices and returns. ▪ To submit a query, just click the link on the left hand-side corresponding to the type of data you want to access. Data Science for Finance 11 CRSP Example: CRSP Daily Stock File The query process is divided in steps. ▪ Step 1: Define the data range you want data for: – It will give you whatever data exists for the selected stocks during that time period. ▪ Step 2.1: Select type of identifier you will use to select the Stocks. Several identifiers are available: – TICKER is not the best option because tickers can be reused in time by different companies; – PERMNO idenitifies uniquely a company in the CRSP database, so that is usually the best option. ▪ Step 2.2: Enter the company codes for the stocks you wish to extract from CRSP. – If you do not know the company code, use Code Lookup (below where you enter the company codes – see example in next slide); – Additionally, you can query the whole database or upload a file with all company codes. ▪ Step 2.3: Conditionally select the data based on available fields: – For example, you may limit extraction to records with price greater than 5 dollars. Data Science for Finance 12 CRSP Example: CRSP Daily Stock File The query process is divided in steps. ▪ Step 3: Select the fields you want to extract: – Identifying information: Information that identifies the company; – Time-Series information: Price and return information across time; – Share information: Number of shares outstanding and share type flag; – Delisting information: Information about the trading status of a stock; – Distribution information: Dividend and acquisition data; ▪ Step 4: Select data format, date format and compression. ▪ For a more detailed explanation go to CLSBE website>Student Affairs>Services>More Information and look for “How to get started with WRDS”: Data Science for Finance 13 Refresher on Statistics Moments Moments allow us to characterize the observed distribution of a variable. ▪ Mean: First raw moment of a variable. Expected value of a variable. = E ( X ) = i =1 xi f ( xi ) T ▪ Variance: Expected difference from the mean, to the power of 2. 2 = E (( X − ) 2 ) = i =1 ( xi − ) 2 f ( xi ), 2 0 T ▪ Skewness: Expected standardized difference from the mean, to the power of 3. 1 1 T S= E (( X − ) 3 ) = ( x − ) 3 f ( xi ) 3 3 i =1 i ▪ Kurtosis: Expected standardized difference from the mean, to the power of 4. 1 1 T K= E (( X − ) 4 ) = ( x − ) 4 f ( xi ), K 0 4 4 i =1 i ▪ Excess Kurtosis: Kurtosis subtracted by 3, the value of the kurtosis for a stnd. Normal dist. 1 1 i =1 i T EK = E (( X − ) 4 ) − 3 = ( x − ) 4 f ( xi ) − 3 4 4 Data Science for Finance 14 Refresher on Statistics Moments: Skewness ▪ Positive skewness indicates a right-skewed distribution. ▪ Negative skewness indicates a left-skewed distribution. Plot source: Rodolfo Hermans at en.wikimedia Data Science for Finance 15 Refresher on Statistics Moments: Kurtosis ▪ Kurtosis larger than 3 (positive excess kurtosis) indicates a leptokurtic distribution. pdf -4 -2 0 2 4 x ▪ Kurtosis smaller than 3 (negative excess kurtosis) indicates a platykurtic distribution. pdf -4 -2 0 2 4 x Data Science for Finance 16 Refresher on Statistics Additional definitions ▪ Covariance: Characterizes how two variables vary together. – Difficult interpretation, as it tied to variable scale. x , y = E (( X − x )(Y − y )) ▪ Correlation coefficient: Characterizes magnitude of variable linear co-movement. – Coefficient value is positive (negative) in case two variables simultaneously positively and negatively (or not) deviate from their means. Cov( x, y ) x, y = ,−1 1 x y – Autocorrelation of order k is the correlation between the variable and itself lagged k periods. Cov( xt , xt −k ) (k ) = ,−1 1 xxt t −k Data Science for Finance 17 Refresher on Statistics Autocorrelation ▪ Autocorrelation or serial-correlation or order k: ▪ Another popular designation is AR(k). ▪ For example, Data Science for Finance 18 Refresher on Statistics Coefficient of correlation ▪ Notice the different patterns that may be described by a correlation coefficient. ▪ Notice that the correlation coefficient only translates linear relations. The figure below is a good