Biostatistics and Basic Epidemiology PDF
Document Details
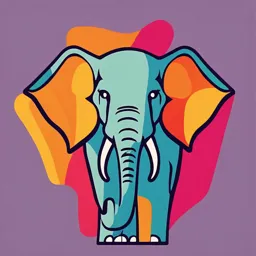
Uploaded by InestimableBandura
Kuwait University
Dr. Areej Al-Ali
Tags
Related
- Introduction to Medical Statistics 2024-25 PDF
- Introduction to Medical Statistics 2024-25 PDF
- BSPH123 Biostatistics Introduction and Descriptive Biostatistics January 2024 PDF
- DENT 1007 Biostatistics Introduction PDF
- Přednáška III. Data, jejich popis a vizualizace (PDF)
- Statistiques I - Introduction aux statistiques biomédicales - 2024-2025 - PDF
Summary
These lecture notes cover biostatistics and basic epidemiology. It includes an explanation on what medical statistics is, different quantitative and qualitative variables. The notes also discuss different data types and how to use them with graphs.
Full Transcript
03/02/2024 Phase I program Biostatistics and Basic Epidemiology Dr Areej Al-Ali MD (KU), PhD in Epidemiology and Public Health (UCL, UK) Departement of Community Medicine and Behavioral Sciences Faculty of Medicine, Kuwait University [email protected] 1 Office Hours 1st week : Tuesday & Wednesd...
03/02/2024 Phase I program Biostatistics and Basic Epidemiology Dr Areej Al-Ali MD (KU), PhD in Epidemiology and Public Health (UCL, UK) Departement of Community Medicine and Behavioral Sciences Faculty of Medicine, Kuwait University [email protected] 1 Office Hours 1st week : Tuesday & Wednesday 12:30 pm – 1:50 pm 2nd week: Tuesday & Thursday 12:30 pm – 1:50 pm 3rd week: Tuesday & Wednesday 12:30 pm – 1:50 pm 4th week: Monday & Wednesday 12:30 pm – 1:50 pm 2 1 03/02/2024 What is Medical Statistics ?? Medical statistics deals with applications of statistics to medicine and the health sciences, including epidemiology, public health, forensic medicine, and clinical research. It is the science of summarizing, collecting, presenting and interpreting data in medical practice, and using them to estimate the magnitude of associations and test hypotheses. Medical statistics is also commonly known as biostatistics 3 John Tukey (1915-2000) “The good thing about being a statistician is that you get to play in everybody’s backyard” 4 2 03/02/2024 THIS SESSION Understanding the difference between a population and a sample from that population Defining different types of variables Displaying data graphically Summarizing data numerically by mean, median and standard deviation 5 Population and Sample Data should always be collected to answer a pre-specified research question. A random sample is generally taken to make inferences about the population from which it is drawn. For example: - Is having asthma during the pre-school years associated with reduced height at age 16? 6 3 03/02/2024 Population and Sample Statistical inference is the process of making inferences from a random sample to the population from which that sample was taken. The target population is the population about which we wish to make inferences. If the sample is not random, then any inferences made may be of little or limited use. 7 Population and Sample Ideally, the objective of statistical analysis is to produce an informative summary of the available data. Sometimes it is possible to deal with an entire population’s observable quantities (e.g., census). In this case, every member of the population is measured and we can produce descriptive analysis. In most cases measuring a whole population is impossible / extremely difficult - Economic reasons: to measure many units might be very expensive - Accuracy reasons: sometimes it is better to measure very precisely some units instead of measuring very imprecisely many units. 8 4 03/02/2024 Therefore We have to use a subset of individuals (“units”) for which a measurement is available, and make inferences about the overall population (which we have not fully observed) 9 Populations vs Samples Population: theoretical concept for an entire group Parameters: quantitative features of a population (objective of inference) Estimation: results from the sample used to make inferences about the parameters Sample: taken from the population 10 5 03/02/2024 Populations vs Samples The population of those having a heart attack Has a mean age at time of heart attack And estimated the mean age of the sample population We sampled 1000 patients 11 Variables (outcomes or risk factors) A variable is any characteristics, number, or quantity that can be measured or counted. It is called a variable because the value varies between entities. It can take different values. A variable may also be called a data item. Examples of variables: age, sex, business income and expenses, country of birth, capital expenditure, class grades, eye colour and vehicle type. 12 6 03/02/2024 Imagine that ØAll males 18-60 years have same pulse rate ØAll high school students have same IQ scores. ØAll people have same immune system response. ØAll lung cancer patients have same disease stages. ØThe temperature in Kuwait every day is 50 C. ØYou eat same type of food every day But Life is So Good Because variability makes us distinct and unique. 