Chapter 2 Digital Transformation and AI PDF
Document Details
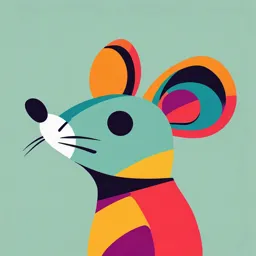
Uploaded by IssueFreeGenius4432
Erandi Thennakoon
Tags
Related
- Nomination-LesBigBoss-livre-blanc-les-clés-du-modern-selling PDF
- BSIT41A Module 1: Digital Transformation PDF
- Demystifying AI: What Digital Transformation Leaders Can Teach You About Realistic Artificial Intelligence (PDF)
- Demystifying AI: What Digital Transformation Leaders Can Teach You About Realistic Artificial Intelligence (2019)
- MCDT Week 5: Drivers of Digital Transformation PDF
- Digitalization PDF
Summary
This document presents a detailed overview of digital transformation and artificial intelligence. It covers business concepts, techniques, and examples to illustrate the core ideas and practical applications of these technologies in managing information and improving business processes.
Full Transcript
PART B MANAGING INFORMATION IN BUSINESS AND DIGITAL TRANSFORMATION Erandi Thennakoon Digital CHAPTER 02 Transformation DIGITAL Management TRANSFORMATI Learning Outcomes Explain the concept of digital ON AND transforma...
PART B MANAGING INFORMATION IN BUSINESS AND DIGITAL TRANSFORMATION Erandi Thennakoon Digital CHAPTER 02 Transformation DIGITAL Management TRANSFORMATI Learning Outcomes Explain the concept of digital ON AND transformation in the context of a digital era Demonstrate the understanding ARTIFICIAL of business process management lifecycle and their impacts to a business INTELLIGENCE Describe how a firm engages in business model transformation Digital Transformation Management Digital Business Use of digital technologies as a central part of its operations and strategy to transforms traditional business processes, models, and operations it involves in leveraging digital technologies such as the internet, mobile devices, cloud computing, big data analytics, artificial intelligence, and social media, to create new opportunities, improve efficiency, enhance customer experiences, and drive innovation. Example: TrendyThreads is an online clothing store. It operates primarily through its website and mobile app, allowing customers to browse, select, and purchase clothing items directly online. This is a core aspect of being a digital business, as its primary interface with customers is through digital platforms E-commerce Website: customers can view product catalogs, read reviews, and make purchases. Digital Payments: It offers various digital payment options, such as credit cards, digital wallets (e.g., PayPal), and even cryptocurrency. Customer Data Analytics: Can use data analytics tools to track customer behavior, preferences, and purchase history. This helps in personalizing recommendations and targeted marketing. Social Media Marketing through social media channels like Instagram, Facebook, Tiktok Automated Inventory Management: It uses software to track inventory levels, manage orders, and automate reordering processes. Customer Support: provides digital customer support through chatbots on its website and social media platforms which allows for quick responses to customer queries. Personalization: It uses data to offer personalized recommendations based on past purchases and browsing behavior. Order Fulfillment: Orders placed online are processed through a digital system that coordinates with the warehouse for packaging and shipping. Delivery Tracking: Customers receive digital tracking information for their orders, allowing them to Digital Transformation Important for organizations seeking to leverage digital technologies to drive innovation, improve efficiency, and stay competitive in today's digital age. This can be described as the process of adopting digital technologies and strategies to fundamentally change how businesses operate, deliver value to its customers, develop potential into drive significant business growth, unlock new revenue streams and create a competitive advantage in today's digital economy. This is a broader and deeper change where digital technologies are used to fundamentally alter how a business operates and delivers value to customers. Example: A retail company moving from just having a physical store to creating an integrated online and offline shopping experience. This includes developing an e- commerce platform, using data analytics to personalize offers, and incorporating automated inventory management systems. Digitization and digitalization Digitization and digitalization are two related but distinct concepts in the realm of digital transformation Digitization Converts analog information or physical assets into a digital format. Example: Scanning paper documents and converting them into electronic files is a form of digitization. Digital versions of analog information are easier to store, access, and manipulation. Digitalization Covers strategic, tactical and operational levels of an organization. It involves transformation of business processes, models, and operations using digital technologies. Leverages digital tools & technologies (example: automation, data analytics, artificial intelligence, and cloud computing), and data to create new business models, improve efficiency, enhance customer experiences, and drive innovation. Example: Implementing a digital system for managing customer relationships (CRM software) that replaces a manual paper-based system. This system helps track interactions with customers and analyze data more effectively. Digital maturity The level of an organization's capability and readiness to effectively leverage digital technologies and strategies to drive business success It reflects the organization's ability to integrate digital