COM 201 Lecture- Introduction to Biostatistics PDF
Document Details
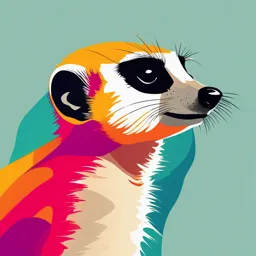
Uploaded by LyricalSheep
Dr. A.G. Omisore
Tags
Summary
This lecture introduces biostatistics, focusing on definitions, uses, variables, and data measurements in public health. It covers descriptive and inferential statistics, methods like frequency tables and diagrams, and examples in medical research, including the work of John Graunt and William Farr.
Full Transcript
Introduction to Biostatistics: Definition, uses, concept of variable and data, measurements in Public Health. DR. A.G. OMISORE. FWACP, MPH, M.B.CH.B STATISTICS Statistics is the science of data. It involves collecting, analysing/ summarizing, interpreting a...
Introduction to Biostatistics: Definition, uses, concept of variable and data, measurements in Public Health. DR. A.G. OMISORE. FWACP, MPH, M.B.CH.B STATISTICS Statistics is the science of data. It involves collecting, analysing/ summarizing, interpreting and presenting data, Using them to estimate the magnitude (level) of associations and test hypothesis. Statistics The discipline concerned with the treatment of numerical data derived from groups of individuals (P. Armitage). The science and art of dealing with variation in data through collection, classification and analysis in such a way as to obtain reliable results ( JM Last). STATISTICS Broadly divided into two - Descriptive - Inferential. Descriptive Statistics- deal with description of characteristics(s) of a finite population Inferential statistics makes deduction from a sample of a population to the population. Methods of Descriptive Statistics Descriptive statistics are those which summarize patterns in the responses of people in a sample. Frequency Tables Diagrams (Graphs/charts) Summary Indices INFERENTIAL STATISTICS 6 Statistical inference is the act of generalizing from a sample to a population with calculated degree of certainty. The importance of inference during data analysis is important The two traditional forms of statistical inference are estimation and hypothesis testing. Estimation predicts the most likely location of a parameter and hypothesis testing ("significance" testing) provides an answer to a statistical question Examples of Inferential Statistics 7 Measures of Association (Chi square) T Test (Paired and Independent) One Way Analysis of Variance Multi-way ANOVA Regression Analysis Correlation Analysis Factor analysis etc MEDICAL STATISTICS/ BIOSTATISTICS Provides appropriate methods for: Collecting, Organizing, Analyzing, Interpreting and Presenting Medical and Health data. BENEFIT OF STATISTICS Has a central role in biomedical investigations Better way of organizing information on a wider and more formal basis (empirical evidence) than exchange of anecdotes and personal experience Takes into account the intrinsic variation inherent in most biological processes (e.g. blood pressure) BENEFIT OF STATISTICS IN HEALTH Measurement of population health Allow for comparisons of the state of health between one period or the other, or between one location and another Thus, statistical presentations/ reports provide the basis for health planning. Actions taken on health issues usually dependent on relevant health information BIOSTATISTICS IN PUBLIC HEALTH HISTORY JOHN GRAUNT John Graunt- founder of vital statistics 1662 Publication - quantified pattern of disease/ mortality in population. Publication based on “Bills of mortality” – a weekly count of people who died since 1592 Observed excess of male births High infants mortality Proportional mortality Seasonal variation in mortality Urban-rural variation William Farr Compiler of statistical abstracts in Britain from 1839- 1880. Annual counts of births, marriages& deaths done Used these as numerators and census data as population data. Thus, crude rates were obtained. Examined the effects of altitude, location (densely& sparsely populated areas)& marital status on John Snow in 1854- Formulated and tested hypothesis concerning the origins of Cholera epidemics in London 20 years before microscope was discovered Described as the father of Field epidemiology Used spot maps to show case distribution Demonstrated water was the source of infection Remove handle of pump to control epidemics Table 1: John Snow’s Table on Cholera Epidemic in London Number of Deaths from Deaths per houses Cholera 10,000 houses Southwark 40,046 1,263 315 & Vauxhall Coy. Lambeth 26,107 98 37 Company Rest of 256,423 1,422 59 London PERTINENT DEFINITIONS IN STATISTICS POPULATION: A set of units (usually People, Objects, Transactions or Events) that we are interested in studying. SAMPLE: A subset of the units of the population. VARIABLE: is a characteristic or property of an individual population unit. A variable is that whose value varies or changes DATA: obtained by measuring the values of one or more variables on the units in a sample. Populations and samples Usually, data is collected on a sample from larger group called the population/universe Samples are not of interests on their own rights, but for what they can tell about the population. Statistics allow us to use the sample to make inferences about the population from which the sample was drawn. Different samples from the same population may give different results due to chance or sampling variation. Samples have to be drawn in a truly representative manner Types of variables: Quantitative & Qualitative Numerical (Quantitative) variables- measured on a naturally occurring numerical scale. Two types- – continuous i.e. measured on a continuous scale including fractions and decimals e.g. age, weight, height etc - discrete i.e. can only take limited numbers of values, usually whole numbers e.g. episodes of diarrhoea in a child, number of men in a village. Types of variables Categorical (Qualitative) variables – non numerical e.g. place of birth, ethnic group, social class, gender - dichotomous (binary) – has only 2 possible outcomes e.g. sex (M/F), survival status (A/D) - ordered categorical – has a natural ordering, but not in a numerical sense e.g. social class (I, II, III, IV, V) ELEMENTS OF STATISTICAL INFERENCE Population Sample One or more variables of interest Reliability RELIABILITY Measures how good a inference is. In using a sample, we introduce an element of uncertainty into our inferences. As much as possible, it is important to determine& report the reliability of each inference made. An inference is incomplete without a measure of its reliability RELIABILITY Measure of reliability that accompanies an inference separates the science of statistics from the art of fortune telling. Measure of reliability- is a statement (usually quantified) about the degree of uncertainty associated with a statistical inference e.g. 95% Confidence interval. NATURE OF STATISTICAL DATA 24 Primary and Secondary data - Primary data: Data originally collected in the process of any statistical inquiry - Secondary data: Data collected by other individual/people/organization Primary source is preferred to secondary source. SOURCES OF HEALTH DATA Census Vital Registration systems Institutions (school health, hospitals, health centers, Veterinary Clinics). Notification centers/ Epidemiological surveillance - (infectious diseases, cancer registries etc). Surveys- CENSUS National census- enumeration of the whole populace in a country, usually done every ten years. The last census in Nigeria was in 206& the popn. Was 140,003,542 North West zone- 35,786,944 most populous followed by South West - 27,581,992 Osun state has a population of 3,423,535 representing 2.45% of Nigeria’s population. Census enables us to calculate crude or total population rates. Figure 1: Population pyramid, Oriade HDSS. >=105 0.13 0.1 100-104 0.11 0.24 95-99 0.12 0.18 90-94 0.4 0.6 85-89 0.6 0.73 A 80-84 1.29 1.88 75-79 1.25 1.29 G 70-74 2.22 2.63 E 65-69 1.71 2.23 60-64 2.47 3.41 55-59 2.05 2.4 FEMALE G 50-54 3.82 4.09 MALE R 45-49 3.62 3.31 40-44 5.26 5.24 O 35-39 5.33 5.68 U 30-34 6.15 6.74 25-29 6.66 7.81 P 20-24 7.31 7.98 15-19 10.82 10.12 10_14 12.85 10.95 05_09 14.97 12.81 0-4 10.81 9.59 20 15 10 5 0 5 10 15 Proportionate Percent population by sex VITAL STATISTICS Records of vital events- births, deaths, marriages& divorces- obtained by registration. Used for generating birth and mortality rates for whole populations or subgroups. Non existing or ineffective “Vital Registration systems” in Nigeria Thus, lack of relevant up to date health and demographic information. Data on important indicators of development e.g. IMR, U-5MR& MMR are estimated. INSTITUTION BASED RECORDS School health records Pre-employment screening (occupational setting) Hospital based records SURVEYS. Usually ad-hoc, but may be routine. Popular National surveys- - NDHS (National Demographic and Health Survey) - HIV Sero prevalence Sentinel study among pregnant women - NARHS (National HIV/AIDS and Reproductive Health Survey). Surveys can be conducted by individual or group of researchers, organizations, governments etc. NATIONAL DEMOGRAPHIC HEALTH SURVEY 1999- 2013 The NDHS is a national sample survey designed to provide up-to-date information on background characteristics of the respondents; fertility levels; nuptiality; sexual activity; fertility preferences; awareness and the use of family planning methods; breastfeeding practices; nutritional status of mothers and young children; early childhood mortality and maternal mortality; maternal and child health; and awareness and behaviour regarding HIV/AIDS and other sexually transmitted