example of patterns that go undetected by the correlation coefficient because they do not follow a linear pattern (bottom). Additionally, it does not capture differences in slope (middle). Plot source: Kiatdd and DenisBoigelot at en.wikimedia, respectively Data Science for Finance 19 Excel tools Statistical functions ▪ Several statistical functions of Excel will be useful, namely: – AVERAGE – arithmetic average of a range; – GEOMEAN – geometric average of a range; – MEDIAN – median of a range; – STDEV – sample standard deviation of a range (STDEVP for population std. dev.); – SKEW – skewness of a range; – KURT – excess kurtosis of a range; – COVAR – covariance of a range – CORREL – correlation of a range; – MIN – minimum of a range – MAX – maximum of a range – COUNT – count the number of observations in a range – PERCENTILE – percentile of a range Data Science for Finance 20 Mom formula-value weighted portfolio VW : w = f(Mk CAP) + E ligger stock gets a bigge wigest Book to Market Valueoften confused Stratyy Proxy For : > - SMB - 5 (because mutral included) HMLt Standed Deviation m t A - 3 Variance - = = Mean c - - - 62 = ) - = Skewness Kurtosis ~ = t[(xi m)3 - - - - 23 - - = - > - Excess Kestois N(0 , 1) Skeruss = ↓↓ 3 Mean Sta deviation. - Kurosis + AR(2) Autoregressive Coefficient of order or Autocorrelation ear correlation r AR()/(2)-> excel : bur out first or first two values to match with not worth El ) Expected. value a , beR VI ). Variance E(a) = a StdDev = F = G (). E(ax + by) aE(x) bE(y) = + Cor(x Covariance E(X zy) E(x) 2E(y) y) = - - , Coor (x y) , Correlation - V(a) = 0 at Vlax + by) = v(x) v(y) 2abcor(X y) + b2 + , & i ,i. d V(X - 2y) V(x) 4V(y) 4(or(x y) = + - , ↓ m identically distributed independent ↓ E(X) Ely) = E(x + y) = t(x) + E(y) - cor(x y) = 0 V(x) V(y) = V(x) + V(y) + 2cr(x V(y + y) y) , = Skew(x) (y) , = Skew i & Ry = R + R2 +....+ Ri E(Ry) 12E(Rm) E(Ry) = E(Rn R2+... + + Rez) 12E(m) => E(Ry) 12E(m) = Sequency = · = V(y) = V(9) v(R2) +... V(Rnz) - + + + 2(r(2) + 2 Cr (R2 , Rs) +.... 2(or(Rufun) + G(ry) = 6(rm) · Fre i , id. = 12V(fr) i. i d.. " V(ky) 12V(m) = = G(ky) v'G(km) = Var(x) = 4 Va(Y) = 0 Var(8x + 4y - 12) = & Var(x) + v(y) + 2(v(x y) , Var(8x + 4y - 12) = 82 Var(X) + 4? Varl-12 = 82. 4 + 42 8. = 64. 4. 10. 8 256 = +128 = 384 Sharpe Ratio SR : SRy = R. Som · a WRDS Assets Total million Computat > - in - Ening Ets ut po juid- - Annualizing average returns and volatility ▪ To annualize average returns: – From daily: multiply by 252 (sometimes 250 or 256 or count number of trading days in that year…your choice) – From monthly: multiply by 12 – From quarterly: multiply by 4 ▪ To annualize volatility: – The same as before with a square root – E.g. Annualized Volatility = sqrt(252) x Daily Volatility Data Science for Finance 3 Sharpe ratio ▪ The Sharpe ratio measures the tradeoff between expected return and risk – How much expected excess return can be obtained per unit of standard deviation ▪ To estimate the Sharpe ratio, replace sample moments ▪ A monthly Sharpe ratio is usually annualized by multiplying by ▪ This works for a long position. For a long-short strategy do not need to subtract the risk-free in the numerator Data Science for Finance 4 Refresher on Statistics Asymptotic distributions ▪ Mean: Distributed assymptotically as: T ( ˆ − ) ~ N (0, 2 ) Data Science for Finance 3 Refresher on Statistics Confidence intervals ▪ Assuming a certain distribution for a variable, a confidence interval allows us to estimate its possible value under a certain confidence level. ▪ For example, a confidence interval for the mean can be written as: ˆ − Z s.e. ˆ + Z s.e. crit (1− ) crit (1− ) 2 2 ( ˆ ) where s.e. = T ▪ Similar reasoning may be applied to the skewness and kurtosis. Data Science for Finance 4 Refresher on Statistics Hypothesis testing: t-Statistics To conduct hypothesis testing, you need to first define the null and alternative hypothesis. H0 : = 0 HA : 0 Assuming: = 5%, ˆ = 10%, ( ˆ ) = 20%, T = 100 with Z 97,5% = 1,96 ▪ Using t-statistics as a decision mechanism ˆ − t ˆ = ~ N (0,1) s.e.