technologies into its operations culture, and overall strategy, and to derive tangible benefits from those digital investments Example: Low Digital Maturity: The company only has a basic website with product listings but does not engage in online sales or use digital tools for operations. Their marketing is mostly done through traditional methods like flyers and in-store promotions. Moderate Digital Maturity: The company has an e-commerce platform where customers can purchase products online, and they use social media for marketing and customer engagement. They also use basic analytics to track online sales and customer behavior. High Digital Maturity: The company seamlessly integrates its e-commerce platform with advanced data analytics and customer relationship management (CRM) systems. They use artificial intelligence to personalize marketing campaigns, optimize inventory based on predictive analytics, and automate various business processes. Their digital tools are deeply embedded into their business strategy, allowing for real-time decision-making and a highly personalized customer experience. Digital maturity model developed by Deloitte (www2.deloitte.com), has 5 core business dimensions and a set of sub-dimensions as shown in Figure 2.01. Process of Digital Transformation The DT process involves a series of steps to guide organizations through the adoption of digital technologies and the integration of digital strategies. BUSINESS PROCESS MANAGEMENT The discipline of managing and improving an organization's business processes to enhance efficiency, productivity, customer satisfaction, and overall performance. BPM involves the systematic design, execution, monitoring and optimization of processes to achieve operational excellence and drive business success (Dumas et al, 2008). There are a number of software tools to perform BPM and process automation (example: http://bizagi.com) Business Process Lifecycle Process Discovery Identify and document the current business processes within the organization. This involves mapping out the activities, tasks, roles, and interactions involved in each process. Process discovery helps gain a comprehensive understanding of existing processes and their interdependencies Process Analysis Analyze the documented processes to identify areas for improvement. Involves evaluating process performance metrics, such as cycle time, throughput, error rates, or customer satisfaction. Identify pain points, redundancies, or areas of low efficiency that can be targeted for improvement. Process Redesign Based on the analysis, redesign the processes to optimize performance and address identified issues. This may involve simplifying steps, eliminating non-value-added activities, automating manual tasks, or reorganizing process flows. The goal is to streamline processes, reduce costs, enhance quality, and improve customer experience. Process Automation Implement automation technologies to streamline and accelerate process execution. This can include workflow automation, robotic process automation (RPA), or integration with enterprise software systems. Automation reduces manual effort, eliminates errors, and improves process efficiency. Low-code No-code Developments of Robotic Process Automation No-Code RPA platforms allow users to automate tasks without any coding skills. They use a drag-and-drop interface and pre-built components, making it easy to set up automated workflows quickly and efficiently. These platforms prioritize simplicity, enabling users to handle repetitive tasks with minimal effort. Low-Code RPA platforms blend visual, drag-and-drop interfaces with the option to add custom code or scripts. They allow users to create automation workflows with minimal coding but offer flexibility for those with coding skills to enhance functionality and integrate with other systems. Commercially Available Process Automation Platforms. Process Execution and Monitoring Establish clear roles, responsibilities, and guidelines for process execution. Monitor process performance in real-time, capturing data and metrics to assess adherence to predefined performance standards. Implement dashboards or reporting mechanisms to provide visibility into process performance. Continuous Improvement Foster a culture of continuous improvement by establishing feedback loops and mechanisms for capturing insights and lessons learned Encourage employees to identify opportunities for process optimization and innovation. Regularly review and refine processes based on feedback and changing business needs Process Modeling and Design Create process models that visually represent the sequence of activities, decision points, and flow of information within a process. Process modeling techniques such as flowcharts, swimlane diagrams, or BPMN (Business Process Model and Notation) diagrams can be used. This step allows for a clear visualization of the process and helps identify inefficiencies or bottlenecks. Illustration 01 Here are the steps of an order-to-cash process: 1.Customer sends a purchase order. 2.Worker checks inventory. 3.Order is confirmed or rejected based on stock. 4.If confirmed, an invoice is sent, and goods are shipped. 