ENH522 Lecture 3 Cohort Study Designs PDF
Document Details
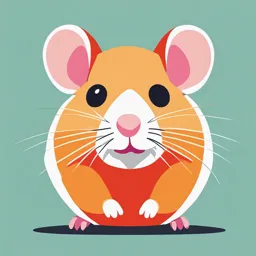
Uploaded by SilentBanjo
McGill University
2024
Emma Lin Buajitti
Tags
Summary
This lecture introduces cohort study designs, explaining the differences between prospective and retrospective approaches. Examples like the Framingham Heart Study and Nurses' Health Study are discussed, highlighting their strengths and limitations. The lecture also covers key concepts like baseline, follow-up time, and person-time.
Full Transcript
Introduction to Cohort Study Designs E N H 5 2 2 : R E S E A RC H M E T H O D S WEEK 4 – SEPTEMBER 17, 2024 E M M A L I N B U A J I TT I E M M A L I N. B U A J I TT I @ M A I L. M C G I L L. C A Readings Redelmeier & Singh (2001). Survival in Academy Award- winning actors and actresses. Topics fo...
Introduction to Cohort Study Designs E N H 5 2 2 : R E S E A RC H M E T H O D S WEEK 4 – SEPTEMBER 17, 2024 E M M A L I N B U A J I TT I E M M A L I N. B U A J I TT I @ M A I L. M C G I L L. C A Readings Redelmeier & Singh (2001). Survival in Academy Award- winning actors and actresses. Topics for today 1. What is a cohort study? 2. Examples of cohort studies 3. Prospective vs. retrospective cohort studies 4. Interpreting data from cohort studies 5. Censoring and survival curves 6. Redelmeier and Singh article 7. Advantages and disadvantages of cohort studies Recall: experimental vs. observational study designs A randomized experiment is (in theory) the gold standard for isolating an effect It works because the researcher can manipulate the explanatory variable and observe the outcome independent of any other factors But some research questions can’t be studied using randomized experiments. Why not? Recall: experimental vs. observational study designs Experiments don’t work well in some cases: Non-modifiable or harmful exposures can’t be randomly assigned Some outcomes are very rare, or take a long time to develop Experiments in health research have sample size and duration limitations due to expense Option 1: narrow target population by limiting inclusion criteria (may limit generalizability) Option 2: use an observational study design! Recall: experimental vs. observational study designs In observational studies, the researcher does not directly manipulate exposure/explanatory variables Most of epidemiology is observational studies Observational studies can be descriptive or analytic 1. What is a cohort study? Cohort studies A very common study observational study design Cohort: a group of people sharing a common characteristic E.g. a birth cohort is all people born in the same year Exposures (explanatory variables) are assessed when the cohort is assembled Carry out research within that group with same characteristic Not all cohorts follow a common characteristics Participants are followed in time to examine outcomes after exposure at the baseline, and then follow them overtime to see what happens Compare base line characteristics with what is observed Useful reference: http://www.iwh.on.ca/wrmb/cohort-study Why is this a good example: ethical Cohort study example: Heart disease is something that life satisfaction, happens over a long period of chronic disease and time, so you would have to death observe overtime Health records are used LIFE HEART DISEASE SATISFACTION ? LIFE DEATH SATISFACTION ? Source: https://www.ncbi.nlm.nih.gov/pmc/articles/PMC63578 02/ COHORT STUDIES Target Population e.g. Defined by exposure, occupation, year of birth Sample identify target population and using that, create a sample COHORT STUDIES Target Population e.g. Defined by exposure, occupation Sample Exposed Not Exposed Collect necessary information to divide sample to two groups- to prevent bias COHORT STUDIES Target Population e.g. Defined by exposure, occupation Sample Exposed Not Exposed TIME Wait for some period of time COHORT STUDIES Target Population e.g. Defined by exposure, occupation After period, Sample capture whatever info you need to Exposed Not Exposed divide your populations into further sections TIME Disease No Disease Disease No Disease COHORT STUDIES Target Population e.g. Defined by exposure, occupation Sample Exposed Not Exposed TIME Death Still alive Death Still alive COHORT STUDIES Target Population e.g. Defined by exposure, occupation Sample Exposed Not Exposed TIME Death Still alive Death Still alive COHORT Target STUDIES Population Sample Exposed Not Exposed TIME Death Still alive Death Still alive Statistical comparison Steps in cohort studies Assemble: Identify study population and initiate a sample that will be the cohort Baseline: Collect initial information about the cohort members (determine exposure) Identify exposed and unexposed: Split study sample into treatment and comparison groups Follow up: go forward in time and watch for events and outcomes Analyze: Compare the number and timing of events in the exposed to the unexposed members of the cohort COHORT Target STUDIES Population Assembl e Sample Baseline Exposed Not Exposed Identify TIME Follow up Death Still alive Death Still alive Statistical Analyze comparison Cohort studies: useful terminology Baseline: Usually when the cohort starts or when someone enters cohort. The time point at which a study participant begins observation and