ECO101 Lecture 1 PDF
Document Details
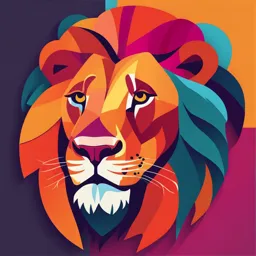
Uploaded by GodGivenFeynman
Xi'an Jiaotong-Liverpool University
Tags
Summary
This document is a lecture from an introductory statistics course at the Xi'an Jiaotong-Liverpool University's International Business School covering various topics on fundamental statistical concepts. No questions are present.
Full Transcript
ECO 101 Introduction to Statistics Announcements Instructors: Dr. Sasan Bakhtiari (week 1-6) Dr. Xin Gu (week 7-12) No tutorial tomorrow! Office hours (Room BS314): Thursdays 2pm-4pm Final exam: 100% Textbook Data Work Data work in your tutoria...
ECO 101 Introduction to Statistics Announcements Instructors: Dr. Sasan Bakhtiari (week 1-6) Dr. Xin Gu (week 7-12) No tutorial tomorrow! Office hours (Room BS314): Thursdays 2pm-4pm Final exam: 100% Textbook Data Work Data work in your tutorials Get hands-on experience Prepare you for FYP and workplace A variety of useful software Excel SPSS R Python Minitab SAS Free Statistics: FirmID Company 1004 AAR CORP Current Assets 1097.9 Total Assets 1833.1 Liabilities 734 Turnover 1990.6 Domestic D SIC 50 … Science of Data AMERICAN AIRLINES GROUP 1045 INC 13572 63058 68260 52788 B 45 1050 CECO ENVIRONMENTAL CORP 281.437 600.291 362.8 544.845 D 35 PINNACLE WEST CAPITAL 1075 CORP 1926.967 24661.153 18376.291 4695.991 D 49 … 1078 ABBOTT LABORATORIES 22670 73214 34387 40109 B 38 1104 ACME UNITED CORP 92.024 149.241 51.343 191.501 D 34 1117 BK TECHNOLOGIES CORP 37.202 49.408 28.097 74.094 D 36 ADAMS RESOURCES & 1121 ENERGY INC 232.471 361.334 268.618 2745.293 D 51 … 1161 ADVANCED MICRO DEVICES 16768 67885 11993 22680 B 36 1166 ASM INTERNATIONAL NV 1999.432 4671.993 1105.252 2911.846 B 35 1186 AGNICO EAGLE MINES LTD 2191.152 28684.949 9262.034 6626.909 D 10 AIR PRODUCTS & CHEMICALS 1209 INC 5200.5 32002.5 16342.2 12600 D 28 … 1210 AIR T INC 116.557 189.562 163.87 247.323 D 45 1224 SPIRE ALABAMA INC 168.6 2449.3 1521.3 571.1 D 49 1225 ALABAMA POWER CO 3077 35780 23447 7050 D 49 1230 ALASKA AIR GROUP INC 2705 14613 10500 10426 B 45 1254 MATSON INC 602.3 4294.6 1893.9 3094.6 D 44 1266 ALICO INC 58.805 428.353 177.976 39.846 D 10 HONEYWELL INTERNATIONAL 1300 INC 23502 61525 45084 36662 B 99 1327 SKYWORKS SOLUTIONS INC 3179.5 8426.7 2344 4772.4 D 36 … 1380 HESS CORP 3430 24007 14405 10511 D 13 1388 AMERICAN AIRLINES INC 20367 69074 62497 52784 D 45 1410 ABM INDUSTRIES INC 1710.8 4933.7 3133.8 8096.4 D 73 Source: Compustat AMERICAN ELECTRIC POWER 1440 CO 6082.1 96684 71355.6 18982.3 D 49......... 2024/9/8 6 Do you recognize any of these? FirmID Company Current Assets Total Assets Liabilities Turnover Domestic SIC 1161 ADVANCED MICRO DEVICES 16768 67885 11993 22680 B 36 1690 APPLE INC 143566 352583 290437 383285 B 36 4503 EXXON MOBIL CORP 96609 376317 163779 334697 B 29 4839 FORD MOTOR CO 121481 273310 230512 176191 B 37 5073 GENERAL MOTORS CO 101618 273064 204757 171842 B 37 6008 INTEL CORP 43269 191572 81607 54228 B 36 12141 MICROSOFT CORP 184257 411976 205753 211915 B 73 34496 TENCENT MUSIC ENTERTAINMENT 4221.884 10652.867 2585.65 3913.874 B 73 35077 UBER TECHNOLOGIES INC 11297 38699 26017 37281 D 41 149683 CHINA AUTOMOTIVE SYSTEMS INC 564.075 766.44 398.018 576.354 D 37 160329 ALPHABET INC 171530 402392 119013 307394 