Statistics - Probability and Descriptive Statistics PDF
Document Details
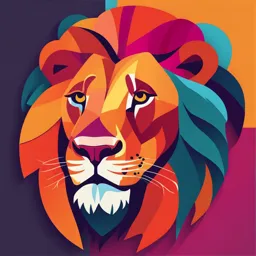
Uploaded by nash300
2023
N.N.
Tags
Summary
This course book covers Probability and Descriptive Statistics, including definitions, independent events, conditional probability, and Bayesian statistics. It also explores random variables, their distributions (PMFs, PDFs, CDFs), joint distributions, and expectation and variance calculations. The text is part of a course offered at IU Internationale Hochschule GmbH in Germany.
Full Transcript
STATISTICS – PROBABILITY AND DESCRIPTIVE STATISTICS DLBDSSPDS01-01 STATISTICS – PROBABILITY AND DESCRIPTIVE STATISTICS MASTHEAD Publisher: IU Internationale Hochschule GmbH IU International University of Applied Sciences Juri-Gagarin-Ring 152 D-99084 Erfurt Mailing address: Albert-Proeller-Stra...
STATISTICS – PROBABILITY AND DESCRIPTIVE STATISTICS DLBDSSPDS01-01 STATISTICS – PROBABILITY AND DESCRIPTIVE STATISTICS MASTHEAD Publisher: IU Internationale Hochschule GmbH IU International University of Applied Sciences Juri-Gagarin-Ring 152 D-99084 Erfurt Mailing address: Albert-Proeller-Straße 15-19 D-86675 Buchdorf [email protected] www.iu.de DLBDSSPDS01-01 Version No.: 001-2023-0901 N.N. © 2023 IU Internationale Hochschule GmbH This course book is protected by copyright. All rights reserved. This course book may not be reproduced and/or electronically edited, duplicated, or distributed in any kind of form without written permission by the IU Internationale Hochschule GmbH (hereinafter referred to as IU). The authors/publishers have identified the authors and sources of all graphics to the best of their abilities. However, if any erroneous information has been provided, please notify us accordingly. 2 TABLE OF CONTENTS STATISTICS – PROBABILITY AND DESCRIPTIVE STATISTICS Introduction Signposts Throughout the Course Book . . . . . . . . . . . . . . . . . . . . . . . . . . . . . . . . . . . . . . . . . . . . . 6 Basic Reading . . . . . . . . . . . . . . . . . . . . . . . . . . . . . . . . . . . . . . . . . . . . . . . . . . . . . . . . . . . . . . . . . . . . 7 Further Reading . . . . . . . . . . . . . . . . . . . . . . . . . . . . . . . . . . . . . . . . . . . . . . . . . . . . . . . . . . . . . . . . . . 8 Learning Objectives . . . . . . . . . . . . . . . . . . . . . . . . . . . . . . . . . . . . . . . . . . . . . . . . . . . . . . . . . . . . . . 10 Unit 1 Probability 1.1 1.2 1.3 1.4 Definitions . . . . . . . . . . . . . . . . . . . . . . . . . . . . . . . . . . . . . . . . . . . . . . . . . . . . . . . . . . . . . . . . . . 14 Independent Events . . . . . . . . . . . . . . . . . . . . . . . . . . . . . . . . . . . . . . . . . . . . . . . . . . . . . . . . . . 22 Conditional Probability . . . . . . . . . . . . . . . . . . . . . . . . . . . . . . . . . . . . . . . . . . . . . . . . . . . . . . . 23 Bayesian Statistics . . . . . . . . . . . . . . . . . . . . . . . . . . . . . . . . . . . . . . . . . . . . . . . . . . . . . . . . . . . 25 Unit 2 Random Variables 2.1 2.2 2.3 2.4 83 Joint Distributions . . . . . . . . . . . . . . . . . . . . . . . . . . . . . . . . . . . . . . . . . . . . . . . . . . . . . . . . . . . 84 Marginal Distributions . . . . . . . . . . . . . . . . . . . . . . . . . . . . . . . . . . . . . . . . . . . . . . . . . . . . . . . . 96 Independent Random Variables . . . . . . . . . . . . . . . . . . . . . . . . . . . . . . . . . . . . . . . . . . . . . . 100 Conditional Distributions . . . . . . . . . . . . . . . . . . . . . . . . . . . . . . . . . . . . . . . . . . . . . . . . . . . . 106 Unit 4 Expectation and Variance 4.1 4.2 4.3 4.4 4.5 31 Random Variables . . . . . . . . . . . . . . . . . . . . . . . . . . . . . . . . . . . . . . . . . . . . . . . . . . . . . . . . . . . 32 Probability Mass Functions and Distribution Functions . . . . . . . . . . . . . . . . . . . . . . . . . . 35 Important Discrete Random Variables . . . . . . . . . . . . . . . . . . . . . . . . . . . . . . . . . . . . . . . . . . 40 Important Continuous Random Variables . . . . . . . . . . . . . . . . . . . . . . . . . . . . . . . . . . . . . . 56 Unit 3 Joint Distributions 3.1 3.2 3.3 3.4 13 113 Expectation of a Random Variable . . . . . . . . . . . . . . . . . . . . . . . . . . . . . . . . . . . . . . . . . . . . 116 Variance and Covariance . . . . . . . . . . . . . . . . . . . . . . . . . . . . . . . . . . . . . . . . . . . . . . . . . . . . 127 Expectations and Variances of Important Probability Distributions . . . . . . . . . . . . . . . 134 Central Moments . . . . . . . . . . . . . . . . . . . . . . . . . . . . . . . . . . . . . . . . . . . . . . . . . . . . . . . . . . . 146 Moment Generating Functions . . . . . . . . . . . . . . . . . . . . . . . . . . . . . . . . . . . . . . . . . . . . . . . 151 3 Unit 5 Inequalities and Limit Theorems 5.1 5.2 5.3 5.4 161 Probability Inequalities . . . . . . . . . . . . . . . . . . . . . . . . . . . . . . . . . . . . . . . . . . . . . . . . . . . . . . 162 Inequalities and Expectations . . . . . . . . . . . . . . . . . . . . . . . . . . . . . . . . . . . . . . . . . . . . . . . . 172 The Law of Large Numbers . . . . . . . . . . . . . . . . . . . . . . . . . . . . . . . . . . . . . . . . . . . . . . . . . . . 177 The Central Limit Theorem . . . . . . . . . . . . . . . . . . . . . . . . . . . . . . . . . . . . . . . . . . . . . . . . . . 182 Appendix List of References . . . . . . . . . . . . . . . . . . . . . . . . . . . . . . . . . . . . . . . . . . . . . . . . . . . . . . . . . . . . . . . 196 List of Tables and Figures . . . . . . . . . . . . . . . . . . . . . . . . . . . . . . . . . . . . . . . . . . . . . . . . . . . . . . . . 197 4 INTRODUCTION WELCOME SIGNPOSTS THROUGHOUT THE COURSE BOOK This course book contains the core content for this course. Additional learning materials can be found on the learning platform, but this course book should form the basis for your learning. The content of this course book is divided into units, which are divided further into sections. Each section contains only one new key concept to allow you to quickly and efficiently add new learning material to your existing knowledge. At the end of each section of the digital course book, you will find self-check questions. These questions are designed to help you check whether you have understood the concepts in each section. For all modules with a final exam, you must complete the knowledge tests on the learning platform. You will pass the knowledge test for each unit when you answer at least 80% of the questions correctly. When you have passed the knowledge tests for all the units, the course is considered finished and you will be able to register for the final assessment. Please ensure that you complete the evaluation prior to registering for the assessment. Good luck! 6 BASIC READING Downey, A.B. (2014). Think stats (2nd ed.). O’Reilly. http://search.ebscohost.com.pxz.iubh. de:8080/login.aspx?direct=true&db=cat05114a&AN=ihb.28838&site=eds-live&scope=s ite Kim, A. (2019). Exponential Distribution - Intuition, Derivation, and Applications. (Available online) Rohatgi, V. K., & Saleh, A. K. E. (2015). An introduction to probability and statistics. John Wiley & Sons, Incorporated. http://search.ebscohost.com.pxz.iubh.de:8080/login.aspx ?direct=true&db=cat05114a&AN=ihb.45506&site=eds-live&scope=site Triola , M.F. (2013). Elementary