( ˆ ) 10% − 0% t ˆ = = 5 Z 97 ,5% 20% 100 – As the critical value for the normal distribution with a 5% significance level is 1,96 (right tail of a bilateral test), we reject the null hypothesis. Data Science for Finance 5 Refresher on Statistics Hypothesis testing: t-Statistics visualized 0.45 pdf 0.4 0.35 0.3 0.25 Bilateral Critical Regions 0.2 Standard Normal Dist. 0.15 0.1 0.05 0 -4 -3 -2 -1 0 1 2 3 4 x Data Science for Finance 6 Refresher on Statistics Hypothesis testing: P-value To conduct hypothesis testing, you need to first define the null and alternative hypothesis. H0 : = 0 HA : 0 Assuming: = 5%, ˆ = 10%, ( ˆ ) = 20%, T = 100 with Z 97,5% = 1,96 ▪ Using p-value as a decision mechanism – The p-value is the probability of getting a value “equal or more extreme” than the t-stat. – The p-value is compared against the significance level. If greater than the significance level, you do not reject the null hypothesis. If smaller than the significance level, you reject the null hypothesis. pvalue = 2 (1 − Z inv (5)) = 5,73 10 −7 5% – In this case, since the p-value is 0,000000573, which is lower than 0,05, you reject the null hypothesis that the mean is equal to zero. Data Science for Finance 7 Refresher on Statistics Hypothesis testing: Confidence Interval To conduct hypothesis testing, you need to first define the null and alternative hypothesis. H0 : = 0 HA : 0 Assuming: = 5%, ˆ = 10%, ( ˆ ) = 20%, T = 100 with Z 97,5% = 1,96 ▪ Informally, you may use confidence intervals as a decision mechanism: 20% 20% 10% − 1,96 10% + 1,96 100 100 6,08% 13,92% – Since zero is not in the confidence interval, for a 5% significance level we reject that the mean is zero. ▪ This is a poor decision mechanism if you consider unilateral tests. See following slides. This is not a proper testing method. Data Science for Finance 8 Refresher on Statistics Hypothesis testing: t-Statistics (unilateral) To conduct hypothesis testing, you need to first define the null and alternative hypothesis. Assuming: = 5%, ˆ = 10%, ( ˆ ) = 20%, T = 100 with Z 95% = 1,645 ▪ Using t-statistics as a decision mechanism ˆ − t ˆ = ~ N (0,1) s.e.( ˆ ) 10% − 0% t ˆ = = 5 Z 95% 20% 100 – As the critical value for the normal distribution with a 5% significance level is 1,645 (right tail of a unilateral test), we reject the null hypothesis. Data Science for Finance 9 Refresher on Statistics Hypothesis testing: t-Statistics visualized 0.45 pdf 0.4 0.35 0.3 0.25 Unilateral Critical Region 0.2 Standard Normal Dist. 0.15 0.1 0.05 0 -4 -3 -2 -1 0 1 2 3 4 x Data Science for Finance 10 Refresher on Statistics Hypothesis testing: P-value (unilateral) To conduct hypothesis testing, you need to first define the null and alternative hypothesis. Assuming: = 5%, ˆ = 10%, ( ˆ ) = 20%, T = 100 with Z 95% = 1,645 ▪ Using p-value as a decision mechanism – The p-value is the probability of getting a value “equal or more extreme” than the t-stat. – The p-value is compared against the confidence level. If greater than the confidence level, you do not reject the null hypothesis. If smaller than the confidence level, you reject the null hypothesis. pvalue = 1 − Z inv (5) = 2,87 10 −7 5% – In this case, since the p-value is 0,000000287, which is lower than 0,05, you reject the null hypothesis that the mean is equal to zero. Data Science for Finance 11 Excel tools Statistical functions ▪ Several statistical functions of Excel will be useful, namely: – NORMSDIST – (cummulative) distribution of a standard normal distribution; i.e. Cummulative NORMSDIST = P(Z x); if tails = 2, TDIST = P(|t| > x) – TDISTINV – inverse of the right tail probability of a t-student distrbution. Always check Excel’s function help to know how each function works. Data Science for Finance 12 Practical Application 1 Continuing …using same file…hypothesis testing ▪ Extract daily historical prices and holding returns for Apple, Microsoft and Bank of America between 2002 jan 1 and 2017 dec 31 using CRSP; get also the CRSP VW index holding returns ▪ Plot prices and returns in this period ▪ Run descriptive statistics including a Sharpe ratio ▪ Test if mean monthly return is equal to zero for each security with a significance level of 5% ▪ Test if mean monthly return of IBM is equal to 0.15 with a significance level of 10% Data Science for Finance 13 Variance ((m - M) - N(0 82) , > - Sex = in X - 6 number of observations - X + sX ⑤ F (New-0)vN(0 6) , -J. eckew = I for class but Kurt-3) ~(0 25) , se.. kunt = 7 in Quiz also ↑ different F (AR(k) 0) - ~W(0 , 1) > - s e.. Arch) = x = 5% => for I any the formula stays the same/doesn't depend on RV Will H Ho 18 3 - m : = Biletare Test or 2 sided Test Alternative H-Ha : M + 18 7 formula from above = t-stat = Ero 1 seX Two Ways to test Hypothesis ① Critical Region (CR) ② p-value DIST(#) NORM S Probi E > - */2. ① 422 5% = 2 5% Encl INV(Prob ).. T NORM S # I -... CR R - 1 96. 