B 73 184996 TESLA INC 49616 106618 43009 96773 B 37 2024/9/8 7 Statistics: Science of Data So many Understanding numbers, so 8,528 Firms what is going much on information Getting a picture out of a large number of observations and variables 2024/9/8 8 The process Looking at data Design of experiment Drawing conclusions Sample design from samples Graphically Data collection Inference reasoning Numerically Probability Distribution curve One vs. two variables (relationships) Statistical Producing data Inference 2024/9/8 9 Looking at Data - Distributions Chapter 1 2024/9/8 10 Looking at Data Data Section 1.1 2024/9/8 11 Cases Cases are individuals we collect information − data − from FirmID Company Current Assets Total Assets 1004 AAR CORP 1097.9 1833.1 AMERICAN AIRLINES Customers 1045 GROUP INC 13572 63058 CECO ENVIRONMENTAL Cases = Firms 1050 CORP 281.437 600.291 Companies PINNACLE WEST CAPITAL 1075 CORP 1926.967 24661.153 1078 ABBOTT LABORATORIES 22670 73214 Subjects in a study 1104 ACME UNITED CORP 92.024 149.241 Participants in an experiment … 2024/9/8 12 Labels Also Label (If names are Label unique) A special variable that uniquely identifies different cases FirmID Company Current Assets Total Assets 1004 AAR CORP 1097.9 1833.1 AMERICAN AIRLINES 1045 GROUP INC 13572 63058 Why generated a label? CECO ENVIRONMENTAL 1050 CORP 281.437 600.291 PINNACLE WEST CAPITAL To anonymise data 1075 CORP 1078 ABBOTT LABORATORIES 1926.967 22670 24661.153 73214 1104 ACME UNITED CORP 92.024 149.241 For data consistency 2024/9/8 13 Variables Variables The characteristics of a case Varies from case to case FirmID Company 1004 AAR CORP Current Assets Total Assets 1097.9 1833.1 AMERICAN AIRLINES Different cases can have different 1045 GROUP INC CECO ENVIRONMENTAL 13572 63058 values for the variable 1050 CORP PINNACLE WEST CAPITAL 281.437 600.291 1075 CORP 1926.967 24661.153 Adds/contains information 1078 ABBOTT LABORATORIES 1104 ACME UNITED CORP 22670 92.024 73214 149.241 2024/9/8 14 Putting together Label them Analyse All students sitting Gender in the last 3 rows Make sure each Major Distribution of in ECO101 lecture case has a unique Hours exercised hours exercised. hall identifier last week Choose cases Fill in variables 2024/9/8 15 Types of variables Quantitative Categorical Takes numerical value Falls into a few (finite) Arithmetic operations are groups or categories possible Number of occurrences can be counted In most cases cannot be ordered 2024/9/8 16 Example 1 Quantitative Categorical FirmID Compnay Current Assets Total Assets Liabilities Turnover Domestic SIC 1004 AAR CORP 1097.9 1833.1 734 1990.6 D 50 AMERICAN AIRLINES GROUP 1045 INC 13572 63058 68260 52788 B 45 1050 CECO ENVIRONMENTAL CORP 281.437 600.291 362.8 544.845 D 35 1075 PINNACLE WEST CAPITAL CORP 1926.967 24661.153 18376.291 4695.991 D 49 1078 ABBOTT LABORATORIES 22670 73214 34387 40109 B 38 1104 ACME UNITED CORP 92.024 149.241 51.343 191.501 D 34 1117 BK TECHNOLOGIES CORP 37.202 49.408 28.097 74.094 D 36 ADAMS RESOURCES & ENERGY 1121 INC 232.471 361.334 268.618 2745.293 D 51 1161 ADVANCED MICRO DEVICES 16768 67885 11993 22680 B 36 1166 ASM INTERNATIONAL NV 1999.432 4671.993 1105.252 2911.846 B 35 1186 AGNICO EAGLE MINES LTD 2191.152 28684.949 9262.034 6626.909 D 10 2024/9/8 17 How