statistics. Pearson Education. http://search.ebscohost.com. pxz.iubh.de:8080/login.aspx?direct=true&db=cat05114a&AN=ihb.45501&site=eds-live &scope=site Wagaman, A.S & Dobrow, R.P. (2021). Probability: With applications and R. Wiley. http://sea rch.ebscohost.com.pxz.iubh.de:8080/login.aspx?direct=true&db=edsebk&AN=294773 4&site=eds-live&scope=site 7 FURTHER READING UNIT 1 Downey, A.B. (2014). Think stats (2nd ed.). O’Reilly. http://search.ebscohost.com.pxz.iubh. de:8080/login.aspx?direct=true&db=cat05114a&AN=ihb.28838&site=eds-live&scope=s ite Rohatgi, V. K., & Saleh, A. K. E. (2015). An introduction to probability and statistics. John Wiley & Sons, Incorporated. (Chapter 1). http://search.ebscohost.com.pxz.iubh.de:808 0/login.aspx?direct=true&db=cat05114a&AN=ihb.45506&site=eds-live&scope=site Wagaman, A.S & Dobrow, R.P. (2021). Probability: With applications and R. Wiley http://sear ch.ebscohost.com.pxz.iubh.de:8080/login.aspx?direct=true&db=edsebk&AN=2947734 &site=eds-live&scope=site UNIT 2 Downey, A.B. (2014). Think Bayes. Sebastopol, CA: O’Reilly. (Chapters 3, 4, 5, and 6) http://s earch.ebscohost.com.pxz.iubh.de:8080/login.aspx?direct=true&db=cat05114a&AN=ih b.28839&site=eds-live&scope=site Rohatgi, V. K., & Saleh, A. K. E. (2015). An introduction to probability and statistics. John Wiley & Sons, Incorporated. (Chapter 5). http://search.ebscohost.com.pxz.iubh.de:808 0/login.aspx?direct=true&db=cat05114a&AN=ihb.45506&site=eds-live&scope=site Wagaman, A.S & Dobrow, R.P. (2021). Probability: With applications and R. Wiley (Chapter 3,6-8). http://search.ebscohost.com.pxz.iubh.de:8080/login.aspx?direct=true&db=eds ebk&AN=2947734&site=eds-live&scope=site UNIT 3 Downey, A.B. (2014). Think Bayes. Sebastopol, CA: O’Reilly. (Chapter 7) http://search.ebsco host.com.pxz.iubh.de:8080/login.aspx?direct=true&db=cat05114a&AN=ihb.28839&sit e=eds-live&scope=site Rohatgi, V. K., & Saleh, A. K. E. (2015). An introduction to probability and statistics. John Wiley & Sons, Incorporated. (Chapter 4). http://search.ebscohost.com.pxz.iubh.de:808 0/login.aspx?direct=true&db=cat05114a&AN=ihb.45506&site=eds-live&scope=site 8 UNIT 4 Rohatgi, V. K., & Saleh, A. K. E. (2015). An introduction to probability and statistics. John Wiley & Sons, Incorporated. (Chapter 7). http://search.ebscohost.com.pxz.iubh.de:808 0/login.aspx?direct=true&db=cat05114a&AN=ihb.45506&site=eds-live&scope=site Triola, M. F. (2013). Elementary statistics. Pearson Education. (Chapter 11). http://search.eb scohost.com.pxz.iubh.de:8080/login.aspx?direct=true&db=cat05114a&AN=ihb.45501& site=eds-live&scope=site Wagaman, A.S. & Dobrow, R.P. (2021). Probability: With applications and R. Wiley (Chapter 9). http://search.ebscohost.com.pxz.iubh.de:8080/login.aspx?direct=true&db=edsebk &AN=2947734&site=eds-live&scope=site UNIT 5 Triola, M. F. (2013). Elementary statistics. Pearson Education. (Chapter 6). http://search.ebs cohost.com.pxz.iubh.de:8080/login.aspx?direct=true&db=cat05114a&AN=ihb.45501&s ite=eds-live&scope=site Wagaman, A.S. & Dobrow. R.P. (2021). Probability: With applications and R. Wiley (Chapter 10). http://search.ebscohost.com.pxz.iubh.de:8080/login.aspx?direct=true&db=edseb k&AN=2947734&site=eds-live&scope=site 9 LEARNING OBJECTIVES Welcome to Statistics - Probability and Descriptive Statistics! This course will provide you with a foundation in mathematical probability, preparing you for further courses in statistical inference and data science. The statistical tools that you will be introduced to in this course will enable you to review, analyze, and draw conclusions from data. You will become familar with the key terms and concepts that are at the core of probability theory, including random experiments, sample spaces, events, and the axioms of probability. You will learn to classify events as mutually exclusive and independent, and how to compute the probability of unions and joint events. You will also learn how to interpret and use conditional probability and apply Bayes’ theorem to selected applications. A random variable is a numerical description of the outcome of a statistical experiment. As a mathematical formalization it quantifies random events. When studying a given data set, we generally consider the data points as an observation of a random occurrence, which can be described by the underlying distribution of a random variable. You will learn to define a random variable and express and interpret its distribution using probability mass functions (PMFs), probability density functions (PDFs), and cumulative distribution functions (CDFs). You will learn about important probability distributions, their characteristics, and how they are used to model real-world experiments. Sometimes data comes in the form of pairs of triples or random variables. The variables in these tuples may be independent or dependent. You will learn how to express the randomness of these tuples using joint distributions, PMFs and PDFs. Marginal and conditional distributions play a key role in isolating the distribution of one variable from the tuple in different ways. You will be provided with examples that will help you to learn how to compute and interpret such distributions. The average and standard deviation are the most popular summaries we can compute from numerical data. These ideas are extended using general notions of the expected value of a random variable as well as other expectation quantities. You will learn how to compute means, variances, general moments, and central moments. More importantly, you will be able to describe certain characteristics of distributions, such as skewness and kurtosis, using these quantities. Finally, you will be introduced to important inequalities and limit theorems. These inequalities and theorems are at the very foundation of the methods of statistical inference, providing a sound framework for drawing conclusions about scientific truths from data. Furthermore, they will be used to define and evaluate performance metrics of learning algorithms in your further studies. Note 10 Given the main focus of this course (on fundamental theories and applications of statistics), it would be preferable for students to have some prior knowledge of basic topics of mathematical analysis (i.e., integral and differential calculus), as well as properties of functions. However, for the sake of completeness, the tools of analysis that are most important for this course will be briefly introduced and discussed at relevant points throughout the course book. 11 UNIT 1 PROBABILITY STUDY GOALS On completion of this unit, you will be able to ... – understand the key terms outcome, event, and sample space and how these terms are used to define and compute probabilities. – identify the three fundamental axioms of probability measures. – compute and interpret probabilities involving mutually exclusive events. – compute and interpret probabilities of two independent events and conditional probabilities. – compute probabilities of two events that are not necessarily independent. – compute probabilities of two events that are not necessarily mutually exclusive. – understand the concept of partitioning a sample space and how it frames the statement of the total law of probability. – apply Bayes’ theorem to real-world examples. 