5.Order is archived. Swimlanes In BPMN 2.0 (Business Process Model and Notation), swimlanes are a visual element used to organize and clarify who or what is responsible for different parts of a process. They help in defining roles and responsibilities and show how different entities or departments interact within the process. BPMN 2.0 uses a 'pool to show the resource class (example: company) and a 'lane' to show the resource sub-class (example finance department) for the above purpose. Business Model Transformation Business model transformation is about the fundamental changes made to an organization's existing business model to create new value propositions, revenue streams, and competitive advantages over its rivals. Domain Transformation, Cultural & Organizational Transformation Domain transformation in digital transformation reshapes an organization's operations and business models to capitalize on digital opportunities. A key aspect is cultural and organizational change, emphasizing agile practices, digital skills, effective communication, customer-centricity, and collaboration with external partners. Example: Travel and Hospitality Traditional Approach: Booking travel involved travel agents, physical brochures, and manual reservation systems. Transformed Approach: Digital transformation has introduced online booking platforms, mobile apps, virtual reality for destination previews, and AI-driven customer service. This has streamlined booking processes, enhanced personalization, and created new customer engagement opportunities. CHAPTER 02 Big Data and Artificial Intelligence Learning Outcomes DIGITAL Explain the concept of Industry 4.0 TRANSFORMATION and its impact on the business world AND ARTIFICIAL Describe the concept of Big Data Explain Al, Data Sciences and INTELLIGENCE demonstrate the understanding of supervised machine learning process INDUSTRY 4.0 The Fourth Industrial Revolution (Industry 4.0) refers to the ongoing transformation of traditional manufacturing and industrial processes via Digital technologies and automation. This will shift the existing systems to smart and inter-connected systems. https://www.youtube.com/watch?v=I MmnSZ7U1qM Smart Manufacturing The main objective of smart manufacturing is to effectively and efficiently use the advanced information and manufacturing technologies to provide greater flexibility in physical processes to cater complex consumer demands. Smart manufacturing use following technologies: Industry 4.0 Cyber physical systems Internet of Things (loT) Digital manufacturing and Additive manufacturing Advanced robotics and simulation Cloud computing and Big Data Augmented Reality https://www.youtube.com/watch?v=jQbXZ2_nVok Big Data "High-volume, high-velocity and/or high-variety information assets that demand cost- effective, innovative forms of information processing that enable enhanced insight, decision making, and process automation.“ (Gartner, 2012) https://www.youtube.com/watch ?v=eVSfJhssXUA Big Data and Performance Management The amount of data available to organizations is increasing ever more rapidly, and big data with its characteristics of volume, velocity, veracity, variety and value highlights both the opportunities and the challenges which new sources of data present to organizations The ability to analyze large, and unstructured, data sets and to uncover previously hidden patterns of information could be an important element of an organization's competitive advantage. Organizations need to have the capacity to store and-more importantly to analyze these data sets. Organizations today have more transactional data than they have ever had before about their customers, their suppliers and their operations. What is Big Data? Datasets whose size is beyond the ability of typical database software to capture, store, manage and analyse - McKinsey Global Institute (2011) Development of digital technologies has led to the generation and storage of large volumes of data (1 petabytes = 1015 B = I million gigabytes). Big Data refers to a large volume Structured Data: Data that is organized in a fixed format, like databases or spreadsheets. Example: Sales records in a company's database showing transactions, dates, and product details. Semi-Structured Data: Data that doesn't fit neatly into a table but has some organizational properties, like tags or markers. Example: Emails with subject lines, timestamps, and body text or XML files with nested data. Unstructured Data: Data that has no predefined format and is often text-heavy or multimedia. Example: Social media posts, customer reviews, or video footage. Big data is generated as a result of transactions, interactions and observations. Transactions: Online purchases or banking transactions. Interactions: Customer service chat logs or website clickstreams. Observations: Sensor data from smart devices or environmental monitoring. Specialized technologies and frameworks to handle big data: Apache Hadoop, Apache Spark, NoQL databases and distributed & parallel computing BIG DATA ATTRIBUTES 3V Volume It refers to the massive amount of data, ranging from terabytes to exabytes, generated and collected by various sources and stored Example : A social media platform like Facebook generates vast amounts of data daily from user posts, comments, likes, and images. This data can reach petabytes (1 petabyte = 1 million gigabytes) in size. Velocity Describes the speed at which data is created, processed, and analyzed. Big Data is generated, collected, and processed at high speeds and in real- time. Stock trading platforms handle and analyze market data in real-time. The data stream includes stock prices, trading volumes, and market news that needs to be processed quickly to make immediate trading decisions.. The ability to process this data quickly enables businesses to gain a competitive advantage, such as offering personalized recommendations based on customer interactions. Variety This attribute refers to the diverse formats and types of Big Data It includes structured (relational databases and spreadsheets), semi-structured (JSON and XML), and