13 Continuous variables Quantitative (numerical) Some quantitative trait. The resulting data are set of numbers on a scale. Variables (Can take any value in an interval) Examples: Age, Height, Weight Discrete variables (Values are limited to whole numbers) Examples: Number of children, Number of ER visits Binary variables (Having two categories only) Qualitative (categorical) Grouped into categories based on some qualitative trait. Ordinal categorical variables (Having more than 2 categories that can be ordered) Nominal categorical variables (Having more than two categories that can not be ordered, so arranged by names/labels) 14 7 03/02/2024 Quantitative variables 15 Continuous Variable Numeric variables that have an infinite number of values between any two values and can take any value in an interval. For example: BMI, blood pressure etc 16 8 03/02/2024 Discrete Variable Numeric variables that are limited to whole numbers. Takes on distinct, countable values. For example: Number of children, Number of ER visits etc 17 Question ! What type of variable is? Years of schooling? The body temperature of patients with the flu? Number of times a coin lands on heads after ten coin tosses? The weight of babies born at maternity hospital? Number of goals made in a soccer match? 18 9 03/02/2024 Qualitative variables 19 Binary Variables: “cases” vs. “non-cases” Persons with disease = “cases” Definition of a case is crucial and must always be specified Examples: - Deaths (yes/ no) - Disease (yes/ no) - Obesity: BMI≥30 - Hypertension: SBP≥ 140 MM Hg or DBP≥90 mm Hg - High cholestrol: ≥ 6.2 mmol/L 20 10 03/02/2024 Categorical Variables Nominal categorical variables - classification data, e.g. marital status (married, divorced, widowed, ever single) - no ordering, e.g. it makes no sense to state that married > divorced Ordinal categorical variables - ordered variables - e.g., resturant star ratings - e.g., severity of pain (none, some, a lot) - e.g., Likert scales, rank on a scale of 1-5 your degree of satisfaction 21 Question What type of variable is ? Self-rated health - Very poor, poor, average, good, very good Total cholesterol concentration Economic activity - Employed, unemployed, housewife, retired Having lung cancer or not Sex 22 11 03/02/2024 Structure of biomedical dataset In a typical biomedical study, a range of different information is collected on each participant. A typical dataset then has: - Rows (normally each participant has one row) - Columns (normally each variable has one column) 23 Example of a dataset id age sex education married weight smoking 1 56 1 2 1 88 1 2 54 2 4 2 57 2 3 53 2 1 4 63 1 4 58 2 3 2 49 1 5 49 1 2 3 79 2 6 55 1 5 4 90 1 7 56 1 3 1 89 1 8 57 2 4 1 63 1 Etc… Etc.. Etc… Etc… Etc… Etc… Etc… 24 12 03/02/2024 Displaying different types of data Using Graphs Using Summary Measures of Statistics 25 Can we work with the data in the same way? NO! Different types of data require different handling 26 13 03/02/2024 Handling Binary/ Categorical Data (Qualitative variables) Using Graphs: - Pie chart - Column chart - Bar chart Using Summary Measures of Statistics: - Frequency table: summarizes a variable with counts and percentages 27 Name the Graph! PIE CHART 28 14 03/02/2024 COLUMN CHART 29 BAR CHART 30 15 03/02/2024 Frequency Table – Gender of first year medical student, KW University Gender Frequency (N) Percent (%) Male 80 40 Female 120 60 Total 200 100 31 Frequency Table – How can we numerically summarize the type of high school that first year medical students graduated from? School type Frequency (N) Percent (%) State School 90 45 American school 35 17.5 British school 30 15 French school 5 2.5 Others 20 10 Total 180 90 32 16 03/02/2024 Handling Continuous/ Discrete Data (Quantitative variables) Using Graphs: - Histogram - Box and whisker plot Using Summary Measures of Statistics: - Measures of central tendency/ location (e.g., mean, median) - Measures of spread (e.g., standard deviation, variance) 33 Name the Graph! HISTOGRAM 34 17 03/02/2024 In the histogram above, intervals of equal width are presented on the x-axis and rectangles with heights which are proportional to the frequencies are erected on the y-axis. The frequency polygon is drawn by joining the intervals midpoints at the tops of the histogram's rectangles. The frequency curve is obtained by smoothening the frequency polygon after increasing the sample size and the number of intervals. 