1. PROBABILITY Introduction Probability is the primary tool we use when we are dealing with random experiments: that is, experiments where the outcome cannot be determined with complete certainty (see Wackerly, Mendenhall & Schaeffer, 2008; Wasserman, 2004). Consider rolling a pair of fair 6-sided dice. The outcome of any such roll cannot be determined with absolute certainty. How many possible outcomes are there? Is a sum of five or eight more likely? What is the most likely sum? What is the least likely sum? The tools we discuss in this unit will help address these and other questions. Perhaps you have heard of the phrase “lucky number 7”. The origin of this statement lies in the fact that when a pair of fair dice are rolled, seven is the most likely sum. On completion of this unit, you will be able to quantify this fact. Furthermore, you will be able to develop the relevant concepts much further in order to answer more complex questions. 1.1 Definitions Sample space This is a set containing all possible outcomes of a random experiment. It is usually denoted by Ω or S. Although we cannot predict the outcome of random experiments with absolute certainty, we can write down all the possible outcomes the experiment could have. For the coin toss random experiment, the possible outcomes, also called elements (see Klenke, 2014), are H (heads) or T (tails). The set containing all the possible outcomes is called the sample space of the experiment. We say that an outcome a is an element of Ω and write a ∈ Ω. Now consider the experiment of tossing two coins. One possible outcome could be to observe heads on the first coin and tails on the second coin. We can summarize this outcome as HT . Using this notation, the sample space can thus be written as Ω = HH, HT , T H, T T Outcome This is a single result from a trial of a random experiment. Each element is an outcome of the random experiment. In general, we can denote the outcome of an experiment by ωi, where i ∈ ℕ is just the index of the outcome. In this notation, the sample space can be denoted as Ω = ω1, ω2, …, ωn for n ∈ ℕ and for a finite sample space and Ω = ω1, ω2, … for a countably infinite sample space. In some applications we are interested in a single outcome and want to calculate the probability of that outcome, but sometimes we are interested in a group of outcomes. Therefore, the next term we will define is an event. An event of a random experiment is a set of outcomes. The following notation is used to denote an event A, which is contained in Ω, 14 A ⊆ Ω. An event is also called a set (see Klenke, 2014). The following notation A ⊂ Ω, Event This is a collection of zero or more outcomes of a random experiment. Events are usually denoted using capital letters: A, B, C, … means that the event A is contained in Ω and at least one outcome exists which is not contained in A, but in Ω. For the two-coin toss experiment, perhaps we are interested in the outcomes where the result for the two coins match. In this case, we are talking about the event A = HH, T T . Note that the order of the elements in a set does not matter, so HH, T T = T T , HH . Finally, we can have an event that contains a single outcome: B = HT . Finally, we will introduce two fundamental operations for any events in the sample space Ω. For two events A, B ⊆ Ω, the union of A and B, which is denoted by A ∪ B = x ∈ Ω x ∈ A or x ∈ B is the event of all outcomes contained in A or in B. In addition, the intersection of A and B, denoted by A ∩ B = x ∈ Ω x ∈ A and x ∈ B , is the event in which all outcomes are common to both A and B. Figure 1: A Venn Diagram of Three Events Union The union of the events A and B is also an event containing all outcomes of A and all outcomes of B. Intersection The intersection of the events A and B is also an n event containing all outcomes of A, which are also contained in B. We can also say the intersection of A and B is the event, which contains all outcomes of B, which are also contained in A. Source: George Dekermenjian (2019). 15 Special Events There are two special events that require a mention here. At one extreme, an event may contain nothing, in which case we have the null event or the empty set: ∅ = . At the other extreme, we have the whole sample space itself which, of course, contains all the possible outcomes. Axioms of Probability Probability measure This is used to assign probabilities to events of a given sample space. Now that we have an understanding of the fundamental terms, we are ready to talk about probability. The probability of an event measures the likelihood of observing that an event when, for example, a random experiment is performed. For a given sample space Ω every probability measure P , which maps an event of Ω to a real number, has to satisfy the following four axioms of probability: 1. P ∅ = 0, 2. For any event A ⊆ Ω it holds that P A ≥ 0, 3. For mutually exclusive events A1,A2,A3,… ⊆ Ω, ∞ P A1 ∪ A2 ∪ A3 ∪ … = ∑i = 1 P Ai , 4. P Ω = 1. Mutually exclusive Two events are called mutually exclusive if their intersection yields an empty set. Two events (sets) are mutually exclusive if they have no common outcomes (elements). For non-mutually exclusive events we can deduce, according to the axioms of probability, that P A ∩ B + P A ∪ B = P A + P B for any events A, B ⊆ Ω . Example 1.1 Consider the random experiment of tossing two coins. We will assume that the probability of each outcome is equally likely so that singleton events (events with only one outcome) have equal probability. Since there are four outcomes, the probability of each singleton 1 event is 4 . P HH =P HT =P TH =P TT = 1 4 1 In practice, if an event contains one element, we can just write P HT = 4 , excluding the brackets. 16 Classical Probability There are two approaches to defining probability: the classical (frequentist) approach and the Bayesian approach. We will discuss the classical approach first and then move on to a discussion of the Bayesian approach. Consider a random experiment with n ∈ ℕ equally likely outcomes. In other words, the sample space contains n outcomes Ω = ω1, ω2, …, ωn The probability of an event A = ωi1, ωi2, …, ωim for m, im ∈ ℕ of this experiment is the ratio of the number of outcomes in A to the size of the sample space. We will denote the number of outcomes in A by A so that A = m. P A = A Ω = m n . Suppose a bag contains seven red marbles denoted by r1, r2, …, r7 and three blue marbles denoted by b1, b2 and b3. We will draw one marble out of this bag at random. The sample space for this experiment is Ω = r1, r2, r3, r4, r5, r6, r7, b1, b2, b3 . We are interested in computing the probability that the marble drawn is blue. The event corresponding to drawing a blue marble is A = b1, b2, b3 . The event contains A = 3 outcomes and the sample space contains Ω = 10 outcomes. Therefore, the probability of drawing a blue marble is P A = A Ω = 3 . 10 Let us now verify that this formulation is a valid probability measure. In other words, we need to verify that the axioms of probability are satisfied. 1. P ∅ = ∅ Ω = 0 n = 0 and P Ω = Ω Ω = n n = 1. A 2. If A is an event, then 0 ≤ A ≤ n. Dividing by Ω = n gives 0 ≤ Ω ≤ 1. In other words, we have 0 ≤ P A ≤ 1 as required. 3. Now suppose that A and B are mutually exclusive events. Then the number of elements in the event A or B is the union A ∪ B. Since they are mutually exclusive, it must hold that A ∪ B = A + B, because a marble cannot be in A and B simultaneously. Dividing by Ω we obtain A ∪ B Ω = A Ω + B Ω . In other words, it holds that P A ∪ B =P A +P B as required. 