do you know if a variable is categorical or quantitative? Ask: How many different values are there? Are there only a few Are they numerical values? and can be ordered Are they making a (small to large)? statement? Quantitative Categorical Note Quantitative variables can be converted into categorical (but not vice versa) Age (quantitative) 1 − 100 Break into categories: (1-10) , (11-19) , (20-35) , (35-55) , (55-70) , (70+) 2024/9/8 19 Looking at Data Displaying Distributions with Graphs Section 1.2 2024/9/8 20 Distribution of a Variable To examine a single variable, we graphically display its distribution. It tells us what values variable takes and how often. The proper choice of graphical tool to display distribution depends on the nature of variable. Categorical variable Quantitative variable Pie chart Histogram Bar graph Stemplot 21 Categorical Variables Categorical variables can only be counted Their distribution lists the categories and gives the count or percentage of cases falling into each category. Pie charts: distribution of a categorical variable as a “pie”; slice sizes reflect the counts or per cents for the categories. Bar graphs: represent categories as bars whose heights show the category counts or per cents. 22 Categorical Variables Pie Chart Bar Chart 23 Pie Charts and Bar Graphs Library 14% Resource Count Percent of total Wikipedia Google or 406 73.6% Google 9% 74% Google Scholar Other 3% Library database 75 13.6% or website Wikipedia or 52 9.4% Online Research Sources online 450 encyclopedia 400 350 Other 19 3.4% Number of Students 300 250 Total 552 100.0% 200 150 100 50 0 Google Library Wikipedia Other 24 Quantitative Variables The distribution of a quantitative variable tells us what values the variable takes on and how often it takes those values. Two ways to show this distribution: Stemplots Histograms 25 Stemplots To construct a stemplot: 1. Separate each observation into a stem (all but the rightmost digit) and a leaf (last digit). 2. Write the stems in a vertical column; draw a vertical line to the right of the stems. 3. Write each leaf in the row to the right of its stem; order the leaves, if desired. 26 Example Survey of 30 people in China and their height (cm) 1. Collected data 2. Run a stem through 3. Distinct values of the 4. Sort the leaves them stem, add leaves 174 169 173 174 169 173 155 171 177 155 171 177 14 9 14 9 157 166 166 157 166 166 15 5785898518 15 1555788889 181 158 155 181 158 155 16 32196241642 16 11222344669 177 178 164 177 178 164 17 4718370 17 0134778 163 162 151 163 162 151 18 1 18 1 158 164 162 158 164 162 162 159 158 162 159 158 155 158 170 155 158 170 161 161 149 161 161 149 27 Example (continued) China 14 9 How likely it is for someone to be 15 1555788889 very tall (>180cm)? 16 11222344669 17 0134778 What is the most common range 18 1 of heights? Example (Continued) Netherlands China 14 9 5 8 8 9 15 1555788889 Which country 2 2 4 6 9 16 11222344669 is taller? 2 5 6 7 7 7 8 9 9 17 0134778 0 2 4 5 5 7 8 18 1 4 6 19 2024/9/8 29 Histograms For large datasets and/or quantitative variables that take many values: 1. Divide the possible values into classes, or intervals of equal width. 2. Count how many observations fall into each interval. Instead of counts, one may also use percentages. 