infections. The target groups were women age 15-49 years& men age 15-59 years in randomly selected households across Nigeria. Information about children age 0-5 years was also collected, including weight and height. Measurement The way variables are measured 32 is very important. Measurement is the assignment of numbers to a variable Measurement determines the choice of relevant statistical method Scales of Measurement Nominal (non- numerical/qualitative) Ordinal (non- numerical/qualitative) Interval (numerical/quantitative) Ratio (numerical/quantitative) Scales of Measurement Nominal scale – lowest level of measurement. Merely classifies the measure into mutually unordered categories; has no notion of numerical magnitude e.g. gender (male, female), blood group (A, B, AB, O) Nominal Variable 35 Classifies persons or objects into two or more categories Members of a category have at least one common characteristic. We cannot quantify or even rank-order those category. For identification purpose, nominal variables are often represented by numbers. The values of the scale have no 'numeric' meaning in the way that you usually think about numbers. Examples of Nominal Variables 36 Variables Categories Assigned code Sex Male 1 Female 2 Residence Rural 1 Urban 2 HIV Status Positive 0 Negative 1 Scales of Measurement Ordinal scale – in addition to its nominal property, has ability to rank or order phenomenon. It is defined by related categories e.g. grades of pain (mild, moderate, severe), social class (I, II, III, IV ,V). Scales of Measurements Interval scale – measurements are expressed in numbers except that the starting point is arbitrary, depending largely on the units of measurement. Meanings can be physically attached to the difference between 2 measurements on this scale, but not to their ratios, as the ratio of any 2 intervals is dependent on the units of measurement e.g. Scales of Measurements Ratio scale – has all the 3 properties of nominal, ordinal and interval scale and in addition, has a true zero point. The ratio of any 2 measurements on the scale is physically meaningful e.g. height (inc or cm zero is same), weight (Ibs or Kg, zero is same), BP (mmHg), Age Scales of Measurements From the above properties of the different scales, one can recognize that arithmetic operations of addition and multiplication are not possible on the nominal or ordinal scales; only addition (subtraction) is possible on the interval, while all operations are possible on the ratio scale. The mutually exclusive nature of the scales, not withstanding, it is sometimes possible or necessary during statistical analysis to transform data from one scale to another so as to remove inconvenient properties of the data that may invalidate statistical theories THANK YOU CONCEPT OF FREQUENCY DISTRIBUTION, MEAN, MEDIAN AND MODE OF GROUPED AND UNGROUPED DATA OMISORE AKINLOLU G. FWACP, MPH, MB.Ch.B RE-CAP OF LAST LECTURE PRESENTATION OF DATA There are various methods of data presentation. Irrespective of the methods, data are usually grouped or collated into frequencies- to determine the rate of occurrence. METHODS OF DATA PRESENTATION Tabular Presentation of Data. Graphical or diagrammatic presentation of Data - Quantitative or numerical Data- Use Histogram, Frequency Polygon. - Qualitative or categorical data- Use Bar OR Pie chart. Dot maps for geographical mapping Summary indices- e.g. Mean, Median & Mode. TABULAR PRESENTATION. Done in form of frequency tables. Can be for both quantitative and qualitative data. Definitions for Frequency Table CLASS- one of the groups into which data can be classified. CLASS FREQUENCY (CF)- is the number of observations (NOB) in the data set falling in a particular class. CLASS RELATIVE FREQUENCY- CF divided by the total NOB in the data set. Example of a Frequency Table Frequency Table CLASS FREQUENCY RELATIVE FREQUENCY Level of Number Education None 254 0.34 Primary 201 0.27 Secondary 119 0.16 Post secondary 97 0.13 Others 75 0.10 Total 746 1.00 7 Methods of summarizing data Measures of location/ central tendency- Measures of dispersion / spread/variation (Measures of partition)- some take this as measures of dispersion too. Measures of Central Tendency Describe the location of the centre of a distribution of numerical and ordinal measurements Indicates the typical experience for a group Values in the data in which other values are distributed They locate the midpoint of a distribution Measures of Central Tendency Frequently used ones are: Mean Median Mode Midrange The Mean (x-bar) Arithmetic average of the observations Most widely used average measure Most reliable and trustworthy Locates the centre of gravity of a distribution Tells where the values for a group are centred The Mean cont’d Used when numbers can be added Suitable for numeric variables- measured on interval or ratio scales Cannot be used for nominal/ordinal variable because they cannot be added. The Mean cont’d Obtained by adding up all the individual observations (summation “∑”) and dividing by the number of observations Xbar= (x1 + x2 + x3 + x4 …+ xn ) N Xbar= ∑x N ∑x= summation of observation, N= number of observations Example: Find the mean Age of the first 10 1st year clinical students in UNIOSUN 23, 19, 21, 20, 23, 21, 22, 24, 22, 22 x= 23 + 19 + 21 + 20 + 23 + 21 + 22 + 24 + 22 + 22 10 = 21.7 years PROCEDURE FOR CALCULATING MEAN (Grouped data) Find class-mid mark for each interval. Multiply class mid-mark in each interval by corresponding frequencies. The class mid- mark for each interval is the average of the class limits. Add results in (ii) across all intervals. Divide results in (iii) by number of observations or total frequency. Mean - (Grouped Data) Example: Marks of students in practical 1 Marks Frequency Class mid mark ∑ fI xI 60-64 10 62 10*62 620 65-69 14 67 14*67 938 70-74 12 72 12*72 864 75-79 20 77 20*77 1540 80-84 10 82 10*82 820 85-89 14 87 14*87 1218 - ∑f = 80 ∑ fi xi = 6000 x = ∑ fi xI ∑ fi x = 6000/80 = 75 Properties of Mean (X) Affected by extremes of values All the other observations lie about it Makes use of all information The sum of the deviations of the values from the mean is always equal zero i.e the mean is subtracted from each value to form deviations (x minus xbar) ∑ (x- Xbar) = 0 (x1 – Xbar)+ (x2 – Xbar) + (x3 – Xbar)…= 0 Advantages of the Mean It is easy to calculate Makes use of all information in the distribution- hence reliable and accurate Amenable to statistical procedures and testing Disadvantages of the mean It may be unduly influenced by abnormal values in the distribution Not used with badly skewed distribution- the more asymmetric the distribution, the less desirable it is to summarize the observation by using the mean The Median The middle number in an array of the data, when the number of observations is odd Or The arithmetic mean of the middle numbers in an array of data when the number of observations is even The Median cont’d The value above or below which half (50%) of the observations fall Bisector of histogram/ polygon For interval, ratio and ordinal scale (not nominal) EXAMPLE ON MEDIAN Find the Median of the first 10 1st year clinical students in UNIOSUN 23, 19, 21, 20, 23, 21, 22, 24, 22, 22 Step 1: Arrange in Ascending Order 19, 20,21,21,22,22,22,23,23,24 Step 2: Pick the middle observation (22+22)/2 = 22 Calculation of Median (Grouped) Sample size (n) = 80 Median position (n/2) = 40th Median class = 75-79, Lower boundary (bL) = 74.5 (for median class) Frequency in median class, f = 20 Cumulative above median class (F) = 36 Class-width ( c ) = 5 Apply formula: Median = bL + (n/2 – F)_ x c fmed CLASS BOUNDARIES& INTERVALS A class or group boundary lies midway between the data values. For example, For data in the class or group labelled: 7.1 – 7.3 (a)The class values 7. 1 and 7.3 are the lower and upper limits of the class and their difference gives the class width. (b)The class boundaries are 0.05 below the lower class limit and 0.05 above the upper class limit (because the figures are in 1Decimal place) (c)The class interval/ width is the difference between the upper and lower class boundaries. (d)Question- What are the class boundaries if the figures are between 7 and 8? 