Research Methods Cheat Sheet PDF
Document Details
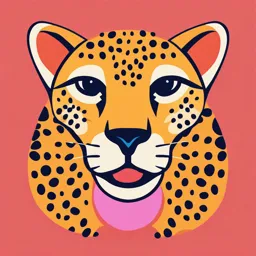
Uploaded by AdoredKangaroo8278
Tags
Summary
This document outlines research methods, including non-experimental and quasi-experimental designs. It covers topics such as correlational studies, interrupted time series, and factorial designs. Key concepts like independent and dependent variables are also discussed.
Full Transcript
Non-experimental & Quasi-experiment: Non-experimental research: useful when it is impossible to manipulate variables, correlational studies: correlational evidence can be useful (be careful about drawing correct conclusion), correlational studies lack internal validity, still satisfy statistical, ex...
Non-experimental & Quasi-experiment: Non-experimental research: useful when it is impossible to manipulate variables, correlational studies: correlational evidence can be useful (be careful about drawing correct conclusion), correlational studies lack internal validity, still satisfy statistical, external, and construct validity, continuous variables, quasi-experiments: studies resembling experiments where IV is implemented, but either a control group is missing or participants are not randomly assigned to conditions, categorical variables, time-lagged eliminates bidirectionality, but not other confounders, medium internal validity, One group post-test design: treatment is implemented and DV measured afterwards in one group, no comparison group, only one level, weakest quasi-experimental design, one group pre/post test: DV measured before implementation and again after implementation in one group, external explanations still threaten internal validity, can’t use control group bc it is one group design, Interrupted time series: set of measurements taken at intervals over a period of time that is interrupted by a treatment, like pre/post test but includes multiple periods of measurement, see trends over time, also hard to rule out alternative explanations, nonequivalent groups design: a between-subjects design in which participants have not been randomly assigned to conditions, posttest only nonequivalent groups design: participants in one group are exposed to a treatment, a nonequivalent group is not exposed to the treatment, and then the two groups are compared, pretest-posttest nonequivalent groups design: reatment group that is given a pretest, receives a treatment, and then is given a posttest. But at the same time there is a nonequivalent control group that is given a pretest, does not receive the treatment, and then is given a posttest, interrupted time-series design with nonequivalent groups: aking a set of measurements at intervals over a period of time both before and after an intervention of interest in two or more nonequivalent groups, pretest-posttest design with switching replication design: nonequivalent groups given pretest, one group receives treatment while other does not, DV measured, other group get treatment while original group continues with treatment, DV measured again, built in replication and controls for time effects, switching replication with treatment removal design: first group gets treatment, Measure DV, second group gets treatment while first group stops, measure DV over time, Comparison groups: should be the same except for the IV Behavioral observation: Naturalistic observation: observing behaviors without interference where they organically occur, can be disguised or undisguised, Participant reactivity: participants behave unnaturally because of awareness in observation, Participant observation: observing behaviors by emerging oneself into the world where they naturally occur, researchers become active participants in the group or situation they are studying structured observation: observing behaviors with parameters established by researcher, may or may not be in natural environment, field experiment: manipulating a variable in a naturalistic setting before making observations or measuring outcomes, coding scheme: organized system of relevant categories and examples used to identify and document occurrences of behaviors, need inter-rater reliability, content analysis: a type of coding system in which researchers specify keywords, phrases, or ideas and then find occurrences of them in a corpus Factorial design: a type of experiment with more than one independent variable that allows us to explore the interplay between them, can combine experimental and non-experimental designs, Factor: each independent variable, must have at least two levels, total number of conditions in the study is found by multiplying the number of conditions in each factor, cell: a unique combination of oe level of one IV with one level of another IV, important for determining sample size, Interaction: when 2 or more variables collectively affect an outcome or dependent variable, moderates the association between another variable and the outcome, Comparison groups: every participant is assigned to a specific cell but we don’t compare each cell directly, we break our results down into component by variable, types of relationships: main effect of IV #1, main effect of IV #2, interaction effect (how each of the IVs interact with each other to affect the outcome), interaction effects are independent from main effects, Main effects: effect of one independent variable on the dependent variable, Types of interactions: with interactions the effect of either predictor or the outcome depends on the level of another, differences in differences, synergistic interaction: effect of IV #1 is reduced or magnified after including IV #2, Buffered interaction: effect of IV #1 is eliminated after including IV #2, Crossover interaction: Effect of IV #1 is reversed after including IV #2, which main effect are present: average is equal= no main effects, parallel = no interaction, mixed-factorial design: combine within subjects and between-subjects approaches in the same study, at least 1 between-studies IV and 1 within-subjects IV, Simple effects: the association between