17 We do not have to deal with the case of infinitely mutually exclusive events since our sample space is finite i.e., it consists of 10 marbles. That means if we assume mutually exclusive events such that A1, A2, A3, … ⊆ Ω then only finite events can contain at least one marble. The rest of the sets must be empty sets. Thus, we reduced the problem to finite mutually disjoint events, which can be discussed in the same way as in the case of two mutually disjoint events. Since the classical definition of probability satisfies all probability axioms, it is a valid probability measure. Example 1.2 Consider the random experiment of tossing three coins. Find the probability of observing at least one . Solution 1.2 Recall that the sample space is Ω = T T T , T T H, T HH, HT H, T HT , HT T , HHT , HHH . The event of observing at least one H is exactly the event A = T T H, T HH, HT H, T HT , HT T , HHT , HHH . This event contains A = 7 outcomes. Furthermore, the sample space contains Ω = 8 outcomes. Therefore, the probability of observing at least one H is P at least on H = P A = A Ω = 7 8 = 0 . 875 . Example 1.3 Consider the experiment of rolling a 6-sided die. a) Write down the sample space. b) Write down the event of observing an even number. c) Calculate the probability of observing an even number. Solution 1.3 a) Ω = 1,2, 3,4, 5,6 . b) A = 2,4, 6 . c) P A = A Ω = 3 6 = 1 2 = 0 . 5 = 50 %. Consider the experiment of rolling a pair of 6-sided dice. For each die, we can observe a number from 1 to 6. If we paired the observations from each die, we would have a single observation from the pair. For example, if the first die lands on 2 and the second lands on 5, we can write down this outcome as (2,5). The sample space S of this experiment is shown in the table below. 18 Table 1: Sample Space of Rolling a Pair of 6-Sided Dice (1,1) (1,2) (1,3) (1,4) (1,5) (1,6) (2,1) (2,2) (2,3) (2,4) (2,5) (2,6) (3,1) (3,2) (3,3) (3,4) (3,5) (3,6) (4,1) (4,2) (4,3) (4,4) (4,5) (4,6) (5,1) (5,2) (5,3) (5,4) (5,5) (5,6) (6,1) (6,2) (6,3) (6,4) (6,5) (6,6) Source: George Dekermenjian (2019). The sample space consists of Ω = 36 outcomes. Using this information, let us explore some questions related to this experiment. Example 1.4 Using the information provided in the table above: a) Write down the event of observing the same number on both dice. b) Write down the event of observing numbers that sum to 4. c) Calculate the probability of each of these events. Solution 1.4 a) A = b) B = 1,1 , 2,2 , 3,3 , 4,4 , 5,5 , 6,6 . 1,3 , 2,2 , 3,1 . c) P A = d) P B = A Ω B Ω = = 6 36 3 36 1 = 6. = 1 . 12 Do we have to write down the outcomes? The formula for probability that we are using only makes use of the number of outcomes. As you can imagine, for more complex experiments, the size of the sample space can become very large, and it would not be wise to write down all the possible outcomes. However, to compute the probability we still need to be able to count the number of outcomes, whether it is in the sample space or for another event. To this end, we will take a short departure from our main topic and review some basic counting techniques that will be useful in answering probability questions. Counting All of the formulas we will discuss here are based on one simple principle: the multiplication principle of counting. If there are N 1 ways of performing task 1 and N 2 ways of performing task 2, then there are N 1 · N 2 ways of performing both tasks. This principle is easily extended to more than two tasks. 19 Suppose a pizza parlor offers its patrons the option of customizing their own pizzas. They are offered three types of crusts, two types of sauces, and can also choose one from a selection of five toppings. To count the number of different pizzas one can order at this pizza parlor, we can break down making a pizza into three tasks: (i) there are N 1 = 3 ways of choosing a crust, (ii) there are N 2 = 2 ways of choosing a sauce, and (iii) N 3 = 5 ways of choosing a topping. Therefore, there are N 1 · N 2 · N 3 = 3 · 2 · 5 = 30 ways of making a pizza. Permutations Suppose you have four different books, and you want to arrange them on a shelf. We want to count the total number of arrangements possible. There are four tasks. At first there are four books to set in place. After placing the first book, there are three books to set in place, then two books, and, finally, after placing these, there is one book left to place on the shelf. Therefore, using the multiplication principle, there are 4 · 3 · 2 · 1 = 24 ways of arranging the four books on the shelf. This is an example of a permutation. Using factorial notation, we can write this computation as 4! = 4 · 3 · 2 · 1. In general, if there are n ∈ ℕ different objects to arrange, there are n! = n n − 1 n − 2 · … · 3 · 2 · 1 permutations (arrangements) possible. Now suppose that we have n = 10 objects, but we want to select and arrange k = 3 of them. We can choose the first objects (10 choices), then the second objects (9 choices), and, finally, the third object (8 choices). Therefore, the total number of arrangements is 10 · 9 · 8 = 720. In general, if we have n distinct objects, the number of permutations of k of these objects is n! n−k ! Combinations Suppose there are 10 people at a dinner party and each person shakes the hands of every other person. We want to work out how many handshakes there would be. Using the multiplication rule, we can argue that observing the event of a handshake involves two tasks: (i) the first person in the handshake (10 people available) and (ii) the second person in the handshake (9 people available). So far, we have 10 · 9. However, the order of the people does not matter. If John shakes hands with Mary and Mary shakes hands with John, the handshake is the same. Therefore, we do not need to count these handshakes twice. We divide the expression to get 10 ⋅ 9 2 = 45 handshakes. This is an example of a combination, which is similar to a permutation, but order does not matter. In general, if we have n ∈ ℕ distinct objects, the number of ways of choosing k ∈ ℕ of them is given by 20 n = k n! . n − k !k! n is read as “n choose k”. For the handshake example, this formula k indeed gives the correct answer: The expression 10 = 2 10! 10 − 2 !2! = 10! 8!2! = 45. Now that we have some efficient tools for counting, we are equipped to tackle some more probability questions. Below is one such example. Example 1.5 Suppose there are five women and four men. We will randomly choose three people. a) Calculate the size of the sample space for this experiment. b) How many ways are there of choosing two women and one man? c) What is the probability of choosing two women and one man? Solution 1.5 a) The sample space consists of all possible groups of three people from nine different people. The order does not matter here. The number of ways is “9 choose 3” or Ω = 9 = 3 9! 9 − 3 !3! = 9! 