exposure/explanatory variables are assessed. Ex: study on lung cancer, but you’re also looking at data on sex, you have to bring in sex in your sample as well Follow up time: The amount of time a member of the study cohort spends under observation. Ex: Bob was followed for 7 years for the study Person-time: A unit for measuring follow up time. For example, one person-year is equal to one year of follow up in one person. Ex: Bob who was studied for 7 years, served 7 years Time-to-event: A way of measuring outcomes (not just in cohort studies) by counting how long participants spend between baseline and event. Time you’re in the cohort and spend time Outcome ascertainment: The methods researchers use to identify outcomes among study participants (can be passive or active) ◦ Example of active outcome ascertainment: Researchers bring participants in for a follow up interview and take new measurements to compare to baseline. ◦ Example of passive outcome ascertainment: Researchers track participants in the Ontario Cancer Registry, which is automatically updated based on health care interactions. How is follow up really done? In most studies, participants are followed through regularly scheduled interviews, surveys, or visits to a health care facility. In many modern cohort studies, this is done remotely via linkage of medical records Maintaining contact with participants is crucial to the validity (generalizability) of study results. Follow up typically ends based on study budget, sufficient event outcomes -when planning, you need to ask “what level of contact do I need to maintain with people and what is the possibility they will participate” because you need to look at whether people will get back to you or continue the study Cohort studies vs. experiments Cohort studies are typically (but not always) less expensive per person, compared to experiments As a result, most cohort studies have larger sample sizes than the average experiment Cohort studies typically collect more information about baseline characteristics in study participants In many cases, cohort study data can be reused for multiple research projects, looking at different exposures or outcomes This is because the exposure wasn’t randomized – so multiple exposures can be studied using the same statistical techniques But, it always depends on what data was collected at baseline ave to collect and during before the information follow-up you get the information 2. Examples of cohort studies Framingham Heart Study Cohort established in 1948, continued through >65 years and three generations Began with 5,209 men and women between 30 and 62 living in Framingham MA At first largely white & middle class; subsequent recruitment to increase diversity Outcomes: mostly cardiovascular disease e.g. heart attacks, stroke, atrial fibrillation etc. Expanded to a variety of health outcomes incl. dementia, arthritis as the cohort aged Exposures: smoking, cholesterol, blood pressure, others https://www.framinghamheartstudy.org/fhs-about/ http://www.framinghamheartstudy.org/fhs-bibliography/index.php - created a calculator to help measure heart health Nurses’ Health Study Cohort of 121,700 married registered nurses 30 to 55 years old in 1976 and living in one of the 11 most populous states in the USA Cohort was launched to examine the long-term effects of oral contraceptives, which were new at the time Second cohort (NHS II) in 1989, and third (NHS III) in 2010 NHSIII expands inclusion criteria to include men (and Canadians) Complete in depth health questionnaires every 2 years More recently added blood, hair, nail samples Many exposures and outcomes assessed over 30 years 1976 they found they needed more information on women’s health, so they d study on nurses Cohort was stared because people were concerned with long term effects of b control- only few research done Did a second study, because there first cohort had limitations People come in every 2 yeas, they take measurements, and ask a lot of quest and now 50 years later since the cohort started the outcomes are assessed Generalizability in cohort studies Many early cohort studies included study participants based on specific recruitment criteria (e.g., registered nurses in NHSI/II, British civil servants in the Whitehall study) This was often done for reasons of feasibility Nurses Health Studies example: licensing organizations already maintained lists of nurses; able to respond to technically worded questionnaires; motivated to participate in a long-term health study The limitation of this recruitment approach is that study populations were limited to predominantly white, employed, and relatively well-off individuals How does this affect generalizability? Bias, impacts the questions that get asked (produce research questions based on what you see) Black Women’s Health Study https://youtu.be/gj6qMYRjhb4 https://www.bu.edu/bwhs/ 3. Prospective vs. retrospective cohort studies Prospective vs. retrospective cohort studies Defined based on when exposure is assessed and when participants are recruited Prospective cohort studies: Exposure is assessed at inception and participants are followed through time E.g. Framingham, NHS, Black Women’s Health Study Retrospective cohort studies: Historical records are used to assemble a