10.Median (Grouped Data) Using last example of mean. Cumulative Marks Boundaries Frequency Frequency (F) 60-64 59.5-64.5 10 10 65-69 64.5-69.5 14 24 70-74 69.5-74.5 12 36 75-79 74.5-79.5 20 56 80-84 79.5-84.5 10 66 85-89 84.5-89.5 14 80 = 74.5 + (40 – 36) x 5 = 75.5 marks 20 Advantages of the median Used with distribution of any shape especially when data are skewed Easy to calculate and understand Better representations when there are outliers Not affected by extreme values “the middle remains the middle” Disadvantages of the median Does not use all information in the distribution Only takes into account one or 2 observations Provides no information about other observations The Mode The value/observation which occurs most frequently when observations are arranged in an array Most fashionable There can be several modes- bimodal, multimodal Example on Mode In the age of 10 medical students 23, 19, 21, 20, 23, 21, 22, 24, 22, 22 The mode is 22 Formula for grouped mode Mode is the value that has the highest frequency in a data set. For grouped data, class mode (or, modal class) is the class with the highest frequency. To find mode for grouped data, use the following formula: Mode= Lb + ∆1 XC ∆1 + ∆2 Where: – * Lb - is the lower boundary of class mode. – * ∆1- is the difference between the frequency of class mode and the frequency of the class before the class mode. – * ∆2 is the difference between the frequency of class mode and the frequency of the class after the class mode. – * C is the class width 10. Mode (Grouped Data) Using last example of median. Cumulative Marks Boundaries Frequency Frequency (F) 60-64 59.5-64.5 10 10 65-69 64.5-69.5 14 24 70-74 69.5-74.5 12 36 75-79 74.5-79.5 20 56 80-84 79.5-84.5 10 66 85-89 84.5-89.5 14 80 Mode= L + ∆1 XC ∆1 + ∆2 L= Lower limit of modal class. C= Class width/Modal class Size ∆1= f1-f0 (frequency of modal class- frequency of the class preceeding modal class. ∆2= f1-f2 (frequency of modal class- frequency of the class succeeding the modal class. = 74.5 + (20-12) x5 (20-12) + (20-10) = 76.7 Advantages of the Mode Easy to compute Not affected by extreme values Main usefulness is for calling attention to distribution in which the values cluster at several places Only average available for nominal scale Disadvantages of the Mode Not the best for biological or medical statistics Several observations with the same frequency - multimodal Least valuable Mid- Range Minimum + maximum, divided by 2 Not affected by extreme of values Does not consider all information in the distribution Choice of measure of central tendency Depends on the shape/nature of the distribution- skewed to the left or right or a normal distribution Depends on the scale of measurement (ordinal or numerical) Choice of measure cont’d For continuous variation with a unimodal and symmetric distribution, the mean, median and mode will be identical and lie on the same plane When the distribution is skewed, the median may be a more informative descriptive measure to use than the mean as it is not affected by extreme values Choice of measure cont’d For statistical analyses and tests of significance, the mean is better whenever possible since it includes information from all observations and its theoretic properties provide for more powerful statistical tests Note If mean = median ….. Symmetry If mean > median ….. Skewed to the right (positive) If mean < median ….. Skewed to the left (negative) Conclusion Measures of central tendency provide good ways of summarizing data. They are in everyday statistical use. Though they are regarded as descriptive statistics, they often provide the basis for making inference about statistics. THANKS FOR YOUR ATTENTION Fundamentals of Biostatistics 8th Edition Bernard Rosner. (Chapters 1 &2). http://galaxy.ustc.edu.cn:30803/zhangwen/Bi ostatistics/Fundamentals+of+Biostatistics+8th +edition.pdf MEASURES OF CENTRAL TENDENCY (LOCATION) AND DISPERSION OMISORE AKINLOLU G. FWACP, MPH, MB.Ch.B Methods of summarizing data Measures of location/ central tendency- Measures of dispersion / spread/variation (Measures of partition)- some take this as measures of dispersion too. Measures of Central Tendency DONE IN AN EARLIER LECTURE ALREADY Measures of dispersion Statistics that describes the spread of numerical data Range Variance Interquartile range Mean Absolute Deviation Standard Deviation Range Difference between maximum (highest) and minimum (lowest) values in a data Range= Maximum – Minimum Defines the normal limits of a biological characteristic e.g systolic B.P- 100-140 Advantages of the Range Simple Easy to compute Disadvantages of the Range Does not use all available information Based on only 2 of the observations and gives no idea of how the other observations are arranged- hence not reliable. Not amenable to statistical procedures and testing Standard Deviation Most frequently used measure of deviation Quantifies the spread of the individual observations around the mean Summary of how widely dispersed the values are around the mean (average distance from the mean) Standard Deviation cont’d Uses all information in the distribution like the mean Affected by extremes of values Standard Deviation cont’d S.D = sq root ∑ (x – Xbar)2 n-1 n-1 = if sample size is < 30 but n if more x-Xbar= deviation from the mean S.D = sq root ∑ (x2) – (∑ x)2/N n- 1 Used when the mean has not been calculated Steps in computing SD 1. Calculate the mean 2. Find the difference of each observation from the mean 3. Square the differences of observation from the mean 4. Add the spread values to get the sum of squares 5. Divide this sum by the number of observations minus 1 to get mean squared deviation called Variance 6. Find the square root of this variance to get “ root-mean squared deviation” called SD Standard Deviation - Practical Example The formula is this: S = (xI - x)2 n-1 Example on standard deviations: The number of crisis experienced by 5 sickle cell patients in a year are 3, 0, 2, 1, 4 Find the mean, variance and standard deviation. Mean = xi = 3 + 0 + 2 + 1 + 4 = 2.0 n 5 Variance: (xI - x)2 = (3-2)2 + (0-2)2 + (2-2)2 + (1-2)2 + (4-2)2 n-1 5-1 = 1 + 4 + 0 + 1 + 4= 10 4 4 = 2.5 2 Standard Deviation = (xI - x) = 10 = 10 n-1 4 2 = 1.58 Note calculators are available to do this. Standard Deviation cont’d A normal distribution is a theoretical model that has been found to fit many naturally occurring phenomena The curve also called the density curve is bell shaped, symmetric. Standard Deviation cont’d In a normal distribution, it is the horizontal measurement from the mean to a point of inflection and equals the square root of the variance 68.27% of cases fall between xbar +/- s 1 S.D 95.45% of cases fall between xbar +/- 2s ………2 S.D 99.73% of cases fall between xbar +/- 3s ………3 S.D Uses of S.D Summarizes the deviation of large distribution from the mean in one figure used as a unit of variation Indicates whether the variation of difference of an individual from the mean is by chance (i.e. natural or real) Interquartile Range Difference between 25th and 75th quartile Q3 – Q1 Not sensitive to extreme values The Standard Error of Mean Measures the variability of the mean as an estimate of the true value of the mean for the population from which the sample was drawn SE (x bar) = SD/ sq root n Mean Deviation The average of the deviation from the arithmetic mean M.D.= ∑ (x-Xbar) N Variance It is the square of the SD The sum of the squares of deviations from the mean, divided by the number of degrees of freedom in the set of observations V = ∑ (x – X)2 n-1 Makes use of all information in the distribution Coefficient of Variation. - Reduces measure of dispersion to a dimensionless quantity. - Calculate by dividing standard deviation by the mean value. - Express result in percentage. - Useful to compare variations between 2 variables not in the same unit. Using measures of dispersion 1. The SD is used when the mean is used (in symmetric numeric data) 2. The interquartile range is used when The median is used i.e. with ordinal data or with skewed numeric data The mean is used but the objective is to compare individual observations with a set of norms Using measures of dispersion 3. The interquartile range is also used to describe the central 50% of a distribution, regardless of its shape 4. The range is used with numerical data when the purpose is to emphasize extreme values Measures of Partition These measures divides the data into parts. They include; ❖Quartiles ❖Percentiles ❖Deciles Quartile Divide the distribution in an array into 4 parts 1st Q1 2nd Q2 3rd Q3 4th Q1= lower quartile Q2= median Q3= upper quartile Percentile Divides a distribution in an array into a hundred equal parts 3rd, 15th, 54th, 70th 71st …….. 50th percentile = 2nd quartile = median Used in growth chart Deciles The distribution in an array into ten equal parts 10th, 20th,30th ……… Conclusion Measures of central tendency and dispersion provide good ways of summarizing data. They are in everyday statistical use. Though they are regarded as descriptive statistics, they often provide the basis for making inference about statistics. THANKS FOR YOUR ATTENTION Bello T. ( Mphil/MPH, B.EMT) Statisticsis the science of data. It involves collecting, analysing/ summarizing, interpreting and presenting data, Using them to estimate the magnitude (level) of associations and test hypothesis. Broadly divided into two - Descriptive - Inferential. Descriptive Statistics- deal with description of characteristics(s) of a finite population Inferential statistics makes deduction from a sample of a population to the population. Primary and Secondary data - Primary data: Data originally collected in the process of any statistical inquiry - Secondary data: Data collected by other individual/people/organization Primary source is preferred to secondary source. 