6!3! = 9⋅8⋅7 3⋅2⋅1 = 84 b) Choosing two women and one man is actually two tasks. We will count the number of ways of performing each task and then multiply them (using the multiplication principle). Task 1: Choosing two women from five. There are Task 2: Choosing one man from four. There are 4 1 5 2 = 10 ways. = 4 ways. According to the multiplication rule, there are 10 · 4 = 40 ways of choosing two women and one man. c) Let us call the event of choosing two women and one man A. We found that A = 40. Therefore, the probability of this event is P A = 40 84 ≈ 0.4762 = 47.62 % . Complementary Events The complement of an event, just like the complement of a set, is the event of not observing the included outcomes. For example, in a dice roll experiment we have the sample space Ω = 1,2, 3,4, 5,6 . If A is the event of observation 1 or 2 A = 1,2 , then the c complement of A is A = 3,4, 5,6 . 21 2 c The probability of A is P A = 6 , and the probability of its complement is P A c Indeed, we have P A + P A = 2 6 4 6 + 4 = 6. = 1. This means that for a given sample Ω it holds that c P A +P A = 1 for any A ⊆ Ω . 1.2 Independent Events Consider the experiment of tossing a fair coin and then rolling a fair 6-sided die. The prob1 Independence of two events Two events are independent if the probability of their intersection yields the same as the product of each probability. 1 1 ability of observing the joint event H, 2 is 2 ⋅ 6 = 12 . That is, we multiply the probabilities. This is because the tossing of a fair coin does not influence the result of rolling a die. The two events are independent. More formally, for a given sample space Ω, two events, A ⊆ Ω and B ⊆ Ω, are said to be independent if P A∩B =P A ·P B . Example 1.6 Suppose we draw two cards at random with replacement from a standard deck of 52 cards. That is, we draw the first card, place it back in the deck, and then draw another card. What is the probability that both cards are spades? Solution 1.6 The event of the first card being a spade is independent from the second card being a spade. Therefore, the probability of both being spades is 13 52 · 13 52 = 1 16 = 0 . 0625 = 6.25 % . Suppose the two events A and B with P A , P B > 0 are disjoint (mutually exclusive). Can they be independent? If the two events are mutually exclusive, then they cannot both occur at the same time, so the probability of the joint event is P A ∩ B = 0. Therefore, the two events are not mutually exclusive, since 0 = P A ∩ B ≠ P A · P B > 0. Example 1.7 Suppose a fair coin is tossed five times. What is the probability of observing at least one tail? 22 Solution 1.7 Note that each of the tosses is independent. Furthermore, it is easier to work with the complement of this event. Let A be the event of observing at least one tail. Then, the comc plement event A is the event of observing no tails (that is, observing heads on each of the five tosses). Let Hi denote the event of observing heads on the itℎtoss for i ∈ ℕ, and then use the formula for the probability of complements. We then have c P A =1 − P A =1 − P H1H2H3H4H5 =1 − P H1 P H2 P H3 P H4 P H5 =1 − 1 5 2 = 31 32 ≈ 0.9688 = 96.88 %. We have used independence in the third equality. In the fourth equality, we used the fact 1 that the probability of observing heads in any toss is 2 . Example 1.8 A bag contains three red marbles and five blue marbles. Two marbles are drawn, one after the other, without replacement. Is the event of observing a red marble on the first draw and a blue marble on the second draw independent? Why or why not? Solution 1.8 The two events are not independent. The result of the first event will change the number of available marbles in the bag, since there is one marble missing for the second draw. We will see how to calculate the probability of joint events that are dependent in the following section. 1.3 Conditional Probability Conditional probability is a way of calculating the probability of an event using prior information. The notation P A B is read as the “probability of A given that we have already observed B”. In other words, while P A is the (unconditional) probability of observing A, P A B is the conditional probability of A conditioned on B. Suppose we have three red marbles and five blue marbles in a bag. We draw two marbles at random without replacement. Let A denote the event of observing a red marble. Let B denote the event of observing a blue marble. The probability of B given that we have already observed A is written as P B A . After observing A, a red marble, there are only seven marbles left in the bag: 5 5 two red and five blue. Therefore, the probability P B A = 7 . In contrast, P B = 8 . Thus for a given sample space Ω, we say that the conditional probability of A ⊆ Ω conditioned on B ⊆ Ω with P B > 0, is defined by 23 P A B = P A∩B P B . Example 1.9 Suppose that the probability of a randomly chosen person having cancer is 1%, and that if a person has cancer, a medical test will yield a positive result with a probability of 98%. What is the probability that the person has cancer and the medical test result shown is positive? Solution 1.9 Let A denote the event that a person has cancer. We know that P A = 0.01. Now let B denote the event that the medical test yields a positive result. We want to find P A ∩ B . We know the conditional probability P B A = 0.98. Using the formula for conditional probability we have P B A = P B∩A P A . Rewriting this formula, we have P A ∩ B = P B A · P A = 0.98 · 0.01 = 0.0098 = 0.98 % . If the two events A and B are independent, then observing one of the events gives us no information about the other event. In other words, P A B = P A . Indeed, we can show this using the result of independent events and the formula for conditional probability as follows: P A∣B = P A∩B P B = P A ⋅P B P B =P A for P B > 0 . Let us revisit the experiment of drawing two cards. Example 1.10 Suppose two cards are drawn out of a deck of 52 cards, one after the other, without replacement. What is the probability that both are spades? Solution 1.10 Let S1 denote the event that the first card is a spade and let S2 denote the event that the second card is a spade. Since these two events are dependent, think about why this is the case. We can use the conditional probability formula in the form P S1 ∩ S2 = P S2 ∣ S1 ⋅ P S1 . 24 The left-hand side is the probability that we observe a spade on both draws. The first factor on the right denotes the probability that the second card is a spade given that the first card was a spade. The last factor is the probability that the first card is a spade. Since there 13 are 13 spades out of 52 cards, we have P S1 = 52 . After having observed a spade, there are only 12 spades left in the deck of a total of 51 cards. Therefore, P S1 S2 = 12 . 51 Therefore, P S1 ∩ S2 = 12 51 ⋅ 13 52 = 1 17 ≈ 0.0588 = 5.88 % . Compare this answer with Example 1.6. Does the result surprise you? 1.4 Bayesian Statistics In contrast to classical statistics, Bayesian statistics is all about modifying conditional probabilities – it uses prior distributions for unknown quantities which it then updates to posterior distributions using the laws of probability. Let us revisit the medical cancer test in Example 1.9. Let us say a randomly chosen person tests positive for cancer. What is the probability that they actually have cancer? Biomedical tests are never perfect; there is typically a small false positive and false negative rate. In the setting of the example, recall that A represents the event that a person has cancer and B represents the event that the medical test returns a positive result. We were given the prevalence of the disease in the general population, which was 1%, so that P A = 0.01. The test is 98% accurate for people who actually have the disease—that is, P B A = 0.98. Finally, suppose the test gives a false positive 20% of the time. We are now interested in finding out P A B . This is the subject of Bayes’ theorem. Before discussing Bayes’ theorem, let us first write down a preliminary result. To motivate the result, suppose that we partition the sample space Ω into disjoint events A1, A2, and A3. That is, these events are mutually exclusive, and together they contain all the outcomes in the sample space, meaning A1 ∪ A2 ∪ A3 = Ω. Partition of an event Let A be an event. When the union of two or more mutually exclusive events is A, the group of events is called a partition of A. Now consider another event . Then it holds B = A1 ∩ B ∪ A2 ∩ B ∪ A3 ∩ B meaning that the events A1 ∩ B, A2 ∩ B, and A3 ∩ B partition the event B. In other words, these events are mutually disjoint and together they contain all of B. See the figure below for an illustration. 