cohort starting at some past date, with exposure assessed using those records, and follow-up continuing to the present. E.g. Academy award winners (this week’s reading) The terms prospective and retrospective refer to the timing of data collection relative to when the study began. The research design is the same. WHAT MAKES IT A COHORT: The assumption is that the exposure assessment is based on information that was collected prior to the outcome,. Even if you as the researcher are using it AFTER Ex: someone’s birthday will always be their birthday Prospective vs. retrospective cohort studies Prospective vs. retrospective cohort studies Prospective Retrospective 2024 2004 2044 2024 4. Interpreting data from cohort studies Measures of occurrence: rates Measures of occurrence are used to count events/outcomes in the study population “Rate” means time is included in the denominator Prevalence calculation (not a rate!): Rate calculation: per person-year Recall: 1 person-year is a unit of person-time, equal to one year of observation in one person (study participant) Small rates are often reported per 1,000 or per 100,000 person-years. For example, 0.01 / person-year = 10 / 1,000 P-Y After 10 years of follow up of 100,000 people, how many person-years have been observed? A million- you take the people you have (100,000), and take number of years (10) you have observation on, and multiply them together: 100,000 x 10 = 1,000,000 Rates in cohorts Mortality rate: # deaths / person-years Incidence rate: # new cases / person-years Over 20 years of follow up in a cohort of 6000 people, 5 deaths were observed. What is the mortality rate per 100,000 person-years? Hint: start by figuring total person-years of follow-up 5 / 120000 = 4.17 per 100,000 Rates in cohorts 20 x 6000 = 120,000 person-years total 5 deaths / 120,000 person-years = 0.0000417 per person-year 4.17 per 100,000 person-years Measures of effect: rate ratios In analytic observational studies, we are usually interested in a relationship between exposure and outcome Measures of effect are used to compare events/outcomes in the exposed and unexposed populations e.g. compare the mortality rate between smokers and non-smokers We do this with a rate ratio Rate ratio calculation: A rate ratio can be interpreted as: “The rate among the exposed was [RR] times greater than the rate among the unexposed.” This means that the event occurred [RR] times faster in the exposed group Rate ratio example We have a cohort of 30,000 people, followed for 10 years. 20,000 are smokers; 10,000 are non-smokers. Over follow up, 10 deaths from lung cancer occur among the non-smokers, 36 deaths from lung cancer occur among smokers. What is the lung cancer mortality rate among smokers per 100,000 person- years? Hint: start by finding the total person-years of follow-up among smokers 10 x 20,000 = 200,000: total person-years What is the lung cancer mortality rate among non-smokers per 100,000 person-years? 5 / 200,000 = 0.00018 0.00018 x 100,000 (perso-years) = mortality rate is 18 What is the lung cancer mortality rate ratio comparing smokers to non- smokers? Rate ratio example Additional rate ratio examples We have a cohort of 12,000 women, followed for 18 years. 4,000 women use oral contraceptives (OC); 8,000 do not. Among OC users, there are 12 cases of pulmonary embolism (PE) over follow up. Among non- users there are 3 cases of PE. ◦ What is the incidence of PE among OC users per 100,000 person-years? ◦ What is the incidence of PE among non-users per 100,000 person-years? ◦ What is the rate ratio of PE comparing OC users to non-users? Additional rate ratio examples Imagine a cohort of 15,000 cyclists. 10,000 are found to always wear a helmet; 5,000 inconsistently or never wear a helmet. You follow the cohort for 7 years. Among helmet wearers, there are 12 head injuries over the course of follow up; and among non-helmet wearers there are 11 head injuries ◦ What is the incidence of head injury among always helmet wearers per 100,000 person-years? ◦ What is the incidence of head injuries among those who don’t always wear helmets per 100,000 person-years? ◦ What is the rate ratio of head injury comparing helmet wearers to non-wearers? Interpreting rate ratio values Rate ratio (RR) Interpretation RR = 1 No relationship between exposure and outcome 0 < RR < 1 Reduced risk of outcome less than one in the exposed 1 > RR > ∞ Increased risk of outcome More than one and in the exposed infinity Note: Rate ratio values will always be between 0 and ∞ The further away from ”1”, the stronger the relationship is Which is the rate ratio showing the strongest relationship between exposure and outcome? A) RR=0 B) RR=1 It is zero because if the rate ratio exists C) RR=2.5 from 0 to infinity, than 0 represents D)RR= 10 stronger effects E)RR= -14 because it’s the smallest Which is the rate ratio showing the strongest relationship between exposure and outcome? A) RR=0 B) RR=1 C) RR=2.5 D)RR= 10 E)RR= -14 5. Censoring and survival curves Person-time is not simple Examples we’ve used are overly simplified! Assume no one is lost in the cohort Assume everyone is always at risk of outcome Assume equal person-time follow up for each subject Calculating person-time for cohorts is analytically complex when people can come in and