4 Census Vital Registration systems Institutions (school health, hospitals, health centers, Veterinary Clinics). Notification centers/ Epidemiological surveillance - (infectious diseases, cancer registries etc). Surveys- National census- enumeration of the whole populace in a country, usually done every ten years. The last census in Nigeria was in 206& the popn. Was 140,003,542 North West zone- 35,786,944 most populous followed by South West - 27,581,992 Osun state has a population of 3,423,535 representing 2.45% of Nigeria’s population. Census enables us to calculate crude or total population rates. Figure 1: Population pyramid, Oriade HDSS. >=105 0.13 0.1 100-104 0.11 0.24 95-99 0.12 0.18 A 90-94 0.4 0.6 85-89 0.6 0.73 G 80-84 1.29 1.88 75-79 1.25 1.29 E 70-74 2.22 2.63 65-69 1.71 2.23 60-64 2.47 3.41 55-59 2.05 2.4 FEMALE G 50-54 3.82 4.09 MALE 45-49 3.62 3.31 R 40-44 5.26 5.24 35-39 5.33 5.68 O 30-34 6.15 6.74 25-29 6.66 7.81 U 20-24 7.31 7.98 15-19 10.82 10.12 P 10_14 12.85 10.95 05_09 14.97 12.81 0-4 10.81 9.59 20 15 10 Proportionate 5Percent population 0 5 by sex 10 15 Records of vital events- births, deaths, marriages& divorces- obtained by registration. Used for generating birth and mortality rates for whole populations or subgroups. Non existing or ineffective “Vital Registration systems” in Nigeria Thus, lack of relevant up to date health and demographic information. Data on important indicators of development e.g. IMR, U-5MR& MMR are estimated. School health records Pre-employment screening (occupational setting) Hospital based records Usually ad-hoc, but may be routine. Popular National surveys- - NDHS (National Demographic and Health Survey) - HIV Sero prevalence Sentinel study among pregnant women - NARHS (National HIV/AIDS and Reproductive Health Survey). Surveys can be conducted by individual or group of researchers, organizations, governments etc. The NDHS is a national sample survey designed to provide up-to-date information on background characteristics of the respondents; fertility levels; nuptiality; sexual activity; fertility preferences; awareness and the use of family planning methods; breastfeeding practices; nutritional status of mothers and young children; early childhood mortality and maternal mortality; maternal and child health; and awareness and behaviour regarding HIV/AIDS and other sexually transmitted infections. The target groups were women age 15-49 years& men age 15-59 years in randomly selected households across Nigeria. Information about children age 0-5 years was also collected, including weight and height. Quantitative and Qualitative Methodologies Quantitative – numbers, percent, means. Explore what? Qualitative- explore why?, how? Quantitative: Age at marriage, age, years of schooling etc. Qualitative: Reason for using condoms. 13 Structured Interview using questionnaire Service Statistics – Information routinely collected; reference can be made to existing records in the system 14 Focus Group Discussion In-depth Interview Observation - Direct - mystery client - ethnological technique 15 Address any ethical concern Prepare written guidelines for how data will be collected Pretest instruments Modify Questionnaires Train all staff involved 16 Parental Permission Informed Consent Voluntary Participation Confidentiality and Privacy 17 Detects difficult questions Verifies duration to complete questionnaire Builds competence in data collector Uncover problems in field procedures 18 Steps to organize your data for analysis. * Field editing * Coding * Data Entry and Tabulation * Data Cleaning 19 Involves systematically reviewing questionnaires for consistencies and completeness Systematic organization of data, recording date, place of interview, other identifier of the respondent 20 Process of organizing and assigning meaning to data eg. CGPA First Class 1 Second Class Upper 2 Second Class Lower 3 Third Class 4 Pass 5 21 Data will usually be entered into a computer program prior to analysis. Statistical Packages with data entry modules are: Epi-info (both DOS and Windows Versions) SPSSPC (DOS) SPSS Data Entry Builder (Windows) ISSA etc 22 Checking for and correcting errors in data entry. Some software packages have built-in- systems that check for data entry errors eg the CHECK PROGRAM in EPI-INFO 23 Missing data Inconsistent data Out of range values 24 Respondent declines to answer a question A data collector failed to ask or record a response A data entry clerk skips a question 25 A respondent may contradict himself thereby creating inconsistency in reporting 26 Impossible or implausible data items eg 30 recorded as number of years of experience for a respondent who is 25 years old 27 Two Types of Analysis: * Descriptive * Inferential Three levels of Analysis: * Univariate Level uni = single variable * Bivariate Level bi = two variables * Multivariate Level multi = 3 or more 28 The way variables are measured is very important. Measurement is the assignment of numbers to a variable Measurement determines the choice of relevant statistical method 30 Nominal (non- numerical/qualitative) Ordinal (non- numerical/qualitative) Interval (numerical/quantitative) Ratio (numerical/quantitative) Nominalscale – lowest level of measurement. Merely classifies the measure into mutually unordered categories; has no notion of numerical magnitude e.g. gender (male, female), blood group (A, B, AB, O) Classifies persons or objects into two or more categories Members of a category have at least one common characteristic. We cannot quantify or even rank-order those category. For identification purpose, nominal variables are often represented by numbers. The values of the scale have no 'numeric' meaning in the way that you usually think about numbers. 33 Variables Categories Assigned code Sex Male 1 Female 2 Residence Rural 1 Urban 2 HIV Status Positive 0 Negative 1 34 Ordinal scale – in addition to its nominal property, has ability to rank or order phenomenon. It is defined by related categories e.g. grades of pain (mild, moderate, severe), social class (I, II, III, IV ,V). Intervalscale – measurements are expressed in numbers except that the starting point is arbitrary, depending largely on the units of measurement. Meanings can be physically attached to the difference between 2 measurements on this scale, but not to their ratios, as the ratio of any 2 intervals is dependent on the units of measurement e.g. Temp on 0C, 0F, Ratioscale – has all the 3 properties of nominal, ordinal and interval scale and in addition, has a true zero point. The ratio of any 2 measurements on the scale is physically meaningful e.g. height (inc or cm zero is same), weight (Ibs or Kg, zero is same), BP (mmHg), Age (yrs or months or From the above properties of the different scales, one can recognize that arithmetic operations of addition and multiplication are not possible on the nominal or ordinal scales; only addition (subtraction) is possible on the interval, while all operations are possible on the ratio scale. The mutually exclusive nature of the scales, not withstanding, it is sometimes possible or necessary during statistical analysis to transform data from one scale to another so as to remove inconvenient properties of the data that may invalidate statistical theories Tabular Presentation of Data. Graphical or diagrammatic presentation of Data Summary indices (next lecture) Done in form of frequency tables. Can be for both quantitative and qualitative data. Definitions for Frequency Table CLASS- one of the groups into which data can be classified. CLASS FREQUENCY (CF)- is the number of observations (NOB) in the data set falling in a particular class. CLASS RELATIVE FREQUENCY- CF divided by the total NOB in the data set. CLASS FREQUENCY RELATIVE FREQUENCY Level of Education Number None 254 0.34 Primary 201 0.27 Secondary 119 0.16 Post secondary 97 0.13 Others 75 0.10 Total 746 1.00 42 A class or group boundary lies midway between the data values. For example, For data in the class or group labelled: 7.1 – 7.3 (a) The class values 7. 1 and 7.3 are the lower and upper limits of the class and their difference gives the class width. (b)The class boundaries are 0.05 below the lower class limit and 0.05 above the upper class limit (because the figures are in 1Decimal place) (c) The class interval/ width is the difference between the upper and lower class boundaries. (d)Question- What are the class boundaries if the figures are between 7 and 8? Diagrams give a very clear & understandable picture of data Comparison can be made between different samples very easily. Diagrams have impressive value also. Can also be used for numerical type of statistical analysis, e.g. to locate Mean, Mode, Median etc. Saves time and energy and is also economical. This data is easily remembered. Data can be condensed with diagrams. The subject matter must be made clear under a broad heading. The size of the scale should neither be too big nor too small. Diagrams should be absolutely neat and clean. Simplicity- diagram should convey meaning clearly& easily. Scale must be presented along with the diagram. Vertical diagram should be preferred to Horizontal diagrams. Dot Plot Stem and Leaf Display Line graphs Bar chart – simple, multiple, component. Pie chart. Histogram Frequency Polygon (Ogives). Scatter diagrams Dot Plot and Stem and leaf display to be demonstrated in class on a white board. A bar chart is made up of columns plotted on a graph. The columns are positioned over a label that represents a qualitative (categorical) variable. The height of the column indicates the size of the group defined by the column label. Weight Category of all respondents 100 89.4% 90 80 70 60 50 Weight Category 40 30 20 10 7.7% 2.9% 0 Normal Weight Overweight Obese 100 93.1 90 80 74.0 70 60 53.5 % 50 46.5 Urban Rural 40 30 26.0 20 10 6.9 0 Normal weight Overweight Obese Weight Category Figure 1: Proportion of urban & rural schoolchildren who experienced bullying in a 1-year period, Osun State, Nigeria, 2009 (Component Bar chart). 