Study Notes on Statistical Analysis PDF
Document Details
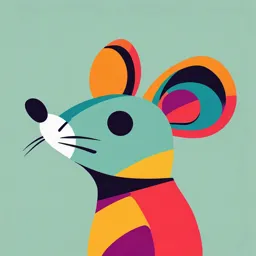
Uploaded by TidyAndradite1584
York University
Tags
Related
Summary
These notes cover various statistical analysis techniques, including correlation, regression, and factor analysis. The material explores different methods and their applications in several examples. Key concepts and assumptions for each method are discussed.
Full Transcript
1.1 State the null hypothesis. 1.2 Choose an alpha level. 1.3 Decide whether to reject or fail to reject the null hypothesis (explain why). hp 5 C Correlation Analysis: variation in scores on one variable corresponds to variation in scores on 2nd v (causation)...
1.1 State the null hypothesis. 1.2 Choose an alpha level. 1.3 Decide whether to reject or fail to reject the null hypothesis (explain why). hp 5 C Correlation Analysis: variation in scores on one variable corresponds to variation in scores on 2nd v (causation) - Correlation coefficients “r”:computed to know how2 variables are related to each other (coefficient of determination = r^2) Types: 1. Point-biserial coefficient:one variable is continuous and other is 2 level categorical/ dichotomous Ex. if one’s driving written test scores are correlated w/ owning a car 2. Spearman Rho Coefficient:both variables used ranked data 3. Phi coefficient:both variables are dichotomous (opposite) Ex. if gender is associated w/ one smoking cigarettes 4. P earson product-moment correlation coefficient:both variables measuredon interval/ ratio scales(Ex. students IQ scores correlated w// final grades) ISSUES: a. Both variables must becontinuous b. Designed to examinelinearrelations c. When relationship between 2 variables iscurvilinear, it suggests a weaker relationship than may actually exist) d. Truncated range:not much variety in distribution of scores due to ceiling/ floor effect (ex. very easy test) egression analysis:Examines nature & strength of relationship between variables /the R relative predictive power(can’t claim causation) - Simple regression:involves a single independent & single dependent variable - Multiple regression:has 2 or more independent variables & a single dependent variable - Standardised regression coefficients:Used to convert the unstandardized coefficients into coefficients w/ the same scale of measurement - Key assumptions for regression analysis: a. There’s a linear relationship between predictor & dependent variables b. Dependent v should be measured on an interval/ ratio scale c. Dependent v should be normally distributed d. Predictor variables are not too strongly correlated w/ each other (too high variance) hp 6 C Factor Analysis:Uses multiple scales to representa single underlying construct (organises items into constructs) - Exploratory factor analysis (EFA):performed to studya multifaceted construct& how many facets/ factorsthe survey items likely represent - Confirmatory factor analysis (CFA):performed to knowhow well survey itemsrep a given set of facetsassociated with a multifaceted construct nobserved (latent variables):construct that survey items are supposed to rep/ measure U (ex. Employee satisfaction, performance, personalities) bserved variables:survey items that actually measure a construct (ex. 4 items for O measuring satisfaction) Factor Extraction:EFA process involving extracting factors from a set of items Factor Rotation:procedure to rotate factors tomaximise distinction between them actor loadings:are comparable/ analogous to correlation coefficients & range from -1.0 to F 1.0 eliability Analysis:Indication of the stability of a set of measurements over repeated R applications of the measurement procedure (how well each construct holds together) eliability coefficients:degree that observed scores correlate with one another, indicates R how well the groups of items hold together hp 7 C Person-centred analysis:cases in a sample are divided into groups according to their characteristics on multiple variables luster Analysis:divides a sample into diff groups based on their similarity on a number of C clustering variables ex. Employee age, education, gender Steps: 1. Select variables used to create clusters 2. Chose method of clustering data (K-means or Hierarchical clusters) 3. Identify list of clusters 4. Perform one-way ANOVA to compare diff clusters on each research variable OR run regression analyses w/in each cluster on the associations between independent and dependent variables atent Class Analysis:A combination of factor and cluster analysis, In contrast to factor L