FDA Finals Reviewer PDF
Document Details
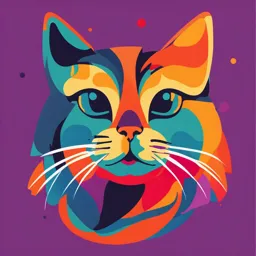
Uploaded by CelebratedStrait
Harvard University
Tags
Summary
This document is a review of FDA final exam material, covering topics such as frequency distribution, relative frequencies, and measures of association. It also discusses various types of variables, data sets, and sampling methods. It includes concepts of descriptive and inferential statistics.
Full Transcript
FDA FINALS REVIEWER Frequency Distribution – number of occurrences of an event Object – the representative or the one who is observing Relative Frequencies – percenta...
FDA FINALS REVIEWER Frequency Distribution – number of occurrences of an event Object – the representative or the one who is observing Relative Frequencies – percentages or proportion Attributes – characteristics/observations Cumulative Frequencies – value of Variables – attribute that is organized and use values that precede for analysis Measures of Association – quantify strength & Nominal Variable – distinctness, non-numerical direction of relationship Ordinal Variable – pertains to order Covariance – linear rs, change in one Interval Variable – not necessary start with zero can change another Ratio Variable – absolute zero origins Correlation – or Pearson correlation coefficient or “r”, both strength and linear rs Discrete – integer Measures of Shape – how data distributed, Continuous – real number pattern Data Sets Symmetric Distribution – mirror image Record Dataset – rows Asymmetric Distribution – biased, with M x N Data Matrix – fixed set numeric specific characteristics attributes Skewness – measure of asymmetry Document Data – vector Positive Skewed/Right-skewed/Positive Transaction Data – transaction, set of skewness – long right tail, positive mean, items median, mode Graph Data Set – interconnections Negative skewed/left-skewed/Negative Skewness – long left tail, negative mean, Ordered Data Set – sequence/pattern median, mode Population – entire collection of individuals Kurtosis – quantifies the shape of probability Parameters – characteristic/describe population distribution Sample – representatives from population Peakedness – data values are concentrated Statistics – describe a sample Mesokurtic – normal distribution Descriptive Statistics – describe & summarize Leptokurtic/heady-tailed distribution – more information than normal distribution, bell shaped Measures of Location – specified amount of Platykurtic/short-tailed distribution – less than percentage data. Deciles (10 parts). Percentiles normal (100 parts). Quartiles (4 parts) Inferential statistics – draw a conclusion Special Percentile – median (50th, half). SAMPLING First Quartile (25th, ¼). Second Quartile (50th=median) Law of Large Number – sample means get closer to the population mean as sample size Measures of Central Tendency – position of gets larger values identify the center, central tendency, mean, median, mode, average Central Limit Theorem – large sample will always fall to normal distribution Measures of Spread/Measures of Dispersion – how close or far, how spread Sampling Frame – Actual list of individuals Range – highest to lowest Probability Sampling – equal chance of being selected Variance – square of standard deviation Simple Random Sampling – everyone Standard Deviation – measures how has an opportunity spread out numbers Stratified Sampling – heterogenous to homogenous strata, propotionally Cluster Sampling – divide into multi- DATA PRE-PROCESSING group or cluster Data Pre-Processing – improving quality for Systematic Sampling – random point to secondary analysis fixed interval DATA CLEANING Multistage Sampling – similar to cluster, Data Cleaning – address noise in data to ensure combination of other sampling accuracy and correctness Non-Probability Sampling – not everyone has a IMPORTANCE chance Ensures Data Accuracy and Reliability Quota Sampling – specific characteristic Improves Data Quality Purposive Sampling – specific criteria or purpose Reduces Errors and Bias in Analysis Snowball of Chain Sampling – referrals Supports Effective Decision-Making Convenience Sampling – availability DATA QUALITY ISSUES DESCRIPTIVE STATISTICS IN BUSINESS Missing Values – incomplete Understanding customer base – demographics Noise & Outliers – data that deviate Financial Analysis – profitability, liquidity Inconsistencies – error, formatting Market Research – trends, preferences Duplicate Data – repeated values Data Visualization – foundation to visualization DATA CLEANING PROCESS DESCRIPTIVE STATISTICS IN HR Handling Missing Values – imputing data Performance Management Smoothing Noisy Data – eliminating outliers Salary & Compensation Analysis Detecting and Deleting Outliers – use box plot Workforce Productivity Fix Structural Errors – all error in words Recruitment & Selection Remove Duplicates – avoid redundancy Evaluating HR Intervention Data Validation – authenticate your data INFERENTIAL STATISTICS IN BUSINESS TASKS A/B Testing – compare website who perform Filling in missing values better Ignore the tuple/row – exclude record Customer Satisfaction Survey – how satisfied with missing