100 10.8 16.2 80 % of students 60 89.2 83.8 40 20 0 Urban Rural Bullying No bullying Source: Omisore et al., 2010 Similar to Bar chart but is in a circle. Each category is given a proportionate portion of the chart based on the angle occupied from the total 3600 of the circle. Weight Category of all respondents 7.7% 2.9% Normal Weight 89.4% Overweight Obese Like a bar chart, a histogram is made up of columns plotted on a graph. There is no space between adjacent columns. The columns are positioned over a label that represents a quantitative variable. The column label can be a single value or a range of values. The height of the column indicates the size of the group defined by the column label. HISTOGRAM WITH NORMAL DISTRIBUTION Used to show the relationship between two objects- used for quantitative variables The magnitude of the relationship is indicated by how closely the dots approximate to a straight line It can identify non-linear relationships. And also detect outliers and skewed distributions r = +1.00 r = -0.54 We live in a data world- everything we hear, see or do is often based on data (collected information). It’s vital that we learn the rudiments of data collection, organization and presentation from now. The process of learning is the process of doing- there is nothing like an hands on experience. Whatsoever you have learnt- go and put it into practice. THANK YOU Introduction to inferential statistics: Elements of probability Adekunle Fakunle Outline ❖Probability ❖Random Variables. Probability Distributions ❖Binominal and Normal distribution ❖Inferential statistics ❖Types of inferential statistic Probability ❖Probability: is the likelihood or chance of an event occurring ❖Outcomes : any possible result of probability event ❖Favorable outcomes: a successful result in a probability event e.g rolling the #1 on a die ❖Possible outcomes: All the result that could occur during probability event 3 Random Variables ▪ A random variable is a numerical description of the outcome of a statistical experiment ▪ A random variable that may assume only a finite number or an infinite sequence of values is said to be discrete; one that may assume any value in some interval on the real number line is said to be continuous Normal distribution Normal Probability Density Function Continuous random Figure: Age distribution variables are described of a pediatric population with probability density with overlying Normal function (pdfs) curves pdf Normal pdfs are recognized by their typical bell-shape Area Under the Curve pdfs should be viewed almost like a histogram Top Figure: The darker bars of the histogram correspond to ages ≤ 9 (~40% of distribution) x− 2 1 − 12 f ( x) = e Bottom Figure: shaded area 2 under the curve (AUC) corresponds to ages ≤ 9 (~40% of area) Parameters μ and σ Normal pdfs have two parameters μ - expected value (mean “mu”) σ - standard deviation (sigma) μ controls location σ controls spread 7: NORMAL PROBABILITY DISTRIBUTIONS 8 Mean and Standard Deviation of Normal Density σ μ 7: NORMAL PROBABILITY DISTRIBUTIONS 9 Standard Deviation σ Points of inflections one σ below and above μ Practice sketching Normal curves Feel inflection points (where slopes change) Label horizontal axis with σ landmarks 7: NORMAL PROBABILITY DISTRIBUTIONS 10 Symmetry in the Tails Because the Normal curve is symmetrical and the total AUC is exactly 1… … we can easily determine the AUC in 95% tails 7: NORMAL PROBABILITY DISTRIBUTIONS Assessing Departures from Normality Approximately Same distribution on Normal histogram Normal “Q-Q” Plot Normal distributions adhere to diagonal line on Quantile-Quantile plot Negative Skew Negative skew shows upward curve on Q-Q plot Positive Skew Positive skew shows downward curve on Q-Q plot Inferential Statistic Inferential Statistic Descriptive Statistics are used to organize and/or summarize the parameters associated with data collection (e.g., mean, median, mode, variance, standard deviation) Inferential Statistics are used to infer information about the relationship between multiple samples or between a sample and a population (e.g., t-test, ANOVA, Chi Square). Inferential Statistics Inferential statistics are used to draw conclusions about a population by examining the sample We want to …but we learn about can only population calculate parameter sample s… statistics Parameters and Statistics We are going to illustrate inferential concept by considering how well a given sample mean “x-bar” reflects an underling population mean µ µ x Inferential Statistic Accuracy of inference depends on representativeness of sample from population Random selection ◦ equal chance for anyone to be selected makes sample more representative Inferential Statistic Inferential statistics help researchers test hypotheses and answer research questions, and derive meaning from the results ◦ a result found to be statistically significant by testing the sample is assumed to also hold for the population from which the sample was drawn ◦ the ability to make such an inference is based on the principle of probability Inferential Statistic Researchers set the significance level for each statistical test they conduct ◦ by using probability theory as a basis for their tests, researchers can assess how likely it is that the difference they find is real and not due to chance Inferential Statistics Provide Two Environments: Test for Difference – To test whether a significant difference exists between groups Tests for relationship – To test whether a significant relationship exist between a dependent (Y) and independent (X) variable/s Relationship may also be predictive Hypothesis Testing Using Basic Statistics Univariate Statistical Analysis ◦ Tests of hypotheses involving only one variable. Bivariate Statistical Analysis ◦ Tests of hypotheses involving two variables. Multivariate Statistical Analysis ◦ Statistical analysis involving three or more variables or sets of variables. Hypothesis Testing Procedure, Cont. H0 – Null Hypothesis ◦ “There is no significant difference/relationship between groups” Ha – Alternative Hypothesis ◦ “There is a significant difference/relationship between groups” Always state your Hypothesis/es in the Null form The object of the research is to either reject or accept the Null Hypothesis/es What is a Null Hypothesis? A type of hypothesis used in statistics that proposes that no statistical significance exists in a set of given observations. The null hypothesis attempts to show that no variation exists between variables, or that a single variable is no different than zero. It is presumed to be true until statistical evidence nullifies it for an alternative hypothesis. Examples Example 1: Three unrelated groups of people choose what they believe to be the best color scheme for a given website. The null hypothesis is: There is no difference between color scheme choice and type of group Example 2: Males and Females rate their level of satisfaction to a magazine using a 1-5 scale The null hypothesis is: There is no difference between satisfaction level and gender Experimental Research: What happens? An hypothesis (educated guess) and then tested. Possible outcomes: Something Will Something Not Not Happen Will Happen It Does Not It Happens Happen Something Will Something Will Happen Happen It Does Not It Happens Happen Significance Levels and p- values Significance Level ◦ A critical probability associated with a statistical hypothesis test that indicates how likely an inference supporting a difference between an observed value and some statistical expectation is true. ◦ The acceptable level of Type I error. p-value ◦ Probability value, or the observed or computed significance level. ◦ p-values are compared to significance levels to test hypotheses Testing for Significant Difference Testing for significant difference is a type of inferential statistic One may test difference based on any type of data Determining what type of test to use is based on what type of data are to be tested. Different types of inferential statistics Chi Square A chi square (X2) statistic is used to investigate whether distributions of categorical (i.e. nominal/ordinal) variables differ from one another. General Notation for a chi square 2x2 Contingency Table Variable 1 Variable 2 Data Type 1 Data Type 2 Totals Category 1 a b a+b Category 2 c d c+d Total a+c b+d a+b+c+d 𝑎𝑑 − 𝑏𝑐 2 𝑎+𝑏+𝑐+𝑑 𝑥2 = 𝑎+𝑏 𝑐+𝑑 𝑏+𝑑 𝑎+𝑐 T test x1 − x2 t= S x1 − x2 x1 = Mean for group 1 x2 = Mean for group 2 S x1 − x2 = Pooled, or combined, standard error of difference between means The pooled estimate of the standard error is a better estimate of the standard error than one based of independent samples. Uses of the t test Assesses whether the mean of a group of scores is statistically different from the population (One sample t test) Assesses whether the means of two groups of scores are statistically different from each other (Two sample t test) Cannot be used with more than two samples (ANOVA) ANOVA In statistics, analysis of variance (ANOVA) is a collection of statistical models, and their associated procedures, in which the observed variance in a particular variable is partitioned into components attributable to different sources of variation. In its simplest form ANOVA provides a statistical test of whether or not the means of several groups are all equal, and therefore generalizes t- test to more than two groups. Doing multiple two-sample t-tests would result in an increased chance of committing a type I error. For this reason, ANOVAs are useful in comparing two, three or more means. ANOVA Hypothesis Testing Tests hypotheses that involve comparisons of two or more populations The overall ANOVA test will indicate if a difference exists between any of the groups However, the test will not specify which groups are different Therefore, the research hypothesis will state that there are no significant difference between any of the groups 𝐻0 : 𝜇1 = 𝜇2 = 𝜇3 ANOVA Assumptions Random sampling of the source population (cannot test) Independent measures within each sample, yielding uncorrelated response residuals (cannot test) Homogeneous variance across all the sampled populations (can test) ◦ Ratio of the largest to smallest variance (F-ratio) ◦ Compare F-ratio to the F-Max table ◦ If F-ratio exceeds table value, variance are not equal Response residuals do not deviate from a normal distribution (can test) ◦ Run a normal test of data by group Regression Analysis The description of the nature of the relationship between two or more variables It is concerned with the problem of describing or estimating the value of the dependent variable on the basis of one or more independent variables. Predictive Versus Explanatory Regression Analysis Prediction – to develop a model to predict future values of a response variable (Y) based on its relationships with predictor variables (X’s) Explanatory Analysis – to develop an understanding of the relationships between response variable and predictor variables Simple Regression Model 𝑦 = 𝑎 + 𝑏𝑥 𝑺𝒍𝒐𝒑𝒆 𝒃 = (𝑁Σ𝑋𝑌 − Σ𝑋 Σ𝑌 ))/(𝑁Σ𝑋2 − Σ𝑋 2) 𝑰𝒏𝒕𝒆𝒓𝒄𝒆𝒑𝒕 𝒂 = (Σ𝑌 − 𝑏 Σ𝑋 )/𝑁 Where: y = Dependent Variable Y = Second Score x = Independent Variable ΣXY = Sum of the product of 1st & 2nd scores b = Slope of Regression Line ΣX = Sum of First Scores a = Intercept point of line ΣY = Sum of Second Scores N = Number of values ΣX2 = Sum of squared First Scores X = First Score Simple regression model y Predicted Values Residuals r = Y − Yˆ Slope (b) i i i Actual Values Intercept (a) x Simple vs. Multiple Regression Simple: Y = a + bx Multiple: Y = a + b1X1 + b2 X2 + b3X3…+biXi Multiple regression model Y X1 X2 Correlation analysis ▪ Pearson correlation: It is the test statistics that measures the statistical relationship, or association, between two continuous variables. It is known as the best method of measuring the association between variables of interest because it is based on the method of covariance. Pearson's correlation is used when you want to see if their is a linear relationship between two quantitative variables. Correlation Analysis ▪ Spearman's correlation: It is the nonparametric version of the Pearson product- moment correlation. Spearman correlation is often used to evaluate relationships involving ordinal variables. For example, you might use a Spearman correlation to evaluate whether the order in which employees complete a test exercise is related to the number of months they have been employed. THANK YOU Introduction to Inferential Statistics Sampling, Probability, Normal distribution, and Hypothesis Testing by Adekunle Fakunle (PhD) Outline Definitions of terms Probability Random Variables. Probability Distributions Binominal and Normal distribution Inferential statistics Types of inferential statistic Important Definitions Probability – the chance that an uncertain event will occur (always between 0 and 1) Impossible Event – an event that has no chance of occurring (probability = 0) Certain Event – an event that is sure to occur (probability = 1). Probability Outcomes: any possible result of probability event Favorable outcomes: a successful result in a probability event e.g rolling the #1 on a die Possible outcomes: All the result that could occur during probability event 4 Random Variables A random variable is a numerical description of the outcome of a statistical experiment A random variable that may assume only a finite number or an infinite sequence of values is said to be discrete; one that may assume any value in some interval on the real number line is said to be continuous The sample Space, S The sample space, S, for a random phenomena is the set of all possible outcomes. Examples 1. Tossing a coin – outcomes S ={Head, Tail} 2. Rolling a die – outcomes S ={ , , , , , } ={1, 2, 3, 4, 5, 6} An Event , E The event, E, is any subset of the sample space, S. i.e. any set of outcomes (not necessarily all outcomes) of the random phenomena Venn S diagram E The event, E, is said to have occurred if after the outcome has been observed the outcome lies in E. S E Examples 1. Rolling a die – outcomes S ={ , , , , , } ={1, 2, 3, 4, 5, 6} E = the event that an even number is rolled = {2, 4, 6} ={ , , } Normal distribution A sample of heights of 10,000 adult males gave rise to the following histogram: Histogram showing the heights of 10000 males 1400 1200 1000 Frequency 800 600 400 200 0 140 148 156 164 172 180 188 More Height (cm) Notice that this histogram is symmetrical and bell-shaped. This is the characteristic shape of a normal distribution. If we were to draw a smooth This is called the curve through the mid-points of normal curve. the bars in the histogram of these heights, it would have the following shape: The normal distribution is an appropriate model for many common continuous distributions, for example: The masses of new-born babies; The IQs of school students; The hand span of adult females; The heights of plants growing in a field; etc. Area Under the Curve A normal distribution should be viewed almost like a histogram Top Figure: The darker bars of the histogram correspond to ages ≤ 9 (~40% of distribution) Bottom Figure: shaded area x 12 2 1 under the curve (AUC) f ( x) 2 e corresponds to ages ≤ 9 (~40% of area) Parameters μ and σ Normal distribution have two parameters μ - expected value (mean “mu”) σ - standard deviation (sigma) μ controls location σ controls spread 7: Normal Probability Distributions 15 Mean and Standard Deviation of Normal Density σ μ 7: Normal Probability Distributions 16 Standard Deviation σ Points of inflections one σ below and above μ Practice sketching Normal curves Feel inflection points (where slopes change) Label horizontal axis with σ landmarks 7: Normal Probability Distributions 17 Symmetry in the Tails Because the Normal curve is symmetrical and the total AUC is exactly 1… … we can easily determine the AUC in 95% tails 7: Normal Probability Distributions Assessing Departures from Normality Approximately Same distribution on Normal histogram Normal “Q-Q” Plot Normal distributions adhere to diagonal line on Quantile-Quantile plot Negative Skew Negative skew shows upward curve on Q-Q plot Positive Skew Positive skew shows downward curve on Q-Q plot Inferential Statistic What is inferential statistics? Inferential statistics is a technique used to draw conclusions about a population by testing the data taken from the sample of that population It is the process of how generalization from sample to population can be made. It is assumed that the characteristics of a sample is similar to the population’s characteristics. It includes testing hypothesis and deriving estimates Inferential Statistic Descriptive Statistics are used to organize and/or summarize the parameters associated with data collection (e.g., mean, median, mode, variance, standard deviation) Inferential Statistics are used to infer information about the relationship between multiple samples or between a sample and a population (e.g., t- test, ANOVA, Chi Square). The process of inferential analysis It comprises of all the data collected from the sample. Depending on the sample size, this data can be large or small set of Raw Data measurements. It summarizes the raw data gathered from the sample of population Sample These are the descriptive statistics (e.g. measures of central tendency) Statistics These statistics then generate conclusions about the population based Inferential on the sample statistics. Statistics Inferential Statistics Inferential statistics are used to draw conclusions about a population by examining the sample We want to …but we learn about can only population calculate parameter sample s… statistics Parameters and Statistics We are going to illustrate inferential concept by considering how well a given sample mean “x-bar” reflects an underling population mean µ µ x Inferential Statistic Accuracy of inference depends on representativeness of sample from population Random selection equal chance for anyone to be selected makes sample more representative Inferential Statistic Inferential statistics help researchers test hypotheses and answer research questions, and derive meaning from the results a result found to be statistically significant by testing the sample is assumed to also hold for the population from which the sample was drawn the ability to make such an inference is based on the principle of probability Inferential Statistic Researchers set the significance level for each statistical test they conduct by using probability theory as a basis for their tests, researchers can assess how likely it is that the difference they find is real and not due to chance Inferential Statistics Provide Two Environments: Test for Difference – To test whether a significant difference exists between groups Tests for relationship – To test whether a significant relationship exist between a dependent (Y) and independent (X) variable/s Relationship may also be predictive Hypothesis Testing Using Basic Statistics Univariate Statistical Analysis Tests of hypotheses involving only one variable Bivariate Statistical Analysis Tests of hypotheses involving two variables Multivariate Statistical Analysis Statistical analysis involving three or more variables or sets of variables. Hypothesis Testing Procedure H0 – Null Hypothesis “There is no significant difference/relationship between groups” Ha – Alternative Hypothesis “There is a significant difference/relationship between groups” Always state your Hypothesis/es in the Null form The object of the research is to either reject or accept the Null Hypothesis/es Examples Example 1: Three unrelated groups of people choose what they believe to be the best color scheme for a given website. The null hypothesis is: There is no difference between