25 Figure 2: Partitions Source: George Dekermenjian (2019). Theorem: The Law of Total Probability Let A1, A2, A3, … be a countably infinite collection that partitions the sample space Ω. In other words, the events A1, A2, A3, … are pairwise mutually exclusive and ∞ ∪ Ai . i=1 Let B ⊆ Ω be another event. Then it follows that ∞ P B = ∑i = 1 P Ai ∩ B . or, equivalently, ∞ P B = ∑i = 1 P B Ai P Ai . We are now ready to state one of the most important theorems in modern probability theory. Theorem: Bayes’ Theorem Let A1, A2, A3, … ⊆ Ω be a countably infinite set of a partition of a sample space Ω such that P Ai > 0 for all i ∈ ℕ. Then for fixed Aj with j ∈ ℕ and B ⊆ Ω such that P B > 0, it holds that 26 P Aj B = P B Aj P Aj ∞ ∑i = 1 P B Ai P Ai . Proof We know for the conditional probability formula yields P Aj B P B = P B Aj P Aj . Dividing by P B and we use the Law of Total Probability for the event B ⊆ Ω yielding the result. Note that as a special case of Bayes’ theorem, we can apply the results with just two c events, A and B, and use the two events A and A as the partition. In this case, the result is reduced to P A B = P B AP A c c P B A P A +P B A P A . Example 1.11 Suppose that the probability of a randomly chosen person having developed cancer is 1% given that if a person has cancer, a medical test will yield a positive result with a probability of 98%. Also, given that if a person does not have cancer, the test will yield a negative result with a probability of 0.80. Now, suppose a randomly chosen person tests positive. What is the probability they have actually developed cancer? Solution 1.11 Let A denote the event that a person has cancer. We know that P A = 0.01 and c P A = 0.99. Let B denote the event that the test returns a positive result. We know that c P B A = 0.98 and P Bc A c P B A = 0.80. We want to find P A B . Note that = 1 – 0.80 = 0.20. Now, using Bayes’ theorem, we have P A B= P B AP A c P B A P A +P B A P A c = 0.98 ⋅ 0.01 0.98 ⋅ 0.01 + 0.20 ⋅ 0.99 0.0098 = 0.0098 + 0.198 ≈ 0.0472 = 4.72%. Note that the result in Solution 1.11 is very low and applies to biomedical tests with significant false positive and false negative rates, making population-wide screening programs of relatively rare diseases with such tests pointless. Tree diagrams and two-way tables help us understand how the total law of probability, Bayes’ theorem, and applications such as the one in Example 1.11 work. Below is an example of such a probability tree together with the associated two-way table. 27 Figure 3: The Probability Tree Diagram from Example 1.11 Source: George Dekermenjian (2019). Table 2: Table of Probabilities from Example 1.11 True Diagnosis Total Medical test result Cancer No Cancer Positive 0.0098 0.1980 0.2078 Negative 0.0002 0.7920 0.7922 0.01 0.99 1 Total Source: George Dekermenjian (2019). Now consider a sample with a size of 10,000. The natural frequencies corresponding to the probabilities help us get a “feel” for how these types of probabilities impact a real-world data set. Below is a tree diagram with natural frequencies followed by the corresponding two-way table. 28 Figure 4: The Tree Diagram of Natural Frequencies from Example 1.11 Source: George Dekermenjian (2019). Table 3: Table of Natural Frequencies for a Sample Size of 10,000 from Example 1.11 True Diagnosis Total Medical test result Cancer No Cancer Positive 98 1980 2078 Negative 2 7920 7922 100 9900 10,000 Total Source: George Dekermenjian (2019). In Bayes’ theorem, P A is interpreted as the prior probability while P A B is the posterior probability. So, for the example above, before knowing the test result, we could say with 1% probability that the person has cancer, but after getting the result of the test, we could say that, based on the new information, the probability that the person has cancer is almost 5%. 29 SUMMARY Several fundamental concepts were introduced in this unit, including random experiment, outcome, event, sample space, probability axioms, and counting techniques. We used these concepts to compute probabilities of certain events for simple experiments. Mutually exclusive events and the sum of probabilities axiom were used to compute probabilities of unions of events: P A ∪ B = P A + P B for mutually exclusive events A, B ⊆ Ω . When events are not mutually exclusive, a general sum of probabilities rule gives P A ∪ B = P A + P B – P A ∩ B for any events A, B ⊆ Ω . The joint event A ∩ B led to a discussion of independent events in which case we have the product of probabilities rule P A ∩ B = P A · P B for any independent events A, B ⊆ Ω . When two events A and B are not independent, P A and P A B are not the same. Therefore, we introduced the conditional probability of A ⊆ Ω conditioned on B ⊆ Ω by P A B = P A∩B P B where P B > 0 . This definition can be interpreted as a general product of probabilities for events that are not necessarily independent. Bayes’ rule is central to understanding Bayesian probability. We discussed instances where a collection of events partitions a sample space and how such a collection induces a partition of any event. These ideas led to an important theorem known as the law of total probability. Finally, building on this theorem, we introduced Bayes’ theorem and discussed a number of applications. 30 UNIT 2 RANDOM VARIABLES STUDY GOALS On completion of this unit, you will be able to ... – describe and compare the properties of discrete and continuous random variables. – understand the roles of PMFs and CDFs for discrete distributions and their properties. – understand the roles of PDFs and CDFs for continuous distributions and their properties. – apply PMFs, PDFs, and CDFs to answer probability questions. – identify important discrete distributions and important continuous distributions. 2. RANDOM VARIABLES Introduction Random variable This is a rule (function) which assigns outcomes of a given sample space to a real number. The sample space is equipped with a probability measure such that the outcomes or events have a defined likelihood. In real-world applications of data analysis and statistics we work with numerical data. In order to describe the occurrence of data points, a mathematical model or formalization, called a random variable, is necessary. From a scientific point of view, we assume that the data points are realizations of random variables. Each random variable has a specific sample space, probability measure and therefore, distribution, which describes the frequency of occurrence of our data points. A random variable is different from traditional variables in terms of the value it takes. It is a function which performs the mapping of the outcomes of a random process to a numeric value. Given their importance, the main subject of this unit will be random variables (see Wackerly, Mendenhall & Schaeffer, 2008) and their mathematical properties. Random variables have many real-world applications and are used, for example, to model stock charts, the temperature, customer numbers, and the number of traffic accidents that occur in a given timeframe or location. 