an IV and the outcome isolated at only one level of another IV, must be conducted if an interaction is found to fully investigate the interaction, they tell us where the action is Case studies: Qualitative design: research that involves non-experimental and non-numerical methods and analytical techniques, less structured as participants lead data collection, great for generating future research questions, more in-depth info with fewer people, often used with hard-to-reach populations, uses descriptive rather than analytical approach, Interviews: unstructured, structured, semi-structured, surveys: open-ended, free response questions, focus groups: interviews with a group of participants, participants may influence other’s responses, get more responses, addresses limitations in surveys, Grounded theory: start with the data, reach theoretical ideas stemming from the data, Theoretical narratives: identify themes that emerge from responses, focus on repeating “big ideas” from participant responses, Mixed Methods: approach using a combination of methods, good for generating research questions, then following up with rigorous hypothesis testing, Case studies: an in-depth, qualitative approach investigation into a single individual or group, might be longitudinal, ex: phineas gage with post accident recovery, jean piaget with stages of cognitive development in his own children, washoe with teaching a non-human asl, proves existence, lots of details, key insights not available through experiments, can inspire new research, lack internal, external, statistical, and construct validity, cannot act as evidence for theories, Small N design: involves studying in detail the behavior of each of a small number of participants, more emphasis on control and gathering structured data, more quantitative, focus on applications of research to achieve an end that is applicable to the individual or group, involve experimental control and manipulated variables, High internal validity, cant satisfy statiscal validity, visual inspections are subjective, cant compare across studies, lack external validity, applied behavioral analysis: branch of experimental psychology that explores how rewards, punishments, and other factors affect behavior, ex is pavlov’s dogs, Reversal (ABA) Design: a single-subject longitudinal experimental design in which a change is made following baseline, and then reversed, Multiple treatment reversal designs: a baseline phase is followed by separate phases in which different treatments are introduced, Alternating treatments: two or more treatments are alternated relatively quickly on a regular schedule, Multiple baseline design: when you can’t return to baseline, research method that evaluates the effect of a treatment on a behavior, person, or setting by measuring it multiple times, across participants: a baseline is established for each of several participants, and the treatment is then introduced for each one, Using several participants, measure baselines and then stagger the time at which the treatment is introduced, across behaviors: multiple baselines are established for the same participant but for different dependent variables, and the treatment is introduced at a different time for each dependent variable, across settings: multiple baselines are established for the same participant but in different settings, Level: comparison in DV across the treatment-baseline, Trend: actual change in the outcome, Latency: how far apart the trend is from the level, Percentage of non-overlapping data, Principle of converging evidence: If our ideas are strong, we should see evidence for them in different research contexts, Applied psychology: a branch of psychology research with a narrower scope dedicated to achieving specific outcomes in individuals/groups which are less generalizable, Some ideas & methods stem from established scientific theories, but specific solutions may be novel and require testing, archival research: a method of analyzing data that has already been collected, data could be qualitative or quantitative, may need coding if quantitative Describing data: before we can test our hypothesis we need to plot and summarize our data first, plotting usually involves succinct visualizations, Data: recordings of operationalized variables often stored in a numerical and labeled format usually in a spreadsheet, our data looks different depending on the scale of measurement, categorical data: data measured in a nominal or ordinal scale, ex: grades, presented with frequency tables, bar graphs, Continuous data: data measured on an interval or ratio scale, ex: numerical grades, can use frequency scale if subdivided into categories, histograms, Central tendency: a distribution is its middle—the point around which the scores in the distribution tend to cluster, mode, mean, and median, mode: the most frequent occurring value in the data, can be calculated with any scale of measurement, bimodal (two modes) or multimodal (multiple modes) are sometimes present, median: the value that segments a variable into the upper and lower half of values, 50th percentile, not usable for nominal data, splits the data based on the quantity of values, mean: arithmetic average of the values, only calculable with interval or ratio data only, sum of all values divided by number of values, value where the total distance of the scores below it equals the total distance of those above it, balancing point for the data, spread: the extent to which the scores vary around their central tendency, range: difference from high to low, can be misleading if the data is highly skewed, IQR: 75th-25th percentiles, not thrown off by extreme outliers, but ignores half of the data, variation: departures from the center, looking at the deviation from the mean can be helpful in understanding the spread of the data but combining all the deviations would result in zero, we square each deviation first and average the squared deviations to overcome this, we take the square root of this to find the average or standard