35 Shapes of Frequency Distribution Curves If a distribution is symmetrical and bellshaped with thin tails, it is said to have a Normal Distribution Positively skewed or skewed to the right Negatively skewed or skewed to the left 36 18 03/02/2024 Shapes of Frequency Distribution Curves Skewness is a measure of the lack of symmetry in a distribution. A normal distribution has a skewness coefficient value of zero, a positively skewed distribution has a positive skewness value, and a negatively skewed distribution has a negative skewness value. Kurtosis is defined as a measure of the degree of peakedness in the distribution. A normal distribution has a value near zero, flat distributions have a negative value, and peaked distributions have a positive value. 37 38 19 03/02/2024 Name the Graph! BOX AND WHISKER PLOT It uses the median, quartiles, and maximum and minimum values as a convenient summary of a frequency distribution. Very good to investigate shape of the distribution and outliers 39 Outliers or extreme values Outliers are data points that differ significantly from other observations in the data set. These values may be real observations from individuals with extreme measurement of the variable, e.g. a weight of 150 kg in a high school students’ sample. They may also result from typing errors. If outliers are real values, they shouldn’t be discarded. 40 20 03/02/2024 Quartiles and Percentiles Quartiles divided the population into 4 equally sized groups: - Q1 = 1st quartile = P25 - Q2 = 2nd quartile = P50 = Median - Q3 = 3rd quartile = P75 - Q4 = 4th quartile = P100 For example Q1 = The value that ¼ of the data points are less than Q3 = The value that ¾ of the data points are less than 41 Quartiles and Percentiles Percentiles are values below which a percentage of data falls. For example - P25 = 25% percentile= the value that 25% of the population is less than. - P97 = 97% percentile= the value that 97% of the population is less than. NB: Q1 and Q3 are sometimes referred to respectively as “25% and 75% percentiles” 42 21 03/02/2024 BOX AND WHISKER PLOT 43 Question ! Vicky scored 75% on a test and her score was at the 40th percentiles Which is true? A. 75% of the students did better than her. B. 40% of the students scored lower than 75% on the test. C. 60% of student scored at least as well as her or better. 44 22 03/02/2024 45 Interpretation of histograms and box and whisker plots Symmetric distribution Skewed Distribution Positive Negative 45 Description of quantitative data Suppose we observe data on weight for 25 individuals. This is all the information that is available from our data. Even with just 25 data points, it can be complex to make sense of the numbers: what do we see in them? Data description Extract main data features and pattern - Central Tendency/ Location - Spread - Shape - Exceptions to general pattern (ie outliers) Summarise the essential information contained in the data Present the results informatively 46 23 03/02/2024 Description of quantitative data Summary statistics Generally, we indicate the data points as (x 1, x 2, x 3 , …, x N ), where N is the size of our population. Sometimes, we only observe a sample of size n from the population, which we indicate as (x 1, x 2, x 3 , …, x n ). Especially when the data size is large it is very difficult to make sense of the data. Our objective is to calculate simple numbers that we can then use to describe the entire distribution of the data. 47 Description of quantitative data Measures of Central Tendency/ Location Mean Median Mode 48 24 03/02/2024 Description of quantitative data Measures of Central Tendency/ Location- (1) Mean Population mean 𝜇= = !!"!""⋯"!# $ &'&() '* +'+,)(&-'%.(&( /(),0 +'+,)(&-'% 1-20 Sample mean 𝜒̅ = = !!"!""⋯"!$ % &'&() '* 1(3+)0.(&( /(),01 1(3+)0 1-20 Every measure can be defined both at the population and at the sample level. To make the distinction clear we use different symbols. The mean can be considered as a single, “average”, representation of the values in the data. 49 Description of quantitative data Measures of Central Tendency/ Location- (1) Mean The mean is the point that makes the distribution of data “balance” 50 25 03/02/2024 Description of quantitative data Measures of Central Tendency/ Location- (1) Mean EXAMPLE 1: The following represents weights in Kgs for 10 children in the 7th grade: 68, 63, 42, 37, 30, 36, 28, 32, 79, 47 The sample mean is given by 𝜒= ̅ 45"46"78"69"6:"64"85"68"9;"79 𝜒= ̅ " 𝜎= 𝝁= population mean $ N= population size = ∑$ 𝔦"# 𝒳𝔦=> " $ 66 33 03/02/2024 𝜒1 − 𝜇 2 + ( 𝜒2 − 𝜇 2 + ⋯ + 𝜒𝑁 − 𝜇 2 𝜎= !!