3. Draw a picture representing the distribution ― each bar height is equal to the number (or percent) of observations in its interval. 30 Example IQ Scores – 60 12th-graders Distribution of IQ Scores 18 Class Count 16 14 75 ≤ IQ Score < 85 2 Number of Students 12 85 ≤ IQ Score < 95 3 10 95 ≤ IQ Score < 105 10 8 105 ≤ IQ Score < 115 16 6 115 ≤ IQ Score < 125 13 4 125 ≤ IQ Score < 135 10 2 0 135 ≤ IQ Score < 145 5 145 ≤ IQ Score < 155 1 IQ Score 31 Examining Distributions Look for the overall pattern You can describe the overall pattern by its shape, center, and spread. Look for striking deviations from that pattern. An important kind of deviation is an outlier, an individual that falls outside the overall pattern. 32 Distributions Symmetric: right and left sides of graph are (approximately) mirror images of each other. Skewed to the right (right-skewed): graph has a longer, thinner upper tail Skewed to the left (left-skewed) graph has a longer, thinner lower tail Symmetric Left-skewed Right-skewed 33 Example: Right Skewed Many economic quantities exhibit right-skewed distributions Many small values A few large values Income Wealth Assets Revenue … Source: Compustat 2024/9/8 34 Outliers They lie outside the overall pattern of a distribution. Always look for outliers and try to explain them. Covid deaths below 50,000 were common amongst countries EXCEPT for united states that recorded exceptionally high number of deaths A large gap in the distribution is typically a sign of an outlier. Source: WHO Explaining Outliers Various reasons we have outliers 120 100 Legitimate observations Count of Observations 80 (previous example) Who is 60 that? Data errors 40 20 0 1-10 11-19 20-35 36-55 56-70 70-99 100-200 200-300 300-400 Age Group 2024/9/8 36 Time Plots They show trend over time: Time is always on the horizontal axis, and the variable being measured is on the vertical axis. Look for an overall pattern (trend) and deviations from this trend. Connecting the data points by lines can reveal this trend. Look for patterns that repeat at known regular intervals (seasonal variations). 37 Example - Trends Closing Gap Upward Trend 2024/9/8 38 Example – Seasonality (Cyclicality) Cyclicality Upward Trend 39 Looking at Data Describing distributions with numbers Section 1.3 2024/9/8 40 Summary statistics Measures of centre: mean, median Measures of spread: quartiles, standard deviation They summarise a distribution in a few numbers 2024/9/8 41 Centre of a Distribution 2024/9/8 42 Measuring Centre: The Mean To compute the mean 𝑥𝑥 (pronounced “x-bar”) of a set of observations, add their values and divide by the number of observations. For n observations x1, x2, x3, …, xn, the mean is sum of observations 𝑥𝑥1 + 𝑥𝑥2 + ⋯ + 𝑥𝑥𝑛𝑛 𝑥𝑥 = = 𝑛𝑛 𝑛𝑛 In a more compact notation: 1 𝑥𝑥 = 𝑥𝑥𝑖𝑖 𝑛𝑛 43 Measuring Centre: The Median Mean cannot resist the influence of extreme observations (outliers) It is not a resistant or robust measure of center Another common measure of center is the median. 44 The Median The median (M) is the number such that half the observations are smaller and half are larger. To find the median: 1. Arrange all observations from smallest to largest. 2. Number of observations n is odd: median, M, is the centre observation. 