deviation, Distribution: a way of quantifying the probability of certain values to appear in a dataset based on central tendency and variability, normal distribution: a probability distribution that is symmetric around the mean, showing that data near the mean are more frequent in occurrence than data far from the mean in either direction, bell curve shape, Skewness: asymmetry in the distribution of data which runs either positive (right-tailed) or negative (left-tailed), describing data: Central tendency and variability go hand in hand. Variability can sometimes guide which measure of central tendency we should use to characterize the data, When data are distributed normally, the mean, median, and mode are virtually identical. Can use any of these, When data are skewed, the mean gets distorted. In this case, best to use the median, capture information about a single variable with bar graphs, histograms, frequency tables, boxplots, etc. This helps us evaluate frequency claims, But for association and causal claims , we also need to be able to say something about the relationships between multiple variables, Scatterplots: visualizations that depict data from 2+ variables simultaneously, continuous variables, best fit lines to depict general trend of association between these variables, strength and direction of the linear association between these variables, important to plot first before trying to establish a relationship, failure to do this may lead to bias that hurts statistical validity, Artefacts: aspects of data that may mislead or throw off clear conclusions about the nature of the associations among variables, outliers: a type of artifact manifesting as an extreme/improbable datapoint, Restrict Range: a type of artifact manifesting as an artificially constricted set of data points (narrower than what we would expect to see in the world), Non-linear trends: a type of artifact manifesting as a curvilinear association between variables, third variable problem: a type of artifact that emerges when an association between two variables can be explained away by the presence of a third variable that is related to each of the other two, Effect Sizes: a value measuring the strength of the relationship between two variables in a population, Whether a statistical association exists is distinct from the magnitude of the association, effect size claims: “small change,” “large correlation”, In many cases, scientists and journalists overclaim by stating a statistical result as if it’s much larger than it really is, That’s why we need to distinguish association/causal claims from effect size claims Experimental Design: Independent variable: what we change/manipulate, must have more than 1 level, comparison groups: categorical levels of the IV manipulated by researchers, DV measured to compare, not the same as control, Dependent variable: what we are measuring, Control: variables that are unchanged and held constant, conditions: more than 2 min, more detailed conditions=more data required, Random Assignment: method to place P’s into experimental groups in unbiased way, crucial in experimental design, P’s must have equal chance to each condition, P’s assignment is independent to other P’s, Block Randomization: all conditions occur once in a sequence before any of them are repeated, Matched-group randomization: P’s matched on traits, 2 by 2, descending down full list of traits, Types of conditions: Treatment and control, Control groups: no stimulus at akk, similar stimulus, standard treatment, waitlist group, placebo group, Randomized control trial: study includes at least one treatment and one control with random assignment, Placebo: Inert variant of a substance that resembles but also lacks the material of the treatment, Placebo effect: any unwanted effect of the inert substance on P’s, driven by power of suggestion, possible explanations: the placebo really works, people got better on their on, naturally occurring fluctuations over time, regression to the mean, doing something gives people a boost, selection efforts, Regression to the mean: when you got people experiencing extreme moments , the next moments will be less extreme, Nocebo effect: absence of what is believed to be harmful, Confounds: Reasonable alternative factors or explanation that can account for obstructed study results, extraneous variable that varie systemically with IV (i.e. race. IQ), solution is random assingment, Noise: extraneous variable that do not systematically vary across conditions but nevertheless makes it difficult to see study signal (i.e. someone’s gf broke up with them, Aced a test), can minimize but it is inevitable, Between subjects research: each participant only tests in one condition, Within subjects research: each participant experiences all conditions, still uses randomization to assign order of conditions, P’s serve as their own control, risk of order effects, Order effects: confounds that occur when DV is impacted by the order of conditions, Carry-over effects: order effects where the condition of a certain condition is still experienced on the DV that came after subsequent condition, Practice effects: order effects where increased experience with DV improves performance regardless of condition, Contrast effects: order effects where experience in conditions impacts perception of the study (reveal study hypothesis), Counterbalancing: offsetting order effects by creating different orders of experimental conditions and presenting orders randomly, Full counterbalance: equal number of P’s complete each possible order of conditions, Partial (random) counterbalancing: order of conditions randomly determined for each participant, Latin square design: every condition appears in each position once, achieved by listing conditions in vertical order, starting next condition in each column, Internal validity: does our experiment measure what we want to measure, External validity: can we generalize results to population beyond the study, Construct validity: do the variables we measure capture