out of the cohort, or if you are evaluating non-repeatable events (e.g., death) Censoring events- when you stop counting We call events that cause people to leave the cohort censoring events Examples: Death Event (if people with events are no longer at risk) Loss to follow up End of study Follow up time is calculated from start of follow up (cohort entry) to time of the first censoring event Source: http://www.goldsteinepi.com/_/rsrc/1445981794206/blog/therelationshipofcumulativeincidencetoincidencerate Survival curves Was seen in this week’s reading A type of plot- common Shows the % of study sample (often cohort) surviving at each time point over the course of follow up “Survival” is a general term – can also be applied to outcome events other than death For death: Survival time = time from cohort entry to death For other outcomes: Survival time = time from cohort entry to event Often called Kaplan-Meier curves http://cancerguide.org/scurve_basic.html Survival at the beginning is 100% The jaggedness or “step” pattern depends on how often outcome events are ascertained The y axis represents percent or proportion surviving of the original cohort Follow-up time (usually years) is represented on x- http://www.scielo.br/scielo.php?pid=S1806-83242013000400349&script=sci_arttext axis. Peters et al. Int J Epidemiol (2013) 42 (5): 1319-1326.DOI: https://doi.org/10.1093/ije/dyt147 Comparing survival curves We can draw two curves, one for each level of exposure/explanatory variable- useful b/c can use to compare & interpret relationships Watch for: Distance between curves Changes in the distance between curves Differences in steepness Curves crossing Prostate cancer survival http://liu.diva-portal.org/smash/get/diva2:25290/ FULLTEXT01.pdf Based on the survival curves, which tumor grade has the best survival? A) G1 B) G2 C) G3 Based on the survival curves, which tumor grade has the best survival? http://circ.ahajournals.org/content/88/ 1/107.short http://www.bmj.com/content/328/7455/1519?variant=full-text&go to=reply 6. Redelmeier and Singh article Redelmeier and Singh 2001 Redelmeier and Singh constructed a cohort based on all people who had ever been nominated for academy awards. Who did they include in the cohort as a “control” group? A) Academy award winners B) Nominees for Grammy awards C) Every other actor registered with the Screen Actors Guild D) Similar aged co-stars as the nominees 7. Advantages and disadvantages of cohort studies Advantages of Cohort Studies Can study many outcomes, even those you may not have been thinking about at inception Examination of rare exposures: can create an “enriched” cohort- where you create a cohort based on something that is rare but you are interested in it ◦ Examples? World Trade Center cohort example Can demonstrate that exposures come before or precede outcomes (temporality)- sequence in where exposure comes from outcome Can show incubation or latency period for health outcomes Permit direct calculation of incidence Disadvantages of cohort studies Although cheaper than experiments, Large sample sizes and lengthy follow up can be very expensive (tracking people down) Can’t manipulate exposure like experiment Must measure all possible confounding variables (no randomization) Even with long follow up and large sample sizes, rare outcomes may not occur in sufficient numbers for analysis Special bias concerns in cohorts Common Threats to Internal Validity No random assignment to exposure: risk of confounding? Possible biases in assessing or ascertaining the outcome (measurement error/information bias) Information bias in data (e.g. exposure data) gathered (measurement error) Bias from differential loss to follow-up (selection bias) Bias from who is included in the study cohort (selection bias) Analytic bias (decisions the analysts make) For these biases to affect the study conclusions, they must be “differential” between exposed/unexposed or outcome/no outcome groups. Special bias concerns in cohorts Selection bias Cohorts don’t need to be representative or have perfect participation rates to be valid: But if participation or loss to follow-up differs between groups, the findings will be inaccurate. Source: http://sphweb.bumc.bu.edu/otlt/MPH-Modules/EP/EP713_CohortStudies/EP713_CohortStudies_print.html Special bias concerns in cohorts Confounders Known confounders can be measured at the same time as exposures (explanatory variables) are measured Statistical techniques (“adjustment”) can be used to account for effects of confounders: allows you to factor out the confounder in estimating the relationship between exposure and outcome. Unknown confounders? Special bias concerns in cohorts Rare outcomes, long latency Some health outcomes are rare and/or can take decades to develop In an analysis of the Nurses Health Study data, following 88,565 women for 16 years (1,417,040 years of follow up), there were 131 cases of Parkinson’s disease In a cohort created from a census sample of Canadians (approximately, 2.1 million members) and followed for 13 years, there were fewer than 25 cases of a rare type of nasal cancer that may be work related If numbers are too small to analyze, what should we do? Resource for Assessing Study Validity https://www.ncbi.nlm.nih.gov/pmc/articles/PMC292 0077/ 79