unstructured data (like text, images, and videos). Example: E-commerce websites collect various types of data, including structured data (like product prices and customer names), semi-structured data (like XML files or emails), and unstructured data (like customer reviews and social media posts). Artificial Intelligence Artificial Intelligence (Al) refers to the simulation of human intelligence in machines that are programmed to think and learn like humans. It is a multidisciplinary field of computer science that encompasses various subfields such as machine learning, natural language processing, computer vision, robotics, and more Data science is a broader and interdisciplinary field that has roots to statistics, mathematics, computer science, application domain expertise and other related disciplines. Data analytics is a sub-field of data science that focuses on analyzing data using statistical and computational techniques to unveil patterns, trends and meaningful information to understand historical data to answer some specific issues and solve business problems. Al involves developing algorithms and intelligent agents & systems that can perceive, reason, learn, and make decisions based on data. An intelligent agent is a system or entity that perceives its environment, processes information, and takes actions to achieve specific goals or objectives, exhibits intelligent behavior and can make decisions autonomously. Ai sub-feilds 1. Machine Learning (ML): ML algorithms enable systems to learn from data without being explicitly programmed, allowing them to make predictions, recommendations, and decisions. Example: Email Spam Filtering. 2. Deep Learning (DL): A subset of ML, DL employs artificial neural networks with multiple layers to process complex data and extract high-level representations. Example: Image Classification. 3. Natural Language Processing (NLP): NLP focuses on enabling computers to understand, interpret, and generate human language, facilitating tasks such as text analysis and chatbots. Example: Chatbots. 4. Computer Vision: Computer Vision enables machines to interpret visual information from images or videos, enabling tasks such as image recognition and object detection. Example: Facial Recognition. 5. Robotics: Robotics involves creating intelligent machines capable of physically interacting with the environment. Example: Industrial Robots AI Technology Across a wide Range of Areas and Disciplines Healthcare Finance Retail Transportation Education Manufacturing Entertainment Agriculture Telecommunication security DATA AND ALORITHM Datum, Data, Information and Knowledge Datum Refers to a single measurement or value within a dataset. It represents a single data point. Datum is the singular form of the word 'data’. Data Data is raw facts, observations, or measurements that are typically collected or recorded. Data can be in various forms, such as numbers, texts, images, audio, or video. It is often unorganized and lacks context or meaning on its own. Data can be structured, where it follows a specific format, or unstructured, where it doesn't adhere to a predefined structure. Example: list of numbers representing daily sales figures, a collection of customer names and addresses, or a set of survey responses Data is broadly categorized into qualitative and quantitative. Knowledge and Information Information Data that has been processed, organized, or structured in a meaningful way to convey knowledge, facts, or instructions. It is the result of interpreting raw data and giving it context or relevance within a particular framework. Can be communicated through various mediums such as text, images, audio, or visual representations. Information is data that has been transformed into a format that is understandable and useful to humans for decision-making, problem-solving, or gaining understanding about a particular subject or situation. Algorithm An algorithm is a step-by-step procedure or set of rules designed to solve a specific problem or perform a specific task. Algorithms have certain attributes or characteristics that define their behaviour and performance. An algorithm is a set of well-defined instructions that take specific inputs and produce predictable outputs consistently. It operates for a finite number of iterations and is precisely defined without ambiguity. The efficiency of an algorithm is determined by how effectively it utilizes computational resources like time and memory. Accuracy depends on the correctness of instructions and the validity of inputs. Modularity is crucial in algorithm development, facilitating reusability, maintainability, and easier testing and debugging. Scalability allows an algorithm to handle problems regardless of their dimensionality, while adaptability enables redesigning to address different scenarios. Algorithm design Step 01: Pre-requisites Define the problem and identify the inputs and the expected outputs. Step 02: Algorithm Design This can be done using flow charts or pseudo codes. Step 03: Testing and Implementing. Testing the algorithm and then implement. Data analytics Descriptive Analytics Descriptive analytics focuses on understanding and summarizing historical data to gain insights into past events and trends. Primary goal - to answer, "what happened?" by analyzing and presenting data in an easily interpretable manner. Steps in Descriptive Analytics: 1.Data Processing: Collection: Gather data from various sources (e.g., databases, files, surveys). Validation: Ensure data accuracy, completeness, and consistency, including cleansing and removing errors or duplicates. Sorting: Arrange data based on criteria like alphabetical, numerical, or chronological order. Transformation: Convert data into a standardized format or structure, including normalization and aggregation. 