1 96. = NORM S INV (0... 025) = NORM. S. INV(0 975). t-stat = 1 34. #CR -do not Ho reject the mill with 5 % I do not reject significance. level that the not 18. I cannot say average grade of quiz is -Ho ② p-value = Prob. [t-stat or wors] Direction of Alternative - = Prob. [211 347. + Prob [23 - 1. 31) = 2 Prob [211 34]. = 2 Prob[1 -Prob (21 -.. 34)] = 18 02%. > - do not reject EXL NORM S DIST (1 34 , :... 1) = 0. 909877 >1 = TRUE If p-value s X - do not reject Ho alternative to calculate If p-value < - > reject Ho W 2. NORM. S DIST(-1 TRUE) , the will with level. - o not reject 5% significance 10 % doesn't +-stal X = - affect Two-Tailed > - we have to change critical region 1 % + 2. 576 1 5%. - 2 241. If t 31 =. 73 then we reject o at (31 % ). 5% 10 % + - 1 1645. 960 alpha of 40 %. 73 One-Sided-Test X= 5% Ho MC2 He M 2 : = : X = 1 1 =)= 2 2 = 10 t-stat = Ero - T = 100 seX - - #% x5 Prob [22 1 3 Probability of will = - true ! Fam being = 1 - Prob[z - 1] ↑ 0 1645 = 1- NORM S DIST(-1.. , TRUE) = 84 13%. at 5 I cannot reject the will significance. ovel We cannot that the is not less equal to 2. say mean or Skeness -If of equal we test to zero Ho shewness 3 if : = 0 we reject the will skewness is significant. H : skewness - O - skew = 0 1. Feeskew - 0 1 0 new 0 408254 - t-stat. = = = => = (i). Seskew * 2 (1 NORM S DIST (0 41 ,TRUE) 60 18% = =. -.... 2 5%. 8 1. 96 P-value & Lamet rejt the will at We cannot reject 5% significance. Ho He : : M M = + 2 2 -statistic : ExMo = = -1 CR : - the to at % significance We cannot that the reject 5 we cannot say. Some definitions ▪ EXPECTED VALUE: E(.) – Generally, this means "Mean" or "Average" ▪ VARIANCE: V (.) (always a non-negative number) – Sometimes it is also denoted as VAR(.) – Can be computed as E((X-E[X])2) or also E(X2) – E(X)2 ▪ STANDARD DEVIATION: (always a non-negative number) – STD.DEV(.) = V(. ) ▪ COVARIANCE: COV(X,Y) – Degree to which 2 variables “change together” (i.e. how linear independent are from each other) ▪ CORRELATION: CORR(X,Y) – Degree to which 2 variables “change together” (i.e. how linear independent are from each other) but is standardized measure (varies between -1 and 1): COV(X,Y) – CORR(X,Y) = V(X). V(Y) Data Science for Finance 3 Rules of operators E(.) and V(.) and related Key Takeaways ▪ Let X, Y be random variables, and where a,b ∈ R (real numbers), then: 1. E (aX + bY) = aE(X) + bE(Y) 2. E(a) = a 3. V(aX bY) = a2V(X) + b2V(Y) 2abCOV(X,Y) (needs to result in a non- negative variance) 4. V(a) = 0 5. COV(aX,bY) = abCOV(X,Y) 6. CORR(aX,bY) = CORR (X,Y) 7. If X and Y are independent, then COV(X,Y) = 0 and also CORR(X,Y) = 0 Data Science for Finance 4 Rules of operators Solved exercises ▪ IF E(X) = 7, THEN: 1. E(2X) = ? 2. E(X+3) = ? 3. E(2X+3) = ? ▪ IF E(X) = 5 AND E(Y) = 3, THEN: 1. E(X+Y) = ? 2. E(X-Y) = ? 3. E(2X-3Y) = ? 4. E(X-Y+4) = ‘ ▪ IF V(X) = 7, THEN: 1. V(2X) = ? 2. V(2X+3) = ? 3. V(-X+4) = ? Data Science for Finance 5 Rules of operators Solved exercises ▪ IF E(X) = 7, THEN: 1. E(2X) = 2E(X) = 2x7 = 14 2. E(X+3) = E(X)+3 = 7+3 = 10 3. E(2X+3) = 2E(X)+3 = 2x7+3 = 17 ▪ IF E(X) = 5 AND E(Y) = 3, THEN: 1. E(X+Y) = E(X)+E(Y) = 5+3 = 8 2. E(X-Y) = E(X) - E(Y) = 5-3 = 2 3. E(2X-3Y) = 2E(X) - 3E(Y) = 2x5-3x3 = 10-9 = 1 4. E(X-Y+4) = E(X) - E(Y)+4 = 5-3+4 = 6 ▪ IF V(X) = 7, THEN: 1. V(2X) = 22V(X) = 4V(X) = 4x7 = 28 2. V(2X+3) = 22V(X)+V(3) = 4V(X) + 0= 4x7 = 28 3. V(-X+4) = (-1)^2V(X)+V(4) = V(X) + 0 = 7 Data Science for Finance 6 Properties of operators Solved exercises ▪ IF V(X) = 5, V(Y) = 3 AND COV(X,Y) = 2, THEN: 1. V(X+Y) =? 2. V(X-Y) = ? 3. V(2X-3Y) = ? 