color scheme choice and type of group Example 2: Males and Females rate their level of satisfaction to a magazine using a 1-5 scale The null hypothesis is: There is no difference between satisfaction level and gender We can make two types of errors in hypothesis testing: In the population, Not reject Ho Reject Ho Ho actually is: True Correct decision made Type 1 error Researcher thinks there is an actual relationship between the variables when there is not False Type II error Correct decision made There is an actual relationship between variables although researcher has accepted null hypothesis Concepts related to Sampling Error Sampling Error: The degree to which a sample differs on a key variable from the population. Confidence Level: The number of times out of 100 that the true value will fall within the confidence interval. Confidence Interval: A calculated range for the true value, based on the relative sizes of the sample and the population. Why is Confidence Level Important? Confidence levels, which indicate the level of error we are willing to accept, are based on the concept of the normal curve and probabilities. Generally, we set this level of confidence at either 90%, 95% or 99%. At a 95% confidence level, 95 times out of 100 the true value will fall within the confidence interval. We can theoretically draw numerous samples from a population that examine the value of one variable. The more samples we draw from the population, the more likely it is that the frequency distribution of that variable will resemble a normal distribution Important concepts about sampling distributions: If a sample is representative of the population, the mean (on a variable of interest) for the sample and the population should be the same. However, there will be some variation in the value of sample means due to random or sampling error. This refers to things you can’t necessarily control in a study or when you collect a sample. The amount of variation that exists among sample means from a population is called the standard error of the mean. Standard error decreases as sample size increases. Significance Levels and p-values Significance Level A critical probability associated with a statistical hypothesis test that indicates how likely an inference supporting a difference between an observed value and some statistical expectation is true. The acceptable level of Type I error. p-value Probability value, or the observed or computed significance level. p-values are compared to significance levels to test hypotheses Testing for Significant Difference Testing for significant difference is a type of inferential statistic One may test difference based on any type of data Determining what type of test to use is based on what type of data are to be tested. Example: Types of Relationships Positive Negative No Relationship Income Education Income Education Income Education ($) (yrs) ($) (yrs) ($) (yrs) 20,000 10 20,000 18 20,000 14 30,000 12 30,000 16 30,000 18 40,000 14 40,000 14 40,000 10 50,000 16 50,000 12 50,000 12 75,000 18 75,000 10 75,000 16 Income and Education 80000 Income 60000 Income 40000 20000 Education 0 1 2 3 4 5 Education In inferential statistics, the hypothesis that is actually tested in the null hypothesis. Therefore, what we must do is disprove that a relationship between the variables does not exist. Different types of inferential statistics Chi Square A chi square (X2) statistic is used to investigate whether distributions of categorical (i.e. nominal/ordinal) variables differ from one another. General Notation for a chi square 2x2 Contingency Table Variable 1 Variable 2 Data Type 1 Data Type 2 Totals Category 1 a b a+b Category 2 c d c+d Total a+c b+d a+b+c+d 𝑎𝑑 − 𝑏𝑐 2 𝑎+𝑏+𝑐+𝑑 𝑥2 = 𝑎+𝑏 𝑐+𝑑 𝑏+𝑑 𝑎+𝑐 T test x1 x2 t S x1 x2 x1 Mean for group 1 x2 Mean for group 2 S x1 x2 Pooled, or combined, standard error of difference between means The pooled estimate of the standard error is a better estimate of the standard error than one based of independent samples. Uses of the t test Assesses whether the mean of a group of scores is statistically different from the population (One sample t test) Assesses whether the means of two groups of scores are statistically different from each other (Two sample t test) Cannot be used with more than two samples (ANOVA) ANOVA In statistics, analysis of variance (ANOVA) is a collection of statistical models, and their associated procedures, in which the observed variance in a particular variable is partitioned into components attributable to different sources of variation. In its simplest form ANOVA provides a statistical test of whether or not the means of several groups are all equal, and therefore generalizes t-test to more than two groups. Doing multiple two-sample t-tests would result in an increased chance of committing a type I error. For this reason, ANOVAs are useful in comparing two, three or more means. ANOVA Hypothesis Testing Tests hypotheses that involve comparisons of two or more populations The overall ANOVA test will indicate if a difference exists between any of the groups However, the test will not specify which groups are different Therefore, the research hypothesis will state that there are no significant difference between any of the groups 𝐻0 : 𝜇1 = 𝜇2 = 𝜇3 Regression Analysis The description of the nature of the relationship between two or more variables It is concerned with the problem of describing or estimating the value of the dependent variable on the basis of one or more independent variables. Predictive Versus Explanatory Regression Analysis Prediction – to develop a model to predict future values of a response variable (Y) based on its relationships with predictor variables (X’s) Explanatory Analysis – to develop an understanding of the relationships between response variable and predictor variables Correlation Analysis Spearman's correlation: It is the nonparametric version of the Pearson product- moment correlation. Spearman correlation is often used to evaluate relationships involving ordinal variables. For example, you might use a Spearman correlation to evaluate whether the order in which employees complete a test exercise is related to the number of months they have been employed. Correlation analysis Pearson correlation: It is the test statistics that measures the statistical relationship, or association, between two continuous variables. It is known as the best method of measuring the association between variables of interest because it is based on the method of covariance. Pearson's correlation is used when you want to see if their is a linear relationship between two quantitative variables. Simple Regression Model 𝑦 = 𝑎 + 𝑏𝑥 𝑺𝒍𝒐𝒑𝒆 𝒃 = (𝑁Σ𝑋𝑌 − Σ𝑋 Σ𝑌 ))/(𝑁Σ𝑋2 − Σ𝑋 2) 𝑰𝒏𝒕𝒆𝒓𝒄𝒆𝒑𝒕 𝒂 = (Σ𝑌 − 𝑏 Σ𝑋 )/𝑁 Where: y = Dependent Variable Y = Second Score x = Independent Variable ΣXY = Sum of the product of 1st & 2nd scores b = Slope of Regression Line ΣX = Sum of First Scores a = Intercept point of line ΣY = Sum of Second Scores N = Number of values ΣX2 = Sum of squared First Scores X = First Score Simple regression model y Predicted Values Residuals r Y Yˆ Slope (b) i i i Actual Values Intercept (a) x Practical demonstration THANK YOU EPIDEMIOLOGIC STUDY DESIGNS- OBSERVATIONAL Epidemiological studies Epidemiological study designs Observational- Experimental- Descriptive RCTs (individual& Analytic community) Clinical trials ANOTHER CLASSIFICATION? Case series Individuals Cross-sectional Descriptive Populations Correlational Epidemiological studies Case control Observational Prospective Cohort Analytical Retrospective Intervention Clinical trials Descriptive Studies Describe the general characteristics of the distribution of a disease – Person – Place – Time Correlational Studies Case Reports and Case Series Cross-Sectional Surveys So why proceed to an analytical study? Often when we need to answer the following type of questions: What is the source of infection for an outbreak of diarrhoeal disease? What are the risk factors for neonatal tetanus? What factors are associated with increased mortality for persons with measles? Does smoking cause lung cancer? Analytical epidemiology Analytic epidemiology Attempts to provide the why? and how? of health related events. Observational studies – Case control studies – Cohort studies Experimental studies Case control studies Aetiologic studies in which comparison are made between individuals who have a disease, cases and individuals who do not, controls Case-Control Study Exposure Disease ? (Case) ? No disease (Control) Retrospective Nature Major Steps in case-control study Define and select cases Select controls Ascertain exposures Compare exposure in cases and controls –proportions/odds ratios.... Test any differences for statistical significance What (Who) is a control ? A “case” AMAP except that they do not have the disease (outcome). Must have same opportunity for exposure as a case Must be subject to same inclusion& exclusion criteria. No one control group is optimal for all situations Scientific, economic and practical considerations Principles of Control Selection From same study base (target population) as cases Selected independently of exposure status!! If they had developed illness, they would have been a case Comparable information to cases General Population Controls Advantages – If all cases in general population known – direct calculation of risk Disadvantages – Cost – Sampling frame Neighborhood Controls Advantages: – Inexpensive,efficient – Matched for potentially confounding variables Disadvantages – Exposure related to