FINALS_PSY 4318 PDF - Between-Subjects Factorial Designs
Document Details
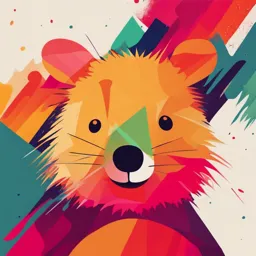
Uploaded by SolidVerisimilitude2838
University of Santo Tomas
Tags
Summary
This document provides an overview of between-subjects factorial designs in psychology research. It details main effects, interaction effects, and statistical analysis of such designs. Keywords to search for include 'factorial designs' and 'research methods'.
Full Transcript
BETWEEN-SUBJECTS FACTORIAL DESIGNS Different groups of subjects participate in the different treatment conditions of the experiment. The experiment has more than one IV Experiments with multiple IVs are efficient, provide more information = preferred by most experimental psychologists...
BETWEEN-SUBJECTS FACTORIAL DESIGNS Different groups of subjects participate in the different treatment conditions of the experiment. The experiment has more than one IV Experiments with multiple IVs are efficient, provide more information = preferred by most experimental psychologists IV = factors ○ Each factor will have 2 or more levels ○ Two-factor experiment = simplest factorial design The results we get from a factorial experiment gives us two kinds of information Main effects - information about the effects of each IV in the experiment Looking for Main effects Main effects ○ the action of a single IV in an experiment ○ A change in behavior associated with a change in the value of single IV within the experiment ○ Kung gaano kadami yung factors (IV), ganon din kadami ang main effect ○ But not all main effects may be important enough to be statistically significant ○ Statistical test: ANOVA When we compute statistical tests for factorial experiments, we first evaluate the separate impact of each IV in the experiment. It-test if each main effect is statistically significant or not. ○ Statistical tests can also tell us whether the two factors operate independently or not. CHATGPT The difference between main effects and interaction effects in statistics and research studies is based on how variables influence an outcome: 1. Main effects: This refers to the direct, individual impact of an independent variable (IV) on the dependent variable (DV). It examines whether a single variable has a statistically significant effect, regardless of other variables. ○ Example: In a study on exercise and diet's impact on weight loss, the main effect of exercise would show whether exercise alone affects weight loss. 2. Interaction effects: This occurs when the effect of one independent variable on the dependent variable changes depending on the level of another independent variable. In other words, it shows how two or more variables work together to influence the outcome. ○ Example: In the same study, an interaction effect might occur if the impact of exercise on weight loss differs based on the type of diet a person follows. The combination of exercise and diet could lead to different results than either variable alone. In summary: Main effects look at the separate influence of each variable. Interaction effects look at how variables influence each other in combination. In a line graph: Main effect: If you see parallel lines, it suggests there is no interaction, meaning each variable has a similar effect on the dependent variable across conditions. Interaction effect: If the lines are non-parallel (e.g., they cross or diverge), it indicates an interaction, meaning the effect of one variable depends on the level of another variable. How many subjects? Very similar samples = small samples Individuals different = larger sample Larger sample = more likely to mirror the actual state of the population Slightly different responses = individual differences Note: we are not interested in the performance of individual participants in the experiment; we know that individual scores differ. Effect size: a statistical estimate of the size or magnitude of the treatment effect. ○ The larger the effect size = the fewer participants needed to detect a treatment effect. ○ Power charts = used to illustrate the relationship between factors or variables It is advisable to have at least 20 participants in each treatment group, some 30 Smaller sample = harder to detect effect size Two-factor experiment - simplest factorial design Gives us information about the effects of each IV (main effects) How does the influence of one IV affect the influence of another IV (interaction effects) Looking for Interactions When the effects of one factor depend on another factor If the effect one IV changes across the levels of another IV If there is an interaction, we cannot get a complete picture of the results of the experiment without considering both factors → the effects of one factor will change depending on the levels of the other IV. Pag may interaction between IV din, magiiba din yung main effects ng isang IV. Interaction effect = can also tell us there could be limits or exceptions to the main effects of one or more factors. = an interaction qualifies the main effects. If main effects are independent = no interaction Number of possible interactions = depends on the number of IV in the experiment ; TWO IV = ONE INTERACTION [chatgpt] 1. How many interaction effects are there if there is 4 IVs? The number of interaction effects depends on the number of independent variables (IVs) and how you combine them. If you have 4 IVs (let's call them A, B, C, and D), you can have different levels of interactions: 1. Two-way interactions (involving 2 IVs at a time): A×B A×C A×D B×C B×D C×D Total two-way interactions = 6 2. Three-way interactions (involving 3 IVs at a time): A×B×C A×B×D A×C×D B×C×D Total three-way interactions = 4 3. Four-way interaction (involving all 4 IVs): A×B×C×D Total four-way interaction = 1 Total number of interaction effects: Add them all together: 6 (two-way) + 4 (three-way) + 1 (four-way) = 11 interaction effects. Higher-order interactions = involves more than one interaction at a time. Shorthand notation - tells us several things about the experiment (ex. 2x2. 3x2) Factor Labeling Methods The names of each factor are placed in parentheses following the numerical notations. ○ Ex. 2x2(type of name x length of name) between subjects factorial design ○ Ex. 2 (type of name) x 2 (length of name) between subjects factorial design ○ Ex. 2 x 2 (Type of name: given, nickname x Length of Name: short, long) between-subjects factorial design ○ Ex. 2 (given name or nickname) x 2 (short or long name) between subjects factorial design Understanding Effects from Factorial Design No significant main effects or interaction Significant main effect for an IV A significant interaction effect A maximum significant interaction effect (cross interaction) A significant main effect and interaction effect Significant main effect for the second IV Two significant main effects It might be tempting to do everything in one experiment. Try to keep your first experiment as simple as possible. ○ Focus on the variables that are critical Why do we need to keep a factorial experiment simple? ○ You have to select many participants. ○ More treatment conditions = more time ○ Results can be complicated → may be uninterpretable There is no point in collecting data we cannot understand. In a two factor analysis there are only 3 possible outcomes: ○ A main effect for each factor ○ An interaction between two factors EXAMPLE 3 x 2 x 4 Between Factorial Design 3 factors Factor A = 3 levels Factor B = 2 levels Factor C = 4 levels Main effects = 3 Interaction effects = 4 interaction effects How many possible outcomes in all = 7 possible outcomes ○ AXB ○ AXC ○ BXC ○ AXBXC ○ A main effect ○ B main effect ○ C main effect How many groups or levels = 24 treatment conditions Statistical analysis = ANOVA