4. V(2X-3Y+4) = ? ▪ IF V(X) = 5, V(Y) = 3 AND X,Y are INDEPENDENT (COVARIANCE = 0), THEN: 1. V(X+Y) = ? 2. V(X-Y) = ? 3. V(Y-X) = ? ▪ Assume that a company's quarterly profits follow a random variable Q, with an expected value of $10,000. What is the expected annual profit? 1. E(Y) = ? Data Science for Finance 7 Properties of operators Solved exercises ▪ IF V(X) = 5, V(Y) = 3 AND COV(X,Y) = 2, THEN: 1. V(X+Y) =V(X)+V(Y)+2COV(X,Y) = 5+3+2x2 = 12 2. V(X-Y) = V(X)+V(Y)-2COV(X,Y) = 5+3-2x2 = 4 3. V(2X-3Y) = 22V(X)+32V(Y)-2x2x3COV(X,Y) = 4x5+9x3-12x2 = 20+27-24 = 23 4. V(2X-3Y+4) = V(2X-3Y)+0 = 23 (same as in exercise 3) ▪ IF V(X) = 5, V(Y) = 3 AND X,Y are INDEPENDENT (COVARIANCE = 0), THEN: 1. V(X+Y) = V(X)+V(Y)+2COV(X,Y) = 5+3+2x0 = 8 2. V(X-Y)= V(X)+V(Y)-2COV(X,Y) = 5+3-2x0 = 8 3. V(Y-X) = V(Y)+V(X)-2COV(X,Y) = 3+5-2x0 = 8 ▪ Assume that a company's quarterly profits follow a random variable Q, with an expected value of $10,000. What is the expected annual profit? 1. E(Y) = E(𝑄1 + 𝑄2 + 𝑄3 + 𝑄4 ) = E(4Q) = 4xE(Q) = 4x10,000 = $40,000 Data Science for Finance 8 Normal Distribution ▪ N(µ,), where µ = mean and = Standard Deviation ▪ We call the standard normal distribution when µ =0 and =1, denoted by N(0,1). Data Science for Finance 9 Normal Distribution Solved exercises ▪ You have a dataset of MSFT US Equity monthly returns. Assuming that they follow a normal distribution with a mean (μ) of 0.1% and a standard deviation (σ) of 0.2%. Calculate the probability that next month’s return will be higher than 0.5%. Data Science for Finance 10 Normal Distribution Solved exercises ▪ You have a dataset of MSFT US Equity monthly returns. Assuming that they follow a normal distribution with a mean (μ) of 0.1% and a standard deviation (σ) of 0.2%. Calculate the probability that next month’s return will be higher than 0.5%. – Standardize the score using the Z-score formula: Z = (X - μ) / σ => Z = (0.5% - 0.1%) / 0.2% = 2 – In this case, you want the probability that Z is greater than 2, which is typically denoted as P(Z > 2). – You know that P(Ac) = 1 - P(A), then P(Z > 2) = 1 – P(Z ≤ 2). – To get P(Z ≤ a) use Excel function NORM.S.DIST(a,TRUE). Then P(Z > 2) = 1 – P(Z ≤ 2) = 1- NORM.S.DIST(2,true) = 1 – 0. 0.9725 ≈ 0.0275=2.75% – So, the probability that next month’s return is above 0.5% is approximately 2.75%. White area: Blue area: 1 - NORM.S.DIST(2,true) NORM.S.DIST(2,true) = 2.75% =97.25% Data Science for Finance 11 Normal Distribution Solved exercises ▪ Given a dataset of adults' heights that follows a normal distribution with μ = 170 cm and σ = 7 cm, find the Z-score for a person who is 180 cm tall. Interpret the meaning of this Z-score in the context of the distribution. Data Science for Finance 12 Normal Distribution Solved exercises ▪ Given a dataset of adults' heights that follows a normal distribution with μ = 170 cm and σ = 7 cm, find the Z-score for a person who is 180 cm tall. Interpret the meaning of this Z-score in the context of the distribution. – Calculate the Z-score using the Z-score formula: Z = (X - μ) / σ => Z = (180 - 170) / 7 ≈ 1.43 – So, the Z-score for a person who is 180 cm tall is approximately 1.43. – This means the person's height is 1.43 standard deviations above the mean height of 170 cm. 1.43 standard deviations above the mean Data Science for Finance 13 Normal Distribution Solved exercises ▪ The IQ scores in a population are normally distributed with μ = 100 and σ = 15. Calculate the 75th percentile (also called third tercile) for IQ scores. What does this percentile represent? Data Science for Finance 14 Normal Distribution Solved exercises ▪ The IQ scores in a population are normally distributed with μ = 100 and σ = 15. Calculate the 75th percentile (also called third tercile) for IQ scores. What does this percentile represent? – You can use the Excel function NORM.S.INV(prob) which gives the a in P(Z a) = prob – In this case, we want P(Z a) = 0.75 which means NORM.S.INV(0.75), which in Excel gives the value a ≈ 0.6745 (rounded to four decimal places) – This a is in the standard normal distribution. But we know that this is not a standard normal distribution; so we have to do the reverse to