neighborhood – Potential bias Hospital Controls Advantages: – Convenient – Come from same catchment area Disadvantages: – Control disease may be linked to exposure – Hospitalized controls differ from general population Friend Controls Advantages – Convenient Disadvantages – Bias – Friends may share same exposure Analysis in Case Control Studies ▪ No calculation of rates ▪ Proportion of exposure Distribution of cases and controls according to exposure in a case control study Cases Controls Exposed a b Not exposed c d Total a+c b+d % exposed a/(a+c) b/(b+d) Odd’s exposed a/c b/d Distribution of cases and controls according to consumption of bottled water in a case-control study Cases Controls 20 5 Bottled water No bottled water 5 20 Total 25 25 % exposed 20/25=80% 5/25=20% Odds exposed a/c=4 b/d=0.25 Intuitively…. If the frequency of exposure is higher among the cases than the controls, then the incidence will probably be higher among the exposed than the non-exposed. Distribution of cases and controls according to exposure in a case- control study Cases Controls Exposed a b c d Not exposed a+c b+d Total Odds Exp = a/c Odds Exp = b/d Cases Controls a/c axd Odds ratio (OR) = a/(a+c) OR b/d bxc Distribution of cases and controls according to bottled water consumption in a case-control study Cases Controls Exposed 20 5 Not exposed 5 20 25 25 Total Odds Exp = Odds Exp = Cases 20/5 Controls 5/20 20/5 20 x 20 a/(a+c) = = 16 Odds ratio (OR) = 5x5 5/20 Strengths of Case Control Studies Rare diseases Multiple exposures Rapid, no latency period Small sample size Low cost No ethical problems Limitations of Case-control Studies No calculation of rates and risks Selection of controls difficult Not suitable for rare exposures Problems with recall Classical examples of case control studies Cigarette Smoking & lung cancer Drugs (Thalidomide) & congenital malformation Maternal smoking & congenital malformation Radiation & leukemia Oral Contraceptives & Hepatocellular cancer Cohort study Definition of cohort A cohort was a 300- 600 man unit in the Roman army A group of people marching through time from an exposure to one or more outcomes The studies follow up two or more groups from exposure to outcome The goal is usually to measure incidence Cohort identification A cohort can be defined by – Population (single cohort with internal comparison group) – Exposure status (double cohort which consists of exposed and unexposed groups) A cohort can be fixed or dynamic – Fixed is defined by an event that has already occurred and no new members can be added – In dynamic members can come in and out Cohort identification Cohorts can be identified through – Geographical area- e.g. Framingham study, – Distinct and measurable exposures e.g. studies of survivors of the Japanese atomic bombs, Gay men – Ease of follow up – e.g. British physicians study, Nurses health study, Classification Prospective (concurrent) – Cohort is assembled, baseline exams and data collected for purpose of study Retrospective (historical) – Cohort is assembled in the past on the basis of records. Some data might be missing Bidirectional (mixed) – Includes both elements Prospective cohort study Investigator begins study here Retrospective cohort studies Information needs to be available on Investigator this population begins study here Steps in conducting cohort studies Identify a cohort Determine exposure status at baseline Follow up over time Ascertain whether outcomes have occurred at intervals or at the end Analyze data Analysis in cohort The unit of study is the individual Goal is to calculate incidence – Cumulative incidence provided there is no loss to follow up (censoring) or competing risks – Incidence density – makes use of person time at risk contributed by each individual Once incidence (I) is calculated for exposed and non- exposed Relative Risk (RR) can then be calculated RR = I exposed/ I un-exposed One RR is obtained one can also get Attributable risk (AR), PAR, etc Distribution of illness according to exposure in a cohort study ILL NOT ILL Risk b a a a+b Exposed a+b Not exposed c d c+d c c+d Relative risk = Risk exposed / Risk not exposed Presentation of cohort data: 2x2 table ill not ill ate ham 49 49 98 did not eat 4 6 10 ham Classical example: foodborne outbreak (cohort = all people who attended a wedding for example) ill not ill / Total Incidence ate ham 49 49 98 50 % did not 4 6 10 40 % eat ham Relative risk 50% / 40% = 1.25 Strengths Allows for the study of rare exposures Allows for the measure of incidence Allows for the study of multiple outcomes of a single exposure Allows for clearer description of the exposure- disease time relationship Less prone to selection bias Less bias from outcome ascertainment if done prospectively Limitations Prone to loss to follow up ( migration, refusal to participate, withdrawals and deaths from competing risks) If prospective costly and time consuming Inefficient for rare diseases Differential bias over time (exposures can change over time) Exposure can change over time Multiple exposures = difficult Classical Examples of cohort study 1951 study of smoking & lung cancer (Doll & Hill) Framingham heart study (1948) OSUN STATE UNIVERSITY LEARNING MANAGEMENT SYSTEM (VIRTUAL CLASS) https://lms.uniosun.edu.ng January, 2021 Demography 2 BY E. O. ASEKUN-OLARINMOYE, B.Sc. (Hons.) Biology, MD, FWACP Course code: COM 201 Course Title: Introduction to Demog raphy and Biostatistics Course Unit: 2 units Outline Trends in general population growth Demographic features of the Nigeria’s populatio n Overview of the Nigerian population policies Population indices Sources of population data Population dynamics and health implications Population structure and population movements – demographic cycle/transition Definition Overpopulation is a condition in which the density of the population expands to a limit which lead to a deterioration of the environment, a decrease in the quality of life or collapse of the population. 1. The world population grew very slowly up until about 1900. 2. The population then exploded and increased rapidly and still continues today. 3. 1900 - the world population were 1.7 billion. 4. By 1950 - it had reached 2.5 billion. More than a 50 % increase in the last 50 years. 5. Between 1950 and 2000 the population grew to 6.2 billion about 1900. 6. The population then exploded and increased rapidly and still continues today. World Population 4. By late 2011, it had reached 7 billion. 5. The near future Global population shows no sign of slowing down. 6. The world population has continued to grow because the birth rate has remained higher than the death rate Patterns of population growth 1. Rates of population growth vary across the world. 2. Although the world's total population is rising rapidly, not all countries are experiencing this growth. 3. In the UK, for example, population growth is slowing, while in Germany the population has started to decline. Patterns of population growth 5 In Bulgaria, the birth rate is 9/1,000 and death rate is 14/1,000. Bulgaria has a declining population. 6 In South Africa, the birth rate is 25/1,000 and death rate is 15/1,000. South Africa has an increasing population with a population growth rate of 1 % Nigeria is one of the most densely populated countrie s in Africa, with approximately 200 million people in a n area of 920,000 km2 (360,000 sq mi) Has largest population in Africa Seventh (7th) largest population in the world. Rate of urbanization being estimated at 4.3%. Has over 250 ethnic groups, with over 500 languages, and gives the country great cultural diversity. Most of the population is a young population, with 42.54% between the ages of 0–14. high dependency ratio of the country at 88.2 depend ants per 100 non-dependants. Nigeria’s Population Structure, 2018 Population projections The UN estimates that Nigeria’s population will reach 391million by 20 50 which will make it the 4th most populous country in the world and in t he year 2100, 545 million. Population:174,507,539 (July 2013 est.) 178.5 million (2014 est.) Nigeria’s Population Structure, 2018 Total Population - 203,452,505 Age structure 0-14 years: 42.45% (male 44,087,799 /female 42,278,742) 15-24 years: 19.81% (male 20,452,045 /female 19,861,371) 25-54 years: 30.44% (male 31,031,253 /female 30,893,168) 55-64 years: 4.04% (male 4,017,658 /female 4,197,739) 65 years and over: 3.26% (male 3,138,206 /female 3,494,524) (2018 est. Birth rate - 35.2 births/1,000 population Death rate - 9.6 deaths/1,000 population Total fertility rate - 4.85 children born/w oman Population growth rate - 2.54% Contraceptive prevalence rate - 13.4% Total Dependency ratio - 88.2 Urbanization - 50.3% of total population Life expectancy at birth total population: 59.3 years (2018 est.)male: 5 7.5 years (2018 est.)female: 61.1 years (2018 e st.) HIV/AIDS adult prevalence rate 2.8% (2017 est.) Literacy definition: age 15 and over can read and write total population: 59.6%male: 69.2%female: 49.7% (2015 est.)Total population: 78.6%Male: 8 4.35%Female: 72.65% (2010 est.) Major cities - population Lagos 11.223 million; Kano 3.375 million; Ibadan 2.9 49 million; ABUJA (capital) 2.153 million; Port Harco urt 1.894 million; Kaduna 1.524 million (2011) Infant mortality rate total: 74.09 deaths/1,000 live births Total fertility rate 5.25 children born/woman (201 4 est.) Contraceptive prevalence rate 14.1% (2011) Maternal mortality rate 630 deaths/100,000 live bir ths (2010) Health expenditure 5.3% of GDP (2011) Reasons for population growth: The creation of modern economics