2.1 Random Variables Informally, a random variable is a rule that assigns a real number to each outcome of the sample space (see Wasserman, 2004). We usually denote random variables using the capital letters X, Y , Z . When appropriate, we sometimes also use subscripts: X1, X2 and so on. Consider the random experiment of tossing a fair coin four times. Let X be the random variable that counts the number of heads. For the outcome HHT H, we have X HHT H = 3 and for another outcome T T T H, we have X T T T H = 1. Now consider the random experiment of rolling two fair 6-sided dice. Let Y denote the random variable that adds the numbers observed from each of the dice. For example, Y 1,2 = 3 and Y 4,4 = 8. Finally, the same random experiment can have many different random variables. For example, for the experiment of rolling two 6-sided dice, let M denote the random variable that gives the maximum of the numbers from the dice. For example, M 1,2 = 2 and M 5,2 = 5. Since the values of a random variable depend on the outcome, which is random, we know that the values of a random variable are random numbers. So far, we have made the connection between the value of a random variable and an outcome of the random experiment. Before moving onto events, we will make this relationship more formal. For a given sample space Ω, equipped with a probability measure P , a random variable X is a mapping from the sample space Ω to the set of real numbers ℝ that assigns for each outcome ω ∈ Ω a real number x ∈ ℝ. In standard notation, this is written as 32 X :Ω ω ℝ, x=X ω . This is the most abstract definition of a random variable we can encounter. For our purposes we will restrict ourselves to discrete and piecewise continuous random variables and describe a wide range of random variables. For random variables with a finite sample space, we can write down the possible values of a given random variable. Consider the random experiment of tossing three coins; let X denote the random variable which counts the number of tails. The table below gives the values of each of the outcomes. Table 4: Values of the Random Variable Counting Tails When a Coin is Tossed Three Times ω X ω HHH 0 HHT 1 HTH 1 THH 1 HTT 2 THT 2 TTH 2 TTT 3 Source: George Dekermenjian (2019). Now we want to establish the connection of random variables with events. Consider the equation X ω = 1 from the table above. There are three outcomes ω that fit this equation. If we put these three outcomes in a set, it becomes an event. More formally, the event X ω = 1 corresponds to the event HHT , HT H, T HH . We can also write this relationship as X–1 1 = HHT , HT H, T HH . Here, X–1 denotes the inverse relation. It takes a value (from ℝ) to an event (in Ω). 33 Figure 5: The Random Variable as a Mapping from the Sample Space to Real Numbers Source: George Dekermenjian (2019). Figure 6: The Inverse Mapping Source: George Dekermenjian (2019). It is standard practice to use shorthand notation when describing events using random variables. Formally, in the example above, the event X ω = 1 describes the event ω ∈ Ω X ω = 1 . However, in practice we usually write this event as X = 1 , meaning that we have the following notation for the event that the random variable X equals one X ω =1 = ω∈ΩX ω =1= X=1 . Since our sample space Ω is equipped with a probability measure P , we can ask ourselves how likely this event is. Consequently, the symbolic form of writing a probability such as “the probability of observing one tail in a sequence of three tosses of a coin” would be written as P X = 1 . When we talk about the probability of all such (single value) events, we are describing a probability mass function. We will look at these functions in the next two sections. 34 Sometimes we are interested in events corresponding to multiple values of a random variable. The event of observing 0 or 1 tail can be written as 0 ≤ X ω ≤ 1 = X − 1 0,1 = ω ∈ Ω 0 ≤ X ω ≤ 1 , which is written in shorthand as 0 ≤ X ≤ 1 . Figure 7: The Inverse Mapping of a Set of Values Source: George Dekermenjian (2019). An important range of values that comes up in the study of probability distributions is the range of values up to and including a specified number, such as X ≤ 1 or X ≤ 2 . For our example above, the former is equivalent to 0 ≤ X ≤ 1 and the latter is equivalent to 0 ≤ X ≤ 2 . When we speak about the probability of such events, we are describing a distribution function. This is the subject of the next section. 2.2 Probability Mass Functions and Distribution Functions In the previous section, we defined a random variable as a rule that connects each outcome of a sample space to a real number. In this section, we will continue building the connection by taking the values of a random variable and connecting them to probabilities. Probability Mass Functions For a given sample space Ω and its corresponding probability measure P , we consider a random variable X: Ω x1, x2, x3, … , where x1, x2, … are real numbers. This random variable is called a discrete random variable, because the set of possible values is countable infinite or finite. Now we consider a function 0,1 . If the function f satisfies the following properties f : x1, x2, x3, … Discrete random variable A random variable which takes only finite or countably infinite values. • P ω ∈ Ω X ω = i = P X = i = f xi for all i ∈ ℕ, ∞ • ∑i = 1 f xi = 1, 35 Probability mass function A series which determines the likelihood that a discrete random variable takes a value. f is then called a probability mass function (PMF). In that case the support of the PMF consists of all xi ∈ ℝ such that P ω ∈ Ω X ω = i = P X = i = f xi > 0 . When we are working with multiple random variables, we can write fX instead of f to specify the random variable to which the PMF refers. Example 2.1 Consider the experiment of tossing a fair two-sided coin three times. Let X denote the random variable that counts the number of tails. Write down the PMF f of X defined by fX x = P X = x . Solution 2.1 The possible values of X are 0, 1, 2, 3 . The table below summarizes the PMF. Table 5: Values, Events, and PMF of Tossing a Fair Coin Three Times x X=x fX x = P X = x 0 HHH 1/8 1 HHT , HT H, T HH 3/8 2 HT T , T HT , T T H 3/8 3 TTT 1/8 Source: George Dekermenjian (2019). Note that each value f x is non-negative and f 0 + f 1 + f 2 + f 3 = 1. Therefore, f is indeed a valid PMF. 36 Figure 8: A Plot of the PMF from Example 2.1 Source: George Dekermenjian (2019). Example 2.2 Suppose that f is a PMF defined using the table below. What is f 3 ? Table 6: A Discrete PMF with a Missing Value x f x 1 0.2 2 0.05 3 ? 4 0.39 5 0.01 6 0.05 7 0.05 8 0.10 Source: George Dekermenjian (2019). 