2.Descriptive Statistics: Combine data points to create summary statistics (e.g., sum, average, count) and higher-level representations. 3.Reporting and Visualization: Executive dashboards Companies are increasingly moving away from traditional paper reports towards Executive Dashboards, which offer a more dynamic and visually engaging way to present current data. These dashboards display information through pictures, graphs, and tables, helping managers make informed decisions quickly. Provide greater flexibility, allowing managers to customize reports to meet their specific needs more effectively Examples: 1.Retail Chain Dashboard: KPIs: Sales figures, average transaction value, foot traffic. Charts/Graphs: Sales trends over time, sales distribution by store. Maps: Store locations and performance metrics by region. Alerts: Notifications about stores falling below sales targets. 2.Healthcare Dashboard: KPIs: Patient satisfaction scores, average wait times, bed occupancy rates. Charts/Graphs: Patient outcomes over time, department performance. Maps: Geographic distribution of patients or resource availability. Alerts: Warnings about critical equipment levels or patient wait times exceeding thresholds. Drill down reports Enhance dashboards by allowing users to explore data at increasing levels of detail. Helps users start with a broad overview and then focus on more specific information as needed, avoiding information overload at the PROVIDE PROGRESSIVE START WITH HIGH-LEVEL outset. EXAMINATION OF DATA OVERVIEW, DRILL DOWN TO SPECIFICS Example: A sales manager uses a dashboard to view company-wide sales data. If the manager notices a decline in overall sales, they can drill down to see sales by department to identify which areas are underperforming. Further drilling down allows the manager to examine EMPOWER MANAGERS TO CONTROL DETAIL sales by individual sales representatives within LEVEL the problematic departments. This structured approach enables the manager to pinpoint specific issues and make targeted decisions. Exceptional reports Used to highlight unusual or critical situations that require management action, reducing information overload. Crucial for avoiding either too many or too few reports. Generated based on predefined Highlight situations requiring Focuses on important performance aspects parameters management action and KPIs. Ensures that the system meets individual data needs effectively. Example: If a company sets an exception report to flag any project that exceeds its Parameters must be budget by over $100,000, only projects with carefully set to avoid overload significant budget overruns will be reported. If set too low, the manager might receive too many reports about minor issues; if set too high, important issues might be missed. Presentation of reports Presentation: A well-presented report that is clearly structured and logically ordered enhances professionalism and readability, making it more likely to be well-received. Standard Format: Adhering to 'house style rules' for report formatting promotes a consistent corporate image and facilitates comparison between reports. It also ensures that key information is easily identifiable. Length: Reports should be appropriately detailed for their purpose. Summary reports should be concise, while detailed reviews should be comprehensive, ensuring that all included material is relevant and purposeful. Data Storage: Properly storing and organizing processed data in databases, data warehouses, or cloud systems ensures that it is accessible for future retrieval and reference. Example: A company uses a standard format for all its monthly performance reports, ensuring they are presented clearly and consistently. Summary reports for executives are kept brief with key metrics highlighted, while detailed reports for analysts include comprehensive data. Archived data is organized in a central database, making it easy for users to access historical performance information as needed. Data warehousing and improved reporting A data warehouse is a centralized repository that integrates data from various internal and external sources, allowing for comprehensive management analysis and decision- making. It enables detailed, multidimensional data analysis using tools like OLAP, which lets users examine data from different perspectives (e.g., product, region, time). Organizations can either build a single data warehouse for the entire organization or create smaller, purpose-specific data marts. Data Marts Quicker and cheaper to build, too many can complicate data management, making a single data warehouse more efficient in some cases A smaller, purpose-specific subset of data, easier and quicker to build than a full data warehouse. Example: A data mart focused on sales data for a specific region. Single Data Warehouse vs. Multiple Data Marts A single warehouse may be more efficient than multiple data marts. Example: Instead of having separate data marts for each department, a central warehouse can provide a unified view for the entire organization. https://www.youtube.com/watch?v=AHR_7jFCMeY Advantages of setting up a data warehouse system Decision makers can access data without affecting the use of operational systems. Having a wide range of data available to be queried easily encourages the taking of a wide perspective on organisational activities. Data warehouses have proved successful in a number of areas: Quantifying the effect of marketing initiatives Improving knowledge of