get the non-standardized distribution; you are a standard deviations above (when positive) or below (when negative) the mean – In this case, you are 0.6745 standard deviations above the mean, i.e., – X = μ + aσ => X = 100 + 0.6745 * 15 ≈ 110 (rounded to zero decimal places) – So, the 75th percentile for IQ scores is approximately 110. – It shows that 75% of the people have a IQ score of less than 110 and 25% of the people have a IQ score of more than 110 Data Science for Finance 15 Normal Distribution Solved exercises ▪ Consider a random variable X that follows a normal distribution with a mean (μ) of 50 and a standard deviation (σ) of 10. Calculate the probability that X is between 40 and 60. Data Science for Finance 16 Normal Distribution Solved exercises ▪ Consider a continuous random variable X that follows a normal distribution with a mean (μ) of 50 and a standard deviation (σ) of 10. Calculate the probability that X is between 40 and 60. – Calculate the Z-scores for 40 and 60: Z = (X - μ) / σ => 𝑍1 = (60 - 50) / 10 = 1 and 𝑍2 = (40 - 50) / 10 = -1 – Find the probabilities associated with these Z-scores using a standard normal distribution table or excel formulas, then: For 𝑍1 = 1, P(Z ≤ 1) = NORM.S.DIST(1,true) ≈ 0.8413 For 𝑍2 = -1, P(Z ≤ -1) = NORM.S.DIST(1,true) ≈ 0.1587 – Calculate the probability between these Z-scores: P(-1 ≤ Z ≤ 1) = P(Z ≤ 1) - P(Z ≤ -1) ≈ 0.8413 - 0.1587 ≈ 0.6826 – Interpretation: This means that there's a 68.26% chance that a randomly selected value from this distribution will be between 40 and 60. Data Science for Finance 17 Distribution Exercises ▪ Assume there are some variable R - returns - that follow a normal distribution with a population mean of 0.80% and a population standard deviation of 15. If you take random samples of size N (N=10, 20, 30, 40, 50) from this population, what is the standard error of the sampling distribution of the sample mean? Interpret what you see. Data Science for Finance 18 Distribution Exercises ▪ Assume there are some variable R - returns - that follow a normal distribution with a population mean of 0.80% and a population standard deviation of 15%. If you take random samples of size N (N=10, 20, 30, 40, 50) from this population, what is the standard error of the the sampling distribution of the sample mean? Interpret what you see. – N = 10 Calculate the standard error = SE = σ / √n = 15% / √10 ≈ 4.74% – If R follows a distribution around the true mean of 0.80% with a standard deviation of 15%, the mean of 15 R follows a distribution with a standard error of only 4.74%; the mean reduces the deviation. N s.e. of mean (%) – If you compute this to the different values: 10 4.74 20 3.35 30 2.74 40 2.37 50 2.12 500 0.67 10000 0.15 – Interpretation: As the sample size increases, the standard error (SE) decreases. This means that with larger sample sizes, the sample mean is more likely to be close to the population mean, leading to a more precise estimate. Data Science for Finance 19 Normal Distribution Solved exercises ▪ If Z~N(0,1), what is the distribution of 5Z? ▪ If Z~N(0,1) what is the distribution of Z+4? ▪ If Z~N(0,1) what is the distribution of 3Z+6? Data Science for Finance 20 Normal Distribution Solved exercises ▪ If Z~N(0,1), what is the distribution of 5Z? – E(5Z) = 5E(Z) = 5x0 = 0 – V(5Z) = 52V(Z) = 25x1 = 25 => = 5 – Thus 5Z ~ N (0,5) ▪ If Z~N(0,1) what is the distribution of Z+4? – E(Z+4) = E(Z)+4 = 0+4 = 4 – V(Z+4) = V(Z)+0 = 1 => = 1 – Thus Z+4 ~ N (4,1) ▪ If Z~N(0,1) what is the distribution of 3Z+6? – E(3Z+6) = 3E(Z)+6 = 3x0+6 = 6 – V(3Z+6) = 32V(Z)+0 = 9x1 = 9 => = 3 – Thus 3Z+6 ~ N (6,3) Data Science for Finance 21 Normal Distribution Exercises ▪ If 𝑍1 ~N(0,1), 𝑍2 ~N(0,1) and 𝑍1 and 𝑍2 are independent, what is the distribution of 𝑍1 +𝑍2 ? ▪ If 𝑍1 ~N(0,1), 𝑍2 ~N(0,1) and 𝑍1 and 𝑍2 are independent, what is the distribution of 3𝑍1 +2𝑍2 ? Data Science for Finance 22 t-student Distribution ▪ In hypothesis testing, when dealing with small sample sizes (usually less than 25 observations), a normal distribution is not adequate ▪ A t-student distribution is similar to a normal but with heavier tails (kurtosis greater than 3, still symmetric) ▪ A