values, not effective if there’s a lot Retail Analytics – crucial for marketing & Filling in the missing value manually decisions Data Imputation – use global constant, INFERENTIAL STATISTICS IN HR attribute mean, attribute mean for all samples of the same class Predictive Analytics – develop predictive models Clean Noisy Data Resource Allocation & Optimization – predicting future outcomes Binning – categorical into numerical components Risk Assessment and Mitigation – identify & mitigate Clustering – use cluster average to represent value Hypothesis Testing – effectiveness, impact Regression – simple regression line to estimate very erratic dataset Combine Computer & Human Inspection – Human Interventions Box Plot – use to identify outliers DATA INTEGRATION DATA TRANSFORMATION TECHNIQUES Data Integration – combining data derived from Data Smoothing – helps predicting trends or various sources. Single data store patterns DATA INTEGRATION PROCESS Data Generalization – low-level to high-level Data Sour Identification Data extraction Attribution Construction – create new features Data Mapping Data Discretization – continuous data to data interval Data Validation & Quality Assurance Data Aggregation – compiling large volume then Data Transformation Data Loading transform Data Synchronization DATA TRANSFORMATION PROCESS Data Governance & Security Data Discovery Data Mapping Metadata Enhancement Data Extraction Code Generation & Execution Data access & analysis Review Sending TECHNIQUES IMPORTANCE Extract, Transform, Load (ETL) – secondary Standardization – consistency & uniformity in processing over, structured data, slower, high data cost, custom solutions Compatibility – integration of data for Extract, Load, Transform – loads directly, all comprehensive analysis types (structured, unstructured), faster, low cost, granular access control Analysis – easier interpretation and analysis Issues During Data Integration – redundant Visualization – easier to visualize data, data value conflicts, entity identification Data Profiling – understand the characteristics problem and quality of data FOUR TYPES OF DATA INTEGRATION: Clear Documentation – ensure repeatability and Inner Join – matching values quality Left Join – left values and matching Quality Assurance – ensure accuracy and values from the right integrity of data Right Join – right values and matching Automation – streamline and standardized values from the left processes Outer Join – the union of all values TECHNIQUES IMPORTANCE & USE Normalization – Adjusting data value Improved Decision-Making – Talent Standardization – Scaling data Management Encoding – converting from categorical Compliance with Regulations – Employee to numerical Engagement Aggregation – Combining multiple data Enhance Employee Insights – Workforce points Planning Filtering – Removing redundant or Streamlined Processes – Compensation & irrelevant data Benefits Imputation – Filling in missing values DATA TRANSFORMATION using mean, median, mode Data Transformation – is a process of OPTION FOR DATA TRANSFORMATION transforming data into another format suitable Normalization – scale specific variable falls to for the analysis. small specific range Min-Max Normalization – transform into a standardized format Z-Score Standardization – transform Feature Creation – create new attributes that into a standard normal distribution captures important information Binning – transforming numerical to categorical feature creation methodologies Equal-width (distance) – uniform grid Feature Extraction – creating new features Equal-depth (frequency) – contains same number of samples Mapping Data or New Space – lower- dimensional space to higher-dimensional space DATA ENCODING – categorical to numerical Feature Construction – built Binary Encoding – transformation taking intermediate features values from 0 to 1 DATA POST-PROCESSING & VISUALIZATION Class-based Encoding – probability of the class variable Data Post-Processing – refining and preparing data for analysis after it has been collected. For Continuous Class – average of the class further analysis variable STEPS DATA REDUCTION Data Cleaning Data Reduction – reduced representation of data but produced same results Data Transformation DATA REDUCTION TECHNIQUES Data Integration Feature Selection – most relevant Data Reduction Dimensionality Reduction – preserve essential Data Enrichment – adding additional information features to enhance dataset’s value Data Compression – encode in more compact Data Validation – ensure that data meets required standard Inner Join – matching values This improves data quality, decision-making, Sampling and facilitates predictive analytics STRATEGIES Data Visualization – representing data in visual Sampling – smaller representative that R or R Studio – software facilities generalize the population Can do graphics, dashboards, matrix Simple Random Sampling – equal algebra, data handling & storage: numeric, probability textual, high-level data analytic & Statistical Sampling without replacement – each functions, and programming language: loops, item is selected, removed from the population branching, subroutine