customers Identifying and understanding an enterprise's most profitable revenue streams Information can be made available to business partners. For example, if customer sales order information is in the data warehouse, it could be made available to customers and even suppliers. Internal information on products and services could also be provided. Predictive analysis Predictive analytics is the practice of using historical and current data, statistical algorithms, and ML techniques to analyze patterns and make predictions about future events or outcomes. Example: Retail Inventory Management A retail company uses predictive analytics to optimize inventory levels. By analyzing historical sales data, customer purchasing patterns, and external factors like seasonal trends and promotions, the company can forecast future product demand. Historical Data: The company examines past sales data to understand patterns in customer buying behavior. Statistical Algorithms: Advanced statistical models are used to identify trends and correlations. Machine Learning (ML): ML algorithms predict future demand based on factors such as upcoming promotions, holidays, and weather conditions. Outcome: The company anticipates higher demand for winter clothing as the season approaches and adjusts inventory levels accordingly. This helps in reducing overstock and stockouts, leading to better customer satisfaction and optimized inventory costs. Machine Learning Modeling Data Acquisition Gathering relevant data from various sources, such as databases, sensors, social media, or customer interactions. This data may include structured data (numbers, categories) and unstructured data (text, images) Data Pre-processing Cleaning and preparing the data for analysis. This step involves tasks such as data cleaning handling missing values, data transformation, and feature engineering (creating new variables or features that may enhance prediction accuracy) Exploratory Data Analysis (EDA) Analysing and visualizing the data to gain insights, identify patterns, and understand the relationships between variables. EDA helps in selecting relevant features and understanding the data's characteristics. Machine Learning (ML) Model Selection: involves choosing the right algorithm or technique based on the problem and the data characteristics. Machine learning, a subset of AI, develops algorithms that enable computers to learn from data and make predictions or decisions without explicit programming for each task. The goal is to improve performance on specific tasks through experience and data exposure. Unsupervised Learning Deals with unlabeled data, Reinforcement Learning Supervised Learning where the algorithm explores Involves agents learning to Models learn from labeled data, data structure or patterns make decisions by interacting making predictions or decisions without explicit output labels. It with an environment and based on input-output pairs. aims to identify meaningful receiving feedback or rewards. patterns or groupings. Model Training: Training Data vs. Test Data Example: For a model predicting house Training Data: This is used to teach the model. prices, the training data might include features like the number of bedrooms It includes input features and their and square footage, along with the corresponding labels. The model learns from actual sale prices of these houses. The this data by adjusting its parameters to model uses this information to learn improve prediction accuracy. how to predict prices based on these features. Example: After training the house price prediction model, test data might Test Data: This is used to evaluate how well the include new house features (e.g., model performs on new, unseen data. It number of bedrooms and square includes input features but the outputs (labels) footage) without the actual sale prices. are withheld during the evaluation. The model’s predictions are compared to the actual sale prices (hidden during testing) to measure its accuracy. Classification Algorithms In supervised learning, training data is used to train the ML model, while test data assesses its performance; classification algorithms categorize data into predefined groups based on learned patterns from training data, including; Support Vector Machine (SVM) Decision trees K-nearest neighbor (KNN) Naïve Bayes Predictive analysis Model Evaluation: Assess the trained model’s performance using metrics such as accuracy, precision, recall, F1-score, or AUC To determine the model’s effectiveness and prediction potential. Model Tuning: Iteratively adjust the model’s parameters or configurations. Techniques Includes cross-validation, hyperparameter tuning, and ensemble methods. To optimize performance and improve accuracy and generalization. Predictions and Deployment: Apply the trained model to new or unseen data to generate forecasts or predictions. Applications: Customer behavior analysis, risk assessment, demand forecasting, fraud detection, and personalized recommendations. Implement the model in a production environment for real-time predictions. Monitoring and Maintenance Continuously track model performance and retrain with new data as needed. To detect and address model decay or drift and ensure ongoing accuracy. Predictive Analytics Applications: Industries: Finance, marketing, healthcare, manufacturing, and supply chain management. Optimizes processes, improves decision-making, identifies opportunities, mitigates risks, and enhances operational efficiency. Predictive analytics provides probabilistic insights rather than absolute certainty. Accuracy relies on data quality, modeling techniques, and assumptions.