t-student distribution has a parameter designated by degrees of freedom (dof) and this is usually the number of observations in the sample ▪ As the number of degrees of freedom of a t-student distribution increases, the t-student distribution converges to a normal distribution as you can see in the graph below ▪ Blue is a normal distribution red 1 dof new red 3 dof new red 5 dof ▪ You can compute the probabilities equivalent to a normal distribution using the Excel functions P(t ≤ a) = T.DIST(a,dof,true) and get the a in the equation P(t ≤ a) = prob from T.INV(prob,dof) Data Science for Finance 24 t-student Distribution Solved exercises Assuming a t-student distribution with 5 degrees of freedom ▪ Prob(t ≤ 1.96) = ? ▪ Prob(t ≤ -0.50) = ? ▪ Prob(t > 1.33) = ? ▪ Compute a in Prob(t ≤ a) = 0.90. Assuming a t-student distribution with 25 degrees of freedom ▪ Prob(t ≤ 1.96) = ? ▪ Prob(t ≤ -0.50) = ? ▪ Prob(t > 1.33) = ? ▪ Compute a in Prob(t ≤ a) = 0.90. Data Science for Finance 25 t-student Distribution Solved exercises Assuming a t-student distribution with 5 degrees of freedom ▪ Prob(t ≤ 1.96) = T.DIST(1.96, 5 , TRUE) = 94.64% ▪ Prob(t ≤ -0.50) = T.DIST(-0.50, 5 , TRUE) = 31.91% ▪ Prob(t > 1.33) = 1 - Prob(t ≤ 1.33) = 1 - T.DIST(1.33, 5 , TRUE) = 12.05% ▪ Compute a in Prob(t ≤ a) = 0.90. It is just T.INV(0.90,5) = 1.48 Assuming a t-student distribution with 25 degrees of freedom ▪ Prob(t ≤ 1.96) = T.DIST(1.96, 25 , TRUE) = 94.94% ▪ Prob(t ≤ -0.50) = T.DIST(-0.50, 25 , TRUE) = 31.07% ▪ Prob(t > 1.33) = 1 - Prob(t ≤ 1.33) = 1 - T.DIST(1.33, 25 , TRUE) = 9.78% ▪ Compute a in Prob(t ≤ a) = 0.90. It is just T.INV(0.90,25) = 1.32 Data Science for Finance 26 Chi-Squared Distribution ▪ Chi-squared distribution (2) is the result of a squared standardized normal distribution If Z~N(0,1), then Z 2 ~𝑋12. In this case, we obtain a chi-squared distribution with 1 degree of freedom. ▪ It is always positive. It is defined by its degrees of freedom “v” -> 𝑋𝑣2 ▪ To obtain more than 1 degree of freedom, you need to add several independent chi- squared distributions of 1 degree of freedom ▪ To get 𝑋𝑣2 simply add 𝑋𝑖2 “v” times, assuming Zi are independent from each other, then: Z~N(0,1) Then: ▪ 𝑍12 + 𝑍22 ~ 𝑋22 ▪ 𝑍12 + 𝑍22 + 𝑍32 ~ 𝑋32 ▪ 𝑍12 + 𝑍22 + 𝑍32 + 𝑍42 ~ 𝑋42 ▪ 𝑍12 + 𝑍22 + 𝑍32 + 𝑍42 + … + 𝑍𝑣2 ~ 𝑋𝑣2 Data Science for Finance 27 t-student Distribution Solved exercises Compute the difference between a normal distribution and a t-student with a specific number of degrees of freedom: 1. At 90% with dof = 10, 25, 50, 100, 1000 2. At 95% with dof = 10, 25, 50, 100, 1000 3. At 99% with dof = 10, 25, 50, 100, 1000 4. At 99.5% with dof = 10, 25, 50, 100, 1000 Data Science for Finance 28 t-student Distribution Solved exercises Compute the difference between a normal distribution and a t-student with a specific number of degrees of freedom: 1. At 90% with dof = 10, 25, 50, 100, 1000 90% normal 1.282 NORM.S.INV(0.9) N t-student 10 1.372 T.INV(0.9;10) 25 1.316 T.INV(0.9;25) 50 1.299 T.INV(0.9;50) 100 1.290 T.INV(0.9;100) 1000 1.282 T.INV(0.9;1000) There is a difference between values from a normal distribution and a t-student distribution that is not so relevant when N>25 Data Science for Finance 29 t-student Distribution Solved exercises Compute the difference between a normal distribution and a t-student with a specific number of degrees of freedom: 2. At 95% with dof = 10, 25, 50, 100, 1000 95% normal 1.645 NORM.S.INV(0.95) N t-student 10 1.812 T.INV(0.95;10) 25 1.708 T.INV(0.95;25) 50 1.676 T.INV(0.95;50) 100 1.660 T.INV(0.95;100) 1000 1.646 T.INV(0.95;1000) There is a difference that is not so relevant when N=1000 Data Science for Finance 30 t-student Distribution Solved exercises Compute the difference between a normal distribution and a t-student with a specific number of degrees of freedom: 2. At 99% with dof = 10, 25, 50, 100, 1000 99% normal 2.326 NORM.S.INV(0.99) N t-student 10 2.764 T.INV(0.99;10) 25 2.485 T.INV(0.99;25) 50 2.403 T.INV(0.99;50) 100 2.364 T.INV(0.99;100) 1000 2.330 T.INV(0.99;1000) There is a difference that is not so relevant when N=1000 Data Science for Finance 31 t-student Distribution Solved exercises Compute the difference