Sampling with replacement – not Can’t do: Not a database but connects removed to DBMS, no spreadsheet view of data, but connect to excel/ms offices, no Stratified Sampling – several partitions professional/commercial support Feature Subset Selection – reduces Strengths – Free and open source, strong user dimensionality, removing redundant community, highly extensible and flexible, Brute Force Approach – all possible flexible graphics and extensible defaults, feature subsets implementation of high-end statistical methods Embedded Approaches – feature Weaknesses – steep learning curve, and slow selection occurs naturally for very large datasets Filter Approaches – selected before PARTS data mining Source Pane – type/edit Wrapper Approaches – as black box to command/code find the best subset Console Pane – command line interface Environment Pane – displays object Files Pane – acts as file explorer Bar Graph – a single point. Quantitative single point to qualitative single point WORKING WITH DATASET WAGE Scatter Graph/Plot – determine position. Data Frame – storing dataset, list of vectors (ex: Individual data points to two-dimensional point dataset wage) Pie Chart – dividing circle into proportional STRUCTURE OF DATASET slices Dataset – organized/ordered collection of data Histogram (numeric) – distribution or frequency Plotting the dataset – creating visuals of occurrence representation Box Plot – distribution through quartiles, BASIC VISUALIZATION OR WAYS TO PLOT DATA parallel Histogram – breaks data into bins (breaks) Tree Maps – hierarchal structures using rectangle size Scatter Plot – for simple data inspection Choropleth Maps – geographical areas, colored Box Plot – visualizing the spread and derive or patterns inferences Chart – structures that relate entities IN THE CONTEXT OF HR Network Diagrams – use nodes/vertices Recruitment & Selection – effectiveness of channels & attracting candidates Flow Chart – sequential, intricacies and decision Employee Retention – predict employee Diagrams – schematic picture, symbolic turnover Pareto Chart – identify prevalent defects Employment Engagement – enhance employee Side-by-side Chart – parallel chart, multiple data satisfaction series Training & Development – identify skill gaps & Steam-and-Leaf Display – organize and present training needs numerical data, identify patterns and outliers Plotting Inside R VISUAL DESIGN PRINCIPLES High-level functions – step-by-step Preattention – without the need of attention manner Color Selection Preattention (color) Low-level functions – detailed control Shape Selection Preattention (form) Geom_bar – describes values on the y axis (bar plot) Preattentive – takes a fixed amount of time Aes () function – specify desired aesthetics Conjunction Features DATA VISUALIZATION & COMMUNICATION Emergent Features, have or don’t have a unique feature Visualization – presentation of info using spatial or graphical representations Asymmetric Pre-attentive Features, asymmetric, sloped, and vertical line Comparison facilitation Block Text – specify desired aesthetics Recognition of patterns Visual Illusions – people don’t perceive the way General decision making they should Two types of using visualization, to Delboeuf illusion explore/calculate and to communicate Mueller-Lyon MEDIUM/TYPES OF VISUALIZATION Ebbinghaus illusion Graph – communicate information with at least two scales, symmetric paired Basic Categories Visual Representation – Graph, Table, Maps, Diagram, Network, Icon Line Graph – interval or a time span, trends & relationships CLASSIFICATION OF VISUAL REPRESENTATION Graphs – position and magnitude of geometric Graphical Tables – quantitative, rows & columns IMPLICATION TO DESCRIPTIVE ANALYTICS Tables – words, number, signs, combination Data Privacy Regulations – how info should be collected, used, and stored. Time Chart – temporal data Data Security – protect from unauthorized Network Chart – rs among components access Structure Diagrams – static description of physic Data Bias & Discrimination – prohibit using Process Diagram- interrelationships & processes biased data Maps – physical geography Data Subject Rights – grant individual rights Cartograms – spatial maps THREE C’s Icons – impart interpretation Consent Control Confidentiality Photorealistic Pictures – realistic pictures Credibility Character TUFTE’S GRAPHIC EXCELLENCE Multifunctional graphical elements TUFTE’S GRAPHICAL INTEGRITY Accurately represent data TUFTE’S INK MAXIMATION Maximize data-ink, minimize non-data- ink ETHICS Code of Ethics – principles of conduct Doing the right thing, protect research participants, ensure credibility, trust, accountability, reduce liabilities and waste resources 3 MAJOR ETHICAL CONCERN Discrimination & bias, integrity, lack of transparency Privacy – protection of personal information APPLICATION TO DESCRIPTIVE ANALYTICS Ethical Data Collection Avoiding Bias Honest Data Presentation Accountability and Impact Integrity – conducted honestly, no bias Transparency – openness and clarity Accountability – taking responsibility HANDLING DATA ETHICALLY Consent Data Minimization Security Access & Control Fair use Secure Disposal