37 Solution 2.2 Since we are told that this is a probability mass function, we know that f 3 ≥ 0 and f 1 + f 2 + f 3 + … + f 8 = 1. Therefore, the second equation reduces to 0.85+f 3 = 1 which gives f 3 = 0.15. Probability mass functions can be represented graphically as point plots with the horizontal axis containing the values of the random variable and the vertical axis containing the values of f . Below is a plot of the PMF for Example 2.2. Figure 9: A Plot of the PMF from Example 2.2 Source: George Dekermenjian (2019). Cumulative Distribution Function Cumulative distribution function A CDF of a random variable X is a function which measures the probability that X will take a value less or equal to x for fixed x. In this section, we consider events corresponding to values of the random variable in the form X ≤ x . Given a random variable X, the cumulative distribution function (CDF) is defined by F X x = P X ≤ x for any x∈R . Formally, a CFD is a function F X such that F X : ℝ 0,1 . We also write F instead of F X if the random variable is clear from the context. In addition, we can prove that for any CDF the following three properties must hold: • F is normalized: x lim and 38 −∞ F x =0 x lim F x = 1 ∞ • F is non-decreasing: F x1 ≤ F x2 for x1 < x2 • F is right-continuous: F x = lim t = x, t > x F t . We can verify the three properties in an intuitive way. For the first property, when x tends to negative infinity, then the set of outcomes that are in the event X ≤ x become the empty set. Also, as x tends to positive infinity, the set of outcomes in the event become the whole sample space, in which case the probability is 1. For the second point, notice that if an outcome is in the event X ≤ x1 and x1 ≤ x2, then automatically, this same outcome must be in X ≤ x2 , which basically means X ≤ x1 ⊆ X ≤ x2 for x1 ≤ x2 . Therefore, the former event is a subset of the latter one. Hence, the former event is, at most, as probable as the latter. For the final property, take t > x, then we have F t −F x =P X ≤t −P X ≤x =P x<X ≤t . The event x < X ≤ t becomes the empty set as t approaches x. Therefore, the probability of this event tends to zero. Example 2.3 Suppose X is a random variable with values in Ω = 0,1,2,3,4 and with PMF f x = 1/5 for all x ∈ Ω. Such a random variable is said to have a discrete uniform distribution. Write down the CDF F x of X and sketch its graph. Solution 2.3 If x < 0, then F x = P X ≤ x = 0 since X cannot take on values outside the given set. If F x = P X ≤ x = P X = 0 = f 0 = 1/5. 0 ≤ x < 1, then For 1 ≤ x < 2F x = P X ≤ x = P X = 0 + P X = 1 = f 0 + f 1 = 2/5 . Continuing in this way, we obtain 39 0 x < 0, F x = 1 5 0 ≤ x < 1, 2 5 1 ≤ x < 2, 3 5 2 ≤ x < 3, 4 5 3 ≤ x < 4, 1 x ≥ 4. given that either the PMF or the CDF of a random variable completely describes everything we may want to know about the random variable. 2.3 Important Discrete Random Variables In this section, we will discuss important discrete random variables, their probability mass functions, and cumulative distribution functions. The Discrete Uniform Distribution A random variable X, which takes on a finite number of integer values, such as 1,2, …, K for K ∈ ℕ, with each value being equally likely, is said to follow a discrete uniform distribution written f as X Uniform 1,2,3,…, K . The probability at each of these integers is P X = k = 1/K for k ∈ 1,2,…, K, and otherwise it is zero. Thus the PMF is f x = 1 K x = 1,2, . . . , K, 0 otherwise. Below are some PMF graphs for different discrete uniform distributions. 40 Figure 10: Plots of Various Discrete Uniform PMFs Source: George Dekermenjian (2019). The distribution function F x = P X ≤ x is given by F x = 0 x < 1, x K 1 ≤ x < K, 1 x ≥ K. Note that the distribution function is non-decreasing, right continuous, and F x x ∞. 1 as Example 2.4 Let X represent the face value of the roll of a fair six-sided die. Write down the PMF and CDF of X. Sketch a graph of both the PMF and CDF. 41 Solution 2.4 The possible values that X can take are 1,2,3,4,5,6 . Furthermore, since this is a fair die, each of the values is equally likely. Therefore, X Uniform 1,2,3,4,5,6 . Its PMF is given by f x = 1 6 for x ∈ 1,2,3,4,5,6 , 0 otherwise. The CDF of X is given by F x = 0 for x < 1, x 6 for 1 ≤ x < 6 1 for Below is a graph of the PMF for Example 2.4. Figure 11: A Plot of the PMF of Example 2.4 Source: George Dekermenjian (2019). We can further simplify this expression to get 42 6 ≥ x. 0 F x = x < 1, 1 6 1 ≤ x < 2, 2 6 2 ≤ x < 3, 3 6 3 ≤ x < 4, 4 6 4 ≤ x < 5, 5 6 5 ≤ x < 6, 1 x ≥ 6. Below is a graph of the CDF for Example 2.4. Figure 12: A Plot of the CDF for Example 2.4 Source: George Dekermenjian (2019). The next few discrete distributions are based on the so-called Bernoulli trial. The Bernoulli trial (or experiment) is a fundamental building block for some of the distributions we will consider in this section. As such, we begin our discussion with this distribution, which is arguably the simplest discrete distribution. Bernoulli Trial A Bernoulli trial, or a Bernoulli experiment, is an experiment that has exactly two possible outcomes. These outcomes are typically labeled “success” and “failure”. Suppose the probability of “success” is p for 0 ≤ p ≤ 1 and, consequently, the probability of “failure” is 1 – p. Now, consider a random variable X defined on this sample space such that X success = 1 and X failure = 0. The PMF of this random variable is given by f 1 = p and f 0 = 1 – p. Such a random variable is called a Bernoulli random variable with parameter p, written as X Bernoulli( p). Formally, the PMF of the Bernoulli distribution is given by 43 1 − p for x = 0, f x = p for x = 1, 0 otherwise. Figure 13: A Plot of Various Bernoulli PMFs Source: George Dekermenjian (2019). We notice that the PMF of that random variable is 0,1 . That means it is non-zero on that set. The distribution function is given by 0 if x < 0, F x = 1 − p if 0 ≤ x < 1, 1 x ≥ 1. 44 Figure 14: A Plot of the CDF for Bernoulli (0.6) Source: George Dekermenjian (2019). The Binomial Distribution Suppose we repeat a Bernoulli trial five times and each trial is independent and has the same probability of success p. Let X be the random variable which counts the total number of successes for these five trials. For example, we can have an outcome such as “FFFFF”, no successes, in which case X = 0. We could have “SSSSS”, five successes, in which case X = 5. These are the two limiting cases. In particular, X can take on values from the set 0,1,2,3,4,5 . Using independence, we can compute the PMF value at 0 as f 0 =P X = 0 = P F F F F F 5 = 1–p ⋅ 1–p ⋅ 1–p ⋅ 1–p ⋅ 1–p = 1–p . Note that we have used the independence assumption in the third equality. Similarly, the PMF value at 5 is f 5 = P X = 5 = P SSSSS = p5. In an effort to compute the value of the PMF for the other values, let us first consider an outcome that has two successes: 3 P SF SF F = p ⋅ 1 – p ⋅ p ⋅ 1 – p ⋅ 1 – p = p2 1 – p . This is not the only outcome with two successes. There are some others: “SSFFF”, “FSSFF”, “FFSSF”, and so on. How many such outcomes are there? We answer this using the combinations formula: counting the number of ways the two successes can occur is like choosing two positions out of five where the successes will appear, and order does not matter. 5 This is given by . Therefore, the probability of observing two successes is 2 f 2 =P X=2 = 5 2 3 p 1−p . 2 45 Let us examine this expression in more detail: 5 2 p2 1−p 3 # outcomes with 2 successes probability of 2 successes