between a normal distribution and a t-student with a specific number of degrees of freedom: 2. At 99% with dof = 10, 25, 50, 100, 1000 99.5% normal 2.576 NORM.S.INV(0.995) N t-student 10 3.169 T.INV(0.995;10) 25 2.787 T.INV(0.995;25) 50 2.678 T.INV(0.995;50) 100 2.626 T.INV(0.995;100) 1000 2.581 T.INV(0.995;1000) There is a difference that is not so relevant when N=1000 (but still a bit different) Data Science for Finance 32 t-student Distribution Solved exercises Conclusion: the value of a t-student and a normal distribution is quite close with N>25 but only if we are not in the very deep tail of the distribution. Thus, if you are doing hypothesis testing you can always use a t-student distribution (which is the most correct) and this is the threshold that you should compare to. For that threshold we take as degrees of freedom the number of observations subtracted by one. Therefore, dof= nr obs – 1. In this class, we assume that a normal distribution is a good approximation for “small number of observations” even at the very deep tail. But you should understand that this assumption is not totally realistic. Data Science for Finance 33 Statistics Chi-squared Distribution (df, k = degrees of freedom) Data Science for Finance 34 Chi-Squared Distribution Exercises 𝑋−5 𝑋−5 2 ▪ If Z~N(5,4), then ~N(0,1), which means that ~𝑋12. 4 4 ▪ If 𝑋1 ~𝑋32 , 𝑋2 ~𝑋42 , and 𝑋3 ~𝑋82 , assuming they are independent, then: 2 2 – 𝑋1 + 𝑋2 + 𝑋3 ~ 𝑋(3+4+8) = 𝑋15 2 2 – 𝑋1 + 𝑋3 ~ 𝑋(3+8) = 𝑋11 ▪ Calculate the mean and standard deviation of a chi-squared distribution with 12 degrees of freedom. – The mean (μ) and variance (VAR) of a chi-squared distribution with v degrees of freedom are given by: μ = v = 12 VAR =2v = 2 x 12 =24 Data Science for Finance 35 ↓ 2 ~ N(0 , 1) = 22 X , Ho : X ~ Normal Distribution Se = X I ~N(0 , 1) HA Normal Distribution 3 2- X * : Skew = 22 ~N(0, 1722 + 22 + 23wX ↑ 2 UN10, 1) Sekurt # Ser E (10 11 , = x , + N(0 1)2 , = x = = X nX3 E JB T M =.. X= 5 % JB is different from normal distribution CR 5404 21. / 7 , * IBM Example : JB = 5404 21 , X 0 95. ; (2) = Chrisa INV. 10. 95 ; 2) distributed ! ↓ ↳ degrees of freedom Reject Ho - not normally 5 99. p-value = Prof [IB-Stat = Prob. (x = 5404. 21] = 1 - Prob(x 25404 25005. % ↳ Ho reject df with AR(4) would be Lung-Box-Test (LB) X 3 > another way of writing #R LB : Ho AR(1) : = AR(2) = AR(z) = 0 AR(x) = p(x) Px = x #A : At least one of AR(X) +0 Wrong Ha i+ ARk) + AR(z) + AR() + 00 X ↓ = 5% CR LB = T( +2) [Ex H x2 0. 95 (3) D 12 34. ↳ Ho 781 reject P-value = Prob[IBStat or worse] Prof = [x 2 - 12. 34] = 1 - Prof[** -123x) = 0 63. %. to end quarte - xeel of get of quarte Quiz 4 Data Stream to trading day get end of g (gives you last : - 2022e.. 31 12.. month - S in > - to change currency x (Mr)-KT > - local currency = USD $ Total RI Return Index um : n p Pl Price Index - charges prices in : but excludes dividends just for eusts here ↳ doesnt use following formules : Ret=en ( Ret = Rit or +1 - to get constituents Datastro or use in array L List LDAX PAX -all that the index ! eg for are now in =. > - time serie - > Yes ! otherwise no data timing - gives rows for what dates Members in August 2018 > LDAXINDX0818 [ to find metes at specific - liner MMYY > - VBA loop for many months ? Spitition : JB : Ho : x ~ N(. ) JB =x Ha + N( ) - : X.. & O L l X2 of ② = # Ho P P Px : K 0 Ps : = = = = =... Ha : at least one of the ps + 0 LB T(++ = 2)[ 1 - z xdf = (k) Filter · announced (Year Deals - Screener - > Deals > - M& A to Date : to Date) > - insef screen Nation aquiror Deal Valur (germany & (210m) · > almost never - normal distributed : In test ask if normally distributed Statistics Jarque-Bera test - Testing for normality ▪ The Jarque-Bera is used to test whether a variable follows a normal distribution. – In Finance, it is commonly used to test whether returns follow a normal distribution; Sˆ 2 ( Kˆ − 3) 2 JB = T + ~ ( 2) 2 6 24 – The null hypothesis is that the variable follows a normal distribution. The alternative hypothesis is that the variable does not follow a normal distribution; – The Jarque-Bera test only follows a Chi-Squared distribution for a large sample size (N > 2000);