3. Number of observations n is even: median, M, is the average of the two centre observations. Cuts data into two halves ½ Obs. ← M → ½ Obs. 2024/9/8 45 Example – Time to start a business Calculate mean and median of the time to start a business (in days) of 24 randomly selected countries. 16 4 5 6 5 7 12 19 10 2 25 19 38 5 24 8 6 5 53 32 13 49 11 17 16 + 4 + 5 + ⋯ + 11 + 17 𝑥𝑥̅ = = 16.29 days 24 2 4 5 5 5 5 6 6 7 8 10 11 11 + 12 Sort it 12 13 16 17 19 19 24 25 32 38 49 53 𝑀𝑀 = = 11.5 days 2 Even n, two centre observations 46 Robustness of Median A simple example 17 23 31 47 59 Mean = 35.5, Median = 31 17 23 31 47 1059 Mean = 235.5, Median = 31 17 23 31 47 10059 Mean = 2035.5, Median = 31 Outlier? 2024/9/8 47 Comparing Mean and Median Symmetric distribution Skewed distribution Mean and median are close together Mean is farther out in the long tail than (almost the same). is the median. Mean Median Mean Median Median Mean 48 Detecting skewness US Public firms and their sales Mean = $6,230.9m Median = $548.9m Mean >> Median Right-skewed 2024/9/8 49 Measuring Spread Two distributions can have the same mean but different spread 10 10 Same centre, different spreads 50 Quartiles Cuts data into four quarters Q1 M Q3 ¼ Obs. ¼ Obs. ¼ Obs. ¼ Obs. Calculating the Quartiles 1. Arrange observations in increasing order and locate the median M. 2. The first quartile Q1 is the median of the observations located to the left of the median in the ordered list. 3. The third quartile Q3 is the median of the observations located to the right of the median in the ordered list. 51 Example Time to start a business (median=11.5) 5+6 𝑄𝑄1 = = 5.5 2 2 4 5 5 5 5 6 6 7 8 10 11 12 13 16 17 19 19 24 25 32 38 49 53 19 + 24 𝑄𝑄3 = = 21.5 2 2024/9/8 52 The Five-Number Summary Minimum and maximum tell us little about the distribution as a whole. Median and quartiles also tell us little about the tails of a distribution. For a quick summary of both center and spread, combine all five numbers. The five-number summary of a distribution consists of the smallest observation, the first quartile, the median, the third quartile, and the largest observation, written in order from smallest to largest. Minimum Q1 M Q3 Maximum 53 Suspected Outliers: 1.5 × IQR Rule Interquartile range (IQR) is defined as IQR = Q3 – Q1. A measure of spread, but can be used as part of a rule of thumb for identifying outliers. The 1.5 × IQR Rule for Outliers Call an observation an outlier if it falls more than 1.5 × IQR above the third quartile or below the first quartile. Business start time data: Q1 = 5.5, Q3 = 21.5 → IQR = 21.5-5.5 = 16 days. For these data, 1.5 × IQR = 1.5(17) = 24 Any business start time shorter than –18.5 Q1 – (1.5 × IQR) = 5.5 – 24 = –18.5 days or longer than 45.5 days is considered an outlier. So 49 and 53 are Q3 + (1.5 × IQR) = 21.5 + 24 = 45.5 outlier. 54 Boxplots To make a box plot 1. Draw and label a number line that includes the range of the distribution. 2. Draw a central box from Q1 to Q3. 3. Note the median M inside the box. 4. Extend lines (whiskers) from the box out to the minimum and maximum values that are not outliers. 55 Boxplot - Example Using business start times data construct a boxplot. 