=> ""( !"=> ""⋯" !#=> " $ = ∑$ 𝔦"# 𝒳𝔦=> " $ 67 $ ! 𝜒𝑖 𝒾"# $ 1 𝜇 = ! 𝜒𝑖 𝑁 𝒾"# Question: What does it mean if one of the values for the deviations from the mean is very high? $ ! 𝜒𝑖 − 𝜇 𝒾"# 2 𝜎= ∑$ 𝔦"# 𝒳𝔦 − 𝜇 𝑁 2 68 34 03/02/2024 Answer: The value 1849 is considered an extreme value or an outlier for this data set. Standard deviation is sensitive to outliers or extreme values (Not Robust). 69 Standard deviation – interpretation A large standard deviation indicates that the data points are far from the mean and small standard deviation indicates that they are clustered closely around the mean. For example, each of the three data sets (0,0,14,14), (0,6,8,14) and (6,6,8,8) has a mean of 7. Their standard deviations are 7,5, and 1, respectively. The third set has a much smaller standard deviation that the other two because its value are all close to 7. NB The standard deviation is zero only if there is no spread, i.e. all observations are identical. 70 35 03/02/2024 Description of quantitative data Measures of Spread- (3) Standard deviation (sample) We can define standard deviation also at the sample level. In this case, we label it as !!=B ! ""( !"=B ! ""⋯" !$=B ! " Sx= standard deviation Sx = %=< ( = sample mean 𝝌 n= sample size = ∑$ ! 𝔦"# 𝒳𝔦=B " %=< Instead of the population mean, we use the sample mean We divide for (n-1), the size of the sample minus 1 This is to have a better estimate of the population value 71 Description of quantitative data Measures of Spread- (4) Variance The square of the standard deviation is called the variance and indicated by 𝝈2 (for population) or s2 (for sample). Its interpretation is essentially the same as for standard deviation: It represents a measure of how dispersed (i.e. how variable) the data are The more scattered the values, the higher is the variance Question - Can a variance be a negative number? - Does a large value for a variance mean low or high variability? 72 36 03/02/2024 Exercises 73 Exercise 1: In a review article on myelodysplastic syndromes, the age distribution of patients presenting in one town was shown as follows: 1. What kind of diagram is this? 2. How would you describe the shape of the distribution? 74 37 03/02/2024 Exercise 2: In a study of blood pressure in one town, the diastolic blood pressure among men was as shown as follows: 75, 89, 90, 80, 80 and 115 1. Can you compute the sample diastolic blood pressure mean? 2. Can you compute the sample diastolic blood pressure mode? 75 Exercise 3: Suppose during diabetes awareness month, you randomly selected 10 children to check for their HbA1c (normal range:4.5 – 5.7) to screen for diabetes in children. The HbA1c were 5.2 , 4.5, 5.7, 4.7, 4.8, 18, 4.9, 5, 5.5, 5.9 1. Compute the mean? 2. Compute the median? 3. Which parameter better reflects the data? 76 38 03/02/2024 Exercise 4: In a study of the use of alternative medicines, households were randomly selected from country centres in South Australia. Three thousand and four people were interviewed; 48.5% of respondents used at least one non-prescribed alternative medicine. The estimated monthly cost for users of alternative medicines ranged from $1 to $500, median $10. 1. What is meant by ranged from $1 to $500, median $10 and what does this tell us about the shape of the distribution of expenditure? 2. If all respondents were included in the distribution of monthly cost, what would the median be? 77 Exercise 5: Infants who died from sudden infant death syndrome were compared to a group of live infants, matched for age and birth-weight. The temperature in the baby’s bedroom and the amount of thermal insulation (clothes and bedding) were measured, to give an estimate of the excess thermal insulation. The dead children had had more excess thermal insulation (mean 2.3, standard deviation 3.4) than the live children (mean 0.6, standard deviation 2.3). What is meant by mean and standard deviation? 78 39 03/02/2024 Exercise 6: The owner of the portland hospital is interested in how much people spend at the hospital’s cafeteria. He examines 10 randomly selected receipts and writes down the following data. 44, 50, 38, 96, 42, 47, 40, 39, 46, 50 Can you calculate the standard deviation and variance? 79 Thank you 80 40