and cluster analysis, LCA uses clustering variables that are measured ona categorical or nominal scale, (T or F, subject studied)NOT interval-ratio data hp 8 C Data-informed decisions:Use data & technology to inform decision making HR Analytics - process of analysing ppl-related quantitative/ qualitative data for the purpose of decision making, achieving goals & sustaining competitive advantage ystems thinking:looks at the fit of all HR pieces and how to address any misalignment in S HR practices - Ex. The ability motivation opportunity model (Performance = ability x motivation x opportunity) Strategy Formulation STEPS: 1. Create mission, vision & values(rules) 2. Analyse internal & external environments 3. Pick strategy type based on Micheal Porter’s strategies(differentiation, cost leadership or focus) 4. Define objectives to satisfy stakeholders 5. Finalise strategy The scientific process:framework to collect, analyse & interpret data hp 9 C HR Information system (HRIS):Steps - store, manipulate, analyse, retrieve & distribute info about organisation’s HR - Steps to develop 1. Initial assessment(gain buy-in from stakeholders) 2. Assess organisational needs & project parameters 3. Evaluate available platforms 4. Design system 5. Choose a vendor(send request for proposal to potential vendors, review proposals, invite 2 or 3 to demonstrate, choose one & finalise contract) - Pros: a. Track employee life cycle to predict future and gain competitive advantage . A b utomated, employee-centered HR functionality c. Data availability for metrics & analytics to fix problems d. Data visualisations made to better understand findings - Cons: a. High money, time cost to become a data-driven culture b. Lack of analytics skills in traditional HR skill sets c. Data privacy & security concerns (anonymous data needed, confidentiality, personally identifiable data) - Lewin’s Model of Change: a. Unfreeze(employees ready for change) b. Change(execute intended change) c. Refreeze(ensure changeis permanent) lectronic HRM (e-HRM):internet based info system that spans across firm levels (ex. E e-recruiting) Enterprise resource planning/ ERP: a. system that has people information b. Creates data ecosystem enabling firm stakeholders to take a systems perspective when making decisions c. Can improve decision making across diff functional areas like HRM hp 10 C Database management system (DBMS):software used to manage & maintain a/ mulitple databases elational database:specific database w/ diff subsets/ collections of data are integrated R through info residing w/in the data themselves Architectures: - Single-tier:users directly interacting with a computer mainframe - Two-tier:use personal computers to access a server - Three-tier:involve accessing separate servers for processing-intensive activities - N-tier:involve multiple web portals - Cloud-based:access databases remotely, run by third parties (ex. emails) ield/ variable:each column in table F Record:each row/case in a table Form:provides a user interface to enter, edit & display data Query:qs posed to a database & used to perform a # of diff actions Report:database object used to summarise & present data residing in the database hp 11 C Job Analysis:analyse work & employee characteristics that needed to perform the work. Ex. HR Planning orkflow Analysis:analyse how work’s accomplished at organisational level & within W organisational units ask-KSAO analysis:carefully defines tasks & KSAOs that make up job (develop list, T document criticality & demonstrate KSAOs linked to tasks) ritical incidents technique:creating examples of good & bad responses to frequently C encountered critical incidents Job designing methods: a. Job enlargement:adding more responsibilities to a job (less boring, more motivating) b. Job enrichment:allows workers to have greater decision-making power c. Job rotation:rotating employees from one job to another (less boring, learn new skills) d. Flextime:workers can choose # of work schedules (improves wellbeing/ motivation) e. Remote work:not physically at office, works substantialamount of time away from office ompetency Modeling:Understanding required attributes and KSAOs for job C groups/organisations. - PROS:broad job description, career development, and alignment with organizational strategies, increased executive buy-in support Job analysis data collecting methods: a. Interviewfrom subject matter experts (SMEs) - most common b. Observeppl doing the work c. Surveysfrom SMEs d. Job analysis from scratch(update existing job analysis using O*NET)