16 4 5 6 5 7 12 19 10 2 25 19 38 5 24 8 6 5 53 32 13 49 11 17 Sort the data 2 4 5 5 5 5 6 6 7 8 10 11 12 13 16 17 19 19 24 25 32 38 49 53 Min = 2 Q1 = 5.5 M = 11.5 Q3 = 21.5 Max = 53 This is an outlier by the 1.5 × IQR rule 0 10 20 30 40 50 60 Start Time 56 Measuring Spread: The Standard Deviation Most common measure of spread Looks at how far each observation is from the mean. Standard deviation, sx , measures average distance of observations from their mean. 1. Compute average squared distances or variance. 𝑥𝑥1 − 𝑥𝑥̅ 2 + 𝑥𝑥2 − 𝑥𝑥̅ 2 + ⋯ + 𝑥𝑥𝑛𝑛 − 𝑥𝑥̅ 2 1 Variance = 𝑠𝑠𝑥𝑥2 = = 𝑥𝑥𝑖𝑖 − 𝑥𝑥̅ 2 𝑛𝑛 − 1 𝑛𝑛 − 1 2. Take the square root to get average distance 1 2 Standard deviation = 𝑠𝑠𝑥𝑥 = 𝑥𝑥𝑖𝑖 − 𝑥𝑥̅ 𝑛𝑛 − 1 57 Example Consider the data concerning the number of pets owned by a group of nine children shown on the dot plot below. 1. Calculate the mean. 2. Calculate each deviation. Deviation = observation – mean Deviation: 1 – 5 = –4 Deviation: 8 – 5 = 3 Number of Pets x =5 58 Example (Cont.) xi (xi-mean) (xi-mean)2 3. Square each deviation. 1 1 – 5 = –4 (–4)2 = 16 3 3 – 5 = –2 (–2)2 = 4 4. Find the “average” squared deviation. This is called the 4 4 – 5 = –1 (–1)2 = 1 variance. 4 4 – 5 = –1 (–1)2 = 1 4 4 – 5 = –1 (–1)2 = 1 3. Calculate the square root of the variance. This is the standard 5 5–5=0 (0)2 = 0 deviation. 7 7–5=2 (2)2 = 4 8 8–5=3 (3)2 = 9 9 9–5=4 (4)2 = 16 Sum = ? Sum = ? “Average” squared deviation = 52/(9 – 1) = 6.5. This is the variance. Standard deviation = square root of variance = 6.5 = 2.55 59 Properties of the Standard Deviation s measures spread about the mean and should be used only when the mean is an appropriate measure of center. s = 0 only when all observations have the same value (there is no spread). Otherwise, s > 0. s is not resistant to outliers. s has the same units of measurement as the original observations. 60 Choosing Measures of Center and Spread We now have a choice between two descriptions for center and spread: Set 1: Mean and standard deviation Set 2: Median and interquartile range Choosing Measures of Center and Spread Skewed distribution or outliers: median and IQR are better than mean and standard deviation. Use mean and standard deviation only for reasonably symmetric distributions that do not have outliers. NOTE: Numerical summaries do not fully describe the shape of a distribution. TRY PLOTTING YOUR DATA FIRST! 61 Changing the Unit of Measurement Variables can be recorded in different units of measurement. Most often, one measurement unit is a linear transformation of another measurement unit: xnew = a + bx. Linear transformations do not change the basic shape of a distribution (skew, symmetry, multimodal). But they do change the measures of center and spread. Multiplying each observation by a positive number b multiplies both measures of center (mean, median) and spread (IQR, s) by b. Adding the same number a (positive or negative) to each observation adds a to the measures of center and to the quartiles, but it does not change measures of spread (IQR, s). 62 Example Temperature in Suzhou on Sept.15 has a mean 33 degree centigrade and a standard deviation of 2.2. What is the mean and standard deviation in Fahrenheits? F=1.8C+32 Mean(F) = 1.8×33+32 = 92.4 StdDev(F) = 1.8×2.2 = 3.96 2024/9/8 63 Next week Chapter 1 4. Density Curves and Normal Distributions Chapter 2 1. Relationships 2. Scatterplots 3. Correlation