real psych phenomena constantly and accurately, Statsictal validity: did we draw approproae conclusions from the data setthrough statistical procedures, Standardization: controlling for extraneous variables, Demand characteristics: features of a study that may alert P’s to study goal or differences among conditions, Pygmalion Effects: when P’s behaviors are influenced by researcher expectations, double-blind study: both the researcher and P do not know what condition they are in/study hypothesis Non-experimental Design: true experiments are not always feasible or ethical, non-experimental design: we only measure variables rather than manipulating them, cant conclude casualty, spurious correlations: a coincidental association between 2 variables that is unlikely to replicate or generalize, correlation evidence: does not necessarily mean causation because several equally plausible alt explanations, x -> y, y -> x (reverse causality) , x -> y AND y -> x (bidirectional causality), z-> y AND z -> x (third variable causation), alt explanations threaten internal validity, Moderation: not all associations are understood by examining direct connections, some occur under certain conditions and when other variables are at a particular level, when the relationship between 2 variables depend on the level of a third variable, special type of association claims, conditional association, valuable tool for looking closer at relationship between predictor and outcome Open science: false positive: the study falsely detected evidence for an effect by chance, False negative: the study falsely failed to detect evidence for an effect by chance, critical difference in the original and replication methodology that accounts for the difference, Reproducibility: a characteristic of research that is achieved when independent scientists arrive at the same results and conclusions using their own data and methods, Access to study dataset & code to re-run analyses, Access to full study materials to run the study with an independent sample, replicability: when a study result can be found by another research team independently, using either the same or different methods, direct replication: repeat the study using the same methods, address the replicability of a specific study result, Relevant to internal & statistical conclusion validity, conceptual replication: repeat the study using different methods, address the replicability of a general idea (hypothesis or theory), Relevant to external & construct validity, Critiques of replications: Conceptual replications are normal part of the scientific process, so a failed replication with different methods doesn’t show that the theory is wrong. It could just be a methods issue, Direct replications only address results from one specific study. So who really cares about that? One study doesn’t tell us much anyway, The Reproducibility Project: Large research team selected 100 papers published in 2008 and tried to reproduce the key result using direct replications, only replicated half the research, Metrics of reproducibility: Significant vs. non-significant relationship between variables. Relevant to association & causal claims, Confidence intervals. Also relevant to association & causal claims, Effect size (small, medium, large). Relevant to effect size claims, Questionable research practices (QRPs): shortcuts used by researchers which distort study findings and threaten replicability, P-hacking & “stopping rules”, Selective reporting,Misleading conclusions, Not sharing materials & data, social priming studies are hard to replicate, experiments have low replication compared to correlational studies due to academic pressures to come up with flashy and clever experiments which leads to wacky ideas and cutting corners, Data sleuth: Gigantic effect size for a counterintuitive result, The small telescopes analogy, inconsistent statistical decisions across studies, Publication bias: when positive results are more likely to get published compared to null results, thus distorting our theories (AKA “ the file drawer effect ”), Open science: a movement towards healthier scientific norms & practices, including transparency and accountability, Pre-registration – holds researchers accountable and makes the scientific process transparent, Open materials & data - increases transparency and the ease of conducting replication studies, Publishing null findings - gives us a more accurate perception of a research field Intro: How do we know things= Repetition: we memorize things we hear repeatedly, Authority: we trust things from others, Experience: we know things we experienced directly, Reason and Logic (Rationalism): we infer info based on other types of info, often plagued with biases. Empirical: basis for scientific method gathering info from observation and experience. 3 Factors of Scientific Method: systematic empiricism: learning based on carefully planned, recording, and analyzing observations, Empirical questions: questions about how the world works that can be observed by systematically observing it, public knowledge: large scale collaboration, test and correct errors. Goals of science: describe, predict, and explain. Describe: what are symptoms of mental illness, predict: who is more likely to suffer, explain: what theory helps us understand. What makes a good research question: is it interesting/will people care, is it useful, is it feasible. Theories: broad, lots of evidence, explanation to a set of phenomena, Hypothesis: specific and have yet to be tested, new phenomena, falsifiable and testable, logical informed by previous research, positive existence of something rather than absence. Descriptive research: what is the divorce rate, Analytic research: what variables might explain divorce, Descriptive Stats: organize or summarize set of data (mean, median, etc). Inferential Data: Draw conclusions about a population based on a sample (statistical sig, type 1=false positive, type 2= false negative), Anchoring: skewed decision making based off reference point